ABSTRACT
Food security, self-sufficiency level and pursuance of environmental stewardship have long been emphasized as the mainstay of Malaysia’s national agendas for development. Achieving all three essentials requires an agricultural sector that is robust to vagaries of nature and market. Current global trends have seen the focus on production and manufacturing shifting towards technologies related to the Industrial Revolution 4.0 (IR4.0). In its complexity and high potential, IR4.0 harnesses together cyber physical systems, internet of things, cloud computing and cognitive computing that allows production and manufacturing systems to be flexible, customizable and robust. This, in turn, allows efficient utilization of resources, reducing waste, provision of traceability and increasing productivity. When applied to agricultural systems, uncertainties in production systems that are based on heuristic and geo-spatial and temporal variables can now be quantified in order for all decisions to be based on empirical data and dynamic models. MARDI’s agricultural research and development (R&D) efforts along the agro-food value chain can be consolidated in a framework that converges all necessary data with the underlying goal of reducing agricultural input, increasing productivity and modernizing the agro-food industry. Subsequently, all R&D activities would then be organized based on a defined cyber physical system (CPS) level, including assessment of current technologies, as well as projection of subsequent technologies to achieve Industry 4.0 standards. This paper provides a snapshot and an outlook into recent research in this field. A strong and relevant R&D output in this field will allow MARDI to be the focal point in reforming Malaysia’s agricultural sector.
Keywords: Sustainable agriculture, food security, industrial revolution 4.0, agro-food production
INTRODUCTION
Malaysia launched its Industry 4.0 (IR4.0) blueprint, “Industry 4WRD: National Policy on Industry 4.0” in October 2018, focusing on the digital transformation of the manufacturing and services related sectors (Ministry of International Trade and Industry (MITI) 2018). However, unlike similar initiatives in Indonesia (Making Indonesia 4.0) and Thailand (Thailand 4.0), food production and in general, the agriculture sector that supports it was merely given a peripheral emphasis. Far from being an oversight, this is consistent with the far bigger contributions of both manufacturing (23%) and services (54.5%) sectors to the national gross domestic products (GDP) (DOS 2018). Nevertheless, despite contributing only 8.2% to the GDP, the agriculture sector plays an integral role in the national socio-economic development agenda, particularly alleviating poverty, ensuring economic equality, food safety and security, as well as sustainability, as prescribed in the Sustainable Development Goals (SDGs) (FAO 2018).
Malaysia aspires to achieve high-incomed nation status by 2020. This is reflected in the sustainable development structure that has been the theme of Malaysia’s five-year economic national development plan. This entails encompassing economic growth with a balanced and equitable growth distribution across all levels of society, ensuring societal well-being including access to education, healthcare, infrastructure and utilities, as well as emphasizing on environmental conservation (EPU 2017). Furthermore, recent agendas are also geared toward complying with the triple-bottom line elements within the SDGs framework, such as Malaysia’s New Economic Model (NEM), where pursuance of sustainable development is tailored toward achieving high income, inclusivity and sustainability. This is achievable through development of food systems that are inclusive, sustainable, efficient, nutritious and healthy.
In the 11th Malaysian Plan (2016-2020), the agricultural sector requires transformation and modernization to ensure food security, increasing productivity, increasing skillsets of farmers, strengthening agro-food supply chain, as well as also enhancing related support and delivery service for all stakeholders. This sector contributed 8.2 percent of the RM1.2 trillion (USD314.5 billion) national Gross Domestic Product (GDP), with oil palm (46.6%) and other agriculture including agro-food sector (18.6%) as its main contributors (DOS 2018). Agriculture trade exports for 2017 was at a surplus of RM31 billion (USD8.2 billion), where paddy production was recorded at 2.6 million metric tons. It is also important to point out that this industry is heavily assisted and subsidized, thus putting a severe implication to its cost and productivity on the backdrop of marginal increase of yield (Kari 2018; Khazanah Research Institute 2019c). Issues such as food availability, food consumption trends, farm labor and demography as well as food waste offer an insight into the overall food system scenario in Malaysia and how it is primed for revamp and restructuring of its fundamentals. It is prudent that any discourse on how IR4.0 elements can be integrated into the agriculture sector should also focus on elements other that the national policies that necessitates it.
Based on recent trends, local food production and imports of certain food groups have contributed towards a more than sufficient dietary energy per capita that points towards food being available and accessible (Sundaram and Tan 2019). However, Sundaram and Tan (2019) also posits that recalibration of national food policies must be done in order to achieve affordability, diversity and safety, as well as food systems that provide adequate nutrition especially for the most vulnerable communities. These communities are more exposed to surges in prices brought by volatilities in the market or adverse and unmitigated environmental impacts on the food system. As Malaysia’s population is projected to increase further by 45% in 2050, increasing from 30.7 million to 44.4 million (UN DESA 2018), more burden will be placed on the existing food system to meet demands.
Existing food systems are also inefficient towards reducing food waste. From a production of about 1.6 million in 2014, an estimated of 3 to 20 percent was lost due to issues related to post harvest losses (New Straits Times 2016). These losses were attributed to deficiencies in post-harvest management and processing practices (Tengku Muda Mohamed 2017) borne out of inadequate knowledge, training and information (Yacob 2009). Losses can also be stymied through a dynamic distribution system that can efficiently move products through its supply chain, as well as systems with shorter supply chain, particularly for more perishable products, where it is located closer to its market.
Figure 1 summarizes this temporal change in food consumption trends, where total calorie intake per capita has considerably shifted form a rice dominated diet to a more varied protein-based diet. This increase in consumption and diversity is however accompanied by a downward trend in per capita production growth, pointing towards a heavy reliance on food imports (Remans et al. 2015). Malaysia is also experiencing a progressively ageing farming demography. Coupled with urban migration and negative appeal of agricultural jobs among youth, this sector is also reliant on foreigners, where about 611,000 foreign laborers working locally in 2017 (DOS 2018).
A myriad of data across the value chain can be harnessed and utilized to form a data-driven agro-food sector capable of not only addressing aforementioned issues. It will also galvanize the sector with technology, thus ensuring Malaysia continues to be integrated with the global economy, making economic progress and subsequently converging with other high-income countries (Khazanah Research Institute 2017).
This paper aims to project the narrative of how implementation of IR4.0 principles within an Agriculture 4.0 framework can be applied to Malaysia’s agro-food sector, through the proposed conceptual Agriculture 4.0 framework, as well as a snapshot of the pull and push factors involved and evaluation of challenges in data chain for existing and related technologies.
BIG DATA WITHIN MALAYSIA’S CONCEPTUAL AGRICULTURE 4.0 FRAMEWORK
In a nutshell, IR4.0 is characterized by the advent of cyber-physical systems that converges physical and digital entities, resulting in both humans and cyber systems to be inextricably connected and undistinguishable. Ultimately, this “singularity” becomes possible due to the ubiquity and exponential progression of innovation and technologies, allowing for smart autonomous devices to engage with each other, as well as achieving high level coordination (EDB 2017; Zambon et al. 2019). Driven by advancement of technologies such as Big Data, Cloud Computing, Machine Learning, Digital Twins and Internet of Things (IoT), IR4.0 permits production systems to gain competitive advantage through increased productivity, flexibility, efficiency and customization. A key currency in this intricate collection of cyber-physical systems is diverse and complex characteristic datasets or Big Data, that represents conditions, parameters, identities and observations collected across the value chain. Big Data can be further divided into three main categories, based on how it was generated; Process-mediated (PM) or traditional business data that is collected from business events of interest (purchasing, feeding, orders, etc); Machine-generated (MG) data collected from various sensors and devices used in the process, typically embedded into IoT systems; and Human-sourced (HS) data that is sourced from records of human experiences, in forms such as texts, videos, photographs and audio logs, either in physical forms such as books or digitized in computers and social media (UNECE 2013; Devlin 2012).
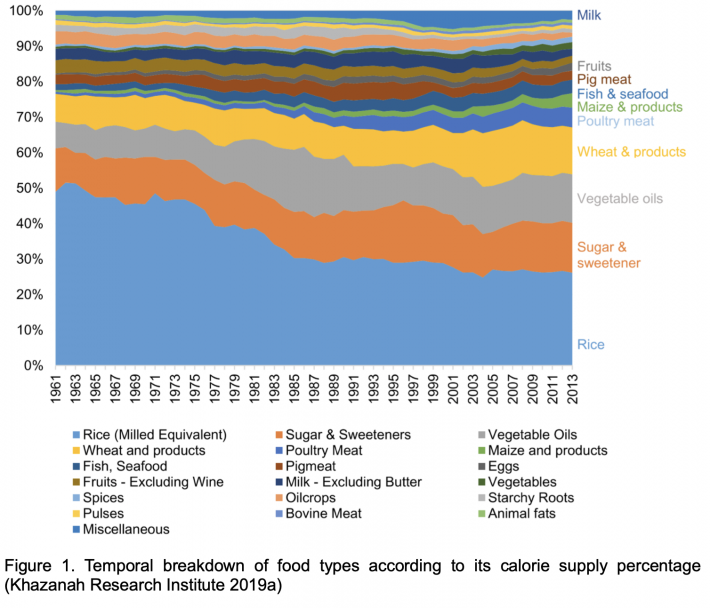
When applied to an agricultural framework, harnessing Big Data using IR4.0 allows farm managers to improve efficiencies across the supply chain, including transforming abilities for real-time forecasting, tracking of physical items and reinventing business processes (Esmeijer et al. 2015; Chen, Mao, and Liu 2014; Devlin 2012). This is achieved through benchmarking, sensor deployment and analytics and using predictive modelling that will then provide predictions of future yields, better farming models, facilitate real-time business decisions and simplify business processes (Devlin 2012). The technologies involved in this framework is categorized further into three main pillars; Automation, Connectivity and Intelligence (EDB 2017; Ministry of International Trade and Industry (MITI) 2018). In a typical farming process, the five main nodes that represents stages of production are locus preparation, production, maintenance, harvesting and post-harvest processes. Visualization of the strategies needed to plan, prepare and provide for an Agriculture 4.0 framework can be done by juxtaposing the technology pillars to these nodes (Figure 2). Automation involves application of technologies that monitor, control and execute processes related to manufacturing of a product or provision of a service. Automation options must be flexible to allow customization of a myriad of farm processes, including seeding, weeding, cutting, harvesting, storing and packing for different types of
product with different sets of physical characteristics. In terms of Connectivity, it refers to the interconnectedness between devices within the system that will allow communication, engagement and data exchange. In this Farm IoT system, data from sensors that monitor conditions on the farms are sent to a cloud-based computing platform, where built in algorithms supported by other data from weather stations, real-time pricing or market demands, will decide upon essential farm decisions such as when to apply pesticides, irrigation or to harvest. This action is carried out by actuators that enables the automation processes discussed earlier. The Intelligence pillar refers to the process of analyzing data collected from various sources that will be processed into actionable decisions. It also refers to the ability of the platform to learn (Machine Learning) from decisions made and related algorithms built in, that will assist farm management to be done accurately and efficiently.
At this juncture, research and development (R&D) is necessary to spur integration of technologies related to these three pillars and its congruent data flow along the value chain. This also must continue preferably with the contribution and collaboration of regulators/agencies and the private sector (Khazanah Research Institute 2019b). As in Figure 3, R&D should proceed along the lines set by the three pillars along the production chain. By identifying related pillars within this chain and how data is managed within it, R&D activities can be more focused. It can be seen that MG data from farms are collected and pushed up to cloud-based platforms for analysis to monitor and determine yield and harvest times. This data is then augmented with PM data as the product moves to post-harvest and to the customers for quality control and traceability and customer satisfaction. HS data from customers are then analyzed with PM data either through agencies and regulators in order to determine food availability, affordability and safety, that would then be regurgitated back into the production system.
In this regard, the Malaysian Agriculture Research and Development Institute (MARDI) plays an integral role in the technology value chain, bringing innovations in agriculture, from new resilient crop varieties and livestock breeds, biotechnology derived pest and disease management methods, as well as engineering solutions for all three pillars within the Agriculture 4.0 framework. An existing body of research is available particularly for efforts in mechanization and automation in the form of combine designs, machines and food processing equipment, as well as controlling variabilities in large farms using precision farming and remote sensing techniques. Progression into Agriculture 4.0 requires strategies that leverages existing assets with an eye towards pushing the boundaries in agricultural solutions in tandem with the exponential development of ‘Transformative Twelve’ technologies such as alternative proteins, improved food sensing technologies used in food safety, quality and traceability, nutragenics for personalized nutrition, Big Data and advanced analytics, IoT and blockchain-enabled real time supply chain and traceability, precision agriculture for input and water use optimization, gene editing of multi-trait seed improvement, microbiome-enhanced crops, as well as new renewable energy alternatives (World Economic Forum and McKinsey & Company 2018). MARDI’s effort can be summarized as seen in Figure 4, where the foundation is already in place in order for Agriculture 4.0 solutions to address current issues in the agricultural sector.
PUSH & PULL FACTORS: TECHNOLOGY PERSPECTIVE
Push and Pull factors (Figure 5) will influence how much Agriculture 4.0 technology adoption and integration can be made across the food production system. Pull factors can be described as the need of these technologies in order to achieve certain goals, whereas Push factors are the enablers that will allow people or organizations to achieve higher or new goals (Wolfert et al. 2017). As discussed previously, policies and public interests are main facets of Pull factors involved in mainstreaming Agriculture 4.0 in Malaysia. Existence of these accommodating policies and increasing public interest will increase demand for solution that addresses perennial agricultural and food production issues. Similar to agricultural practices elsewhere, the scenario in Malaysia’s can be defined by its reliance on heuristic experience-based methods, where there is a high degree of supply chain uncertainty and lack of predictability, with regards to environmental factors, stochastic events, soil nutrient dynamics and pest infestation (Zambon et al. 2019; Ge, Gray, and Nolan 2015). In other words, tacit data of farmers experience and skill are not easily quantified, understood and replicated to ensure efficiency and productivity. Thus, addressing this need and providing platforms to efficiently utilize data produced by agriculture and food production systems goes beyond Pull factors derived from policies and public interests, but also Push factors of related technologies available and in development.
As discussed in the previous chapter, existing technologies such as precision farming, unmanned aerial vehicles (UAV) and mechanization and automation solutions can be harnessed with emerging technologies such as a higher capacity 5G network, IoT modules, Blockchain and robotic implements. MARDI is currently expanding its research and evaluation of precision farming techniques for paddy, where several technologies such as methods and equipment for water management, precision land levelling, variable rate (VRT) seeding and fertilization, early warning systems for detection of pest and disease, as well as yield monitoring sensors (Figure 6). In land preparation, a flat topography is ideal in ensuring uniform crop establishment and providing adequate protection from weed growth when the fields are flooded. GIS-based land survey is used to map out land level features and to obtain the land level index to be used in the seeding map. Levelling index is then calculated (Agarwal and Goel 1981), where each 10 x 10m grid are designated with either ‘even’, ‘high’ or ‘low’ notations in the seeding map. Seeding is done based on the land levels observed by the model, where 10 kg is added to the 120kg/ha seeding rate for grids that are designated with ‘low’. VRT is used to produce more consistent crop establishment compared to conventional broadcast seeding (Abu Bakar et al. 2017).
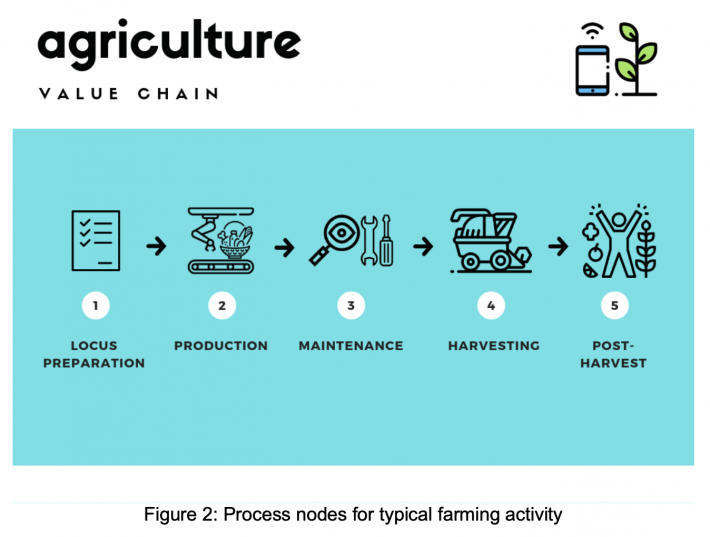
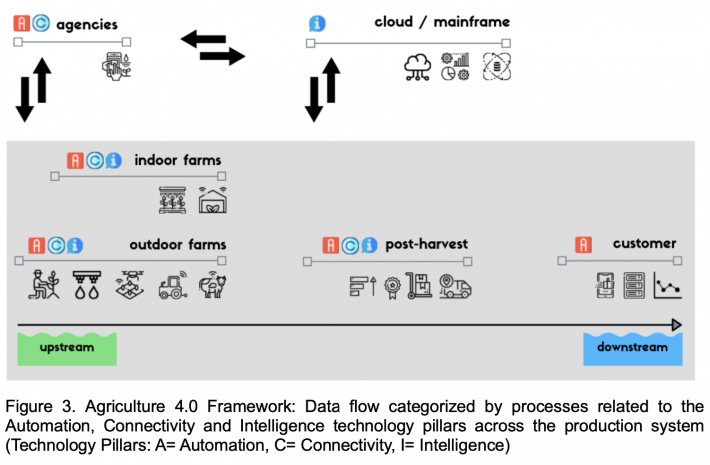
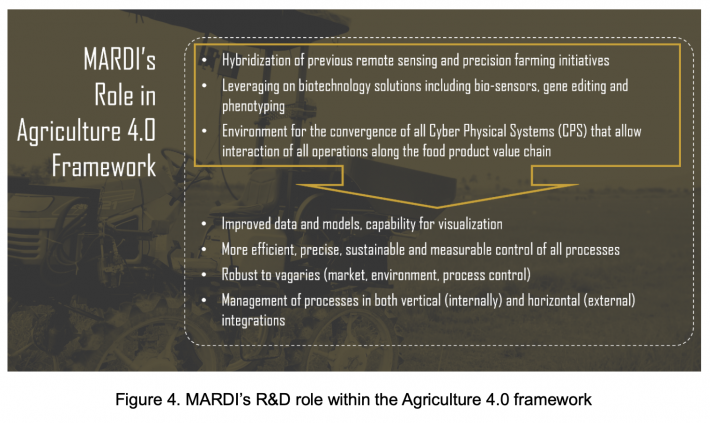
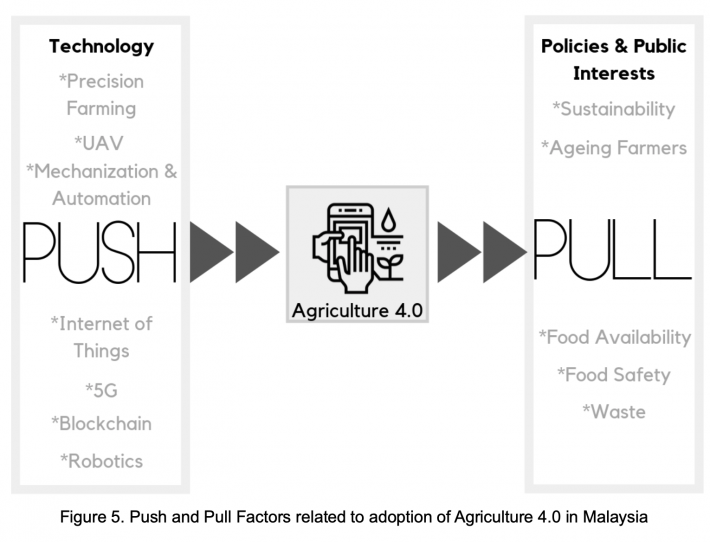
Fertilizer application with VRT provides nutrients specifically to the plant’s requirement. Conventionally, this is done on the field by blanket spreading without discrimination and reference to the plant’s condition, as well as available soil nutrient content. This eventually will result in uneven application of fertilizer, either under supplying required nutrients to the plants that will adversely affect plant growth, or oversupplying it, causing increased input cost and harmful environmental effects. MARDI’s VRT Fertilizer system is based on Green Area Index (GAI) model that evaluates soil fertility and plant condition. This system includes a GIS-based mapping system that is processed and analyzed to develop Soil Plant Analysis Development (SPAD) and GAI maps. A digital GAI treatment map will then be produced and then compiled into a geospatial and temporal representation of soil fertility for subsequent planting seasons. This system was observed to save 17% of total fertilization cost with an increase of about 20% efficiency over conventional fertilization methods. Evaluation of these technologies is done on a 60-ha paddy plot in FELCRA Seberang Perak, where preliminary figures have shown improved agricultural input utilization without significant loss in yield. Data collected in these studies will be used to improve accuracies and assumptions, thus increasing the likelihood of adoption among farmers that value higher profits from more efficient and precise use of resources.
Beyond paddy, MARDI is also conducting R&D for applications in Plant Factory with Artificial Lighting (PFAL). This setup not only allow plants such as vegetables and fruity plants to receive nutrients, moisture and lighting in a controlled manner, but also provides a clean and pest-free environment for plants to thrive. High quality and yield are distinctive features of PFAL systems, and if it is erected in vacant building spaces in urban areas, significantly shortening the supply chain and ensuring fresh, high quality, nutritious and pesticide-free produce is supplied to surrounding communities. Research in this area that is conducted by MARDI includes generating innovations and integration of available technologies in areas of building structure, seeding system, multi-tier hydroponic system, LED lighting design, IoT based control and monitoring system, Standard Operating Procedures (SOP) for management of pest and disease, post-harvest management system and waste management (Sayuti, Ahmad, and Kassim 2019). Application and integration of robotic actuators for automation is also being conducted in MARDI. This robot assisted harvesting system consists of a collaborative robot (COBOT), 3D camera and soft grippers for application in harvest of tomatoes and melons. This 3D camera will provide essential harvest data such as color and shape, that will then be analyzed using machine learning to determine optimal harvest traits. When paired with a gantry system (Figure 7), the harvesting automation cycle is completed by employing a gantry system to deliver plant pots to the harvesting robot and back.
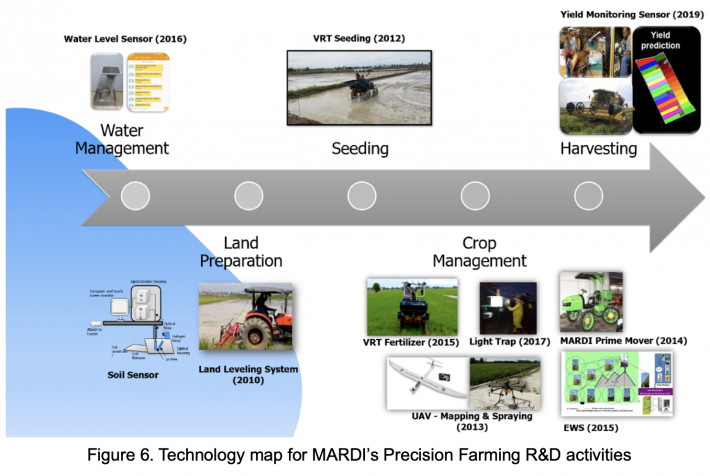
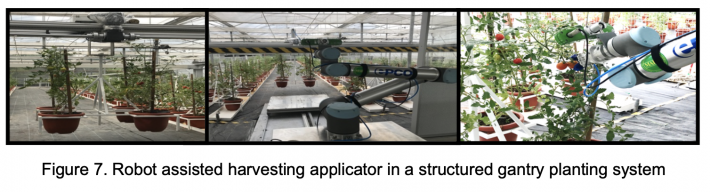
Observing the interest of mushroom as meat substitutes, MARDI is involved in R&D for high capacity production of mushroom in a controlled environment. Similar to PFAL, these mushroom houses, offer a suitable environment for mushrooms to be produced with consistent quality and yield, by controlling parameters such as temperature, relative humidity, carbon dioxide levels, and air flow. Other than integrating IoT applications to digitize data for monitoring and control, research is also done to identify suitable mushroom varieties that have high commercial value and/or high nutrient content.
Advancement in telecommunications industry will further boost application of Agriculture 4.0 and Big Data in Malaysia. Policy makers in Malaysia is aggressively pushing for implementation of 5G initiatives with the objective in the technology pioneer in this region (Malay Mail 2019; New Straits Times 2019a). A 5G taskforce was formed with participation of policy makers, industry and academia as a platform for discourse, and recently numerous trial centers and testbeds deployments were made in selected areas to provide demonstration of the network’s capabilities. With Blockchain capabilities imbedded into the food production system, market participants, authorities and consumers will have access to transparent and decentralized information on food such as its origins, agriculture practice and price. DSTE CHAIN was launched by a home-grown agro-tech firm, DSTE Capital Limited, to provide Blockchain-based Distributed Ledger Technologies (DLT) service for the agricultural chain, where data of farmers, plantation, logistics, to food processing, transportation, retail and consumer can be trace to ensure transparency and increase efficiency of food production (Sun Media Corporation 2019)
CHALLENGES OF BIG DATA CHAIN IN THE MALAYSIAN LANDSCAPE
According to the findings and summary discussed by Wolfert et. al. (2017), adoption and utilization of Big Data involves both technical and governance issues across different stages of the chain, with more dominant governance challenges as it approaches the back-end of the chain. The situation in Malaysia appears promising in relation to potential of both technical and governance issues to provide room for enrichment. Table 1 summarizes the levels in regard to issues in each stage along the data chain. In this initial stage, R&D and strong participation of the industry, whether they are big agricultural companies (John Deer, Monsanto, Sime Darby, etc) or start-ups, must be congruent with existing policies and governance to offer necessary solutions that enable data to move through the chain. Currently, technologies related to data capture, storage and transfer in Malaysia Agricultural sector are moving in the right direction with research and applications of emerging technologies. Data capture, storage and transfer is limited only by the cost involved to acquire devices or availability of internet access.
Technologies related to the second half of the data chain stage remains at a lower level, as data is currently in silos and not readily accessible. Malaysia intends to introduce a Smart Farming Policy soon (New Straits Times 2019b), focusing on creating opportunities for funding. Indication of identifying data governance related to its sharing in the food production system is not available at this moment. Data ownership is currently privately held and is governed under the Personal Data Protection Act 2010 (PDPA), where data collected by companies or entities cannot be sold or shared to a third party without consent. Other related laws such as Communications and Multimedia Act 1998, the Computer Crimes Act 1997, and the Penal Code also subjects privacy and ownership of data to its originator and the initial intended use, where adoption and manipulation of these data requires consent. Proliferation can be enhanced in an open-sourced platform where adopters willingly share data. However, this requires a transparent and elaborate discourse to guide future policies in order to ensure privacy, ownership and control of data does not concentrate at a single party, rather it should be shared to all participants in the food production system. Often, public or technical discourses are conducted separately, since agriculture, science and technology, and communications sectors operate under its respective ministries. In order for an encompassing framework that prioritizes both technology and governance to be developed, unification of these interests must proceed in urgency.
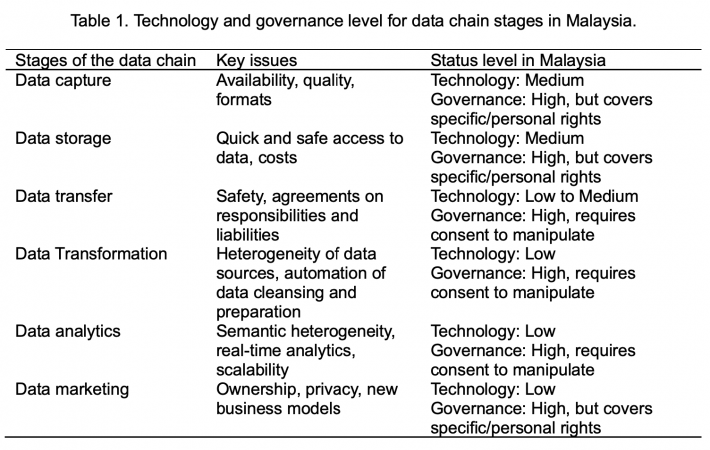
CONCLUSION
Malaysia is keen to embrace adoption of IR4.0 to galvanize its socio-economic development and achieve high incomed nation status. Agriculture and food production were seen as a main driver to achieve this. Existing policies were enacted to provide support for proliferation of technology uptake, in order to transform and modernize all elements in the value chain. Big Data, along with IoT and Blockchain are emerging technologies identified as vital facets that embodies the technologies required to achieve this transformation. A conceptual Agriculture 4.0 framework was proposed to strategize and map existing and necessary technologies related to the agriculture and food production system, according to the technology pillars of automation, connectivity and intelligence. Current adoption and integration of technologies seems low but promising for Agriculture 4.0 to move in the right transitional direction, however governance that ensure privacy and transparency of data used in this system should also be addressed and included in the discourse.
REFERENCES
Abu Bakar, B, M. A. M. Bookeri, S. S. Mohamad Ghazali, M. S. Abd. Rahman, M. T. Ahmad, and R. Abdullah, M. Z. K. Ismail. 2017. “Automated Land Leveling Index Determination for Variable Rate Seeding of Paddy.” In Proceedings of International Conference on Sustainable Development ICoSD 2017. Putrajaya, Malaysia.
Agarwal, M C, and A C Goel. 1981. “Effect of Field Levelling Quality on Irrigation.” Agric Water Manag 4: 457–64.
Chen, Min, Shiwen Mao, and Yunhao Liu. 2014. “Big Data: A Survey.” Mobile Networks and Applications 19 (2): 171–209. https://doi.org/10.1007/s11036-013-0489-0.
Devlin, Barry. 2012. “The Big Data Zoo--Taming the Beasts: The Need for an Integrated Platform for Enterprise Information.” Cape Town: 9sight Consulting.
DOS. 2018. “Malaysia Selected Agricultural Indicators.” 2018. https://www.dosm.gov.my/v1/index.php?r=column/cthemeByCat&cat=72&bul_id=....
EDB, Singapore Economic Development Board. 2017. “The Singapore Smart Industry Readiness Index.”
EPU, Economic Planning Unit. 2017. “Malaysia Sustainable Development Goals Voluntary National Review 2017.”
Esmeijer, Jop, Tom Bakker, Merel Ooms, Bas Kotterink, and Sponsor Ministerie van Economische Zaken. 2015. “Data-Driven Innovation in Agriculture: Case Study for the OECD KBC2-Programme.” TNO Report TNO, R10154.
FAO. 2018. “Transforming Agriculture Food And To Achieve The SDGs.”
Ge, Houtian, Richard Gray, and James Nolan. 2015. “Agricultural Supply Chain Optimization and Complexity: A Comparison of Analytic vs Simulated Solutions and Policies.” International Journal of Production Economics 159 (June 2019): 208–20. https://doi.org/10.1016/j.ijpe.2014.09.023.
Kari, Fatimah. 2018. Evaluation of Agricultural Subsidies and Welfare of Rice Farmers.
Khazanah Research Institute. 2017. “The Times, They Are Changing: Technology, Employment, and the Malaysian Economy.” Technical Services Quarterly. Vol. 34. https://doi.org/10.1080/07317131.2017.1286838.
———. 2019a. “The Fourth Industrial Revolution & Our Food ( Part 1 ).”
———. 2019b. “The Fourth Industrial Revolution & Our Food ( Part 3 ) Driving Agritech Development.”
———. 2019c. “The Status of the Paddy and Rice Industry in Malaysia.”
Malay Mail. 2019. “Malaysia on Track to Become Potential 5G Pioneer in Region, Say Experts | Malaysia | Malay Mail.” 2019. https://www.malaymail.com/news/malaysia/2019/09/24/malaysia-on-track-to-....
Ministry of International Trade and Industry (MITI). 2018. “Industry4WRD.” 2018. https://www.miti.gov.my/index.php/pages/view/industry4WRD?mid=559.
New Straits Times. 2016. “Curbing Food Wastage at Source.” New Straits Times, 2016. https://www.nst.com.my/news/2016/06/151334/curbing-food-wastage-source.
———. 2019a. “5G Trials to Go Nationwide next Month: Gobind | New Straits Times | Malaysia General Business Sports and Lifestyle News.” 2019. https://www.nst.com.my/news/nation/2019/09/524145/5g-trials-go-nationwid....
———. 2019b. “Azmin: Smart Farming Policy Can Modernise Agricultural Landscape | New Straits Times | Malaysia General Business Sports and Lifestyle News.” 2019. https://www.nst.com.my/news/nation/2019/08/513824/azmin-smart-farming-po....
Remans, Roseline, Fabrice A. J. DeClerck, Gina Kennedy, and Jessica Fanzo. 2015. “Expanding the View on the Production and Dietary Diversity Link: Scale, Function, and Change over Time.” Proceedings of the National Academy of Sciences 112 (45): E6082–E6082. https://doi.org/10.1073/PNAS.1518531112.
Sayuti, Zulhazmi, Mohammad Abid Ahmad, and Rosniza Kassim. 2019. “Kilang Tanaman MARDI: Teknologi Pengeluaran Sayuran.”
Sun Media Corporation. 2019. “DSTE Brings Smart Farming Solution to Agriculture Industry.” 2019. https://www.thesundaily.my/spotlight/dste-brings-smart-farming-solution-....
Sundaram, Jomo Kwame, and Zhai Gen Tan. 2019. “Achieving Food Security for All Malaysians.”
Tengku Muda Mohamed, Mahmud. 2017. An Unsung Solution for Postharvest.
UN DESA. 2018. “World Population Prospects 2017.” 2018. https://population.un.org/wpp/Download/Standard/Population/.
UNECE. 2013. “Classification of Types of Big Data.” 2013. http://www1.unece.org/stat/platform/ display/bigdata/Classification+of+Types+of+Big+Data.
Wolfert, Sjaak, Lan Ge, Cor Verdouw, and Marc Jeroen Bogaardt. 2017. “Big Data in Smart Farming – A Review.” Agricultural Systems. https://doi.org/10.1016/j.agsy.2017.01.023.
World Economic Forum, and McKinsey & Company. 2018. “Innovation with a Purpose: The Role of Technology Innovation.” World Economic Forum, no. January: 1–42.
Yacob, Masturina. 2009. “POST HARVEST HANDLING PRACTICES ON SELECTED LOCAL FRUITS” 2: 39–53.
Zambon, Ilaria, Massimo Cecchini, Gianluca Egidi, Maria Grazia Saporito, and Andrea Colantoni. 2019. “Revolution 4.0: Industry vs. Agriculture in a Future Development for SMEs.” Processes 7 (1): 36. https://doi.org/10.3390/pr7010036.
Date submitted: October 29, 2019
Reviewed, edited and uploaded: November 28, 2019 |
Agriculture 4.0: Data-Driven Approach to Galvanize Malaysia’s Agro-Food Sector Development
ABSTRACT
Food security, self-sufficiency level and pursuance of environmental stewardship have long been emphasized as the mainstay of Malaysia’s national agendas for development. Achieving all three essentials requires an agricultural sector that is robust to vagaries of nature and market. Current global trends have seen the focus on production and manufacturing shifting towards technologies related to the Industrial Revolution 4.0 (IR4.0). In its complexity and high potential, IR4.0 harnesses together cyber physical systems, internet of things, cloud computing and cognitive computing that allows production and manufacturing systems to be flexible, customizable and robust. This, in turn, allows efficient utilization of resources, reducing waste, provision of traceability and increasing productivity. When applied to agricultural systems, uncertainties in production systems that are based on heuristic and geo-spatial and temporal variables can now be quantified in order for all decisions to be based on empirical data and dynamic models. MARDI’s agricultural research and development (R&D) efforts along the agro-food value chain can be consolidated in a framework that converges all necessary data with the underlying goal of reducing agricultural input, increasing productivity and modernizing the agro-food industry. Subsequently, all R&D activities would then be organized based on a defined cyber physical system (CPS) level, including assessment of current technologies, as well as projection of subsequent technologies to achieve Industry 4.0 standards. This paper provides a snapshot and an outlook into recent research in this field. A strong and relevant R&D output in this field will allow MARDI to be the focal point in reforming Malaysia’s agricultural sector.
Keywords: Sustainable agriculture, food security, industrial revolution 4.0, agro-food production
INTRODUCTION
Malaysia launched its Industry 4.0 (IR4.0) blueprint, “Industry 4WRD: National Policy on Industry 4.0” in October 2018, focusing on the digital transformation of the manufacturing and services related sectors (Ministry of International Trade and Industry (MITI) 2018). However, unlike similar initiatives in Indonesia (Making Indonesia 4.0) and Thailand (Thailand 4.0), food production and in general, the agriculture sector that supports it was merely given a peripheral emphasis. Far from being an oversight, this is consistent with the far bigger contributions of both manufacturing (23%) and services (54.5%) sectors to the national gross domestic products (GDP) (DOS 2018). Nevertheless, despite contributing only 8.2% to the GDP, the agriculture sector plays an integral role in the national socio-economic development agenda, particularly alleviating poverty, ensuring economic equality, food safety and security, as well as sustainability, as prescribed in the Sustainable Development Goals (SDGs) (FAO 2018).
Malaysia aspires to achieve high-incomed nation status by 2020. This is reflected in the sustainable development structure that has been the theme of Malaysia’s five-year economic national development plan. This entails encompassing economic growth with a balanced and equitable growth distribution across all levels of society, ensuring societal well-being including access to education, healthcare, infrastructure and utilities, as well as emphasizing on environmental conservation (EPU 2017). Furthermore, recent agendas are also geared toward complying with the triple-bottom line elements within the SDGs framework, such as Malaysia’s New Economic Model (NEM), where pursuance of sustainable development is tailored toward achieving high income, inclusivity and sustainability. This is achievable through development of food systems that are inclusive, sustainable, efficient, nutritious and healthy.
In the 11th Malaysian Plan (2016-2020), the agricultural sector requires transformation and modernization to ensure food security, increasing productivity, increasing skillsets of farmers, strengthening agro-food supply chain, as well as also enhancing related support and delivery service for all stakeholders. This sector contributed 8.2 percent of the RM1.2 trillion (USD314.5 billion) national Gross Domestic Product (GDP), with oil palm (46.6%) and other agriculture including agro-food sector (18.6%) as its main contributors (DOS 2018). Agriculture trade exports for 2017 was at a surplus of RM31 billion (USD8.2 billion), where paddy production was recorded at 2.6 million metric tons. It is also important to point out that this industry is heavily assisted and subsidized, thus putting a severe implication to its cost and productivity on the backdrop of marginal increase of yield (Kari 2018; Khazanah Research Institute 2019c). Issues such as food availability, food consumption trends, farm labor and demography as well as food waste offer an insight into the overall food system scenario in Malaysia and how it is primed for revamp and restructuring of its fundamentals. It is prudent that any discourse on how IR4.0 elements can be integrated into the agriculture sector should also focus on elements other that the national policies that necessitates it.
Based on recent trends, local food production and imports of certain food groups have contributed towards a more than sufficient dietary energy per capita that points towards food being available and accessible (Sundaram and Tan 2019). However, Sundaram and Tan (2019) also posits that recalibration of national food policies must be done in order to achieve affordability, diversity and safety, as well as food systems that provide adequate nutrition especially for the most vulnerable communities. These communities are more exposed to surges in prices brought by volatilities in the market or adverse and unmitigated environmental impacts on the food system. As Malaysia’s population is projected to increase further by 45% in 2050, increasing from 30.7 million to 44.4 million (UN DESA 2018), more burden will be placed on the existing food system to meet demands.
Existing food systems are also inefficient towards reducing food waste. From a production of about 1.6 million in 2014, an estimated of 3 to 20 percent was lost due to issues related to post harvest losses (New Straits Times 2016). These losses were attributed to deficiencies in post-harvest management and processing practices (Tengku Muda Mohamed 2017) borne out of inadequate knowledge, training and information (Yacob 2009). Losses can also be stymied through a dynamic distribution system that can efficiently move products through its supply chain, as well as systems with shorter supply chain, particularly for more perishable products, where it is located closer to its market.
Figure 1 summarizes this temporal change in food consumption trends, where total calorie intake per capita has considerably shifted form a rice dominated diet to a more varied protein-based diet. This increase in consumption and diversity is however accompanied by a downward trend in per capita production growth, pointing towards a heavy reliance on food imports (Remans et al. 2015). Malaysia is also experiencing a progressively ageing farming demography. Coupled with urban migration and negative appeal of agricultural jobs among youth, this sector is also reliant on foreigners, where about 611,000 foreign laborers working locally in 2017 (DOS 2018).
A myriad of data across the value chain can be harnessed and utilized to form a data-driven agro-food sector capable of not only addressing aforementioned issues. It will also galvanize the sector with technology, thus ensuring Malaysia continues to be integrated with the global economy, making economic progress and subsequently converging with other high-income countries (Khazanah Research Institute 2017).
This paper aims to project the narrative of how implementation of IR4.0 principles within an Agriculture 4.0 framework can be applied to Malaysia’s agro-food sector, through the proposed conceptual Agriculture 4.0 framework, as well as a snapshot of the pull and push factors involved and evaluation of challenges in data chain for existing and related technologies.
BIG DATA WITHIN MALAYSIA’S CONCEPTUAL AGRICULTURE 4.0 FRAMEWORK
In a nutshell, IR4.0 is characterized by the advent of cyber-physical systems that converges physical and digital entities, resulting in both humans and cyber systems to be inextricably connected and undistinguishable. Ultimately, this “singularity” becomes possible due to the ubiquity and exponential progression of innovation and technologies, allowing for smart autonomous devices to engage with each other, as well as achieving high level coordination (EDB 2017; Zambon et al. 2019). Driven by advancement of technologies such as Big Data, Cloud Computing, Machine Learning, Digital Twins and Internet of Things (IoT), IR4.0 permits production systems to gain competitive advantage through increased productivity, flexibility, efficiency and customization. A key currency in this intricate collection of cyber-physical systems is diverse and complex characteristic datasets or Big Data, that represents conditions, parameters, identities and observations collected across the value chain. Big Data can be further divided into three main categories, based on how it was generated; Process-mediated (PM) or traditional business data that is collected from business events of interest (purchasing, feeding, orders, etc); Machine-generated (MG) data collected from various sensors and devices used in the process, typically embedded into IoT systems; and Human-sourced (HS) data that is sourced from records of human experiences, in forms such as texts, videos, photographs and audio logs, either in physical forms such as books or digitized in computers and social media (UNECE 2013; Devlin 2012).
When applied to an agricultural framework, harnessing Big Data using IR4.0 allows farm managers to improve efficiencies across the supply chain, including transforming abilities for real-time forecasting, tracking of physical items and reinventing business processes (Esmeijer et al. 2015; Chen, Mao, and Liu 2014; Devlin 2012). This is achieved through benchmarking, sensor deployment and analytics and using predictive modelling that will then provide predictions of future yields, better farming models, facilitate real-time business decisions and simplify business processes (Devlin 2012). The technologies involved in this framework is categorized further into three main pillars; Automation, Connectivity and Intelligence (EDB 2017; Ministry of International Trade and Industry (MITI) 2018). In a typical farming process, the five main nodes that represents stages of production are locus preparation, production, maintenance, harvesting and post-harvest processes. Visualization of the strategies needed to plan, prepare and provide for an Agriculture 4.0 framework can be done by juxtaposing the technology pillars to these nodes (Figure 2). Automation involves application of technologies that monitor, control and execute processes related to manufacturing of a product or provision of a service. Automation options must be flexible to allow customization of a myriad of farm processes, including seeding, weeding, cutting, harvesting, storing and packing for different types of
product with different sets of physical characteristics. In terms of Connectivity, it refers to the interconnectedness between devices within the system that will allow communication, engagement and data exchange. In this Farm IoT system, data from sensors that monitor conditions on the farms are sent to a cloud-based computing platform, where built in algorithms supported by other data from weather stations, real-time pricing or market demands, will decide upon essential farm decisions such as when to apply pesticides, irrigation or to harvest. This action is carried out by actuators that enables the automation processes discussed earlier. The Intelligence pillar refers to the process of analyzing data collected from various sources that will be processed into actionable decisions. It also refers to the ability of the platform to learn (Machine Learning) from decisions made and related algorithms built in, that will assist farm management to be done accurately and efficiently.
At this juncture, research and development (R&D) is necessary to spur integration of technologies related to these three pillars and its congruent data flow along the value chain. This also must continue preferably with the contribution and collaboration of regulators/agencies and the private sector (Khazanah Research Institute 2019b). As in Figure 3, R&D should proceed along the lines set by the three pillars along the production chain. By identifying related pillars within this chain and how data is managed within it, R&D activities can be more focused. It can be seen that MG data from farms are collected and pushed up to cloud-based platforms for analysis to monitor and determine yield and harvest times. This data is then augmented with PM data as the product moves to post-harvest and to the customers for quality control and traceability and customer satisfaction. HS data from customers are then analyzed with PM data either through agencies and regulators in order to determine food availability, affordability and safety, that would then be regurgitated back into the production system.
In this regard, the Malaysian Agriculture Research and Development Institute (MARDI) plays an integral role in the technology value chain, bringing innovations in agriculture, from new resilient crop varieties and livestock breeds, biotechnology derived pest and disease management methods, as well as engineering solutions for all three pillars within the Agriculture 4.0 framework. An existing body of research is available particularly for efforts in mechanization and automation in the form of combine designs, machines and food processing equipment, as well as controlling variabilities in large farms using precision farming and remote sensing techniques. Progression into Agriculture 4.0 requires strategies that leverages existing assets with an eye towards pushing the boundaries in agricultural solutions in tandem with the exponential development of ‘Transformative Twelve’ technologies such as alternative proteins, improved food sensing technologies used in food safety, quality and traceability, nutragenics for personalized nutrition, Big Data and advanced analytics, IoT and blockchain-enabled real time supply chain and traceability, precision agriculture for input and water use optimization, gene editing of multi-trait seed improvement, microbiome-enhanced crops, as well as new renewable energy alternatives (World Economic Forum and McKinsey & Company 2018). MARDI’s effort can be summarized as seen in Figure 4, where the foundation is already in place in order for Agriculture 4.0 solutions to address current issues in the agricultural sector.
PUSH & PULL FACTORS: TECHNOLOGY PERSPECTIVE
Push and Pull factors (Figure 5) will influence how much Agriculture 4.0 technology adoption and integration can be made across the food production system. Pull factors can be described as the need of these technologies in order to achieve certain goals, whereas Push factors are the enablers that will allow people or organizations to achieve higher or new goals (Wolfert et al. 2017). As discussed previously, policies and public interests are main facets of Pull factors involved in mainstreaming Agriculture 4.0 in Malaysia. Existence of these accommodating policies and increasing public interest will increase demand for solution that addresses perennial agricultural and food production issues. Similar to agricultural practices elsewhere, the scenario in Malaysia’s can be defined by its reliance on heuristic experience-based methods, where there is a high degree of supply chain uncertainty and lack of predictability, with regards to environmental factors, stochastic events, soil nutrient dynamics and pest infestation (Zambon et al. 2019; Ge, Gray, and Nolan 2015). In other words, tacit data of farmers experience and skill are not easily quantified, understood and replicated to ensure efficiency and productivity. Thus, addressing this need and providing platforms to efficiently utilize data produced by agriculture and food production systems goes beyond Pull factors derived from policies and public interests, but also Push factors of related technologies available and in development.
As discussed in the previous chapter, existing technologies such as precision farming, unmanned aerial vehicles (UAV) and mechanization and automation solutions can be harnessed with emerging technologies such as a higher capacity 5G network, IoT modules, Blockchain and robotic implements. MARDI is currently expanding its research and evaluation of precision farming techniques for paddy, where several technologies such as methods and equipment for water management, precision land levelling, variable rate (VRT) seeding and fertilization, early warning systems for detection of pest and disease, as well as yield monitoring sensors (Figure 6). In land preparation, a flat topography is ideal in ensuring uniform crop establishment and providing adequate protection from weed growth when the fields are flooded. GIS-based land survey is used to map out land level features and to obtain the land level index to be used in the seeding map. Levelling index is then calculated (Agarwal and Goel 1981), where each 10 x 10m grid are designated with either ‘even’, ‘high’ or ‘low’ notations in the seeding map. Seeding is done based on the land levels observed by the model, where 10 kg is added to the 120kg/ha seeding rate for grids that are designated with ‘low’. VRT is used to produce more consistent crop establishment compared to conventional broadcast seeding (Abu Bakar et al. 2017).
Fertilizer application with VRT provides nutrients specifically to the plant’s requirement. Conventionally, this is done on the field by blanket spreading without discrimination and reference to the plant’s condition, as well as available soil nutrient content. This eventually will result in uneven application of fertilizer, either under supplying required nutrients to the plants that will adversely affect plant growth, or oversupplying it, causing increased input cost and harmful environmental effects. MARDI’s VRT Fertilizer system is based on Green Area Index (GAI) model that evaluates soil fertility and plant condition. This system includes a GIS-based mapping system that is processed and analyzed to develop Soil Plant Analysis Development (SPAD) and GAI maps. A digital GAI treatment map will then be produced and then compiled into a geospatial and temporal representation of soil fertility for subsequent planting seasons. This system was observed to save 17% of total fertilization cost with an increase of about 20% efficiency over conventional fertilization methods. Evaluation of these technologies is done on a 60-ha paddy plot in FELCRA Seberang Perak, where preliminary figures have shown improved agricultural input utilization without significant loss in yield. Data collected in these studies will be used to improve accuracies and assumptions, thus increasing the likelihood of adoption among farmers that value higher profits from more efficient and precise use of resources.
Beyond paddy, MARDI is also conducting R&D for applications in Plant Factory with Artificial Lighting (PFAL). This setup not only allow plants such as vegetables and fruity plants to receive nutrients, moisture and lighting in a controlled manner, but also provides a clean and pest-free environment for plants to thrive. High quality and yield are distinctive features of PFAL systems, and if it is erected in vacant building spaces in urban areas, significantly shortening the supply chain and ensuring fresh, high quality, nutritious and pesticide-free produce is supplied to surrounding communities. Research in this area that is conducted by MARDI includes generating innovations and integration of available technologies in areas of building structure, seeding system, multi-tier hydroponic system, LED lighting design, IoT based control and monitoring system, Standard Operating Procedures (SOP) for management of pest and disease, post-harvest management system and waste management (Sayuti, Ahmad, and Kassim 2019). Application and integration of robotic actuators for automation is also being conducted in MARDI. This robot assisted harvesting system consists of a collaborative robot (COBOT), 3D camera and soft grippers for application in harvest of tomatoes and melons. This 3D camera will provide essential harvest data such as color and shape, that will then be analyzed using machine learning to determine optimal harvest traits. When paired with a gantry system (Figure 7), the harvesting automation cycle is completed by employing a gantry system to deliver plant pots to the harvesting robot and back.
Observing the interest of mushroom as meat substitutes, MARDI is involved in R&D for high capacity production of mushroom in a controlled environment. Similar to PFAL, these mushroom houses, offer a suitable environment for mushrooms to be produced with consistent quality and yield, by controlling parameters such as temperature, relative humidity, carbon dioxide levels, and air flow. Other than integrating IoT applications to digitize data for monitoring and control, research is also done to identify suitable mushroom varieties that have high commercial value and/or high nutrient content.
Advancement in telecommunications industry will further boost application of Agriculture 4.0 and Big Data in Malaysia. Policy makers in Malaysia is aggressively pushing for implementation of 5G initiatives with the objective in the technology pioneer in this region (Malay Mail 2019; New Straits Times 2019a). A 5G taskforce was formed with participation of policy makers, industry and academia as a platform for discourse, and recently numerous trial centers and testbeds deployments were made in selected areas to provide demonstration of the network’s capabilities. With Blockchain capabilities imbedded into the food production system, market participants, authorities and consumers will have access to transparent and decentralized information on food such as its origins, agriculture practice and price. DSTE CHAIN was launched by a home-grown agro-tech firm, DSTE Capital Limited, to provide Blockchain-based Distributed Ledger Technologies (DLT) service for the agricultural chain, where data of farmers, plantation, logistics, to food processing, transportation, retail and consumer can be trace to ensure transparency and increase efficiency of food production (Sun Media Corporation 2019)
CHALLENGES OF BIG DATA CHAIN IN THE MALAYSIAN LANDSCAPE
According to the findings and summary discussed by Wolfert et. al. (2017), adoption and utilization of Big Data involves both technical and governance issues across different stages of the chain, with more dominant governance challenges as it approaches the back-end of the chain. The situation in Malaysia appears promising in relation to potential of both technical and governance issues to provide room for enrichment. Table 1 summarizes the levels in regard to issues in each stage along the data chain. In this initial stage, R&D and strong participation of the industry, whether they are big agricultural companies (John Deer, Monsanto, Sime Darby, etc) or start-ups, must be congruent with existing policies and governance to offer necessary solutions that enable data to move through the chain. Currently, technologies related to data capture, storage and transfer in Malaysia Agricultural sector are moving in the right direction with research and applications of emerging technologies. Data capture, storage and transfer is limited only by the cost involved to acquire devices or availability of internet access.
Technologies related to the second half of the data chain stage remains at a lower level, as data is currently in silos and not readily accessible. Malaysia intends to introduce a Smart Farming Policy soon (New Straits Times 2019b), focusing on creating opportunities for funding. Indication of identifying data governance related to its sharing in the food production system is not available at this moment. Data ownership is currently privately held and is governed under the Personal Data Protection Act 2010 (PDPA), where data collected by companies or entities cannot be sold or shared to a third party without consent. Other related laws such as Communications and Multimedia Act 1998, the Computer Crimes Act 1997, and the Penal Code also subjects privacy and ownership of data to its originator and the initial intended use, where adoption and manipulation of these data requires consent. Proliferation can be enhanced in an open-sourced platform where adopters willingly share data. However, this requires a transparent and elaborate discourse to guide future policies in order to ensure privacy, ownership and control of data does not concentrate at a single party, rather it should be shared to all participants in the food production system. Often, public or technical discourses are conducted separately, since agriculture, science and technology, and communications sectors operate under its respective ministries. In order for an encompassing framework that prioritizes both technology and governance to be developed, unification of these interests must proceed in urgency.
CONCLUSION
Malaysia is keen to embrace adoption of IR4.0 to galvanize its socio-economic development and achieve high incomed nation status. Agriculture and food production were seen as a main driver to achieve this. Existing policies were enacted to provide support for proliferation of technology uptake, in order to transform and modernize all elements in the value chain. Big Data, along with IoT and Blockchain are emerging technologies identified as vital facets that embodies the technologies required to achieve this transformation. A conceptual Agriculture 4.0 framework was proposed to strategize and map existing and necessary technologies related to the agriculture and food production system, according to the technology pillars of automation, connectivity and intelligence. Current adoption and integration of technologies seems low but promising for Agriculture 4.0 to move in the right transitional direction, however governance that ensure privacy and transparency of data used in this system should also be addressed and included in the discourse.
REFERENCES
Abu Bakar, B, M. A. M. Bookeri, S. S. Mohamad Ghazali, M. S. Abd. Rahman, M. T. Ahmad, and R. Abdullah, M. Z. K. Ismail. 2017. “Automated Land Leveling Index Determination for Variable Rate Seeding of Paddy.” In Proceedings of International Conference on Sustainable Development ICoSD 2017. Putrajaya, Malaysia.
Agarwal, M C, and A C Goel. 1981. “Effect of Field Levelling Quality on Irrigation.” Agric Water Manag 4: 457–64.
Chen, Min, Shiwen Mao, and Yunhao Liu. 2014. “Big Data: A Survey.” Mobile Networks and Applications 19 (2): 171–209. https://doi.org/10.1007/s11036-013-0489-0.
Devlin, Barry. 2012. “The Big Data Zoo--Taming the Beasts: The Need for an Integrated Platform for Enterprise Information.” Cape Town: 9sight Consulting.
DOS. 2018. “Malaysia Selected Agricultural Indicators.” 2018. https://www.dosm.gov.my/v1/index.php?r=column/cthemeByCat&cat=72&bul_id=....
EDB, Singapore Economic Development Board. 2017. “The Singapore Smart Industry Readiness Index.”
EPU, Economic Planning Unit. 2017. “Malaysia Sustainable Development Goals Voluntary National Review 2017.”
Esmeijer, Jop, Tom Bakker, Merel Ooms, Bas Kotterink, and Sponsor Ministerie van Economische Zaken. 2015. “Data-Driven Innovation in Agriculture: Case Study for the OECD KBC2-Programme.” TNO Report TNO, R10154.
FAO. 2018. “Transforming Agriculture Food And To Achieve The SDGs.”
Ge, Houtian, Richard Gray, and James Nolan. 2015. “Agricultural Supply Chain Optimization and Complexity: A Comparison of Analytic vs Simulated Solutions and Policies.” International Journal of Production Economics 159 (June 2019): 208–20. https://doi.org/10.1016/j.ijpe.2014.09.023.
Kari, Fatimah. 2018. Evaluation of Agricultural Subsidies and Welfare of Rice Farmers.
Khazanah Research Institute. 2017. “The Times, They Are Changing: Technology, Employment, and the Malaysian Economy.” Technical Services Quarterly. Vol. 34. https://doi.org/10.1080/07317131.2017.1286838.
———. 2019a. “The Fourth Industrial Revolution & Our Food ( Part 1 ).”
———. 2019b. “The Fourth Industrial Revolution & Our Food ( Part 3 ) Driving Agritech Development.”
———. 2019c. “The Status of the Paddy and Rice Industry in Malaysia.”
Malay Mail. 2019. “Malaysia on Track to Become Potential 5G Pioneer in Region, Say Experts | Malaysia | Malay Mail.” 2019. https://www.malaymail.com/news/malaysia/2019/09/24/malaysia-on-track-to-....
Ministry of International Trade and Industry (MITI). 2018. “Industry4WRD.” 2018. https://www.miti.gov.my/index.php/pages/view/industry4WRD?mid=559.
New Straits Times. 2016. “Curbing Food Wastage at Source.” New Straits Times, 2016. https://www.nst.com.my/news/2016/06/151334/curbing-food-wastage-source.
———. 2019a. “5G Trials to Go Nationwide next Month: Gobind | New Straits Times | Malaysia General Business Sports and Lifestyle News.” 2019. https://www.nst.com.my/news/nation/2019/09/524145/5g-trials-go-nationwid....
———. 2019b. “Azmin: Smart Farming Policy Can Modernise Agricultural Landscape | New Straits Times | Malaysia General Business Sports and Lifestyle News.” 2019. https://www.nst.com.my/news/nation/2019/08/513824/azmin-smart-farming-po....
Remans, Roseline, Fabrice A. J. DeClerck, Gina Kennedy, and Jessica Fanzo. 2015. “Expanding the View on the Production and Dietary Diversity Link: Scale, Function, and Change over Time.” Proceedings of the National Academy of Sciences 112 (45): E6082–E6082. https://doi.org/10.1073/PNAS.1518531112.
Sayuti, Zulhazmi, Mohammad Abid Ahmad, and Rosniza Kassim. 2019. “Kilang Tanaman MARDI: Teknologi Pengeluaran Sayuran.”
Sun Media Corporation. 2019. “DSTE Brings Smart Farming Solution to Agriculture Industry.” 2019. https://www.thesundaily.my/spotlight/dste-brings-smart-farming-solution-....
Sundaram, Jomo Kwame, and Zhai Gen Tan. 2019. “Achieving Food Security for All Malaysians.”
Tengku Muda Mohamed, Mahmud. 2017. An Unsung Solution for Postharvest.
UN DESA. 2018. “World Population Prospects 2017.” 2018. https://population.un.org/wpp/Download/Standard/Population/.
UNECE. 2013. “Classification of Types of Big Data.” 2013. http://www1.unece.org/stat/platform/ display/bigdata/Classification+of+Types+of+Big+Data.
Wolfert, Sjaak, Lan Ge, Cor Verdouw, and Marc Jeroen Bogaardt. 2017. “Big Data in Smart Farming – A Review.” Agricultural Systems. https://doi.org/10.1016/j.agsy.2017.01.023.
World Economic Forum, and McKinsey & Company. 2018. “Innovation with a Purpose: The Role of Technology Innovation.” World Economic Forum, no. January: 1–42.
Yacob, Masturina. 2009. “POST HARVEST HANDLING PRACTICES ON SELECTED LOCAL FRUITS” 2: 39–53.
Zambon, Ilaria, Massimo Cecchini, Gianluca Egidi, Maria Grazia Saporito, and Andrea Colantoni. 2019. “Revolution 4.0: Industry vs. Agriculture in a Future Development for SMEs.” Processes 7 (1): 36. https://doi.org/10.3390/pr7010036.
Reviewed, edited and uploaded: November 28, 2019