ABSTRACT
The agricultural sector in Malaysia faces numerous challenges that impede productivity, including low yields, post-harvest losses, limited arable land, increasing demand, and the impacts of climate change. The rise of smart farming technologies, such as precision agriculture and Controlled Environment Agriculture (CEA), offers promising solutions to address these issues. This paper examines the transformation of vegetable cultivation in Malaysia, focusing on the integration of smart agriculture technologies in CEA systems, such as greenhouses and plant factories. It provides both global and local perspectives on smart farming, highlighting key barriers to adoption such as, high implementation costs, limited technical expertise, and insufficient infrastructure. Additionally, the study analyzes national policies, such as the National Agro-Food Policy and the Fourth Industrial Revolution (4IR) Policy, which aim to promote the adoption of smart farming technologies. Harnessing these technological innovations can enhance agricultural productivity and bolster food security, especially in urban areas, where population growth continues to strain food production systems.
Keywords: Smart farming, Controlled environment agriculture, Challenges, Policy intervention, Malaysia.
INTRODUCTION
Most vegetable growers in Malaysia consist of smallholders or private industries, both of which are significantly impacted by the challenges faced in the agricultural sector (FAO, 2011a). The main challenges include high production costs, a shortage of skilled farmers, limited access to financing, insufficient investments, low technology adoption, climate change, shifting government policies, and deteriorating soil quality unsuitable for cultivation (Sengodan, 2022). Additionally, low labor productivity in the agricultural sector is exacerbated by technological advancements and innovations that are not aligned with the skills of farmers. As a result, agricultural practices require transformation through the adoption of smart farming methods to increase production and reduce labor dependency (HRD Corp, 2019).
The drastic increase in the world population over the last two decades has drawn global attention to food insufficiency, particularly in urban areas. The Food and Agriculture Organization (FAO) predicts that food production will need to increase by 70% to feed the global population by 2050 (FAO, 2018). Like many developing countries, Malaysia’s population is also projected to double between 2019 and 2050 (United Nations, 2019). According to Figure 1, Malaysia’s total population has shown a steady increase, from 10.9 million in 1970 to 33.4 million in 2023, with a compound annual growth rate (CAGR) of 2.28%. It is further estimated that the population will reach 41.5 million by 2040 (DOSM, 2023).
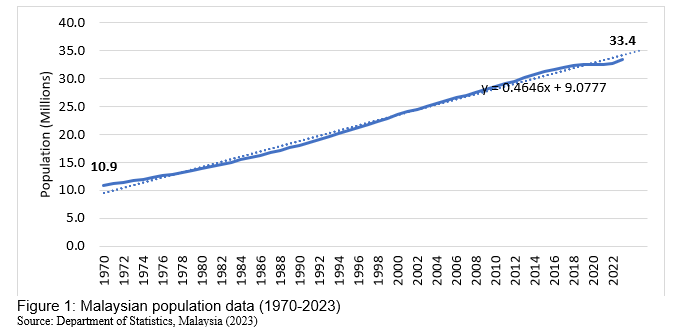
Figure 2 illustrates an increase in vegetable production in 2013, followed by a downward trend from 2014 to 2017, with only a slight increase observed in 2021. The data indicates a strong positive correlation between vegetable production and the planted area, where a reduction in the planted area leads to decreased production.
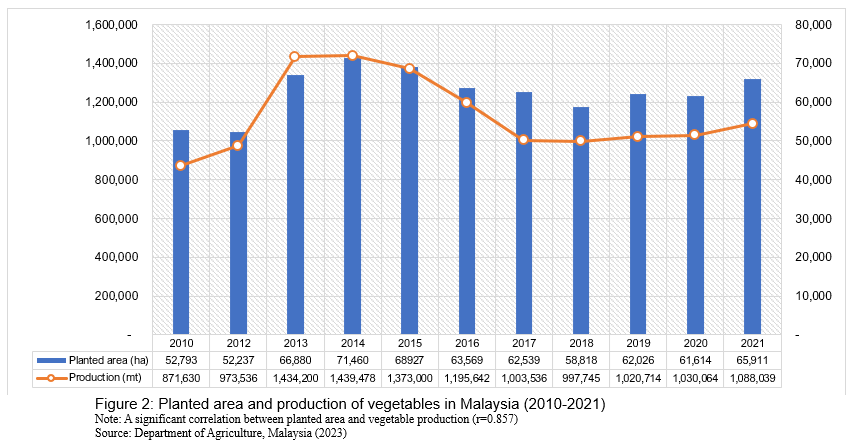
In addition to the factors mentioned above, several other elements contribute to the decline in vegetable production, such as postharvest losses, plant diseases, soil fertility issues, and unpredictable weather. Moreover, population growth exacerbates challenges by leading to limited agricultural land, depletion of natural resources, insufficient water supply, and increased soil pollution (Despommier, 2011). In Malaysia, approximately 37% of the total agricultural land was found to be unsuitable for optimal agricultural use. According to estimates from 2001, only 5.5% of the available land could be cultivated, with the remainder cleared for urban development, settlements, industry, and oil palm plantations (Yahya, 2001).
Researchers and scientists have developed new farming techniques to increase food productivity. As a result, various alternatives to traditional agriculture have emerged, such as soilless cultivation using hydroponic methods, closed farming systems with agricultural technology in controlled environments, genetically modified seeds, and biofortification to enhance the concentration of micronutrients in plants (Benke & Tomkins, 2017; Sharath Kumar et al., 2020; Zeidler & Schubert, 2015). Although Controlled Environment Agriculture (CEA) is commonly used in temperate-climate countries to ensure a year-round supply, it has also been adopted in other regions to boost production and create markets for premium vegetables (Somerville et al., 2014).
In recent years, CEA has been introduced to intensify production in response to growing urban populations and increased demand for food. In Malaysia, specifically, CEA is practiced through vegetable cultivation in rain shelter structures and buildings, such as plant factories. These controlled environments typically utilize hydroponic and vertical farming techniques, which help address challenges associated with limited agricultural land and other issues encountered in conventional farming.
Agricultural development involving these technologies requires significant energy consumption, which indirectly raises production costs. In an era of globalization, it is crucial that the technologies being developed prioritize efficiency and productivity. Controlled Environment Agriculture (CEA) also demands the integration of smart agricultural technologies to maximize benefits for both farmers and consumers. Furthermore, smart agricultural technologies offer a valuable opportunity to attract younger generations to the field, presenting a key avenue for the government to strengthen the nation’s agricultural sector.
This study aims to enhance the understanding of smart agricultural technologies, particularly in their application to vegetable cultivation within CEA environments such as greenhouses and plant factories. It also examines the challenges farmers face when adopting smart farming technologies. Lastly, the study outlines the national policies implemented by the government to encourage the adoption of these technologies by vegetable farmers.
THE REVOLUTION OF SMART FARMING TECHNOLOGY
World scenario
The smart agriculture roadmap in the agricultural industry aims to transition from traditional farming practices to smart farming techniques. In the 17th century, farmers used tools such as sickles, hoes, and grass cutters for cultivation, a period commonly referred to as Agriculture 1.0 (Rapela, 2019). This method required a significant amount of labor to perform agricultural activities; however, production levels remained low.
In the 20th century, Agriculture 2.0 emerged, introducing early-generation innovations that aimed to improve productivity. During this phase, various machines and agricultural equipment were developed for tasks such as harvesting, weeding, watering, sowing, and seed preparation, with the goal of reducing labor costs and increasing output. Additionally, advancements in technology during this period led to the widespread use of gas and oil as primary energy sources.
Moreover, improvements in transportation infrastructure contributed to a more advanced food supply chain, enabling the shipment of agricultural goods over longer distances.
In the third generation of agriculture (Agriculture 3.0), advancements in innovation, particularly in communication technology, software engineering, and the Internet of Things (IoT), have significantly enhanced the capabilities of modern agricultural equipment. The integration of renewable or green energy sources, including hydropower, photovoltaic systems, and wind power, is also being explored. Additionally, Agriculture 3.0 seeks to implement smart agricultural technologies such as crop forecasting, crop recommendation systems, yield monitoring, and knowledge-sharing platforms for farmers (Narvaez et al., 2017). In summary, these three agricultural revolutions have substantially increased crop productivity. Furthermore, traditional labor-intensive agricultural practices are being replaced by modern techniques.
The development of Agriculture 3.0 has begun to emphasize cost-effectiveness and increased productivity. However, several challenges must be addressed in the context of smart agriculture, such as digitization, the agri-food supply chain, ecological issues, and crop productivity. As a result, the integration of smart technologies is essential to advancing agricultural practices (De Clercq et al., 2018). Fourth-generation agriculture (Agriculture 4.0) was introduced to address the limitations of its predecessor, combining several innovative technologies such as big data, machine learning, deep learning, artificial intelligence, IoT, blockchain, robotics, autonomous systems, cyber-physical systems, and computing. This integration of technologies is commonly referred to as smart farming.
Smart farming practices differ significantly from traditional farming due to the incorporation of equipment, technology, machines, devices, sensors, information technology, and computing systems. The future of agriculture will rely heavily on advanced tools and technologies such as GPS, robotics, humidity sensors, and aerial imagery. The development of smart farming systems integrates several techniques, including real-time data collection and processing. Consequently, the shift towards smart agriculture is expected to transform the entire agricultural ecosystem, fostering modern farm management.
By utilizing real-time data, decision support systems can enhance productivity, optimize resource allocation, adapt to climate change, improve food supply chains, and detect crop diseases more efficiently. Recent examples of smart agricultural technology include innovations in automated disease diagnosis using computational systems (Shah et al., 2016; Pantazi et al., 2019; Kaur & Bhatia, 2020). The continuous monitoring and classification of plant diseases have become a major area of interest for researchers and agronomists (Singh & Misra, 2017). Over the past decade, numerous researchers have introduced technologies that have revolutionized the global agricultural landscape.
Kamilaris et al., (2017) conducted a systematic review of recent studies on big data in agriculture, offering solutions to pertinent challenges. Van Klompenburg et al., (2020) conducted a survey to extract and synthesize the characteristics of machine learning algorithms applied in agriculture. Kamilaris and Prenafeta-Boldú (2018) discussed several deep learning models used in various agricultural and food production applications. Elijah et al., (2018) proposed an IoT ecosystem that merges smart agricultural technological innovation with business and product marketability. Sattari et al., (2021) demonstrated an AI-based technique to address real-time optimization challenges in smart agriculture. Finally, Kamilaris et al., (2019) explored the impact of blockchain technology on food supply chains.
Malaysian scenario
Agricultural technology adoption in Malaysia has been evolving, with a growing emphasis on enhancing productivity, sustainability, and efficiency. The country is increasingly leveraging technologies such as precision farming, the Internet of Things (IoT), automation, and digital tools to modernize its agricultural sector. These advancements aim to address labor shortages, improve climate resilience, and promote sustainable practices (World Bank, 2019).
Specifically, Malaysia's agricultural development progresses in parallel with advancements in science and technology, with IR4.0 technologies marking a new paradigm for Agriculture 4.0 (De Clercq et al., 2018; Yahya, 2018; Klerkx et al., 2019). In this paradigm, land preparation, planting, and crop management—including weeding, pest control, and harvesting—are driven by digitalization, automation, IoT, robotics, and artificial intelligence (AI) (Yahya, 2018). There are two primary approaches to achieving Agriculture 4.0: integrating IR4.0 technologies into existing systems or developing entirely new systems when integration is not feasible or when no existing system is available (Santos Valle & Kienzle, 2020).
In Malaysia, most smart agricultural systems have been developed by integrating IR4.0 technologies into existing frameworks. For example, a tractor can be transformed into an Unmanned Guided Vehicle (UGV) by incorporating various sensors, such as ultrasonic sensors, compasses, gyroscopes, accelerometers, and AI systems (Grigore et al., 2020). Simultaneously, mapping can be performed through the integration of GPS and GNSS with advanced sensors and light detection (LiDAR) systems to create an intelligent navigation system (Zhou et al., 2021). This modified machine can prepare land without the need for labor.
Among the latest examples of smart agricultural technology is the use of systems that automatically diagnose plant diseases using computers (Shah et al., 2016; Pantazi et al., 2019; Kaur and Bhatia, 2020). The detection and classification of plant diseases through continuous monitoring has become a major focus for researchers and agronomists (Singh and Misra, 2017). Over the past decade, several researchers have introduced technologies that have transformed agricultural systems worldwide. For instance, Kamilaris et al., (2017) conducted a systematic review of recent research in the field of big data in agriculture, providing solutions to related challenges. Van Klompenburg et al., (2020) conducted a survey to extract and synthesize the characteristics of machine learning algorithms used in agriculture. Kamilaris and Prenafeta-Boldú (2018) highlighted several deep learning models used in various agricultural and food production applications. Elijah et al., (2018) proposed an IoT ecosystem that combines smart agricultural technology with innovations in business and product marketability. Sattari et al. (2021) demonstrated an AI-based technique to address real-time optimization challenges in smart agriculture. Kamilaris et al. (2019) explored the effects of blockchain technology on the food supply chain.
In the soil preparation stage, lightweight autonomous soil parameter retrieval robots can be used to generate soil fertility maps. The combination of the intelligent autonomous system developed and the automatic index system will be a smart automatic levelling machine for land preparation in rice fields (Abu Bakar et al., 2019). For crop management, fertilizer and pesticide applications can be done intelligently with a combination of Unmanned Aerial Vehicles (UAV) and autonomous fertilizer application or chemical spraying systems using variable-rate technology (VRT) in rice production. GIS-based mapping of soil fertility and plant conditions obtained by the UAV is processed and analyzed with AI systems to produce treatment maps. The treatment map enables specific crop management in the planting plot with autonomous systems or smart VRT drones to apply fertilizers and pesticides in the right amount, in the right place and at the right time. Therefore, it can optimize the use of fertilizers and pesticides without waste (ST Ten et al., 2023). The implementation of precision farming technologies mentioned above has been applied to the cultivation of rice, palm oil, and rubber in Malaysia. (Ramli et al., 2022).
Controlled Environment Agriculture scenario in Malaysia
The concept of Controlled Environment Agriculture (CEA) encompasses agricultural practices that optimize environmental conditions for plant growth. These cultivation activities are conducted inside structures such as greenhouses, rain shelters, and indoor plant factories (Game and Primus, 2015; AlKodmany, 2018). CEA technology monitors and controls key parameters for plant growth, including nutrient solution levels, light quality and quantity, temperature, relative humidity, and carbon dioxide (CO2) levels (Zeidler & Schubert, 2014). Despommier (2011) demonstrated that CEA is more advantageous than traditional agriculture because it enables year-round production, protects crops from natural disasters (e.g., typhoons, floods, and droughts), requires no heavy machinery, and minimizes water use. Moreover, Zeidler and Schubert (2015) emphasized that CEA facilitates consumer access to local produce and ensures a stable food supply. Despommier (2018) predicts that this agricultural concept will be commercialized globally within the next 10 to 20 years.
In Malaysia, indoor cultivation systems, such as plant factories, have been emerging since 2016, with most located in the Klang Valley region (Ahmad et al., 2024). A plant factory is an intelligent agricultural system designed for vegetable production. It employs vertical farming techniques and is fully integrated with IoT-based smart control and monitoring systems. These systems allow for comprehensive monitoring and regulation via artificial intelligence (AI), managing the building’s microclimate, including temperature, humidity, CO2 levels, airflow, hydroponic systems, and artificial lighting (ST Ten et al., 2023).
The plant factory’s controlled-environment system comprises three main components: the mobile application system, environmental sensors, and the repeater module. The installed sensors include temperature, humidity, CO2, and airflow sensors. The e-farm application, developed on the Android platform, displays data on a tablet and enables control through the mobile app to ensure optimal growing conditions. For example, the optimal temperature range for lettuce is estimated to be between 23°C and 28°C, with a relative humidity of 60% to 70%. Monitoring and control systems are essential for micro-irrigation systems, especially to meet the specific growth requirements of plants. Depending on the growth conditions, plants need certain levels of nutrients (Ahmad et al., 2022). Micro-irrigation systems consist of a fertigation tank, a water pump, an electric injector for fertilizer, and a control system. The sensor system includes a pH sensor, an electrical conductivity (EC) sensor, and a water temperature sensor. These sensors are connected to a Supervisory Control and Data Acquisition (SCADA) system, which sets parameters for EC and pH, and the data is visualized through the SCADA control panel. Irrigation data is also stored on a local server located in a controlled room, and all data is synced to cloud storage and can be viewed on a dashboard (Ahmad et al., 2022; ST Ten et al., 2023). This system has been integrated into the IoT framework by modifying port connections and extending it to Wi-Fi and 4G LTE connections. Consequently, the smart agriculture-based cultivation in these plant factories is expected to increase productivity per unit area by 4 to 6 times, producing high-quality, nutritious, and pesticide-free vegetables (Sayuti, 2022).
TECHNOLOGY USED FOR VEGETABLE FARMING ACTIVITIES USING CEA CONCEPT IN MALAYSIA
Greenhouse
In this study, the technology used by most of the farmers in Malaysia is also listed to give a clear picture and the actual scenario of vegetable cultivation in a controlled agricultural system. The list of technologies applied in greenhouses is based on the vegetable cultivation technology manual published by MARDI. These technologies encompass various stages of the cultivation process, from seedling management to post-harvest handling. A more detailed overview is provided in Table 1.
Table 1. The technology used for vegetable cultivation in greenhouse from seedling to harvest
No.
|
Activity
|
Component
|
1.
|
Seedlings
|
- Divided into 2 components which is sowing either using plastic trays or peat cubes.
- Seedling care, which is fertilizing and watering, is usually carried out in the nursery room.
|
2.
|
Cultivation
|
- If planting is done on land, 4 activities are included, namely ploughing, liming, border preparation and mulch installation. If planting is carried out hydroponically or fertigation, the planting process begins with the preparation of media.
- Among the components of smart agriculture that are usualy involved in the cultivation process are environmental monitoring and control activities. For example: the use of sensors, IoT applications or robotics (AGV) and control systems such as Ubibot and Arduino.
|
3.
|
Fertilization
|
- Fertilization methods are also divided into 2 either planting on the ground or using fertigation or hydroponic systems.
- If planting on the ground, the fertilization method is carried out either using labor, semi-mechanization such as sprayers or automation using drones and others.
- The application of fertilizer using fertigation and hydroponics can be done automatically with the integration of timer or sensors.
|
4.
|
Irrigation
|
- Irrigation methods are also divided into 2, namely planting on the ground or using systems (fertigation and hydroponics).
- If planting on the ground, the irrigation is done using labour, semi-mechanization such as sprayers or irrigation pumps or automation using drones and others.
- The irrigation method for fertigation and hydroponic can also be done automatically using timer or sensors.
|
5.
|
Pest and disease control
|
- It is customary to monitor pests and diseases using canventional detection methods (labor). While the pest and disease control process is controlled through the process of pesticide spraying. This process can be done either using a semi-mechanized method (sprayer) or automation (spraying machine).
- Pest and disease management activities using smart agriculture technology are based on IoT technology or AI-based detection model.
|
6.
|
Weed management
|
- The weed management practice is usually for planting on land either manually using labour force, semi-mechanized using sprayer or automation which is spraying machine.
- While planting using fertigation and hydroponic systems does not have weed management activities.
|
7.
|
Harvesting and postharvest handling
|
- This harvesting method can be done manually using labour (picking), semi-mechanized or automated.
- Postharvest handling which consists of sorting, grading, and packing methods is also done using manual or semi-mechanized.
|
Source: MARDI Vegetable Cultivation in Greenhouse Manual (MARDI, 2022)
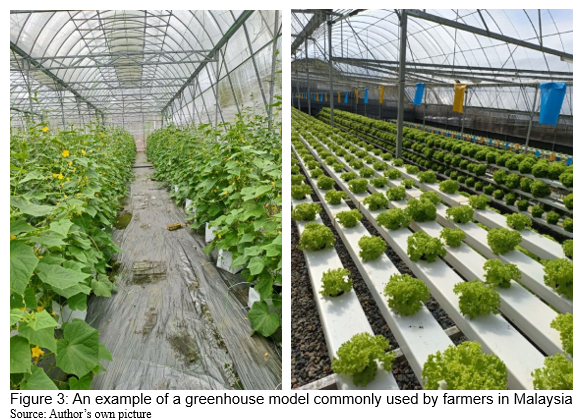
Plant factory
The list of technology listed in this paper is based on the plant factory technology book published by MARDI. Research for plant factory technology is one of the initiatives taken by MARDI to develop plant factory technology that can be used as a guide for entrepreneurs. These technologies encompass various stages of the cultivation process, from seedling management to post-harvest handling are shown in Table 2.
Table 2. The technology used for vegetable cultivation in plant factory from seedling to harvest
No.
|
Activity
|
Component
|
1.
|
Seedling
|
- Seedling management is divided into 2 components which are sowing either using plastic trays or peat cubes and it is done conventionally.
- The second is seedling care, which is fertilization and irrigation which is usually carried out in the nursery room using a multi-level Ebb and Flow type hydroponic system with LED lighting.
|
2.
|
Planting
|
- The planting process begins with the media preparation and it is done manually.
- Transplanting is usually done and carried out manually to a multi-level planting system.
- The use of LED lights is also either using timers or sensors.
- Among the components of smart agriculture that are used in this planting process are environmental monitoring and control activities (Figure 4). For example: the use of sensors, IoT applications, robotics (AGV) and control systems such as Ubibot and Arduino)
|
3.
|
Fertilization
|
- Ebb & flow type hydroponic systems equipped with LED lighting are used in irrigation and fertilization systems in plant factories.
|
4.
|
Irrigation
|
5.
|
Pest and disease control
|
- There are 2 processes that are involved, namely the pest and disease monitoring process and the pest and disease control process.
- Pest and disease management activities using smart agriculture technology are measured in terms of the use of IoT or AI detection applications.
|
7.
|
Harvesting and post-harvest handling
|
- This harvesting method is measured by whether it is done manually using labour (picking), semi-mechanized or automated.
- Post-harvest handling which consists of sorting, grading, and packing methods is also done using manual or semi-mechanized.
|
Source: MARDI Plant Factory Technology (MARDI, 2022)
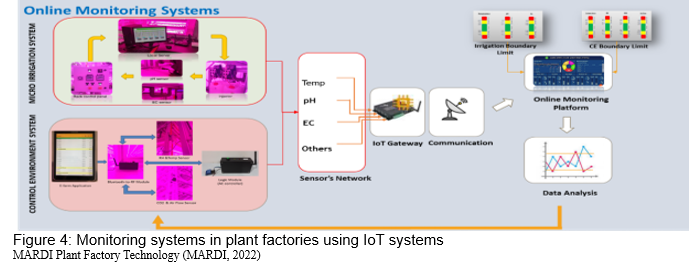
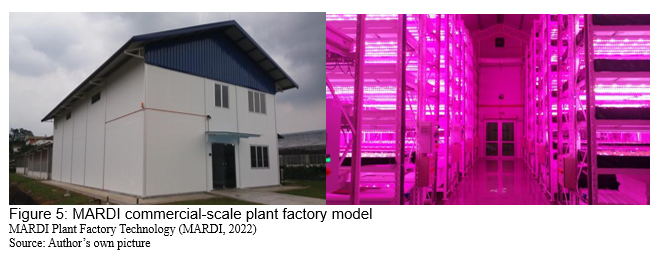
CHALLENGES TO ADOPTING SMART AGRICULTURE TECHNOLOGY
Controlled Environment Agriculture (CEA) refers to advanced agricultural practices such as hydroponics, aeroponics, and vertical farming, where conditions like light, temperature, humidity, and nutrient levels are meticulously controlled. While these technologies are widely known and already employed by many vegetable farmers, the integration of smart technologies (e.g., sensors, automation, data analytics, and IoT) can further enhance productivity and resource efficiency. However, the adoption of smart agriculture technology in CEA has been slower compared to other industries, largely due to several significant challenges.
One of the primary obstacles is the high cost of implementing smart farming technologies, including climate control systems, advanced sensors, and automated irrigation, which are especially burdensome for smallholder farmers. These farmers often lack the financial resources necessary for such investments. Although there are government initiatives to subsidize smart farming, the financial support provided may be insufficient for widespread adoption. Farmers are also concerned about the return on investment (ROI), particularly since the ROI for smart technologies tends to be longer (Baumont de Oliveira et al., 2022).
Another key challenge is the limited technical expertise and skills required for successful adoption. Smart technology in CEA demands a strong understanding of digital tools, data analytics, and automation systems. In Malaysia, many farmers, particularly those in rural areas, lack the technical knowledge needed to operate and maintain these systems. Continuous education is essential to keep farmers abreast of rapidly evolving technologies. The lack of access to such resources hampers the uptake of smart farming (Lioutas and Charatsari, 2020).
Infrastructure and internet connectivity are also crucial factors that must be addressed. Many smart technologies rely on consistent and reliable internet access for monitoring and data management. In rural areas of Malaysia, internet connectivity can be unreliable or entirely unavailable, limiting the use of IoT devices and cloud-based systems in CEA (Gong, 2023). Additionally, controlled environments that depend on artificial lighting, climate control, and other automated systems require a stable and continuous electricity supply. In regions where electricity is inconsistent, it becomes challenging to implement and maintain these smart technologies.
While Controlled Environment Agriculture (CEA) systems such as greenhouses or plant factories are energy-intensive, the high cost of electricity in Malaysia can make these systems expensive to operate, affecting the long-term viability of adopting smart technologies. Additionally, the maintenance of smart equipment adds to the financial burden. Therefore, research is needed to reduce energy usage in plant factories and controlled environments, with a focus on developing energy-efficient technologies. One alternative is the use of solar power (Kubo et al., 2016). Another example is the use of reflectors, which can minimize the quantity of LED lights needed in plant factories. According to Shaari et al. (2021), a large portion of energy consumption in plant factories comes from air-conditioning systems (51%) and LED lighting (36%). Thus, installing reflectors to reduce LED use would contribute to lower energy consumption.
Moreover, smart farming technologies generate large volumes of data—such as soil moisture levels, temperature, light intensity, and nutrient status—that many farmers may struggle to interpret effectively without specialized software or training. Mismanagement of this data can lead to inefficiencies in farm operations, counteracting the benefits of smart technologies. Additionally, the components of smart farming systems, such as sensors, irrigation, and climate control, may not always be fully integrated. This fragmentation can result in isolated operations where systems work independently, reducing the overall effectiveness of the CEA setup (Wolfert et al., 2017).
The profitability of investing in smart technologies for vegetable cultivation is influenced by market demand and price fluctuations. Malaysian farmers may be hesitant to adopt expensive systems due to uncertainty regarding economic returns, particularly given that vegetable prices can vary with seasonality, imports, and consumer demand. While CEA enables high-quality vegetable production, smallholder farmers may struggle to access premium markets that offer higher prices for such produce. Without guaranteed higher income, investing in smart technologies becomes riskier (Ahmad et al., 2024).
Another critical factor is cultural resistance and traditional farming practices (Ahmad et al., 2023). Many Malaysian farmers are accustomed to methods passed down through generations, and the perceived complexity and novelty of smart farming technologies can lead to skepticism. Farmers may be hesitant to abandon established methods, particularly in regions with an aging farming population, where older farmers are less inclined to adopt new technologies. Younger generations, who might be more open to smart farming, often migrate to urban areas, leaving older farmers in charge of operations.
Although Malaysia has implemented initiatives such as the National Agri-Food Policy (NAP 2.0) and the Smart Farming Initiative, these policies may not fully address the unique challenges faced by small-scale vegetable farmers in adopting smart technologies. The bureaucratic process of accessing grants and subsidies can be slow and complicated, discouraging farmers from seeking government assistance. Moreover, a lack of standardized regulations governing the use of smart technologies in agriculture can result in farmers purchasing substandard or incompatible equipment, further hindering adoption.
In conclusion, adopting smart technologies for vegetable cultivation within CEA systems in Malaysia presents significant challenges, including high costs, limited technical knowledge, infrastructure constraints, and market uncertainties. Overcoming these barriers will require collaborative efforts between government bodies, financial institutions, educational institutions, and the private sector. Addressing these challenges through targeted policies, improved training, financial support, and better infrastructure can help accelerate the adoption of smart farming technologies in Malaysia’s agricultural sector.
NATIONAL POLICY TO SUPPORT ADOPTING SMART AGRICULTURE TECHNOLOGY
Agri-food sector contribute income to the Malaysia economy with Gross Domestic Product (GDP) growth increased 6.8% which is US$11.43 billion in 2020 compared to US$6.3 billion in 2011. The government implement policies to expedite smart agriculture transformation in Malaysia. Among the policies are:
• National Agri-Food Policy (2021-2030)
• National Fourth Industrial Revolution (4IR) policy (2021-2030)
• National Food Security Action Plan (2021-2025)
National Agri-Food Policy 2.0 (NAP 2.0)
Launched as an updated version of the National Agri-Food Policy, this policy emphasizes the modernization of agriculture by encouraging the adoption of smart farming technologies. One of the key targets is improving the efficiency of food production, particularly vegetables, through the application of precision agriculture, IoT (Internet of Things), AI, drones, and data analytics. The policy also includes initiatives to promote research, development, commercialization, and innovation (R&D&C&I) in agriculture, aimed at encouraging the creation and adoption of new smart farming solutions.
National Fourth Industrial Revolution (4IR) policy (2021-2030)
The Malaysia National Fourth Industrial Revolution (4IR) Policy (2021-2030) is a strategic plan aimed at driving Malaysia’s transition into the Fourth Industrial Revolution. This policy outlines the country's approach to adopting advanced technologies and fostering innovation to boost economic growth and enhance competitiveness. Key components of Malaysia's 4IR policy include promoting the use of technologies like AI, IoT, and robotics across various sectors. Developing robust digital infrastructure to support the implementation of 4IR technologies. Secondly, is enhancing skills and training programs to prepare the workforce for new technological demands. The third is to encourage research and development, as well as fostering collaboration between public and private sectors. The policy also plans to establish guidelines to ensure the ethical and secure use of new technologies and it will also be supporting the growth of emerging industries and reducing dependency on traditional sectors. The goal is to position Malaysia as a regional leader in 4IR technologies, enhance its global competitiveness, and ensure sustainable economic development.
National Food Security Action Plan (2021-2025)
The Malaysia National Food Security Action Plan (2021-2025) is a strategic initiative aimed at strengthening the country’s food security by enhancing agricultural productivity, improving supply chain resilience, and promoting sustainable practices. It focuses on boosting local food production, supporting farmers, and ensuring equitable access to affordable, nutritious food for all Malaysians. The plan also emphasizes the importance of monitoring and evaluating progress to adapt and refine strategies, ultimately seeking to reduce reliance on food imports and enhance overall food security.
Malaysia Digital Economy Blueprint (MyDIGITAL)
MyDIGITAL, which outlines Malaysia’s digital transformation, includes provisions for developing a smart agriculture ecosystem. It encourages the use of IoT, AI, and data analytics in agriculture. The blueprint provides a framework for integrating smart farming into the national digital infrastructure and aims to make Malaysia a regional leader in digital agriculture technologies.
CONCLUSION
In conclusion, smart farming technologies present a transformative opportunity for Malaysia’s agricultural sector, particularly in the realm of controlled environment agriculture (CEA). The adoption of these technologies is hindered by high costs, limited technical knowledge, and inadequate infrastructure—challenges that are particularly pronounced for small-scale farmers. Government interventions including subsidies, financial support, and specialized training programs, are essential to mitigating these barriers. National frameworks like the National Agri-Food Policy and the 4IR Policy are already facilitating this transition by promoting innovation and investment in smart farming. Collaborative efforts between the government, the private sector, and farmers will be crucial to addressing the remaining challenges and accelerating the adoption of smart farming technologies. Such efforts will ensure that Malaysia’s agricultural sector remains sustainable and competitive in the global market.
RECOMMENDATION
To accelerate the adoption of smart farming technologies among vegetable farmers in Malaysia, several key actions are recommended. First, the government should enhance financial support by providing more targeted subsidies, low-interest loans, and grants specifically for the acquisition of smart farming technologies like sensors, automated irrigation systems, and drones. Expanding comprehensive training programs is also crucial to equipping farmers with the technical skills needed to effectively use these technologies. Strengthening partnerships with private sector innovators and technology providers can help reduce the cost of advanced agricultural solutions, making them more accessible to small-scale farmers. Additionally, improving digital infrastructure in rural areas, such as internet connectivity, will ensure farmers can fully leverage IoT-based systems. Promoting the success stories of early adopters and offering incentives for pioneers can also build trust and encourage more widespread acceptance of smart farming practices. Finally, simplifying the bureaucratic processes for accessing government support and ensuring continuous education will ensure sustained progress in transforming Malaysia’s agriculture sector.
REFERENCES
Abu Bakar, B., Ahmad, M. T., Ghazali, M. S. S., Abd Rani, M. N. F., Mhd Bookeri, M. A., Abdul Rahman, M. S., ... & Ismail, R. (2020). Leveling-index based variable rate seeding technique for paddy. Precision Agriculture, 21, 729-736.
Ahmad, M.A., Basir, M.H., Tahir, M.A.M., Shafie, K.A. & Sulaiman, A.S.S. (2022). Sistem Pengeluaran dalam Kilang Tanaman. In Sayuti, Z., Ahmad, M.A., Yusoff, M.M, & Amaddin, P.A.M (Eds.), Kilang Tanaman MARDI: Teknologi Moden Pengeluaran Tanaman Makanan in Malaysia. Penerbit MARDI.
Ahmad. A.A, Omar, N.R.N, Yusoff M.M, & Ahmad. M.A. (2024). From Farm to Table Concept: The Profile and Dynamics of Urban Farming on Agrofood Supply Chain in Malaysia. FFTC Journal of Agricultural Policy. 17-34. Vol 2.
Ahmad, A. A., Kasron, N., Noh, N. H. M., Azmon, H., & Chuang, T. C. (2023). The acceptance of decision support system (DSS) in vegetable cultivation among farmers in Malaysia. Economic and Technology Management Review, Vol. 21:1-18.
Al-Kodmany, K. (2018). The vertical farm: A review of developments and implications for the vertical city. Buildings, 8(2), 24.
Baumont de Oliveira, F. J., Ferson, S., Dyer, R. A., Thomas, J. M., Myers, P. D., & Gray, N. G. (2022). How high is high enough? Assessing financial risk for vertical farms using imprecise probability. Sustainability, 14(9), 5676.
Benke, K., & Tomkins, B. (2017). Future food-production systems: Vertical farming and controlled-environment agriculture. Sustainability: Science, Practice and Policy, 13(1), 13-26. https://doi.org/10.1080/15487733.2017.1394054
De Clercq, M., Vats, A., & Biel, A. (2018). Agriculture 4.0: The future of farming technology. Proceedings of the world government summit, Dubai, UAE, 11-13.
Despommier, D. (2011). The vertical farm: Controlled environment agriculture carried out in tall buildings would create greater food safety and security for large urban populations. Journal für Verbraucherschutz und Lebensmittelsicherheit, 6(2), 233-236. https://doi.org/10.1007/s00003-010-0654-3
Despommier, D. (2011). The vertical farm: Controlled environment agriculture carried out in tall buildings would create greater food safety and security for large urban populations. Journal für Verbraucherschutz und Lebensmittelsicherheit, 6(2), 233-236. https://doi.org/10.1007/s00003-010-0654-3
Despommier, D. (2018). The Vertical Farm: Feeding the world in the 21st century. Retrieved October 19, 2021, from http://www.verticalfarm.com/?page_id=75
DOSM. (2023). Compendium of environment statistics, Malaysia 2019. Department of Statistics Malaysia. Retrieved July 15, 2024, from https://open.dosm.gov.my/ms-MY/data-catalogue/population_malaysia
Elijah, O., Rahman, T. A., Orikumhi, I., Leow, C. Y., & Hindia, M. N. (2018). An overview of Internet of Things (IoT) and data analytics in agriculture: Benefits and challenges. IEEE Internet of things Journal, 5(5), 3758-3773.
FAO. (2011a). AQUASTAT country profile - Malaysia. Food and Agriculture Organization of the United Nations, Rome. Retrieved August 29, 2021, from http://www.fao.org/aquastat/en/countries-and-basins/country-profiles.
Game, I., Primus, R. (2015). GSDR 2015 Brief: Urban Agriculture End Hunger, Achieve. Food Security and Improved Nutrition and Promote Sustainable Agriculture.
Gong, Rachel. 2023. Connecting the Last Mile: Solutions for Rural and Remote Communities. Kuala Lumpur: Khazanah Research Institute. License: Creative Commons Attribution CC BY 3.0. Available at: https://www.krinstitute.org/assets/contentMS/img/template/editor/230731%20rural%20connectivity%20v1.5.1%20pub%202.pdf (Accessed: 16 September 2024).
HRD Corp. (2019). HRDF Human Capital Report (Agriculture Sector Overview: Will Agriculture Be The Next Sector Covered Under PSMB Act?). Human Resources Development Corporation.
Kamilaris, A., & Prenafeta-Boldú, F. X. (2018). Deep learning in agriculture: A survey. Computers and electronics in agriculture, 147, 70-90.
Kamilaris, A., Kartakoullis, A., & Prenafeta-Boldú, F. X. (2017). A review on the practice of big data analysis in agriculture. Computers and Electronics in Agriculture, 143, 23-37.
Kamilaris, A., van den Brink, C., & Karatsiolis, S. (2019). Training deep learning models via synthetic data: Application in unmanned aerial vehicles. In Computer Analysis of Images and Patterns: CAIP 2019 International Workshops, ViMaBi and DL-UAV, Salerno, Italy, September 6, 2019, Proceedings 18 (pp. 81-90). Springer International Publishing.
Kaur, M., & Bhatia, R. (2020). Leaf disease detection and classification: A comprehensive survey. In Proceedings of International Conference on IoT Inclusive Life (ICIIL 2019), NITTTR Chandigarh, India (pp. 291-304). Springer Singapore.
Klerkx, L., Jakku, E., & Labarthe, P. (2019). A review of social science on digital agriculture, smart farming and agriculture 4.0: New contributions and a future research agenda. NJAS-Wageningen journal of life sciences, 90, 100315.
KPKM (2023). National Food Security Policy Action Plan 2021-2025. Putrajaya: Ministry of Agriculture and Food Security. Retrieved from https://www.kpkm.gov.my/en/agro-food-policy/pelan-tindakan-dasar-sekuriti-makanan-2021-2025
KPKM.(2021). National Agrofood Policy 2021-2030 (NAP 2.0). Putrajaya: Ministry of Agriculture and Food Security. Retrieved from https://www.kpkm.gov.my/en/agro-food-policy/national-agrofood-policy
Kubo, H., Murayama, S., Tanimoto, M., Okoso, K., & Maeno, S. (2016, September). A possibility of open zero energy plant factory. In 2016 Electronics Goes Green 2016+(EGG) (pp. 1-8). IEEE.
Lioutas, E. D., & Charatsari, C. (2020). Smart farming and short food supply chains: Are they compatible?. Land Use Policy, 94, 104541.
Malaysian Agricultural Research and Development Institute, MARDI (2022). Manual Teknologi Penanaman Kubis Bunga Tanah Rendah di Bawah Struktur Pelindung Hujan (Penerbit MARDI). Malaysia.
Malaysian Agricultural Research and Development Institute, MARDI (2022). Kilang Tanaman MARDI (Penerbit MARDI). Malaysia.
Ministry of Economy (2021). Malaysia Digital Economy Blueprint (MyDIGITAL). Putrajaya: Ministry of Economy. https://www.ekonomi.gov.my/sites/default/files/2021-02/malaysia-digital-economy-blueprint.pdf
Ministry of Economy (2021). National Fourth Industrial Revolution (4IR) Policy (2021-2030). Putrajaya: Ministry of Economy. https://www.ekonomi.gov.my/sites/default/files/2021-07/National-4IR-Policy.pdf
Narvaez, F. Y., Reina, G., Torres-Torriti, M., Kantor, G., & Cheein, F. A. (2017). A survey of ranging and imaging techniques for precision agriculture phenotyping. IEEE/ASME Transactions on Mechatronics, 22(6), 2428-2439.
Pantazi, X. E., Moshou, D., & Tamouridou, A. A. (2019). Automated leaf disease detection in different crop species through image features analysis and One Class Classifiers. Computers and electronics in agriculture, 156, 96-104.
Ramli, Z. F., Abd Karim, H., Amirnordin, N. A., Zainal, D. N., Md Akhir, N., & Tengku Sulaiman, T. S. R. M. (2022). Pelaksanaan teknologi pertanian tepat, mekanisasi, automasi dan aplikasi teknologi Internet of Things (IoT) dalam sektor pertanian di Malaysia. ASPIRASI FPP, 35-39.
Rapela, M. A. (2019). Fostering innovation for agriculture 4.0. Springer International Publishing.
Santos Valle, S., & Kienzle, J. (2020). Agriculture 4.0–agricultural robotics and automated equipment for sustainable crop production.
Sattari, M. A., Roshani, G. H., Hanus, R., & Nazemi, E. (2021). Applicability of time-domain feature extraction methods and artificial intelligence in two-phase flow meters based on gamma-ray absorption technique. Measurement, 168, 108474.
Sayuti. Z. (2022). Prospek, Status Semasa dan Hala Tuju Pertanian Moden. (2022). In Sayuti, Z., Ahmad, M.A., Yusoff, M.M.,& Amaddin, P.A.M (Eds.), Kilang Tanaman MARDI: Teknologi Moden Pengeluaran Tanaman Makanan in Malaysia. Penerbit MARDI
Sengodan, P. (2022). An Overview of Vertical Farming: Highlighting the Potential in Malaysian High-Rise Buildings. Pertanika Journal of Science & Technology, 30(2).
Shaari, A. M., Razuan, M. S. M., Taweekun, J., Batcha, M. F. M., Abdullah, K., Sayuti, Z., ... & Tahir, M. A. M. (2021). Energy Profiling of a Plant Factory and Energy Conservation Opportunities. Journal of Advanced Research in Fluid Mechanics and Thermal Sciences, 80(1), 13-23.
Shah, J. P., Prajapati, H. B., & Dabhi, V. K. (2016, March). A survey on detection and classification of rice plant diseases. In 2016 IEEE International Conference on Current Trends in Advanced Computing (ICCTAC) (pp. 1-8). IEEE.
SharathKumar, M., Heuvelink, E., & Marcelis, L. F. M. (2020). Vertical farming: Moving from genetic to environmental modification. Trends in Plant Science, 25(8), 724-727. https://doi.org/10.1016/j.tplants.2020.05.012
Singh, V., & Misra, A. K. (2017). Detection of plant leaf diseases using image segmentation and soft computing techniques. Information processing in Agriculture, 4(1), 41-49.
Somerville, C., Cohen, M., Pantanella, E., Stankus, A., & Lovatelli, A. (2014). Small-scale aquaponic food production-Integrated fish and plant farming. Food and Agriculture Organization Rome.
Ten, S. T., Shafie, K. A. B., & Abdul Rani, R. B. (2023). Achieving agriculture 4.0 through modernization and enhancement with mechanization, automation and advanced technologies. In Smart Agriculture for Developing Nations: Status, Perspectives and Challenges (pp. 49-58). Singapore: Springer Nature Singapore.
United Nation. (2019). World population prospects 2019: Highlights. Population Division. http://creativecommons.org/licenses/by/3.0/igo/
Van Klompenburg, T., Kassahun, A., & Catal, C. (2020). Crop yield prediction using machine learning: A systematic literature review. Computers and electronics in agriculture, 177, 105709.
Wolfert, S., Ge, L., Verdouw, C., & Bogaardt, M. J. (2017). Big data in smart farming–a review. Agricultural systems, 153, 69-80.
World Bank. (2019). Agricultural Transformation and Inclusive Growth: The Malaysian Experience. https://openknowledge.worldbank.org/server/api/core/bitstreams/1c25a5d4-fbc0-5325-8e44-1ccef47c82f5/content
Yahya, N. (2018). Agricultural 4.0: Its implementation toward future sustainability. Green Urea: For Future Sustainability, 125-145.
Yahya, T. M. T. (2001). Crop diversification in Malaysia. In M. K. Papademetriou & F. J. Dent (Eds.), Crop Diversification in the asia-pacific region (pp. 71-87). Food and Agriculture Organisation of the United Nations.
Zeidler, C., & Schubert, D. (2014). From bioregenerative life support systems for space to vertical farming on earth - The 100% spin-off . In Life in Space for Life on Earth Symposium 2014, Waterloo, Canada.
Zeidler, C., and Schubert, D. (2015). Vertical Farm 2.0: Designing an Economically Feasible Vertical Farm – A Combined European Endeavor for Sustainable Urban Agriculture (Association for Vertical Farming). https://www.researchgate.net/ publication/321427717
Zhou, T., Hasheminasab, S. M., & Habib, A. (2021). Tightly-coupled camera/LiDAR integration for point cloud generation from GNSS/INS-assisted UAV mapping systems. ISPRS Journal of Photogrammetry and Remote Sensing, 180, 336-356.
The Smart Agriculture Revolution Transforming Controlled Environment Farming in Malaysia
ABSTRACT
The agricultural sector in Malaysia faces numerous challenges that impede productivity, including low yields, post-harvest losses, limited arable land, increasing demand, and the impacts of climate change. The rise of smart farming technologies, such as precision agriculture and Controlled Environment Agriculture (CEA), offers promising solutions to address these issues. This paper examines the transformation of vegetable cultivation in Malaysia, focusing on the integration of smart agriculture technologies in CEA systems, such as greenhouses and plant factories. It provides both global and local perspectives on smart farming, highlighting key barriers to adoption such as, high implementation costs, limited technical expertise, and insufficient infrastructure. Additionally, the study analyzes national policies, such as the National Agro-Food Policy and the Fourth Industrial Revolution (4IR) Policy, which aim to promote the adoption of smart farming technologies. Harnessing these technological innovations can enhance agricultural productivity and bolster food security, especially in urban areas, where population growth continues to strain food production systems.
Keywords: Smart farming, Controlled environment agriculture, Challenges, Policy intervention, Malaysia.
INTRODUCTION
Most vegetable growers in Malaysia consist of smallholders or private industries, both of which are significantly impacted by the challenges faced in the agricultural sector (FAO, 2011a). The main challenges include high production costs, a shortage of skilled farmers, limited access to financing, insufficient investments, low technology adoption, climate change, shifting government policies, and deteriorating soil quality unsuitable for cultivation (Sengodan, 2022). Additionally, low labor productivity in the agricultural sector is exacerbated by technological advancements and innovations that are not aligned with the skills of farmers. As a result, agricultural practices require transformation through the adoption of smart farming methods to increase production and reduce labor dependency (HRD Corp, 2019).
The drastic increase in the world population over the last two decades has drawn global attention to food insufficiency, particularly in urban areas. The Food and Agriculture Organization (FAO) predicts that food production will need to increase by 70% to feed the global population by 2050 (FAO, 2018). Like many developing countries, Malaysia’s population is also projected to double between 2019 and 2050 (United Nations, 2019). According to Figure 1, Malaysia’s total population has shown a steady increase, from 10.9 million in 1970 to 33.4 million in 2023, with a compound annual growth rate (CAGR) of 2.28%. It is further estimated that the population will reach 41.5 million by 2040 (DOSM, 2023).
Figure 2 illustrates an increase in vegetable production in 2013, followed by a downward trend from 2014 to 2017, with only a slight increase observed in 2021. The data indicates a strong positive correlation between vegetable production and the planted area, where a reduction in the planted area leads to decreased production.
In addition to the factors mentioned above, several other elements contribute to the decline in vegetable production, such as postharvest losses, plant diseases, soil fertility issues, and unpredictable weather. Moreover, population growth exacerbates challenges by leading to limited agricultural land, depletion of natural resources, insufficient water supply, and increased soil pollution (Despommier, 2011). In Malaysia, approximately 37% of the total agricultural land was found to be unsuitable for optimal agricultural use. According to estimates from 2001, only 5.5% of the available land could be cultivated, with the remainder cleared for urban development, settlements, industry, and oil palm plantations (Yahya, 2001).
Researchers and scientists have developed new farming techniques to increase food productivity. As a result, various alternatives to traditional agriculture have emerged, such as soilless cultivation using hydroponic methods, closed farming systems with agricultural technology in controlled environments, genetically modified seeds, and biofortification to enhance the concentration of micronutrients in plants (Benke & Tomkins, 2017; Sharath Kumar et al., 2020; Zeidler & Schubert, 2015). Although Controlled Environment Agriculture (CEA) is commonly used in temperate-climate countries to ensure a year-round supply, it has also been adopted in other regions to boost production and create markets for premium vegetables (Somerville et al., 2014).
In recent years, CEA has been introduced to intensify production in response to growing urban populations and increased demand for food. In Malaysia, specifically, CEA is practiced through vegetable cultivation in rain shelter structures and buildings, such as plant factories. These controlled environments typically utilize hydroponic and vertical farming techniques, which help address challenges associated with limited agricultural land and other issues encountered in conventional farming.
Agricultural development involving these technologies requires significant energy consumption, which indirectly raises production costs. In an era of globalization, it is crucial that the technologies being developed prioritize efficiency and productivity. Controlled Environment Agriculture (CEA) also demands the integration of smart agricultural technologies to maximize benefits for both farmers and consumers. Furthermore, smart agricultural technologies offer a valuable opportunity to attract younger generations to the field, presenting a key avenue for the government to strengthen the nation’s agricultural sector.
This study aims to enhance the understanding of smart agricultural technologies, particularly in their application to vegetable cultivation within CEA environments such as greenhouses and plant factories. It also examines the challenges farmers face when adopting smart farming technologies. Lastly, the study outlines the national policies implemented by the government to encourage the adoption of these technologies by vegetable farmers.
THE REVOLUTION OF SMART FARMING TECHNOLOGY
World scenario
The smart agriculture roadmap in the agricultural industry aims to transition from traditional farming practices to smart farming techniques. In the 17th century, farmers used tools such as sickles, hoes, and grass cutters for cultivation, a period commonly referred to as Agriculture 1.0 (Rapela, 2019). This method required a significant amount of labor to perform agricultural activities; however, production levels remained low.
In the 20th century, Agriculture 2.0 emerged, introducing early-generation innovations that aimed to improve productivity. During this phase, various machines and agricultural equipment were developed for tasks such as harvesting, weeding, watering, sowing, and seed preparation, with the goal of reducing labor costs and increasing output. Additionally, advancements in technology during this period led to the widespread use of gas and oil as primary energy sources.
Moreover, improvements in transportation infrastructure contributed to a more advanced food supply chain, enabling the shipment of agricultural goods over longer distances.
In the third generation of agriculture (Agriculture 3.0), advancements in innovation, particularly in communication technology, software engineering, and the Internet of Things (IoT), have significantly enhanced the capabilities of modern agricultural equipment. The integration of renewable or green energy sources, including hydropower, photovoltaic systems, and wind power, is also being explored. Additionally, Agriculture 3.0 seeks to implement smart agricultural technologies such as crop forecasting, crop recommendation systems, yield monitoring, and knowledge-sharing platforms for farmers (Narvaez et al., 2017). In summary, these three agricultural revolutions have substantially increased crop productivity. Furthermore, traditional labor-intensive agricultural practices are being replaced by modern techniques.
The development of Agriculture 3.0 has begun to emphasize cost-effectiveness and increased productivity. However, several challenges must be addressed in the context of smart agriculture, such as digitization, the agri-food supply chain, ecological issues, and crop productivity. As a result, the integration of smart technologies is essential to advancing agricultural practices (De Clercq et al., 2018). Fourth-generation agriculture (Agriculture 4.0) was introduced to address the limitations of its predecessor, combining several innovative technologies such as big data, machine learning, deep learning, artificial intelligence, IoT, blockchain, robotics, autonomous systems, cyber-physical systems, and computing. This integration of technologies is commonly referred to as smart farming.
Smart farming practices differ significantly from traditional farming due to the incorporation of equipment, technology, machines, devices, sensors, information technology, and computing systems. The future of agriculture will rely heavily on advanced tools and technologies such as GPS, robotics, humidity sensors, and aerial imagery. The development of smart farming systems integrates several techniques, including real-time data collection and processing. Consequently, the shift towards smart agriculture is expected to transform the entire agricultural ecosystem, fostering modern farm management.
By utilizing real-time data, decision support systems can enhance productivity, optimize resource allocation, adapt to climate change, improve food supply chains, and detect crop diseases more efficiently. Recent examples of smart agricultural technology include innovations in automated disease diagnosis using computational systems (Shah et al., 2016; Pantazi et al., 2019; Kaur & Bhatia, 2020). The continuous monitoring and classification of plant diseases have become a major area of interest for researchers and agronomists (Singh & Misra, 2017). Over the past decade, numerous researchers have introduced technologies that have revolutionized the global agricultural landscape.
Kamilaris et al., (2017) conducted a systematic review of recent studies on big data in agriculture, offering solutions to pertinent challenges. Van Klompenburg et al., (2020) conducted a survey to extract and synthesize the characteristics of machine learning algorithms applied in agriculture. Kamilaris and Prenafeta-Boldú (2018) discussed several deep learning models used in various agricultural and food production applications. Elijah et al., (2018) proposed an IoT ecosystem that merges smart agricultural technological innovation with business and product marketability. Sattari et al., (2021) demonstrated an AI-based technique to address real-time optimization challenges in smart agriculture. Finally, Kamilaris et al., (2019) explored the impact of blockchain technology on food supply chains.
Malaysian scenario
Agricultural technology adoption in Malaysia has been evolving, with a growing emphasis on enhancing productivity, sustainability, and efficiency. The country is increasingly leveraging technologies such as precision farming, the Internet of Things (IoT), automation, and digital tools to modernize its agricultural sector. These advancements aim to address labor shortages, improve climate resilience, and promote sustainable practices (World Bank, 2019).
Specifically, Malaysia's agricultural development progresses in parallel with advancements in science and technology, with IR4.0 technologies marking a new paradigm for Agriculture 4.0 (De Clercq et al., 2018; Yahya, 2018; Klerkx et al., 2019). In this paradigm, land preparation, planting, and crop management—including weeding, pest control, and harvesting—are driven by digitalization, automation, IoT, robotics, and artificial intelligence (AI) (Yahya, 2018). There are two primary approaches to achieving Agriculture 4.0: integrating IR4.0 technologies into existing systems or developing entirely new systems when integration is not feasible or when no existing system is available (Santos Valle & Kienzle, 2020).
In Malaysia, most smart agricultural systems have been developed by integrating IR4.0 technologies into existing frameworks. For example, a tractor can be transformed into an Unmanned Guided Vehicle (UGV) by incorporating various sensors, such as ultrasonic sensors, compasses, gyroscopes, accelerometers, and AI systems (Grigore et al., 2020). Simultaneously, mapping can be performed through the integration of GPS and GNSS with advanced sensors and light detection (LiDAR) systems to create an intelligent navigation system (Zhou et al., 2021). This modified machine can prepare land without the need for labor.
Among the latest examples of smart agricultural technology is the use of systems that automatically diagnose plant diseases using computers (Shah et al., 2016; Pantazi et al., 2019; Kaur and Bhatia, 2020). The detection and classification of plant diseases through continuous monitoring has become a major focus for researchers and agronomists (Singh and Misra, 2017). Over the past decade, several researchers have introduced technologies that have transformed agricultural systems worldwide. For instance, Kamilaris et al., (2017) conducted a systematic review of recent research in the field of big data in agriculture, providing solutions to related challenges. Van Klompenburg et al., (2020) conducted a survey to extract and synthesize the characteristics of machine learning algorithms used in agriculture. Kamilaris and Prenafeta-Boldú (2018) highlighted several deep learning models used in various agricultural and food production applications. Elijah et al., (2018) proposed an IoT ecosystem that combines smart agricultural technology with innovations in business and product marketability. Sattari et al. (2021) demonstrated an AI-based technique to address real-time optimization challenges in smart agriculture. Kamilaris et al. (2019) explored the effects of blockchain technology on the food supply chain.
In the soil preparation stage, lightweight autonomous soil parameter retrieval robots can be used to generate soil fertility maps. The combination of the intelligent autonomous system developed and the automatic index system will be a smart automatic levelling machine for land preparation in rice fields (Abu Bakar et al., 2019). For crop management, fertilizer and pesticide applications can be done intelligently with a combination of Unmanned Aerial Vehicles (UAV) and autonomous fertilizer application or chemical spraying systems using variable-rate technology (VRT) in rice production. GIS-based mapping of soil fertility and plant conditions obtained by the UAV is processed and analyzed with AI systems to produce treatment maps. The treatment map enables specific crop management in the planting plot with autonomous systems or smart VRT drones to apply fertilizers and pesticides in the right amount, in the right place and at the right time. Therefore, it can optimize the use of fertilizers and pesticides without waste (ST Ten et al., 2023). The implementation of precision farming technologies mentioned above has been applied to the cultivation of rice, palm oil, and rubber in Malaysia. (Ramli et al., 2022).
Controlled Environment Agriculture scenario in Malaysia
The concept of Controlled Environment Agriculture (CEA) encompasses agricultural practices that optimize environmental conditions for plant growth. These cultivation activities are conducted inside structures such as greenhouses, rain shelters, and indoor plant factories (Game and Primus, 2015; AlKodmany, 2018). CEA technology monitors and controls key parameters for plant growth, including nutrient solution levels, light quality and quantity, temperature, relative humidity, and carbon dioxide (CO2) levels (Zeidler & Schubert, 2014). Despommier (2011) demonstrated that CEA is more advantageous than traditional agriculture because it enables year-round production, protects crops from natural disasters (e.g., typhoons, floods, and droughts), requires no heavy machinery, and minimizes water use. Moreover, Zeidler and Schubert (2015) emphasized that CEA facilitates consumer access to local produce and ensures a stable food supply. Despommier (2018) predicts that this agricultural concept will be commercialized globally within the next 10 to 20 years.
In Malaysia, indoor cultivation systems, such as plant factories, have been emerging since 2016, with most located in the Klang Valley region (Ahmad et al., 2024). A plant factory is an intelligent agricultural system designed for vegetable production. It employs vertical farming techniques and is fully integrated with IoT-based smart control and monitoring systems. These systems allow for comprehensive monitoring and regulation via artificial intelligence (AI), managing the building’s microclimate, including temperature, humidity, CO2 levels, airflow, hydroponic systems, and artificial lighting (ST Ten et al., 2023).
The plant factory’s controlled-environment system comprises three main components: the mobile application system, environmental sensors, and the repeater module. The installed sensors include temperature, humidity, CO2, and airflow sensors. The e-farm application, developed on the Android platform, displays data on a tablet and enables control through the mobile app to ensure optimal growing conditions. For example, the optimal temperature range for lettuce is estimated to be between 23°C and 28°C, with a relative humidity of 60% to 70%. Monitoring and control systems are essential for micro-irrigation systems, especially to meet the specific growth requirements of plants. Depending on the growth conditions, plants need certain levels of nutrients (Ahmad et al., 2022). Micro-irrigation systems consist of a fertigation tank, a water pump, an electric injector for fertilizer, and a control system. The sensor system includes a pH sensor, an electrical conductivity (EC) sensor, and a water temperature sensor. These sensors are connected to a Supervisory Control and Data Acquisition (SCADA) system, which sets parameters for EC and pH, and the data is visualized through the SCADA control panel. Irrigation data is also stored on a local server located in a controlled room, and all data is synced to cloud storage and can be viewed on a dashboard (Ahmad et al., 2022; ST Ten et al., 2023). This system has been integrated into the IoT framework by modifying port connections and extending it to Wi-Fi and 4G LTE connections. Consequently, the smart agriculture-based cultivation in these plant factories is expected to increase productivity per unit area by 4 to 6 times, producing high-quality, nutritious, and pesticide-free vegetables (Sayuti, 2022).
TECHNOLOGY USED FOR VEGETABLE FARMING ACTIVITIES USING CEA CONCEPT IN MALAYSIA
Greenhouse
In this study, the technology used by most of the farmers in Malaysia is also listed to give a clear picture and the actual scenario of vegetable cultivation in a controlled agricultural system. The list of technologies applied in greenhouses is based on the vegetable cultivation technology manual published by MARDI. These technologies encompass various stages of the cultivation process, from seedling management to post-harvest handling. A more detailed overview is provided in Table 1.
Table 1. The technology used for vegetable cultivation in greenhouse from seedling to harvest
No.
Activity
Component
1.
Seedlings
2.
Cultivation
3.
Fertilization
4.
Irrigation
5.
Pest and disease control
6.
Weed management
7.
Harvesting and postharvest handling
Source: MARDI Vegetable Cultivation in Greenhouse Manual (MARDI, 2022)
Plant factory
The list of technology listed in this paper is based on the plant factory technology book published by MARDI. Research for plant factory technology is one of the initiatives taken by MARDI to develop plant factory technology that can be used as a guide for entrepreneurs. These technologies encompass various stages of the cultivation process, from seedling management to post-harvest handling are shown in Table 2.
Table 2. The technology used for vegetable cultivation in plant factory from seedling to harvest
No.
Activity
Component
1.
Seedling
2.
Planting
3.
Fertilization
4.
Irrigation
5.
Pest and disease control
7.
Harvesting and post-harvest handling
Source: MARDI Plant Factory Technology (MARDI, 2022)
CHALLENGES TO ADOPTING SMART AGRICULTURE TECHNOLOGY
Controlled Environment Agriculture (CEA) refers to advanced agricultural practices such as hydroponics, aeroponics, and vertical farming, where conditions like light, temperature, humidity, and nutrient levels are meticulously controlled. While these technologies are widely known and already employed by many vegetable farmers, the integration of smart technologies (e.g., sensors, automation, data analytics, and IoT) can further enhance productivity and resource efficiency. However, the adoption of smart agriculture technology in CEA has been slower compared to other industries, largely due to several significant challenges.
One of the primary obstacles is the high cost of implementing smart farming technologies, including climate control systems, advanced sensors, and automated irrigation, which are especially burdensome for smallholder farmers. These farmers often lack the financial resources necessary for such investments. Although there are government initiatives to subsidize smart farming, the financial support provided may be insufficient for widespread adoption. Farmers are also concerned about the return on investment (ROI), particularly since the ROI for smart technologies tends to be longer (Baumont de Oliveira et al., 2022).
Another key challenge is the limited technical expertise and skills required for successful adoption. Smart technology in CEA demands a strong understanding of digital tools, data analytics, and automation systems. In Malaysia, many farmers, particularly those in rural areas, lack the technical knowledge needed to operate and maintain these systems. Continuous education is essential to keep farmers abreast of rapidly evolving technologies. The lack of access to such resources hampers the uptake of smart farming (Lioutas and Charatsari, 2020).
Infrastructure and internet connectivity are also crucial factors that must be addressed. Many smart technologies rely on consistent and reliable internet access for monitoring and data management. In rural areas of Malaysia, internet connectivity can be unreliable or entirely unavailable, limiting the use of IoT devices and cloud-based systems in CEA (Gong, 2023). Additionally, controlled environments that depend on artificial lighting, climate control, and other automated systems require a stable and continuous electricity supply. In regions where electricity is inconsistent, it becomes challenging to implement and maintain these smart technologies.
While Controlled Environment Agriculture (CEA) systems such as greenhouses or plant factories are energy-intensive, the high cost of electricity in Malaysia can make these systems expensive to operate, affecting the long-term viability of adopting smart technologies. Additionally, the maintenance of smart equipment adds to the financial burden. Therefore, research is needed to reduce energy usage in plant factories and controlled environments, with a focus on developing energy-efficient technologies. One alternative is the use of solar power (Kubo et al., 2016). Another example is the use of reflectors, which can minimize the quantity of LED lights needed in plant factories. According to Shaari et al. (2021), a large portion of energy consumption in plant factories comes from air-conditioning systems (51%) and LED lighting (36%). Thus, installing reflectors to reduce LED use would contribute to lower energy consumption.
Moreover, smart farming technologies generate large volumes of data—such as soil moisture levels, temperature, light intensity, and nutrient status—that many farmers may struggle to interpret effectively without specialized software or training. Mismanagement of this data can lead to inefficiencies in farm operations, counteracting the benefits of smart technologies. Additionally, the components of smart farming systems, such as sensors, irrigation, and climate control, may not always be fully integrated. This fragmentation can result in isolated operations where systems work independently, reducing the overall effectiveness of the CEA setup (Wolfert et al., 2017).
The profitability of investing in smart technologies for vegetable cultivation is influenced by market demand and price fluctuations. Malaysian farmers may be hesitant to adopt expensive systems due to uncertainty regarding economic returns, particularly given that vegetable prices can vary with seasonality, imports, and consumer demand. While CEA enables high-quality vegetable production, smallholder farmers may struggle to access premium markets that offer higher prices for such produce. Without guaranteed higher income, investing in smart technologies becomes riskier (Ahmad et al., 2024).
Another critical factor is cultural resistance and traditional farming practices (Ahmad et al., 2023). Many Malaysian farmers are accustomed to methods passed down through generations, and the perceived complexity and novelty of smart farming technologies can lead to skepticism. Farmers may be hesitant to abandon established methods, particularly in regions with an aging farming population, where older farmers are less inclined to adopt new technologies. Younger generations, who might be more open to smart farming, often migrate to urban areas, leaving older farmers in charge of operations.
Although Malaysia has implemented initiatives such as the National Agri-Food Policy (NAP 2.0) and the Smart Farming Initiative, these policies may not fully address the unique challenges faced by small-scale vegetable farmers in adopting smart technologies. The bureaucratic process of accessing grants and subsidies can be slow and complicated, discouraging farmers from seeking government assistance. Moreover, a lack of standardized regulations governing the use of smart technologies in agriculture can result in farmers purchasing substandard or incompatible equipment, further hindering adoption.
In conclusion, adopting smart technologies for vegetable cultivation within CEA systems in Malaysia presents significant challenges, including high costs, limited technical knowledge, infrastructure constraints, and market uncertainties. Overcoming these barriers will require collaborative efforts between government bodies, financial institutions, educational institutions, and the private sector. Addressing these challenges through targeted policies, improved training, financial support, and better infrastructure can help accelerate the adoption of smart farming technologies in Malaysia’s agricultural sector.
NATIONAL POLICY TO SUPPORT ADOPTING SMART AGRICULTURE TECHNOLOGY
Agri-food sector contribute income to the Malaysia economy with Gross Domestic Product (GDP) growth increased 6.8% which is US$11.43 billion in 2020 compared to US$6.3 billion in 2011. The government implement policies to expedite smart agriculture transformation in Malaysia. Among the policies are:
• National Agri-Food Policy (2021-2030)
• National Fourth Industrial Revolution (4IR) policy (2021-2030)
• National Food Security Action Plan (2021-2025)
National Agri-Food Policy 2.0 (NAP 2.0)
Launched as an updated version of the National Agri-Food Policy, this policy emphasizes the modernization of agriculture by encouraging the adoption of smart farming technologies. One of the key targets is improving the efficiency of food production, particularly vegetables, through the application of precision agriculture, IoT (Internet of Things), AI, drones, and data analytics. The policy also includes initiatives to promote research, development, commercialization, and innovation (R&D&C&I) in agriculture, aimed at encouraging the creation and adoption of new smart farming solutions.
National Fourth Industrial Revolution (4IR) policy (2021-2030)
The Malaysia National Fourth Industrial Revolution (4IR) Policy (2021-2030) is a strategic plan aimed at driving Malaysia’s transition into the Fourth Industrial Revolution. This policy outlines the country's approach to adopting advanced technologies and fostering innovation to boost economic growth and enhance competitiveness. Key components of Malaysia's 4IR policy include promoting the use of technologies like AI, IoT, and robotics across various sectors. Developing robust digital infrastructure to support the implementation of 4IR technologies. Secondly, is enhancing skills and training programs to prepare the workforce for new technological demands. The third is to encourage research and development, as well as fostering collaboration between public and private sectors. The policy also plans to establish guidelines to ensure the ethical and secure use of new technologies and it will also be supporting the growth of emerging industries and reducing dependency on traditional sectors. The goal is to position Malaysia as a regional leader in 4IR technologies, enhance its global competitiveness, and ensure sustainable economic development.
National Food Security Action Plan (2021-2025)
The Malaysia National Food Security Action Plan (2021-2025) is a strategic initiative aimed at strengthening the country’s food security by enhancing agricultural productivity, improving supply chain resilience, and promoting sustainable practices. It focuses on boosting local food production, supporting farmers, and ensuring equitable access to affordable, nutritious food for all Malaysians. The plan also emphasizes the importance of monitoring and evaluating progress to adapt and refine strategies, ultimately seeking to reduce reliance on food imports and enhance overall food security.
Malaysia Digital Economy Blueprint (MyDIGITAL)
MyDIGITAL, which outlines Malaysia’s digital transformation, includes provisions for developing a smart agriculture ecosystem. It encourages the use of IoT, AI, and data analytics in agriculture. The blueprint provides a framework for integrating smart farming into the national digital infrastructure and aims to make Malaysia a regional leader in digital agriculture technologies.
CONCLUSION
In conclusion, smart farming technologies present a transformative opportunity for Malaysia’s agricultural sector, particularly in the realm of controlled environment agriculture (CEA). The adoption of these technologies is hindered by high costs, limited technical knowledge, and inadequate infrastructure—challenges that are particularly pronounced for small-scale farmers. Government interventions including subsidies, financial support, and specialized training programs, are essential to mitigating these barriers. National frameworks like the National Agri-Food Policy and the 4IR Policy are already facilitating this transition by promoting innovation and investment in smart farming. Collaborative efforts between the government, the private sector, and farmers will be crucial to addressing the remaining challenges and accelerating the adoption of smart farming technologies. Such efforts will ensure that Malaysia’s agricultural sector remains sustainable and competitive in the global market.
RECOMMENDATION
To accelerate the adoption of smart farming technologies among vegetable farmers in Malaysia, several key actions are recommended. First, the government should enhance financial support by providing more targeted subsidies, low-interest loans, and grants specifically for the acquisition of smart farming technologies like sensors, automated irrigation systems, and drones. Expanding comprehensive training programs is also crucial to equipping farmers with the technical skills needed to effectively use these technologies. Strengthening partnerships with private sector innovators and technology providers can help reduce the cost of advanced agricultural solutions, making them more accessible to small-scale farmers. Additionally, improving digital infrastructure in rural areas, such as internet connectivity, will ensure farmers can fully leverage IoT-based systems. Promoting the success stories of early adopters and offering incentives for pioneers can also build trust and encourage more widespread acceptance of smart farming practices. Finally, simplifying the bureaucratic processes for accessing government support and ensuring continuous education will ensure sustained progress in transforming Malaysia’s agriculture sector.
REFERENCES
Abu Bakar, B., Ahmad, M. T., Ghazali, M. S. S., Abd Rani, M. N. F., Mhd Bookeri, M. A., Abdul Rahman, M. S., ... & Ismail, R. (2020). Leveling-index based variable rate seeding technique for paddy. Precision Agriculture, 21, 729-736.
Ahmad, M.A., Basir, M.H., Tahir, M.A.M., Shafie, K.A. & Sulaiman, A.S.S. (2022). Sistem Pengeluaran dalam Kilang Tanaman. In Sayuti, Z., Ahmad, M.A., Yusoff, M.M, & Amaddin, P.A.M (Eds.), Kilang Tanaman MARDI: Teknologi Moden Pengeluaran Tanaman Makanan in Malaysia. Penerbit MARDI.
Ahmad. A.A, Omar, N.R.N, Yusoff M.M, & Ahmad. M.A. (2024). From Farm to Table Concept: The Profile and Dynamics of Urban Farming on Agrofood Supply Chain in Malaysia. FFTC Journal of Agricultural Policy. 17-34. Vol 2.
Ahmad, A. A., Kasron, N., Noh, N. H. M., Azmon, H., & Chuang, T. C. (2023). The acceptance of decision support system (DSS) in vegetable cultivation among farmers in Malaysia. Economic and Technology Management Review, Vol. 21:1-18.
Al-Kodmany, K. (2018). The vertical farm: A review of developments and implications for the vertical city. Buildings, 8(2), 24.
Baumont de Oliveira, F. J., Ferson, S., Dyer, R. A., Thomas, J. M., Myers, P. D., & Gray, N. G. (2022). How high is high enough? Assessing financial risk for vertical farms using imprecise probability. Sustainability, 14(9), 5676.
Benke, K., & Tomkins, B. (2017). Future food-production systems: Vertical farming and controlled-environment agriculture. Sustainability: Science, Practice and Policy, 13(1), 13-26. https://doi.org/10.1080/15487733.2017.1394054
De Clercq, M., Vats, A., & Biel, A. (2018). Agriculture 4.0: The future of farming technology. Proceedings of the world government summit, Dubai, UAE, 11-13.
Despommier, D. (2011). The vertical farm: Controlled environment agriculture carried out in tall buildings would create greater food safety and security for large urban populations. Journal für Verbraucherschutz und Lebensmittelsicherheit, 6(2), 233-236. https://doi.org/10.1007/s00003-010-0654-3
Despommier, D. (2011). The vertical farm: Controlled environment agriculture carried out in tall buildings would create greater food safety and security for large urban populations. Journal für Verbraucherschutz und Lebensmittelsicherheit, 6(2), 233-236. https://doi.org/10.1007/s00003-010-0654-3
Despommier, D. (2018). The Vertical Farm: Feeding the world in the 21st century. Retrieved October 19, 2021, from http://www.verticalfarm.com/?page_id=75
DOSM. (2023). Compendium of environment statistics, Malaysia 2019. Department of Statistics Malaysia. Retrieved July 15, 2024, from https://open.dosm.gov.my/ms-MY/data-catalogue/population_malaysia
Elijah, O., Rahman, T. A., Orikumhi, I., Leow, C. Y., & Hindia, M. N. (2018). An overview of Internet of Things (IoT) and data analytics in agriculture: Benefits and challenges. IEEE Internet of things Journal, 5(5), 3758-3773.
FAO. (2011a). AQUASTAT country profile - Malaysia. Food and Agriculture Organization of the United Nations, Rome. Retrieved August 29, 2021, from http://www.fao.org/aquastat/en/countries-and-basins/country-profiles.
Game, I., Primus, R. (2015). GSDR 2015 Brief: Urban Agriculture End Hunger, Achieve. Food Security and Improved Nutrition and Promote Sustainable Agriculture.
Gong, Rachel. 2023. Connecting the Last Mile: Solutions for Rural and Remote Communities. Kuala Lumpur: Khazanah Research Institute. License: Creative Commons Attribution CC BY 3.0. Available at: https://www.krinstitute.org/assets/contentMS/img/template/editor/230731%20rural%20connectivity%20v1.5.1%20pub%202.pdf (Accessed: 16 September 2024).
HRD Corp. (2019). HRDF Human Capital Report (Agriculture Sector Overview: Will Agriculture Be The Next Sector Covered Under PSMB Act?). Human Resources Development Corporation.
Kamilaris, A., & Prenafeta-Boldú, F. X. (2018). Deep learning in agriculture: A survey. Computers and electronics in agriculture, 147, 70-90.
Kamilaris, A., Kartakoullis, A., & Prenafeta-Boldú, F. X. (2017). A review on the practice of big data analysis in agriculture. Computers and Electronics in Agriculture, 143, 23-37.
Kamilaris, A., van den Brink, C., & Karatsiolis, S. (2019). Training deep learning models via synthetic data: Application in unmanned aerial vehicles. In Computer Analysis of Images and Patterns: CAIP 2019 International Workshops, ViMaBi and DL-UAV, Salerno, Italy, September 6, 2019, Proceedings 18 (pp. 81-90). Springer International Publishing.
Kaur, M., & Bhatia, R. (2020). Leaf disease detection and classification: A comprehensive survey. In Proceedings of International Conference on IoT Inclusive Life (ICIIL 2019), NITTTR Chandigarh, India (pp. 291-304). Springer Singapore.
Klerkx, L., Jakku, E., & Labarthe, P. (2019). A review of social science on digital agriculture, smart farming and agriculture 4.0: New contributions and a future research agenda. NJAS-Wageningen journal of life sciences, 90, 100315.
KPKM (2023). National Food Security Policy Action Plan 2021-2025. Putrajaya: Ministry of Agriculture and Food Security. Retrieved from https://www.kpkm.gov.my/en/agro-food-policy/pelan-tindakan-dasar-sekuriti-makanan-2021-2025
KPKM.(2021). National Agrofood Policy 2021-2030 (NAP 2.0). Putrajaya: Ministry of Agriculture and Food Security. Retrieved from https://www.kpkm.gov.my/en/agro-food-policy/national-agrofood-policy
Kubo, H., Murayama, S., Tanimoto, M., Okoso, K., & Maeno, S. (2016, September). A possibility of open zero energy plant factory. In 2016 Electronics Goes Green 2016+(EGG) (pp. 1-8). IEEE.
Lioutas, E. D., & Charatsari, C. (2020). Smart farming and short food supply chains: Are they compatible?. Land Use Policy, 94, 104541.
Malaysian Agricultural Research and Development Institute, MARDI (2022). Manual Teknologi Penanaman Kubis Bunga Tanah Rendah di Bawah Struktur Pelindung Hujan (Penerbit MARDI). Malaysia.
Malaysian Agricultural Research and Development Institute, MARDI (2022). Kilang Tanaman MARDI (Penerbit MARDI). Malaysia.
Ministry of Economy (2021). Malaysia Digital Economy Blueprint (MyDIGITAL). Putrajaya: Ministry of Economy. https://www.ekonomi.gov.my/sites/default/files/2021-02/malaysia-digital-economy-blueprint.pdf
Ministry of Economy (2021). National Fourth Industrial Revolution (4IR) Policy (2021-2030). Putrajaya: Ministry of Economy. https://www.ekonomi.gov.my/sites/default/files/2021-07/National-4IR-Policy.pdf
Narvaez, F. Y., Reina, G., Torres-Torriti, M., Kantor, G., & Cheein, F. A. (2017). A survey of ranging and imaging techniques for precision agriculture phenotyping. IEEE/ASME Transactions on Mechatronics, 22(6), 2428-2439.
Pantazi, X. E., Moshou, D., & Tamouridou, A. A. (2019). Automated leaf disease detection in different crop species through image features analysis and One Class Classifiers. Computers and electronics in agriculture, 156, 96-104.
Ramli, Z. F., Abd Karim, H., Amirnordin, N. A., Zainal, D. N., Md Akhir, N., & Tengku Sulaiman, T. S. R. M. (2022). Pelaksanaan teknologi pertanian tepat, mekanisasi, automasi dan aplikasi teknologi Internet of Things (IoT) dalam sektor pertanian di Malaysia. ASPIRASI FPP, 35-39.
Rapela, M. A. (2019). Fostering innovation for agriculture 4.0. Springer International Publishing.
Santos Valle, S., & Kienzle, J. (2020). Agriculture 4.0–agricultural robotics and automated equipment for sustainable crop production.
Sattari, M. A., Roshani, G. H., Hanus, R., & Nazemi, E. (2021). Applicability of time-domain feature extraction methods and artificial intelligence in two-phase flow meters based on gamma-ray absorption technique. Measurement, 168, 108474.
Sayuti. Z. (2022). Prospek, Status Semasa dan Hala Tuju Pertanian Moden. (2022). In Sayuti, Z., Ahmad, M.A., Yusoff, M.M.,& Amaddin, P.A.M (Eds.), Kilang Tanaman MARDI: Teknologi Moden Pengeluaran Tanaman Makanan in Malaysia. Penerbit MARDI
Sengodan, P. (2022). An Overview of Vertical Farming: Highlighting the Potential in Malaysian High-Rise Buildings. Pertanika Journal of Science & Technology, 30(2).
Shaari, A. M., Razuan, M. S. M., Taweekun, J., Batcha, M. F. M., Abdullah, K., Sayuti, Z., ... & Tahir, M. A. M. (2021). Energy Profiling of a Plant Factory and Energy Conservation Opportunities. Journal of Advanced Research in Fluid Mechanics and Thermal Sciences, 80(1), 13-23.
Shah, J. P., Prajapati, H. B., & Dabhi, V. K. (2016, March). A survey on detection and classification of rice plant diseases. In 2016 IEEE International Conference on Current Trends in Advanced Computing (ICCTAC) (pp. 1-8). IEEE.
SharathKumar, M., Heuvelink, E., & Marcelis, L. F. M. (2020). Vertical farming: Moving from genetic to environmental modification. Trends in Plant Science, 25(8), 724-727. https://doi.org/10.1016/j.tplants.2020.05.012
Singh, V., & Misra, A. K. (2017). Detection of plant leaf diseases using image segmentation and soft computing techniques. Information processing in Agriculture, 4(1), 41-49.
Somerville, C., Cohen, M., Pantanella, E., Stankus, A., & Lovatelli, A. (2014). Small-scale aquaponic food production-Integrated fish and plant farming. Food and Agriculture Organization Rome.
Ten, S. T., Shafie, K. A. B., & Abdul Rani, R. B. (2023). Achieving agriculture 4.0 through modernization and enhancement with mechanization, automation and advanced technologies. In Smart Agriculture for Developing Nations: Status, Perspectives and Challenges (pp. 49-58). Singapore: Springer Nature Singapore.
United Nation. (2019). World population prospects 2019: Highlights. Population Division. http://creativecommons.org/licenses/by/3.0/igo/
Van Klompenburg, T., Kassahun, A., & Catal, C. (2020). Crop yield prediction using machine learning: A systematic literature review. Computers and electronics in agriculture, 177, 105709.
Wolfert, S., Ge, L., Verdouw, C., & Bogaardt, M. J. (2017). Big data in smart farming–a review. Agricultural systems, 153, 69-80.
World Bank. (2019). Agricultural Transformation and Inclusive Growth: The Malaysian Experience. https://openknowledge.worldbank.org/server/api/core/bitstreams/1c25a5d4-fbc0-5325-8e44-1ccef47c82f5/content
Yahya, N. (2018). Agricultural 4.0: Its implementation toward future sustainability. Green Urea: For Future Sustainability, 125-145.
Yahya, T. M. T. (2001). Crop diversification in Malaysia. In M. K. Papademetriou & F. J. Dent (Eds.), Crop Diversification in the asia-pacific region (pp. 71-87). Food and Agriculture Organisation of the United Nations.
Zeidler, C., & Schubert, D. (2014). From bioregenerative life support systems for space to vertical farming on earth - The 100% spin-off . In Life in Space for Life on Earth Symposium 2014, Waterloo, Canada.
Zeidler, C., and Schubert, D. (2015). Vertical Farm 2.0: Designing an Economically Feasible Vertical Farm – A Combined European Endeavor for Sustainable Urban Agriculture (Association for Vertical Farming). https://www.researchgate.net/ publication/321427717
Zhou, T., Hasheminasab, S. M., & Habib, A. (2021). Tightly-coupled camera/LiDAR integration for point cloud generation from GNSS/INS-assisted UAV mapping systems. ISPRS Journal of Photogrammetry and Remote Sensing, 180, 336-356.