ABSTRACT
This work reports the development of a precision farming technology package by the Malaysian Agricultural Research and Development Institute (MARDI) and the willingness of Malaysian farmers to adopt to the technology. Specifically, this work touches on the efforts to reduce fertilizer use in large-scale paddy production. The precision farming technology package comprising of variable rate fertilizer application has the potential to save input cost. The technique calls for inputs such as fertilizers to be applied based on the actual need of the plants. This data rich approach enables farm owners and estate managers to make informed decisions in managing their crops. A survey of farmers in three paddy granary areas was conducted to gauge the willingness of Malaysian farmers to engage in this new technology. It was found that farmers were generally open to the idea. However some skepticism remain to be addressed regarding the cost to benefit ratio.
Keywords: Precision Farming, Variable Rate Technology (VRT), paddy, fertilizer
INTRODUCTION
Paddy is one of the major agro-food commodities in Malaysia with a planted area of 685,548 hectares and a production of about 2.57 million tons nationwide in 2017 (MOA, 2017). This represented a production value of about 737.9 thousand USD. The self-sufficiency level in Malaysia for this crop hovered around 70% from the year 2008 – 2017 with an average yield of 3.75 tons per hectare.
The National Agrofood Policy [NAP] 2011-2020 was introduced by the government to address three main issues: food supply and safety; competitiveness and sustainability of the industry; and increasing the income level of its target groups (MOA, 2011). Precision farming is a technology that has the potential to address issues mentioned above. It incorporates information and technology to achieve site-specific crop management.
Variable rate technology or VRT is one component of the precision farming technology package (Bakhtiari & Hematian, 2013; Sawyer, 1994). It refers to technologies that are used to enable the variable rate application (VRA) of crop inputs such as fertilizers, herbicides, lime and seeds. In the case of fertilizers, the strategy in the past was to ensure that potential yield is met and this was to apply enough quantity of fertilizer to cover any uncertainties. It was found that applying quantities of fertilizer more than required actually harmed not only the crop production and potential yield, but also the ecosystem (Gastal & Lemaire, 2002). In contrast, the VRA approach in fertilizer management determines the amount of applied fertilizer depending on the level of crop growth variability. This ensures that the right amount of fertilizer is applied at the right time to obtain optimal yield and minimize its impact on the environment.
There are two basic methods of VRA: map-based and sensor based. Map-based VRA systems depend on an electronic prescription map to adjust the application rate of inputs. The map is generated by sampling soil or crop data across points in a field using a positioning system such as a GPS or a grid. One advantage of this system is that the user has a database that can assist in management-related decisions such as the acquired knowledge of the needed amount of chemicals or inputs prior to entering a field. Another advantage is that the user has more control of the applicate rate planning. On the other hand, some disadvantages to this system are the cost and the fairly complicated processing that are needed to generate a map. There is also the time delay between crop properties measurement and application of inputs. Examples of research work on this type of system can be seen in (Daud et al., 2014; Fraisse, Sudduth, & Kitchen, 2001; Han, Evans, Hodges, & Rawlins, 1995; Teoh, Hassan, Radzali, & Jafni, 2012; Weisz, Fleischer, & Smilowitz, 1995). Companies such as PrecisionHawk, URSULA Agriculture Ltd. and Agribotix provide commercial solutions using map-based VRA.
Sensor-based VRA systems, on the other hand, adjust the application rate of inputs by measuring soil or crop properties “on the go” using sensors mounted on the applicator. The continuous stream of information is sent to an on-board controller which governs the application rate based on an algorithm or model. The advantage of this system is that it does not necessarily require a geo-referenced location system or a map. Another advantage is that there is no time delay between measurement of soil or crop properties and application of inputs. The system is working “on the go” or real-time. The sensors produce a far denser dataset than traditional sampling methods. Moreover, the system is self-contained which reduces the risk of communication and interfacing error. Research work on this type of system can be seen in (Holland & Schepers, 2010; Schepers & Holland, 2011).
Regardless of which category of VRA is chosen, they both require the process of soil or crop property measurement in order to generate a strategy to apply the input. With regards to fertilizer management, suitable soil or crop properties that relate to crop variability need to be measured in order for the VRA system to adjust fertilizer input rate.
(Gastal & Lemaire, 2002) found that crop growth variability is directly related to the crop’s nitrogen (N). Different methods have been developed to measure the N content (Daud, Hamid, & Mohamad Ghazali, 2010; Gitelson, 2003; Lawless, Semenov, & Jamieson, 2005; Mistele & Schmidhalter, 2008; Sieling, Böttcher, & Kage, 2013). Specific to paddy, (Gholizadeh, Amin, Anuar, & Aimrun, 2009) concluded that Soil Plant Analysis Development (SPAD) reading was a viable method to predict the paddy canopy total N amount and future crop N requirements.
PRECISION FARMING IN MALAYSIA
The Malaysian Agricultural Research and Development Institute or MARDI has introduced a precision farming technology package for paddy (Chan, 2013). A model was developed to determine the N uptake based on the green area index (GAI) of the plant (Daud et al., 2010). The authors determined that the best parameters to describe the volume of green material of the crop were plant population, canopy and shoot size. The GAI model can be used to calculate the deviation of N content of the actual grown crop with respect to the N content of a reference crop.
(Daud et al., 2014) developed a map-based fertilizer VRA system for paddy production based on the GAI model and SPAD readings. Figure 1 shows the flow diagram of the fertilizer application map generation process.
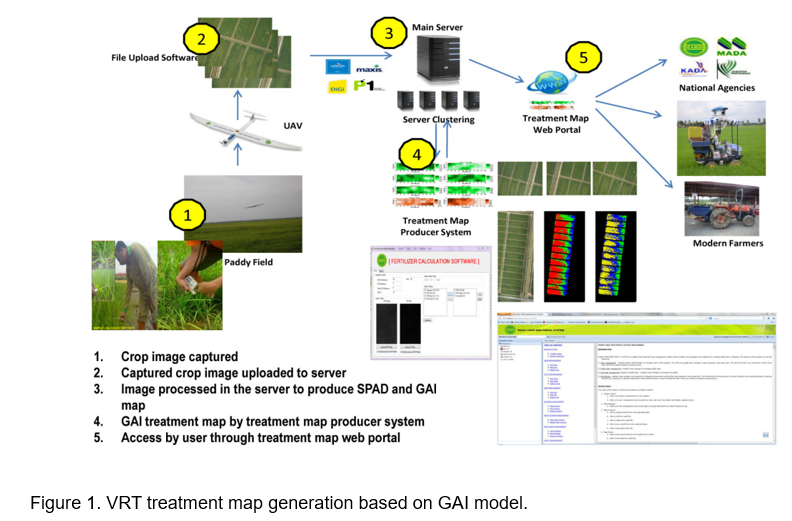
A fertilizer application map was generated from the developed model which can then be utilized by a variable rate applicator on the field. The process of generating an application map involved two stages. In the first stage, ground truth data had to be collected by going into the field and sampling a number of small quadratic area (25cm x 25cm) in each paddy field to manually count the tiller population in the area, measure the shoot size and taking a SPAD reading with a hand held device. An unmanned aerial vehicle (UAV) with a camera mounted underneath was then used to obtain images of the paddy fields. In the second stage, the images were manually transformed into SPAD and population map using the ground truth data collected as a reference. These two maps were then processed to produce a treatment map. Figure 2 shows the image processing steps.
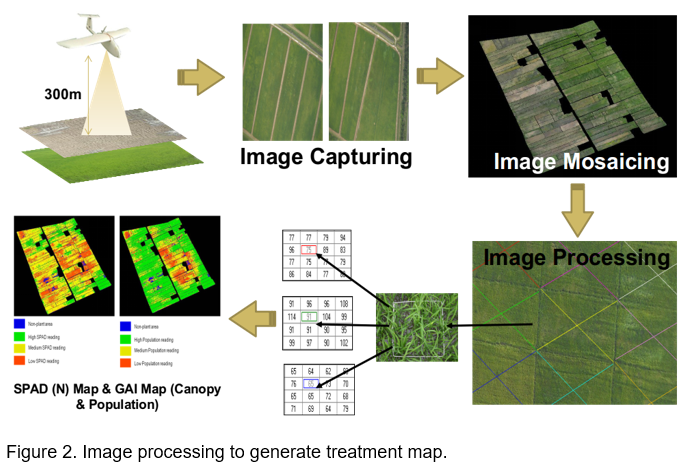
ACCEPTANCE OF PRECISION FARMING IN MALAYSIA
The developed precision farming technology package for paddy was introduced to three granary areas in Malaysia to gauge the acceptance and willingness to implement the new technology by local farmers. These granary areas were Muda granary area (MADA), Kemubu granary area (KADA), and the Integrated Agriculture Development Area (IADA) of Selangor. Figure 3 shows the locations of these granary areas in Malaysia. A series of seminars and workshops were conducted throughout 2017 and 2018 to expose the farmers to the concept of precision farming. A survey of the participants was conducted after the technology was demonstrated to them (Rahim, 2018 et. al.). A total of 400 respondents took part in the survey.

Figure 4 to figure 6 shows the result of the survey of the participants. The data showed that farmers from the three granary areas were generally keen to accept the technology.
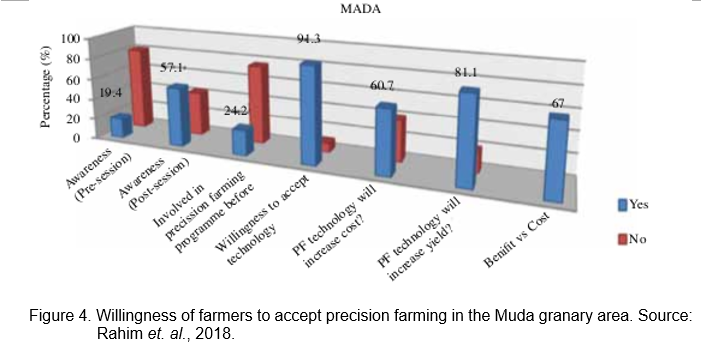
Almost all participants agreed that the implementation of precision farming technologies would be more expensive to implement.
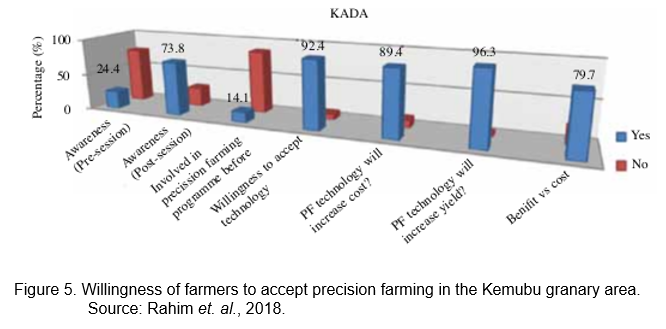
Just under 80% of farmers in the Kemubu granary area saw that the benefit of implementing precision farming outweighed the cost as compared 67% of farmers in the Muda granary area and 57% of farmers in the Selangor granary area. This may be due to the fact that the farmers in the Kemubu were willing to take more risks in return for higher income.
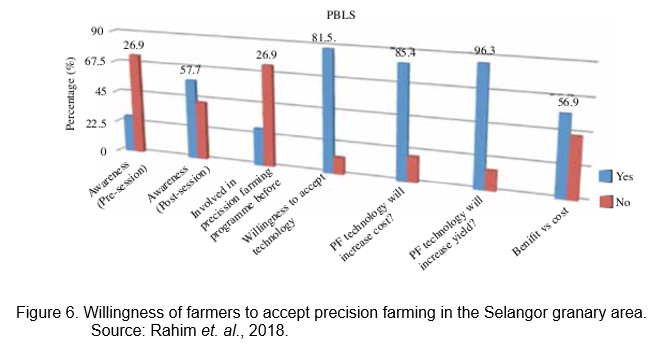
CONCLUSION
This work reported on the development of a variable rate application technique to reduce the amount of fertilizer use in large scale paddy production. It was argued that this precision farming technology could potentially be used to make the paddy production industry more efficient.
A survey was conducted to participants after exposing them to the new technology. It was found that the respondents were keen to implement the technology so long as the benefit outweighed the cost. The result from the survey could be used as a guide by the government in drafting policies that would promote the use of precision farming technologies in the future.
REFERENCES
Bakhtiari, A. A., and Hematian, A. (2013). Precision Farming Technology, Opportunities and Difficulty. International Journal for Science and Emerging Technologies with Latest Trends, 5(1), 1–14.
Chan, C. W. (2013). Precision Agriculture: The Way Forward in Mechanised Agriculture. Malaysian Agricultural Research and Development Institute (MARDI).
Daud, A. H., Abdul Rahman, S., Hamid, A., Teoh, C. C., Fakhrul Radzi, F. Z., and Rubiyah, Y. (2014). Variable Rate Fertilizer Application ( VRT ) for Rice Precision Farming Technology, In the proceedings of National conference on Agricultural food mechanization (NCAFM), May 2014, 20–22.
Daud, A. H., Hamid, A., and Mohamad Ghazali, M. S. S. (2010). Monitoring crop canopy development for nutrient management. In Proceeding of the national rice conference, 28-30 June (pp. pp. 450–453). Lumut, Perak.
Fraisse, C. W., Sudduth, K. A., and Kitchen, N. R. (2001). Delineation of site-specific management zones by unsupervised classification of topographic attributes and soil electrical conductivity. Transactions of the ASAE, 44(1), 155–166.
Gastal, F., and Lemaire, G. (2002). N uptake and distribution in crops: an agronomical and ecophysiological perspective. Journal of Experimental Botany, 53(370), 789–799. Gholizadeh, A., Amin, M. S.., Anuar, A. R., and Aimrun, W. (2009). Evaluation of SPAD Chlorophyll Meter in Two Different Rice Growth Stages and its Temporal Variability, European Journal of Scientific Research, 37(4), 591–598.
Gitelson, A. a. (2003). Remote estimation of leaf area index and green leaf biomass in maize canopies. Geophysical Research Letters, 30(5), 1248.
Han, S., Evans, R. G., Hodges, T., and Rawlins, S. L. (1995). Linking a geographic information system with a potato simulation model for site-specific crop management. Journal of Environmental Quality, 24(4), 772–777.
Rahim, H, Abdul Wahab, M.A.M., Mat Amin, M.Z., Harun, A., and Haimid, M.T. (2018). Perception and acceptance of farmers on precision farming technology in selected granary area. Economic and Technology Management Review, vol. 13, 97-110.
Holland, K. H., and Schepers, J. S. (2010). Derivation of a variable rate nitrogen application model for in-Season fertilization of corn. Agronomy Journal, 102, 1415–1424.
Lawless, C., Semenov, M. a., and Jamieson, P. D. (2005). A wheat canopy model linking leaf area and phenology. European Journal of Agronomy, 22(1), 19–32.
Mistele, B., and Schmidhalter, U. (2008). Estimating the nitrogen nutrition index using spectral canopy reflectance measurements. European Journal of Agronomy, 29(4), 184–190.
MOA. (2011). National Agrofood Policy 2011-2020. Percetakan Watan Sdn. Bhd.
MOA. (2017). Agrofood statistic 2017. Ministry of Agriculture and Agro-Based Industry Malaysia.
Sawyer, J. E. (1994). Concepts of variable rate technology with considerations for fertilizer application. Journal of Production Agriculture, 7(2), 195–201.
Schepers, J. S., and Holland, K. H. (2011). Active-crop sensor calibration using the virtual-reference concept. In J. V. Stafford (Ed.), Precision Agriculture ’11, p. 644, Wageningen: Wageningen Academic Publication.
Sieling, K., Böttcher, U., and Kage, H. (2013). Growth stage specific optima for the green area index of winter wheat. Field Crops Research, 148, 34–42.
Teoh, C. C., Hassan, D. A., Radzali, M. M., and Jafni, J. J. (2012). Prediction of SPAD chlorophyll meter readings using remote sensing technique, Journal of Tropical Agriculture and Food Science, 40(1), 127–136.
Weisz, R., Fleischer, S., and Smilowitz, Z. (1995). Site-specific integrated pest management for high value crops: sample units for map generation using the Colorado potato beetle (Coleoptera: Chrysomelidae) as a model system. Journal of Economic Entomology, 88(5), 1069–1080.
Saving Fertilizer in Malaysia's Large-Scale Paddy Production through Precision Farming
ABSTRACT
This work reports the development of a precision farming technology package by the Malaysian Agricultural Research and Development Institute (MARDI) and the willingness of Malaysian farmers to adopt to the technology. Specifically, this work touches on the efforts to reduce fertilizer use in large-scale paddy production. The precision farming technology package comprising of variable rate fertilizer application has the potential to save input cost. The technique calls for inputs such as fertilizers to be applied based on the actual need of the plants. This data rich approach enables farm owners and estate managers to make informed decisions in managing their crops. A survey of farmers in three paddy granary areas was conducted to gauge the willingness of Malaysian farmers to engage in this new technology. It was found that farmers were generally open to the idea. However some skepticism remain to be addressed regarding the cost to benefit ratio.
Keywords: Precision Farming, Variable Rate Technology (VRT), paddy, fertilizer
INTRODUCTION
Paddy is one of the major agro-food commodities in Malaysia with a planted area of 685,548 hectares and a production of about 2.57 million tons nationwide in 2017 (MOA, 2017). This represented a production value of about 737.9 thousand USD. The self-sufficiency level in Malaysia for this crop hovered around 70% from the year 2008 – 2017 with an average yield of 3.75 tons per hectare.
The National Agrofood Policy [NAP] 2011-2020 was introduced by the government to address three main issues: food supply and safety; competitiveness and sustainability of the industry; and increasing the income level of its target groups (MOA, 2011). Precision farming is a technology that has the potential to address issues mentioned above. It incorporates information and technology to achieve site-specific crop management.
Variable rate technology or VRT is one component of the precision farming technology package (Bakhtiari & Hematian, 2013; Sawyer, 1994). It refers to technologies that are used to enable the variable rate application (VRA) of crop inputs such as fertilizers, herbicides, lime and seeds. In the case of fertilizers, the strategy in the past was to ensure that potential yield is met and this was to apply enough quantity of fertilizer to cover any uncertainties. It was found that applying quantities of fertilizer more than required actually harmed not only the crop production and potential yield, but also the ecosystem (Gastal & Lemaire, 2002). In contrast, the VRA approach in fertilizer management determines the amount of applied fertilizer depending on the level of crop growth variability. This ensures that the right amount of fertilizer is applied at the right time to obtain optimal yield and minimize its impact on the environment.
There are two basic methods of VRA: map-based and sensor based. Map-based VRA systems depend on an electronic prescription map to adjust the application rate of inputs. The map is generated by sampling soil or crop data across points in a field using a positioning system such as a GPS or a grid. One advantage of this system is that the user has a database that can assist in management-related decisions such as the acquired knowledge of the needed amount of chemicals or inputs prior to entering a field. Another advantage is that the user has more control of the applicate rate planning. On the other hand, some disadvantages to this system are the cost and the fairly complicated processing that are needed to generate a map. There is also the time delay between crop properties measurement and application of inputs. Examples of research work on this type of system can be seen in (Daud et al., 2014; Fraisse, Sudduth, & Kitchen, 2001; Han, Evans, Hodges, & Rawlins, 1995; Teoh, Hassan, Radzali, & Jafni, 2012; Weisz, Fleischer, & Smilowitz, 1995). Companies such as PrecisionHawk, URSULA Agriculture Ltd. and Agribotix provide commercial solutions using map-based VRA.
Sensor-based VRA systems, on the other hand, adjust the application rate of inputs by measuring soil or crop properties “on the go” using sensors mounted on the applicator. The continuous stream of information is sent to an on-board controller which governs the application rate based on an algorithm or model. The advantage of this system is that it does not necessarily require a geo-referenced location system or a map. Another advantage is that there is no time delay between measurement of soil or crop properties and application of inputs. The system is working “on the go” or real-time. The sensors produce a far denser dataset than traditional sampling methods. Moreover, the system is self-contained which reduces the risk of communication and interfacing error. Research work on this type of system can be seen in (Holland & Schepers, 2010; Schepers & Holland, 2011).
Regardless of which category of VRA is chosen, they both require the process of soil or crop property measurement in order to generate a strategy to apply the input. With regards to fertilizer management, suitable soil or crop properties that relate to crop variability need to be measured in order for the VRA system to adjust fertilizer input rate.
(Gastal & Lemaire, 2002) found that crop growth variability is directly related to the crop’s nitrogen (N). Different methods have been developed to measure the N content (Daud, Hamid, & Mohamad Ghazali, 2010; Gitelson, 2003; Lawless, Semenov, & Jamieson, 2005; Mistele & Schmidhalter, 2008; Sieling, Böttcher, & Kage, 2013). Specific to paddy, (Gholizadeh, Amin, Anuar, & Aimrun, 2009) concluded that Soil Plant Analysis Development (SPAD) reading was a viable method to predict the paddy canopy total N amount and future crop N requirements.
PRECISION FARMING IN MALAYSIA
The Malaysian Agricultural Research and Development Institute or MARDI has introduced a precision farming technology package for paddy (Chan, 2013). A model was developed to determine the N uptake based on the green area index (GAI) of the plant (Daud et al., 2010). The authors determined that the best parameters to describe the volume of green material of the crop were plant population, canopy and shoot size. The GAI model can be used to calculate the deviation of N content of the actual grown crop with respect to the N content of a reference crop.
(Daud et al., 2014) developed a map-based fertilizer VRA system for paddy production based on the GAI model and SPAD readings. Figure 1 shows the flow diagram of the fertilizer application map generation process.
A fertilizer application map was generated from the developed model which can then be utilized by a variable rate applicator on the field. The process of generating an application map involved two stages. In the first stage, ground truth data had to be collected by going into the field and sampling a number of small quadratic area (25cm x 25cm) in each paddy field to manually count the tiller population in the area, measure the shoot size and taking a SPAD reading with a hand held device. An unmanned aerial vehicle (UAV) with a camera mounted underneath was then used to obtain images of the paddy fields. In the second stage, the images were manually transformed into SPAD and population map using the ground truth data collected as a reference. These two maps were then processed to produce a treatment map. Figure 2 shows the image processing steps.
ACCEPTANCE OF PRECISION FARMING IN MALAYSIA
The developed precision farming technology package for paddy was introduced to three granary areas in Malaysia to gauge the acceptance and willingness to implement the new technology by local farmers. These granary areas were Muda granary area (MADA), Kemubu granary area (KADA), and the Integrated Agriculture Development Area (IADA) of Selangor. Figure 3 shows the locations of these granary areas in Malaysia. A series of seminars and workshops were conducted throughout 2017 and 2018 to expose the farmers to the concept of precision farming. A survey of the participants was conducted after the technology was demonstrated to them (Rahim, 2018 et. al.). A total of 400 respondents took part in the survey.
Figure 4 to figure 6 shows the result of the survey of the participants. The data showed that farmers from the three granary areas were generally keen to accept the technology.
Almost all participants agreed that the implementation of precision farming technologies would be more expensive to implement.
Just under 80% of farmers in the Kemubu granary area saw that the benefit of implementing precision farming outweighed the cost as compared 67% of farmers in the Muda granary area and 57% of farmers in the Selangor granary area. This may be due to the fact that the farmers in the Kemubu were willing to take more risks in return for higher income.
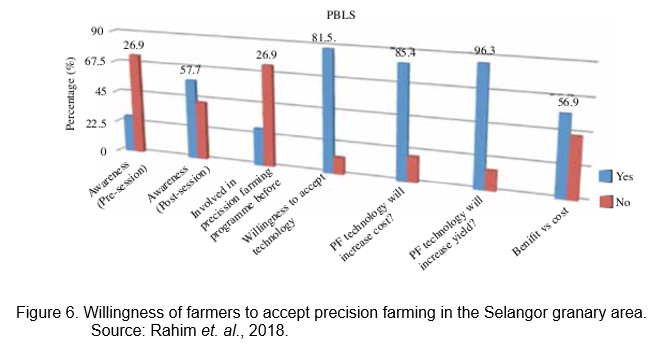
CONCLUSION
This work reported on the development of a variable rate application technique to reduce the amount of fertilizer use in large scale paddy production. It was argued that this precision farming technology could potentially be used to make the paddy production industry more efficient.
A survey was conducted to participants after exposing them to the new technology. It was found that the respondents were keen to implement the technology so long as the benefit outweighed the cost. The result from the survey could be used as a guide by the government in drafting policies that would promote the use of precision farming technologies in the future.
REFERENCES
Bakhtiari, A. A., and Hematian, A. (2013). Precision Farming Technology, Opportunities and Difficulty. International Journal for Science and Emerging Technologies with Latest Trends, 5(1), 1–14.
Chan, C. W. (2013). Precision Agriculture: The Way Forward in Mechanised Agriculture. Malaysian Agricultural Research and Development Institute (MARDI).
Daud, A. H., Abdul Rahman, S., Hamid, A., Teoh, C. C., Fakhrul Radzi, F. Z., and Rubiyah, Y. (2014). Variable Rate Fertilizer Application ( VRT ) for Rice Precision Farming Technology, In the proceedings of National conference on Agricultural food mechanization (NCAFM), May 2014, 20–22.
Daud, A. H., Hamid, A., and Mohamad Ghazali, M. S. S. (2010). Monitoring crop canopy development for nutrient management. In Proceeding of the national rice conference, 28-30 June (pp. pp. 450–453). Lumut, Perak.
Fraisse, C. W., Sudduth, K. A., and Kitchen, N. R. (2001). Delineation of site-specific management zones by unsupervised classification of topographic attributes and soil electrical conductivity. Transactions of the ASAE, 44(1), 155–166.
Gastal, F., and Lemaire, G. (2002). N uptake and distribution in crops: an agronomical and ecophysiological perspective. Journal of Experimental Botany, 53(370), 789–799. Gholizadeh, A., Amin, M. S.., Anuar, A. R., and Aimrun, W. (2009). Evaluation of SPAD Chlorophyll Meter in Two Different Rice Growth Stages and its Temporal Variability, European Journal of Scientific Research, 37(4), 591–598.
Gitelson, A. a. (2003). Remote estimation of leaf area index and green leaf biomass in maize canopies. Geophysical Research Letters, 30(5), 1248.
Han, S., Evans, R. G., Hodges, T., and Rawlins, S. L. (1995). Linking a geographic information system with a potato simulation model for site-specific crop management. Journal of Environmental Quality, 24(4), 772–777.
Rahim, H, Abdul Wahab, M.A.M., Mat Amin, M.Z., Harun, A., and Haimid, M.T. (2018). Perception and acceptance of farmers on precision farming technology in selected granary area. Economic and Technology Management Review, vol. 13, 97-110.
Holland, K. H., and Schepers, J. S. (2010). Derivation of a variable rate nitrogen application model for in-Season fertilization of corn. Agronomy Journal, 102, 1415–1424.
Lawless, C., Semenov, M. a., and Jamieson, P. D. (2005). A wheat canopy model linking leaf area and phenology. European Journal of Agronomy, 22(1), 19–32.
Mistele, B., and Schmidhalter, U. (2008). Estimating the nitrogen nutrition index using spectral canopy reflectance measurements. European Journal of Agronomy, 29(4), 184–190.
MOA. (2011). National Agrofood Policy 2011-2020. Percetakan Watan Sdn. Bhd.
MOA. (2017). Agrofood statistic 2017. Ministry of Agriculture and Agro-Based Industry Malaysia.
Sawyer, J. E. (1994). Concepts of variable rate technology with considerations for fertilizer application. Journal of Production Agriculture, 7(2), 195–201.
Schepers, J. S., and Holland, K. H. (2011). Active-crop sensor calibration using the virtual-reference concept. In J. V. Stafford (Ed.), Precision Agriculture ’11, p. 644, Wageningen: Wageningen Academic Publication.
Sieling, K., Böttcher, U., and Kage, H. (2013). Growth stage specific optima for the green area index of winter wheat. Field Crops Research, 148, 34–42.
Teoh, C. C., Hassan, D. A., Radzali, M. M., and Jafni, J. J. (2012). Prediction of SPAD chlorophyll meter readings using remote sensing technique, Journal of Tropical Agriculture and Food Science, 40(1), 127–136.
Weisz, R., Fleischer, S., and Smilowitz, Z. (1995). Site-specific integrated pest management for high value crops: sample units for map generation using the Colorado potato beetle (Coleoptera: Chrysomelidae) as a model system. Journal of Economic Entomology, 88(5), 1069–1080.