ABSTRACT
The Soil carbon 4 permille was launched to increase global soil organic matter stocks as a compensation for emission of greenhouse gases by anthropogenic sources. Specifically, soil organic carbon was treated as important due to soil productivity and soil health as well as carbon cycling. In this study, we conducted spatial prediction of soil organic carbon and its application in arable lands to find out the optimum soil organic matter management for sustainable productivity. Soil test database was used to Kriging model for organic carbon prediction and its application. Ordinary kriging model using soil test database was beneficial to predict overall soil organic carbon and N requirement in regional arable. This result indicates that soil test database would contribute to establish strategy of best soil management for soil health and climate changes control.
Keywords: Soil organic carbon prediction, Nitrogen requirement, Fit model, Soil test database
INTRODUCTION
Climate change will have a great impact on soils by change in atmospheric temperature and elevated CO2 concentrations, increased rainfall variability, and altered land-use. Soil organic carbon (SOC) and its potential to become a managed sink for atmospheric CO2 has been discussed widely [Jobbágy and Jackson, 2000; Smith et al., 2008]. Also, the SOC has been acknowledged on soil quality and soil function assoil structure, water holding capacity, pH buffering capacity and nutrient supply [Ash et al., 2010]. Like these, it might be necessary to manage soil carbon for sustainable crop cultivation under climate change.
The consequences of intensive crop cultivation and land degradation in Korea have led to an increase in the need for sustainable soil management. However, the financial and labor for the collection of soil data have been made for a long time. Using modeling, soil properties that are expensive to measure or unavailable can be predicted from other more easily measured properties using basic soil information from surveys or geographic information system (GIS) data layers to be translated into other more laborious and expensively determined soil properties (Minasry and Hartemink, 2011).
Previous work demonstrated the use of legacy soil data on a single soil property, e.g., mapping of soil carbon, soil pH, or available water capacity (Hong et al., 2013; Mulder et al., 2016). Understanding soil properties may provide a better understanding of the storage of carbon and nutrients supply and mobilization, and gas exchange between soil and atmosphere (Reynolds et al., 2013). These studies may show the effects of soil management and sustainable agricultural systems.
Soils on farmers’ fields are periodically sampled for soil fertility assessment and inorganic fertilizer recommendations. The advantages of such data are that the analytical methods from certified laboratories are usually standardized, and that the data is available in digital format (soil.rda.go.kr/soil/index.jsp). In this study, we predicted soil organic carbon and its application as N requirement for the regional scale using soil test database.
MATERIALS AND METHODS
Soil testing database. The Korean Soil Information System is an online system (http://soil.rda.go.kr) that is made up of soil maps and a soil fertility database. The fertilizer recommendation system was initiated based on the soil testing results. Soil samples (0–15 cm) are usually collected before irrigation for rice transplanting in spring (March–April), or after harvest in autumn (mid-October–November). The soil samples were analyzed in the laboratory and analytical results were uploaded in the national soil test database. The soil test database contains the geographical location, soil series, and soil pH and electrical conductivity (1:5 soil to water), soil organic matter (Tyurin method), available P2O5 (Lancaster method), exchangeable K, Ca, Mg (IN-ammonium acetate method), and available SiO2 (1N-sodium acetate method).
Data processing in ArcGIS. The study area comprises most of arable fields in Jeon Buk province (Fig. 1) and is in the southwestern part of Korea. It has an area of about 8,066 km2 and accounts for 8.06% of the total national area. Total arable land is occupied 194,000 ha (paddy: 1450,000 ha, upland: 70,000 ha). Soil test data was used 313,456 of point data in 2017 among total soil test database (Table 1). Before modeling, these data were converted from point address to coordinates using ArcGIS program.

Prediction of SOC using ordinary Kriging modeling. We used R cording for prediction of soil organic carbon (SOC) and its application in whole processing. Brief fit modeling process was followed; Arable lands were extracted using Digital farm map in Korea, and soil test data was jointed on its map in the extracted sites. All data investigated univariate and normality analysis for determination of data transformation. Ordinary kriging model for spatial soil prediction was used and validated the difference between simulated data and observed data such as R2, MES, RMSE values. Spatial organic carbon map was assessed uncertainty by Melone et al., 2011.
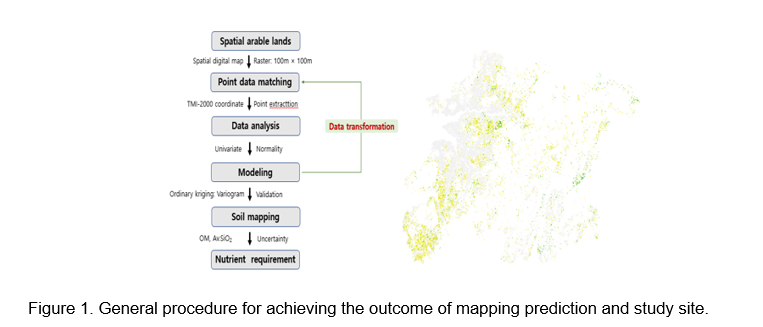
Estimation of nitrogen requirement. Estimation of optimum fertilizer recommendation is based on soil test data for stable crop cultivation. We hypothesized that spatial fit model using soil test database might be possible to estimate the whole fertilizer requirement in each region. Nitrogen fertilizer formula for fertilizer recommendation composed of SOC and available silicate content in rice cultivation. We predicted the amount of total nitrogen fertilizer using fit model. Optimum nitrogen fertilizer was calculated by the following formula:
Nitrogen requirement (kg N ha-1) = [11.17 – (0.133 × OM) + (0.025 × SiO2)]×10
RESULTS AND DISCUSSION
Soil organic carbon (SOC) prediction
Figure 2 shows the validation results for soil organic carbon. The Root mean squared deviation (RMSD) value was 29.9. The small RSMD means good fit model for prediction of soil organic carbon. Unfortunately, our results had shown high RMSE value. Some reasons for poorer performance of ordinary kriging may be that it mainly depends on the quality of the data, and soil test data was affected by the amount and types of nutrients and organic sources according to each farming system. The coefficients of determination (R2) were reasonable at 46% for SOC. Nevertheless, adding the model prediction to the interpolated validation residuals might be possible to improve fit model using addition of soil test data.
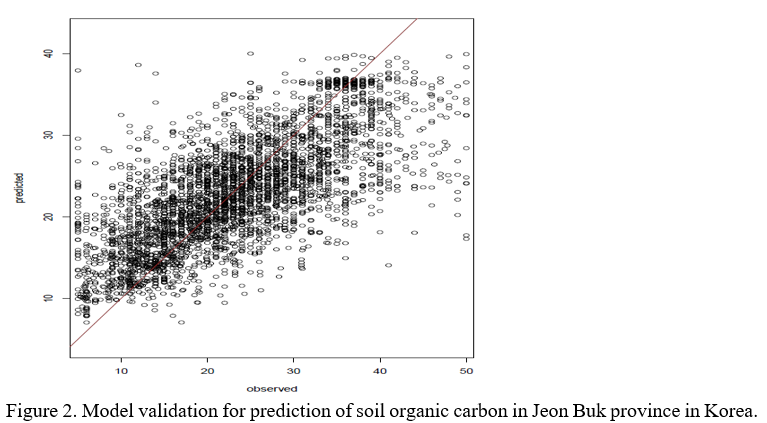
Figure 3 shows spatial prediction map of soil organic carbon using soil test data in Jeon Buk province. Soil organic carbon in arable land was possible to check the spatial difference between the selected point sites. Local ordinary Kriging may not always present the best result, but not worse. The prediction results of kriging techniques highly depend on the quality of available soil observations. It can provide more information to gain an insight in soil organic carbon in arable lands. Ordinary kriging might be useful when we have high density data in various parts of the area.
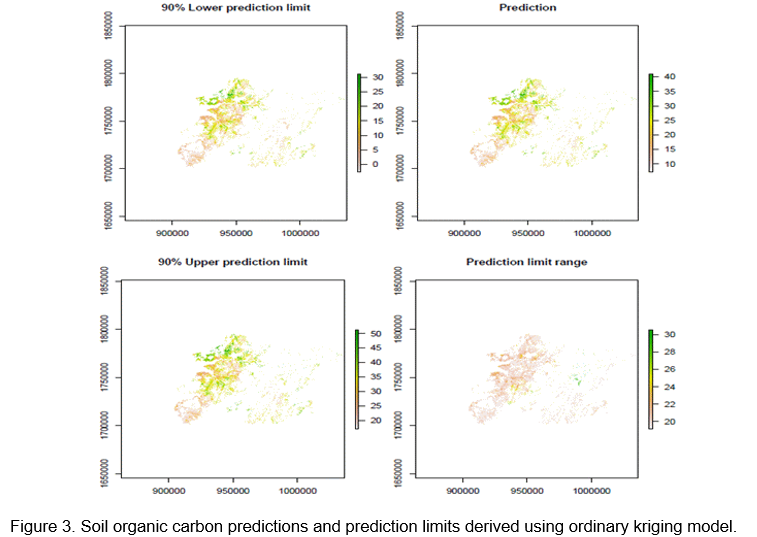
Nitrogen requirement for rice cultivation
Agricultural production and soil condition are linked through soil carbon levels, soil erosion, acidification, salinization, and soil nutrient imbalances. An important change is soil acidification due to agricultural activities, which has been reported to occur worldwide and it is mostly attributed to the use of N fertilizers (Barak et al., 1997; Guo et al., 2010; Marchant et al., 2015).
Rice is the main staple food, and farmers receive subsidies through fertilizer recommendation and supplies. We applied ordinary Kriging model to predict available SiO2 content for estimation of optimum N input in rice cultivation. Available SiO2 content was possible to predict using soil test data. We estimated the amount of N requirement for rice cultivation in Jeon Buk province (Figure 4), and it was estimated about 3,500 tons.
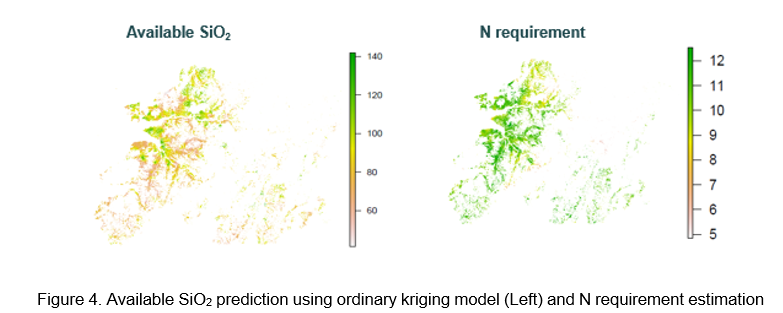
Soil test results can be used to estimate fertilizer requirement in key soil chemical properties at a regional scale. Using soil test database, quantification of the dynamics in soil condition proved possible including an analysis of some of the driving factors. Such information provides directions for adjusting the national soil fertility and fertilizer programs.
CONCLUSION
This study was conducted for spatial prediction of soil organic carbon and N requirement using point data in regional scale. Ordinary kriging model was beneficial to predict overall soil organic carbon in arable area. Also, it was possible to use the estimation of N requirement using fertilizer recommendation formula. Spatial prediction modeling would contribute to establishing the strategy of best soil organic carbon management for soil health and climate changes control. However, data quality management might be needed for future soil information services to the sustainable soil management.
REFERENCES
Ash, C., B. R. Jasny, D. A. Malakoff, and A. M. Sugden (2010), Feeding the future, Science, 327(5967): 797.
Aune, J. B., and R. Lal (1997), Agricultural productivity in the tropics and critical limits of properties of Oxisols, Ultisols, and Alfisols, J. Trop. Agric., 74(2), 96–103.
Hong, S.Y., Minasny, B., Han, K.H., Kim, Y., Lee, K., 2013. Predicting and mapping soil available water capacity in Korea. Peer J, e71.
Jobbágy, E. G., and R. B. Jackson (2000), The vertical distribution of soil organic carbon and its relation to climate and vegetation, Ecol. Appl., 10(2): 423–436.
Malone, B.P., McBratney, A.B., Minasny, B. 2011. Empirical estimates of uncertainty for mapping continuous depth functions of soil attributes. Geoderma, 160: 614–626.
Minasny, B., Hartemink, A.E. 2011. Predicting soil properties in the tropics. Earth-Science Reviews, 106: 52–62.
Minasny, B., Hong, S.Y., Hartemink, A.E., Kim, Y.H., Kang, S.S. 2016. Soil pH increase under paddy in South Korea between 2000 and 2012. Agric. Ecosyst. Environ., 221: 205–213.
Mulder, V.L., Lacoste, M., Richer-de-Forges, A.C., Martin, M.P., Arrouays, D., 2016. National versus global modelling the 3D distribution of soil organic carbon in mainland France. Geoderma, 263: 16–34.
Reynolds, B., Chamberlain, P., Poskitt, J., Woods, C., Scott, W., Rowe, E., Robinson, D., Frogbrook, Z., Keith, A., Henrys, P., 2013. Countryside survey: national soil change 1978–2007 for topsoils in Great Britain—acidity, carbon, and total nitrogen status. Vadose Zone J., 12: 1–15.
Smith, P., C. Fang, J. J. C. Dawson, and J. B. Moncrieff (2008), Impact of global warming on soil organic carbon, Adv. Agron., 97: 1–43.
Case Study on the Prediction of Soil Organic Carbon and Fertilizer Requirement Using Legacy Data in Korea
ABSTRACT
The Soil carbon 4 permille was launched to increase global soil organic matter stocks as a compensation for emission of greenhouse gases by anthropogenic sources. Specifically, soil organic carbon was treated as important due to soil productivity and soil health as well as carbon cycling. In this study, we conducted spatial prediction of soil organic carbon and its application in arable lands to find out the optimum soil organic matter management for sustainable productivity. Soil test database was used to Kriging model for organic carbon prediction and its application. Ordinary kriging model using soil test database was beneficial to predict overall soil organic carbon and N requirement in regional arable. This result indicates that soil test database would contribute to establish strategy of best soil management for soil health and climate changes control.
Keywords: Soil organic carbon prediction, Nitrogen requirement, Fit model, Soil test database
INTRODUCTION
Climate change will have a great impact on soils by change in atmospheric temperature and elevated CO2 concentrations, increased rainfall variability, and altered land-use. Soil organic carbon (SOC) and its potential to become a managed sink for atmospheric CO2 has been discussed widely [Jobbágy and Jackson, 2000; Smith et al., 2008]. Also, the SOC has been acknowledged on soil quality and soil function assoil structure, water holding capacity, pH buffering capacity and nutrient supply [Ash et al., 2010]. Like these, it might be necessary to manage soil carbon for sustainable crop cultivation under climate change.
The consequences of intensive crop cultivation and land degradation in Korea have led to an increase in the need for sustainable soil management. However, the financial and labor for the collection of soil data have been made for a long time. Using modeling, soil properties that are expensive to measure or unavailable can be predicted from other more easily measured properties using basic soil information from surveys or geographic information system (GIS) data layers to be translated into other more laborious and expensively determined soil properties (Minasry and Hartemink, 2011).
Previous work demonstrated the use of legacy soil data on a single soil property, e.g., mapping of soil carbon, soil pH, or available water capacity (Hong et al., 2013; Mulder et al., 2016). Understanding soil properties may provide a better understanding of the storage of carbon and nutrients supply and mobilization, and gas exchange between soil and atmosphere (Reynolds et al., 2013). These studies may show the effects of soil management and sustainable agricultural systems.
Soils on farmers’ fields are periodically sampled for soil fertility assessment and inorganic fertilizer recommendations. The advantages of such data are that the analytical methods from certified laboratories are usually standardized, and that the data is available in digital format (soil.rda.go.kr/soil/index.jsp). In this study, we predicted soil organic carbon and its application as N requirement for the regional scale using soil test database.
MATERIALS AND METHODS
Soil testing database. The Korean Soil Information System is an online system (http://soil.rda.go.kr) that is made up of soil maps and a soil fertility database. The fertilizer recommendation system was initiated based on the soil testing results. Soil samples (0–15 cm) are usually collected before irrigation for rice transplanting in spring (March–April), or after harvest in autumn (mid-October–November). The soil samples were analyzed in the laboratory and analytical results were uploaded in the national soil test database. The soil test database contains the geographical location, soil series, and soil pH and electrical conductivity (1:5 soil to water), soil organic matter (Tyurin method), available P2O5 (Lancaster method), exchangeable K, Ca, Mg (IN-ammonium acetate method), and available SiO2 (1N-sodium acetate method).
Data processing in ArcGIS. The study area comprises most of arable fields in Jeon Buk province (Fig. 1) and is in the southwestern part of Korea. It has an area of about 8,066 km2 and accounts for 8.06% of the total national area. Total arable land is occupied 194,000 ha (paddy: 1450,000 ha, upland: 70,000 ha). Soil test data was used 313,456 of point data in 2017 among total soil test database (Table 1). Before modeling, these data were converted from point address to coordinates using ArcGIS program.
Prediction of SOC using ordinary Kriging modeling. We used R cording for prediction of soil organic carbon (SOC) and its application in whole processing. Brief fit modeling process was followed; Arable lands were extracted using Digital farm map in Korea, and soil test data was jointed on its map in the extracted sites. All data investigated univariate and normality analysis for determination of data transformation. Ordinary kriging model for spatial soil prediction was used and validated the difference between simulated data and observed data such as R2, MES, RMSE values. Spatial organic carbon map was assessed uncertainty by Melone et al., 2011.
Estimation of nitrogen requirement. Estimation of optimum fertilizer recommendation is based on soil test data for stable crop cultivation. We hypothesized that spatial fit model using soil test database might be possible to estimate the whole fertilizer requirement in each region. Nitrogen fertilizer formula for fertilizer recommendation composed of SOC and available silicate content in rice cultivation. We predicted the amount of total nitrogen fertilizer using fit model. Optimum nitrogen fertilizer was calculated by the following formula:
Nitrogen requirement (kg N ha-1) = [11.17 – (0.133 × OM) + (0.025 × SiO2)]×10
RESULTS AND DISCUSSION
Soil organic carbon (SOC) prediction
Figure 2 shows the validation results for soil organic carbon. The Root mean squared deviation (RMSD) value was 29.9. The small RSMD means good fit model for prediction of soil organic carbon. Unfortunately, our results had shown high RMSE value. Some reasons for poorer performance of ordinary kriging may be that it mainly depends on the quality of the data, and soil test data was affected by the amount and types of nutrients and organic sources according to each farming system. The coefficients of determination (R2) were reasonable at 46% for SOC. Nevertheless, adding the model prediction to the interpolated validation residuals might be possible to improve fit model using addition of soil test data.
Figure 3 shows spatial prediction map of soil organic carbon using soil test data in Jeon Buk province. Soil organic carbon in arable land was possible to check the spatial difference between the selected point sites. Local ordinary Kriging may not always present the best result, but not worse. The prediction results of kriging techniques highly depend on the quality of available soil observations. It can provide more information to gain an insight in soil organic carbon in arable lands. Ordinary kriging might be useful when we have high density data in various parts of the area.
Nitrogen requirement for rice cultivation
Agricultural production and soil condition are linked through soil carbon levels, soil erosion, acidification, salinization, and soil nutrient imbalances. An important change is soil acidification due to agricultural activities, which has been reported to occur worldwide and it is mostly attributed to the use of N fertilizers (Barak et al., 1997; Guo et al., 2010; Marchant et al., 2015).
Rice is the main staple food, and farmers receive subsidies through fertilizer recommendation and supplies. We applied ordinary Kriging model to predict available SiO2 content for estimation of optimum N input in rice cultivation. Available SiO2 content was possible to predict using soil test data. We estimated the amount of N requirement for rice cultivation in Jeon Buk province (Figure 4), and it was estimated about 3,500 tons.
Soil test results can be used to estimate fertilizer requirement in key soil chemical properties at a regional scale. Using soil test database, quantification of the dynamics in soil condition proved possible including an analysis of some of the driving factors. Such information provides directions for adjusting the national soil fertility and fertilizer programs.
CONCLUSION
This study was conducted for spatial prediction of soil organic carbon and N requirement using point data in regional scale. Ordinary kriging model was beneficial to predict overall soil organic carbon in arable area. Also, it was possible to use the estimation of N requirement using fertilizer recommendation formula. Spatial prediction modeling would contribute to establishing the strategy of best soil organic carbon management for soil health and climate changes control. However, data quality management might be needed for future soil information services to the sustainable soil management.
REFERENCES
Ash, C., B. R. Jasny, D. A. Malakoff, and A. M. Sugden (2010), Feeding the future, Science, 327(5967): 797.
Aune, J. B., and R. Lal (1997), Agricultural productivity in the tropics and critical limits of properties of Oxisols, Ultisols, and Alfisols, J. Trop. Agric., 74(2), 96–103.
Hong, S.Y., Minasny, B., Han, K.H., Kim, Y., Lee, K., 2013. Predicting and mapping soil available water capacity in Korea. Peer J, e71.
Jobbágy, E. G., and R. B. Jackson (2000), The vertical distribution of soil organic carbon and its relation to climate and vegetation, Ecol. Appl., 10(2): 423–436.
Malone, B.P., McBratney, A.B., Minasny, B. 2011. Empirical estimates of uncertainty for mapping continuous depth functions of soil attributes. Geoderma, 160: 614–626.
Minasny, B., Hartemink, A.E. 2011. Predicting soil properties in the tropics. Earth-Science Reviews, 106: 52–62.
Minasny, B., Hong, S.Y., Hartemink, A.E., Kim, Y.H., Kang, S.S. 2016. Soil pH increase under paddy in South Korea between 2000 and 2012. Agric. Ecosyst. Environ., 221: 205–213.
Mulder, V.L., Lacoste, M., Richer-de-Forges, A.C., Martin, M.P., Arrouays, D., 2016. National versus global modelling the 3D distribution of soil organic carbon in mainland France. Geoderma, 263: 16–34.
Reynolds, B., Chamberlain, P., Poskitt, J., Woods, C., Scott, W., Rowe, E., Robinson, D., Frogbrook, Z., Keith, A., Henrys, P., 2013. Countryside survey: national soil change 1978–2007 for topsoils in Great Britain—acidity, carbon, and total nitrogen status. Vadose Zone J., 12: 1–15.
Smith, P., C. Fang, J. J. C. Dawson, and J. B. Moncrieff (2008), Impact of global warming on soil organic carbon, Adv. Agron., 97: 1–43.