ABSTRACT
Agricultural production towards an oriented market is generally exposed to a variety of risks that may lead to income fluctuations. Correct identification of the risk variety will be a very important base for making good management decisions to minimize their negative effects. Results indicated 14 risk sources that may affect production of farmers in the study area. Of risk sources, seeding quality, climate variability, and disease/pest issue are most serious as single risks by fruit farmer's perception. Moreover, the result showed a number of socio-economic factors that have significant impacts on risk perception of farmers. Notably, accessing agricultural extension service and credit sources are found to reduce worries and risk perception. In addition, the findings also suggest possible policy intervention to minimize negative influence of risk sources on farmer's agricultural production.
Keywords: Linear multiple regression, agriculture, risk source, Principal Component Analysis.
BACKGROUND
Agriculture plays an important role in Viet Nam, contributing to 15% of the Gross Domestic Product (GDP). The agricultural sector is the main source of livelihood for about 70% of the rural workforce. The export turnover of agricultural products in recent years has continuously increased in terms of output and value - especially the export of vegetables and fruits. In 2003, the export value of vegetables and fruits reached US$151.5 million, and increased to US$2.5 billion in 2016. The average growth rate during this period is 1.25 times/year. In 2018, the export value of vegetables and fruits reached US$3.8 billion – an increase of over 47.3% compared to the export value of 2017, in which, the value of fruit products accounted for over 80% of the total export value of vegetables and fruits in the country. Vietnam has the advantage of producing many kinds of fruits with high economic value, including mango, longan and citrus (orange, pomelo). Mango is grown in many provinces in the country - 59/63 provinces and cities including Son La with 15,700 ha are being harvested. The province's mango production is estimated at about 35,500 tons in 2019. Son La is also the main mango production area of the North, accounting for 58% of the total output supplied to the market (Son La Statistical Office, 2019). Meanwhile, longan is a crop with a large area grown in many districts in the province, accounting for 11.3% of the total fruit area of the province. The total area of longan in Son La is twice as large as that of Hung Yen province. With the citrus group, the area and output have also continuously increased in recent years, with a high average annual growth rate. In the 10 years from 2009 to 2019, the area of citrus trees in the northern mountainous provinces grew by an average of 10%/year - equivalent to about 7,300 ha/year. Citrus production also grows at an average of 12.5% per year – equivalent to 69,400 tons/year (MARD, 2020).
Agricultural production in general faces many risks and uncertainties because production activities are closely tied to natural and environmental conditions (Akcaoz & Ozkan, 2005). Risk sources are not uniform across households and are not constant over time (Riwthong et al., 2017). There are many possible causes of risks in agricultural production such as variations in climate and weather, the complex nature of biological processes associated with crops and livestock, seasonality in agriculture, variety of geographical conditions, fluctuations in output and input prices in the production process (Jain & Parshad, 2007). Fruit production in Vietnam is also typical of agricultural production in general and can be affected by the risk factors mentioned above. Households often avoid or tend to reduce investment in production if they perceive risks that are likely to cause the failure of planned investments (Alderman, 2008). Because risks negatively and directly affect production output, and indirectly affect household income, identifying and managing risk sources is of great importance to households (Drollette, 2009).
Risk is one of the important research topics to support effective management decisions in production, so the study of risk has received much attention from scholars and managers. A full understanding of farmers' risk perceptions and their management responses is important in designing appropriate and effective risk management systems/policies (Flaten et al., 2005). The literature review indicates that risks in agricultural production can be divided into production risk, price risk, disaster risk and technology risk (Miller et al., 2004). According to the research results of the European Commission, risks are classified into human health risks, institutional risks, financial risks, production risks, and input and output price risks. Besides, risk sources can also be divided into 5 main groups: production risk; market risk; financial risk; environmental and legal risk; and human resource risk (USDA, 1997). Although understanding about risk perception and factors affecting risk perception of farmers is important in managing and limiting losses in agricultural production, information about risk sources and the factors affecting farmers' awareness are quite limited in Vietnam. Therefore, this study was conducted to identify risk sources and factors affecting risk perception of commodity fruit farmers in the study area.
RESEARCH METHODOLOGY
Research and data collection
Son La is one of the central provinces of the Northwest Mountain region of Vietnam and is a province with strengths in the production of agricultural products and commodities with a total area of about over 147,272 hectares. The group of fruit trees with the largest production area includes longan, mango and citrus groups. Therefore, this study selects Son La province to conduct the research and collect data from July to October 2020.
Secondary and primary data are used in this study. Secondary data is the basic information collected from statistical reports in the area and is used to select the study area. Primary data is the key quantitative information used in the analysis of the results in this study. Key information was collected through interviews with farmers in the study area using a pre-designed questionnaire. Possible risks are selected based on the actual situation of agricultural commodity production in the locality and tested in practice through pilot interviews with a number of households before conducting formal data collection. The risks are divided into increasing levels from 1 to 5 based on the Likert scale, and farmers are asked to rank the risks within this range. Level 1 represents the lowest level of influence and level 5 implies the highest level of influence/importance. A total of 172 households were interviewed from the two districts of Mai Son and Van Ho - which represent the major fruit growing areas of the province.
Analytical methods and statistical processing
The risk perception of farmers in commodity fruit production was analyzed using descriptive statistical methods. Because in this study, the risk sources are quite large, the statistical technique - basic component analysis (PCA-Principal Component Analysis) is used to reduce the magnitude and dispersion of the variables. According to Abdi & William (2010), PCA is one of the statistical techniques that allows efficient analysis of data sets in which the quantitatively dependent variables are correlated with each other. The purpose of this technique is to extract the important information represented from the data set as a new set of variables called the PCA. PCA is an effective tool to reduce dispersion without losing important information. Therefore, this method is widely applied in many risk studies (Flaten et al., 2005; Meuwissen et al., 2001). Therefore, PCA was also selected for data analysis in this study. The relationship between farmers' perception of risk sources and socio-economic factors is explored by applying multiple linear regression models, and is described in the form of the formula as follows:
Yi = â0 + â1X1 + â2X2 + â3X3 + âijXi + åi
In which, Yi is the score of the household on risk perception achieved after PCA estimation (i = 1, 2, ... n); Xi are the explanatory variables of the analytical model (j = 1, 2, ..., n) and are described as in the table; âij is the coefficient estimated in the regression model; åi is the random error of the model.
Description of variables used in the regression model
The dependent variable is the household's perception of risk source - four basic components extracted from PCA analysis. The explanatory or independent variables of the model are selected on the basis of economic theory, the actual situation of agricultural production in the area and refer to the results of a number of related studies. Many studies have shown that household risk perception is influenced by household and farm characteristics such as age of household head, ethnicity, level of general education, occupation, labor force in agriculture, crop area, access to extension services and household credit (Flaten et al., 2005; Riwthong et al., 2017). Statistics for the variables are presented in Table 1. The panel data shows that the fruit-growing area of households varies widely from 0.02 to 6 ha, and the average area is quite small, about 0.83 ha /household. The average age of the household head is 44 years old, and 70% of the fruit farmers in the area are Kinh people. The main livelihood of farmers is based on agricultural production – accounting for 80% of surveyed households. The head of household mainly has a lower secondary education level. In the surveyed area, households producing fruit products according to VietGAP standards account for about 60% of the total surveyed households.
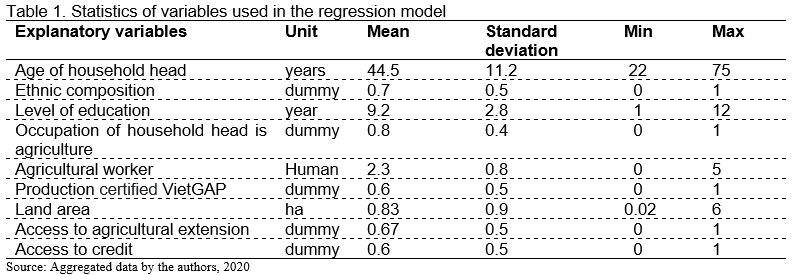
RESULTS AND DISCUSSION
Perception of risk sources of households
Perception of sources of risk in agricultural production of households are presented in Table 2. Accordingly, in the study area Farmers are aware that there are 14 sources of risk affecting their agricultural production. The perceived risk level of the household is ranked in column 2 (from left) based on the average risk source index. The risk index according to farmers' perception fluctuates from 2.55 to 4.18. A higher risk index implies a greater degree of influence on household output. In general, the agricultural output of the household is closely related and greatly influenced by the quality of seedlings, weather conditions and pests and diseases. This is the group of 3 risk sources with the highest ranking according to household perception. The quality of seedlings clearly has a great influence on the quality and yield of crops in general. Good seedlings (right species, and good health) will be a favorable premise for future care and good quality. On the contrary, bad seedlings will give low yield, even no yield. Healthy seedlings will grow faster, helping farmers reduce time and cost of fertilization. According to the survey, households all depend on the sources of seedlings purchased from different places, so it is easy for people to worry about the quality of seedlings. Agricultural production in general is greatly affected by weather conditions. And the change of weather conditions will directly affect the quality and output of agricultural products. This feature is also correlated with the development of pests and diseases. Therefore, pests and diseases are also one of the three sources of risk according to households that have a great influence on production results. The sources of risk that are assessed to have the least impact are respectively the loan interest rate and the State's regulations on product quality standards.
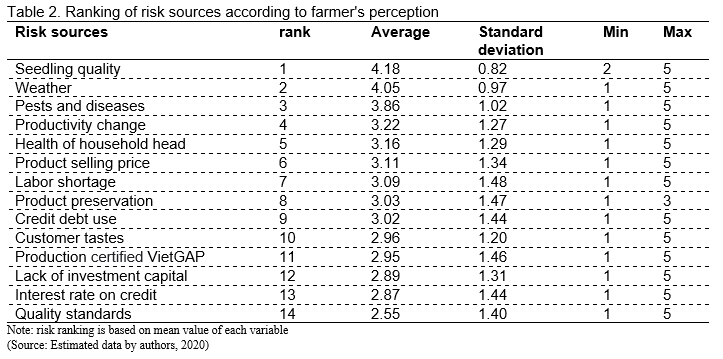
Before applying PCA, The Kaiser-Meyer-Olkin index (KMO) is estimated to assess the reliability of the sample data (sampling adequacy). In other words, the KMO indicator shows whether the data being used is suitable for the use of the PCA technique. According to Chow (2004), the KMO index fluctuates from 0 to 1 and the overall KMO index must be at least 0.5 or greater for the sample data to be considered appropriate. In this study, the KMO value was estimated to be 0.71, implying that the sample data are suitable for the application of the Principal Component Analysis technique. Table 3 presents the factor loading according to the perpendicular rotation of the risk sources. Through PCA, the number of risk sources is reduced from 14 variables to 4 components corresponding to an eigenvalue greater than 1. These four risk components have an explanatory value for about 63.6% total variance in this study. Based on the factor loading in Table 3 and the relationship between risk sources, components 1 – 4 are named “Finance,” “Human Resources,” “Production,” and “Market.” In component 1, the load factor of the capital risk variable has the largest value. This comes from the fact that the households need large investment capital, especially in the early stages of basic construction. In this first stage, farmers need a lot of investment capital (land preparation, seeds, fertilizers, etc.) for their orchards and this period lasts about 5 years without any revenue to cover costs. In addition, for farms with a harvest, the required annual investment capital is also very important. It is the addition of fertilizers, labor, and pest control for plants. Many households lack investment capital in the first stage of investment, so they need to borrow capital from credit institutions in the area. Therefore, interest rates and loan repayment are risks affecting farmers' perception.
The health of household heads and agricultural workers has a large coefficient of variation in the 2-Human components. Human resources play an important role in production in general and production in particular - the industry that requires the largest labor in Vietnam's economy today. Human resource risks reflect the lack of key workers – the head of the household and other workers involved in the production process. The head of the household is not only the main worker but also the one who makes decisions that can greatly affect production. Meanwhile, other agricultural labor affects the care and especially the stage of harvesting products to bring to the market. The lack of harvesting labor or the high cost of hired labor both directly and indirectly affect the production results (income) of farmers. Since fruit ripens and yields seasonally, lack of harvesting labor will lead to over ripens, fruit drop, and yield loss. While the high labor cost will increase production costs and directly reduce the income of farmers. In fact, the survey shows that the number of household workers involved in production is quite small - on average 2.3 people/household, and not enough to meet the requirements in the production process. Therefore, outsourcing labor is a mandatory requirement for households.
Seedling quality, pest and disease control, weather changes, postharvest preservation of agricultural products and changes in crop yield are grouped into component 3-Production. As analyzed above, good seedling quality greatly affects yield and product quality. In contrast, bad plant varieties will generate costs and production time for farmers. Therefore, the load factor in this component has the second largest value after the load factor of weather fluctuations. Fruit production takes place closely with external factors such as weather. Weather is an important factor and is beyond the control of farmers - farmers can only minimize the harm (if any) but cannot prevent it. On the other hand, changes in weather are closely related to pests and diseases. Favorable weather will positively affect productivity and product quality. The household's agricultural products are fresh fruits. Keeping fruit fresh and undamaged when reaching consumers directly affects the selling price of the product and the income of farmers. In addition, the farmer also assessed that the change in productivity also affects the production results of the household. In fact, fluctuations in crop yield are closely related to pests and diseases, seedling quality and unfavorable weather conditions.
About the component 4 “Market,” farmers' perception of risks related to the market, including product price fluctuations, customer tastes, regulations on product quality and production according to VietGAP standards. In this component, the largest load factor is the customer's taste, the second is production according to VietGAP standards, and the next is the product selling price and government regulations, respectively. Changes in market prices are beyond the control of farmers but directly affect household incomes. Consumer tastes are also related to price fluctuations. Customers prefer imported agricultural products - fruits will affect the price of domestic fruits. While consumer confidence in the quality of domestic products or VietGAP standard products will affect the selling price and income of farmers, state regulations on quality also affect the quality of products and their market in general. Adequate and reasonable regulations and strict enforcement of these regulations can increase consumer confidence in the quality of domestic agricultural products – indirectly affecting the selling price and income of households.
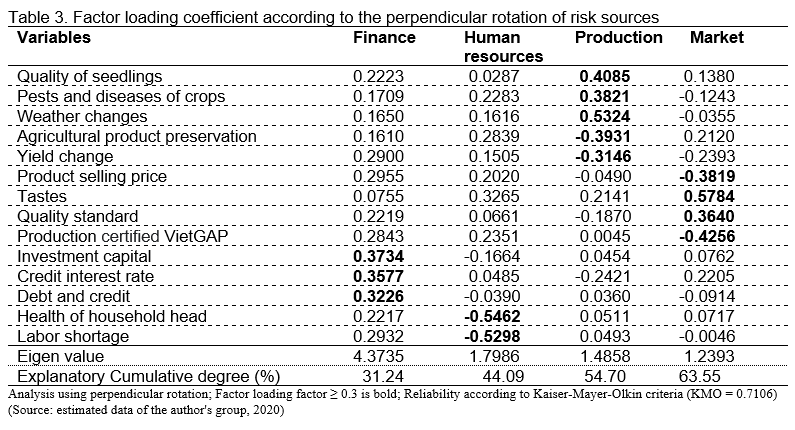
Factors influencing farmers' risk perception
Linear regression model Multivariate analysis was applied to determine the relationship between farmer's risk perception and influencing factors. The estimated coefficient (âij) and the fit of the regression model are presented in Table 4. The results show that all 4 models have high significance in terms of relevance in this study. According to Gujarati (2011), the low coefficient R2 value in the models is normal and acceptable because of the 1-year data type (cross-sectional data) with many observations with high dispersion. Synthesis of research literature also shows that many previous similar studies also have a rather low value of R2 coefficient (Meuwissen et al., 2001; Flaten et al., 2005).
Table 4 shows that there are 9 explanatory variables in the regression model. The estimated coefficients with different signs (+/-) reflect the relationship in different directions between the dependent variable and the explanatory variable. The results show that there is no explanatory variable that has a significant effect on household risk perception in all 4 regression models. In other words, farmers' perception of risk sources is significantly influenced by different factors. Occupation of household head and access to credit are two factors that have a statistically significant influence on perceived financial risk. Households with the main source of livelihood from agriculture perceive greater financial risks than other households. Because agricultural production is the main source of household income, the annual capital requirement for production investment is mandatory. For these households, the lack of capital or financial constraints means difficulties in production investment such as tilling, fertilizing, harvesting, and renovating orchards. No investment or limited investment such as insufficient fertilizer application and wrong time will directly affect the productivity and quality of output agricultural products of households. For households lacking production capital, finding sources of credit to borrow is necessary. Therefore, households receiving credit are perceived to have less financial risk to invest in production than households without loans.
Human resources are an important factor in agricultural production of households. There are two factors that significantly affect the risk perception on human resources of farmers, including the occupation of the household head and production methods according to VietGAP standards. The main occupation of the household head is agriculture, which means that the household's income is mainly based on agricultural production. While agricultural production is labor-intensive and concentrated with high seasonality, the lack of labor, especially in the care and harvesting stages, will directly affect crop yields and quality. Statistics show that with the number of agricultural workers/households being small and not enough for care and harvesting, every year households have to hire labor or exchange labor with other households in the area to overcome the situation of seasonal labor shortage. Households producing agricultural products according to VietGAP standards also have a similar perception of human risk. In addition to labor requirements in the production and harvesting of agricultural products in general, farmers producing agricultural products according to VietGAP standards need more labor than those producing non-standard products because production according to VietGAP standards requires more labor, stricter requirements on process and quality control. This also means that households producing according to VietGAP must use more labor per unit area than households not producing according to this standard. Therefore, it is easy to understand that households producing according to this standard consider human resources as one of the important factors for them.
Access to agricultural extension services and credit sources both have a significant influence on risk perception in production and markets. With production risks, the participation in technical training, access to information from agricultural extension services and loans for production helps farmers reduce worries in production such as lack of technology, lack of investment capital. As described in Table 3, seedling quality, pest control and storage are the primary risks in production. Providing a reliable seedling sales address, training on production techniques to prevent pests and diseases, and linking to support farmers to apply production standards according to VietGAP are the basic functions of agricultural extension work in the area. Besides, the application of VietGAP standards to agricultural products also helps households reduce market risks. Currently, the market's requirements for the quality of agricultural products are increasing, qualified agricultural products such as VietGAP contribute to stabilizing selling prices and increasing incomes for farmers. Besides, production certified VietGAP also leads to increased production costs (labor, certification costs, renewal...). Therefore, loans from credit funds also contribute to reducing worries for farmers in this case.
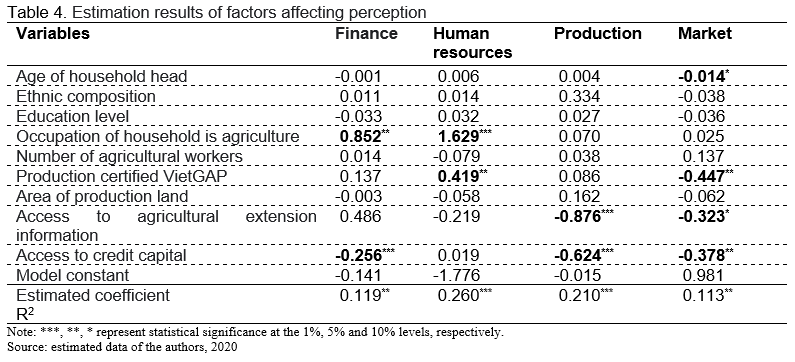
CONCLUSION
The study was carried out in Son La province - a locality with strengths in commodity fruit production in the northwest region of Vietnam. Descriptive statistical analysis was used to rank the sources of risk. PCA technique is implemented to reduce data dispersion, and retain the most important information. Next, a multivariate regression model was applied to determine the factors affecting the perception of risk sources of households. In this study, 14 risk sources were identified by fruit farmers in the area and ranked in order. Among these, the group of risk sources that can have the greatest impact on household production according to farmers' perceptions includes seedling quality, weather variability, and crop pests and diseases. After the PCA analysis, the risk sources are divided into 4 groups of basic components such as Finance, Human Resources, Production, and Market. The research results also show the important factors that explain the perception of farmers for each risk component. Notably, access to information from agricultural extension services and access to credit contributes to reducing concerns for farmers in production. Besides, producing products with good quality such as meeting VietGAP standards will contribute to reducing market risks for farmers. Therefore, policies to support agricultural production should aim to encourage farmers to produce agricultural products according to VietGAP standards. Additionally, maintaining and improving the efficiency of agricultural extension activities should be focused. In addition, increasing the level of access to credit to support agricultural production also contributes to helping farmers who lack capital to reduce risks in production and markets.
REFERENCES
Abdi, H. and Williams, LJ (2010), 'Principal component analysis', WIREs Computational Statistics, 2, 433–459. doi:10.1002/wics.101.
Akcaoz, H., & Ozkan, B. (2005), 'Determining risk sources and strategies among farmers of contrasting risk awareness: A case study for Cukurova region of Turkey', Journal of Arid Environment, 62, 661-675.
Alderman, H. (2008), 'Managing Risks to Increase Efficiency and Reduce Poverty', World Development Report 2008, World Bank, Washington, DC.
MARD, (2020), Report on Area of fruits tree in different regions of Viet Nam, http://www.cuctrongtrot.gov.vn/TinTuc/Index/4377 (accessed 22/3/2021).
Sơn La Statistical Office, (2019), Report on Area and Production of fruits tree in Sơn La province, https://sonla.gov.vn/4/469/63804/545755/thong-tin-ve-nong-san/nam-2019-tong-dien-tich-nong-san-cay-an-qua-chinh-cua-tinh-dat-147-272-ha (accessed 19/3/2021).
Drollette, SA (2009), Managing Production Risk in Agriculture, Department of Applied Economics, Utah State University, United States.
Flaten, O., Lien, G., Koesling, M., Valle, PS & Ebbesvik, M. (2005), 'Comparing risk perceptions and risk management in organic and conventional dairy farming: empirical results from Norway', Livestock Production Science, 95 (1–2): 11–25.
Gujarati, D. (2011), 'Econometrics by example', Palgrve Macmillan, Hamphire, UK, 25-81.
Jain, RCA, Parshad, M. (2007), 'Working Group on Risk Management in Agriculture for XI Five Year Plan', Government of India, Planning Commission, New Delhi.
Meuwissen, MPM, Huirne, RBM & Hardaker, JB (2001), 'Risk and risk management: An empirical analysis of Dutch livestock farmers', Livestock Production Science, 69, 43-53.
Miller, A., Dobbins, C., Pritchett, J., Boehlje, M. & Ehmke, C. (2004), Risk Management for Farmers, Staff Paper 04-11, Department of Agricultural Economics, Purdue University, 1-27.
Riwthong, S., Schreinemachers, P., Grovermann, C. & Berger, T. (2016), 'Agricultural commercialization: Risk perceptions, risk management and the role of pesticides in Thailand', Kasetsart Journal of Social Sciences (2016).
USDA. (1997), Introduction to Risk Management, United States Department of Agriculture, Risk Management Agency.
Factors Affecting Risk Perception of Farmers in Agricultural Production in Son La Province, Northwest Mountain of Vietnam
ABSTRACT
Agricultural production towards an oriented market is generally exposed to a variety of risks that may lead to income fluctuations. Correct identification of the risk variety will be a very important base for making good management decisions to minimize their negative effects. Results indicated 14 risk sources that may affect production of farmers in the study area. Of risk sources, seeding quality, climate variability, and disease/pest issue are most serious as single risks by fruit farmer's perception. Moreover, the result showed a number of socio-economic factors that have significant impacts on risk perception of farmers. Notably, accessing agricultural extension service and credit sources are found to reduce worries and risk perception. In addition, the findings also suggest possible policy intervention to minimize negative influence of risk sources on farmer's agricultural production.
Keywords: Linear multiple regression, agriculture, risk source, Principal Component Analysis.
BACKGROUND
Agriculture plays an important role in Viet Nam, contributing to 15% of the Gross Domestic Product (GDP). The agricultural sector is the main source of livelihood for about 70% of the rural workforce. The export turnover of agricultural products in recent years has continuously increased in terms of output and value - especially the export of vegetables and fruits. In 2003, the export value of vegetables and fruits reached US$151.5 million, and increased to US$2.5 billion in 2016. The average growth rate during this period is 1.25 times/year. In 2018, the export value of vegetables and fruits reached US$3.8 billion – an increase of over 47.3% compared to the export value of 2017, in which, the value of fruit products accounted for over 80% of the total export value of vegetables and fruits in the country. Vietnam has the advantage of producing many kinds of fruits with high economic value, including mango, longan and citrus (orange, pomelo). Mango is grown in many provinces in the country - 59/63 provinces and cities including Son La with 15,700 ha are being harvested. The province's mango production is estimated at about 35,500 tons in 2019. Son La is also the main mango production area of the North, accounting for 58% of the total output supplied to the market (Son La Statistical Office, 2019). Meanwhile, longan is a crop with a large area grown in many districts in the province, accounting for 11.3% of the total fruit area of the province. The total area of longan in Son La is twice as large as that of Hung Yen province. With the citrus group, the area and output have also continuously increased in recent years, with a high average annual growth rate. In the 10 years from 2009 to 2019, the area of citrus trees in the northern mountainous provinces grew by an average of 10%/year - equivalent to about 7,300 ha/year. Citrus production also grows at an average of 12.5% per year – equivalent to 69,400 tons/year (MARD, 2020).
Agricultural production in general faces many risks and uncertainties because production activities are closely tied to natural and environmental conditions (Akcaoz & Ozkan, 2005). Risk sources are not uniform across households and are not constant over time (Riwthong et al., 2017). There are many possible causes of risks in agricultural production such as variations in climate and weather, the complex nature of biological processes associated with crops and livestock, seasonality in agriculture, variety of geographical conditions, fluctuations in output and input prices in the production process (Jain & Parshad, 2007). Fruit production in Vietnam is also typical of agricultural production in general and can be affected by the risk factors mentioned above. Households often avoid or tend to reduce investment in production if they perceive risks that are likely to cause the failure of planned investments (Alderman, 2008). Because risks negatively and directly affect production output, and indirectly affect household income, identifying and managing risk sources is of great importance to households (Drollette, 2009).
Risk is one of the important research topics to support effective management decisions in production, so the study of risk has received much attention from scholars and managers. A full understanding of farmers' risk perceptions and their management responses is important in designing appropriate and effective risk management systems/policies (Flaten et al., 2005). The literature review indicates that risks in agricultural production can be divided into production risk, price risk, disaster risk and technology risk (Miller et al., 2004). According to the research results of the European Commission, risks are classified into human health risks, institutional risks, financial risks, production risks, and input and output price risks. Besides, risk sources can also be divided into 5 main groups: production risk; market risk; financial risk; environmental and legal risk; and human resource risk (USDA, 1997). Although understanding about risk perception and factors affecting risk perception of farmers is important in managing and limiting losses in agricultural production, information about risk sources and the factors affecting farmers' awareness are quite limited in Vietnam. Therefore, this study was conducted to identify risk sources and factors affecting risk perception of commodity fruit farmers in the study area.
RESEARCH METHODOLOGY
Research and data collection
Son La is one of the central provinces of the Northwest Mountain region of Vietnam and is a province with strengths in the production of agricultural products and commodities with a total area of about over 147,272 hectares. The group of fruit trees with the largest production area includes longan, mango and citrus groups. Therefore, this study selects Son La province to conduct the research and collect data from July to October 2020.
Secondary and primary data are used in this study. Secondary data is the basic information collected from statistical reports in the area and is used to select the study area. Primary data is the key quantitative information used in the analysis of the results in this study. Key information was collected through interviews with farmers in the study area using a pre-designed questionnaire. Possible risks are selected based on the actual situation of agricultural commodity production in the locality and tested in practice through pilot interviews with a number of households before conducting formal data collection. The risks are divided into increasing levels from 1 to 5 based on the Likert scale, and farmers are asked to rank the risks within this range. Level 1 represents the lowest level of influence and level 5 implies the highest level of influence/importance. A total of 172 households were interviewed from the two districts of Mai Son and Van Ho - which represent the major fruit growing areas of the province.
Analytical methods and statistical processing
The risk perception of farmers in commodity fruit production was analyzed using descriptive statistical methods. Because in this study, the risk sources are quite large, the statistical technique - basic component analysis (PCA-Principal Component Analysis) is used to reduce the magnitude and dispersion of the variables. According to Abdi & William (2010), PCA is one of the statistical techniques that allows efficient analysis of data sets in which the quantitatively dependent variables are correlated with each other. The purpose of this technique is to extract the important information represented from the data set as a new set of variables called the PCA. PCA is an effective tool to reduce dispersion without losing important information. Therefore, this method is widely applied in many risk studies (Flaten et al., 2005; Meuwissen et al., 2001). Therefore, PCA was also selected for data analysis in this study. The relationship between farmers' perception of risk sources and socio-economic factors is explored by applying multiple linear regression models, and is described in the form of the formula as follows:
Yi = â0 + â1X1 + â2X2 + â3X3 + âijXi + åi
In which, Yi is the score of the household on risk perception achieved after PCA estimation (i = 1, 2, ... n); Xi are the explanatory variables of the analytical model (j = 1, 2, ..., n) and are described as in the table; âij is the coefficient estimated in the regression model; åi is the random error of the model.
Description of variables used in the regression model
The dependent variable is the household's perception of risk source - four basic components extracted from PCA analysis. The explanatory or independent variables of the model are selected on the basis of economic theory, the actual situation of agricultural production in the area and refer to the results of a number of related studies. Many studies have shown that household risk perception is influenced by household and farm characteristics such as age of household head, ethnicity, level of general education, occupation, labor force in agriculture, crop area, access to extension services and household credit (Flaten et al., 2005; Riwthong et al., 2017). Statistics for the variables are presented in Table 1. The panel data shows that the fruit-growing area of households varies widely from 0.02 to 6 ha, and the average area is quite small, about 0.83 ha /household. The average age of the household head is 44 years old, and 70% of the fruit farmers in the area are Kinh people. The main livelihood of farmers is based on agricultural production – accounting for 80% of surveyed households. The head of household mainly has a lower secondary education level. In the surveyed area, households producing fruit products according to VietGAP standards account for about 60% of the total surveyed households.
RESULTS AND DISCUSSION
Perception of risk sources of households
Perception of sources of risk in agricultural production of households are presented in Table 2. Accordingly, in the study area Farmers are aware that there are 14 sources of risk affecting their agricultural production. The perceived risk level of the household is ranked in column 2 (from left) based on the average risk source index. The risk index according to farmers' perception fluctuates from 2.55 to 4.18. A higher risk index implies a greater degree of influence on household output. In general, the agricultural output of the household is closely related and greatly influenced by the quality of seedlings, weather conditions and pests and diseases. This is the group of 3 risk sources with the highest ranking according to household perception. The quality of seedlings clearly has a great influence on the quality and yield of crops in general. Good seedlings (right species, and good health) will be a favorable premise for future care and good quality. On the contrary, bad seedlings will give low yield, even no yield. Healthy seedlings will grow faster, helping farmers reduce time and cost of fertilization. According to the survey, households all depend on the sources of seedlings purchased from different places, so it is easy for people to worry about the quality of seedlings. Agricultural production in general is greatly affected by weather conditions. And the change of weather conditions will directly affect the quality and output of agricultural products. This feature is also correlated with the development of pests and diseases. Therefore, pests and diseases are also one of the three sources of risk according to households that have a great influence on production results. The sources of risk that are assessed to have the least impact are respectively the loan interest rate and the State's regulations on product quality standards.
Before applying PCA, The Kaiser-Meyer-Olkin index (KMO) is estimated to assess the reliability of the sample data (sampling adequacy). In other words, the KMO indicator shows whether the data being used is suitable for the use of the PCA technique. According to Chow (2004), the KMO index fluctuates from 0 to 1 and the overall KMO index must be at least 0.5 or greater for the sample data to be considered appropriate. In this study, the KMO value was estimated to be 0.71, implying that the sample data are suitable for the application of the Principal Component Analysis technique. Table 3 presents the factor loading according to the perpendicular rotation of the risk sources. Through PCA, the number of risk sources is reduced from 14 variables to 4 components corresponding to an eigenvalue greater than 1. These four risk components have an explanatory value for about 63.6% total variance in this study. Based on the factor loading in Table 3 and the relationship between risk sources, components 1 – 4 are named “Finance,” “Human Resources,” “Production,” and “Market.” In component 1, the load factor of the capital risk variable has the largest value. This comes from the fact that the households need large investment capital, especially in the early stages of basic construction. In this first stage, farmers need a lot of investment capital (land preparation, seeds, fertilizers, etc.) for their orchards and this period lasts about 5 years without any revenue to cover costs. In addition, for farms with a harvest, the required annual investment capital is also very important. It is the addition of fertilizers, labor, and pest control for plants. Many households lack investment capital in the first stage of investment, so they need to borrow capital from credit institutions in the area. Therefore, interest rates and loan repayment are risks affecting farmers' perception.
The health of household heads and agricultural workers has a large coefficient of variation in the 2-Human components. Human resources play an important role in production in general and production in particular - the industry that requires the largest labor in Vietnam's economy today. Human resource risks reflect the lack of key workers – the head of the household and other workers involved in the production process. The head of the household is not only the main worker but also the one who makes decisions that can greatly affect production. Meanwhile, other agricultural labor affects the care and especially the stage of harvesting products to bring to the market. The lack of harvesting labor or the high cost of hired labor both directly and indirectly affect the production results (income) of farmers. Since fruit ripens and yields seasonally, lack of harvesting labor will lead to over ripens, fruit drop, and yield loss. While the high labor cost will increase production costs and directly reduce the income of farmers. In fact, the survey shows that the number of household workers involved in production is quite small - on average 2.3 people/household, and not enough to meet the requirements in the production process. Therefore, outsourcing labor is a mandatory requirement for households.
Seedling quality, pest and disease control, weather changes, postharvest preservation of agricultural products and changes in crop yield are grouped into component 3-Production. As analyzed above, good seedling quality greatly affects yield and product quality. In contrast, bad plant varieties will generate costs and production time for farmers. Therefore, the load factor in this component has the second largest value after the load factor of weather fluctuations. Fruit production takes place closely with external factors such as weather. Weather is an important factor and is beyond the control of farmers - farmers can only minimize the harm (if any) but cannot prevent it. On the other hand, changes in weather are closely related to pests and diseases. Favorable weather will positively affect productivity and product quality. The household's agricultural products are fresh fruits. Keeping fruit fresh and undamaged when reaching consumers directly affects the selling price of the product and the income of farmers. In addition, the farmer also assessed that the change in productivity also affects the production results of the household. In fact, fluctuations in crop yield are closely related to pests and diseases, seedling quality and unfavorable weather conditions.
About the component 4 “Market,” farmers' perception of risks related to the market, including product price fluctuations, customer tastes, regulations on product quality and production according to VietGAP standards. In this component, the largest load factor is the customer's taste, the second is production according to VietGAP standards, and the next is the product selling price and government regulations, respectively. Changes in market prices are beyond the control of farmers but directly affect household incomes. Consumer tastes are also related to price fluctuations. Customers prefer imported agricultural products - fruits will affect the price of domestic fruits. While consumer confidence in the quality of domestic products or VietGAP standard products will affect the selling price and income of farmers, state regulations on quality also affect the quality of products and their market in general. Adequate and reasonable regulations and strict enforcement of these regulations can increase consumer confidence in the quality of domestic agricultural products – indirectly affecting the selling price and income of households.
Factors influencing farmers' risk perception
Linear regression model Multivariate analysis was applied to determine the relationship between farmer's risk perception and influencing factors. The estimated coefficient (âij) and the fit of the regression model are presented in Table 4. The results show that all 4 models have high significance in terms of relevance in this study. According to Gujarati (2011), the low coefficient R2 value in the models is normal and acceptable because of the 1-year data type (cross-sectional data) with many observations with high dispersion. Synthesis of research literature also shows that many previous similar studies also have a rather low value of R2 coefficient (Meuwissen et al., 2001; Flaten et al., 2005).
Table 4 shows that there are 9 explanatory variables in the regression model. The estimated coefficients with different signs (+/-) reflect the relationship in different directions between the dependent variable and the explanatory variable. The results show that there is no explanatory variable that has a significant effect on household risk perception in all 4 regression models. In other words, farmers' perception of risk sources is significantly influenced by different factors. Occupation of household head and access to credit are two factors that have a statistically significant influence on perceived financial risk. Households with the main source of livelihood from agriculture perceive greater financial risks than other households. Because agricultural production is the main source of household income, the annual capital requirement for production investment is mandatory. For these households, the lack of capital or financial constraints means difficulties in production investment such as tilling, fertilizing, harvesting, and renovating orchards. No investment or limited investment such as insufficient fertilizer application and wrong time will directly affect the productivity and quality of output agricultural products of households. For households lacking production capital, finding sources of credit to borrow is necessary. Therefore, households receiving credit are perceived to have less financial risk to invest in production than households without loans.
Human resources are an important factor in agricultural production of households. There are two factors that significantly affect the risk perception on human resources of farmers, including the occupation of the household head and production methods according to VietGAP standards. The main occupation of the household head is agriculture, which means that the household's income is mainly based on agricultural production. While agricultural production is labor-intensive and concentrated with high seasonality, the lack of labor, especially in the care and harvesting stages, will directly affect crop yields and quality. Statistics show that with the number of agricultural workers/households being small and not enough for care and harvesting, every year households have to hire labor or exchange labor with other households in the area to overcome the situation of seasonal labor shortage. Households producing agricultural products according to VietGAP standards also have a similar perception of human risk. In addition to labor requirements in the production and harvesting of agricultural products in general, farmers producing agricultural products according to VietGAP standards need more labor than those producing non-standard products because production according to VietGAP standards requires more labor, stricter requirements on process and quality control. This also means that households producing according to VietGAP must use more labor per unit area than households not producing according to this standard. Therefore, it is easy to understand that households producing according to this standard consider human resources as one of the important factors for them.
Access to agricultural extension services and credit sources both have a significant influence on risk perception in production and markets. With production risks, the participation in technical training, access to information from agricultural extension services and loans for production helps farmers reduce worries in production such as lack of technology, lack of investment capital. As described in Table 3, seedling quality, pest control and storage are the primary risks in production. Providing a reliable seedling sales address, training on production techniques to prevent pests and diseases, and linking to support farmers to apply production standards according to VietGAP are the basic functions of agricultural extension work in the area. Besides, the application of VietGAP standards to agricultural products also helps households reduce market risks. Currently, the market's requirements for the quality of agricultural products are increasing, qualified agricultural products such as VietGAP contribute to stabilizing selling prices and increasing incomes for farmers. Besides, production certified VietGAP also leads to increased production costs (labor, certification costs, renewal...). Therefore, loans from credit funds also contribute to reducing worries for farmers in this case.
CONCLUSION
The study was carried out in Son La province - a locality with strengths in commodity fruit production in the northwest region of Vietnam. Descriptive statistical analysis was used to rank the sources of risk. PCA technique is implemented to reduce data dispersion, and retain the most important information. Next, a multivariate regression model was applied to determine the factors affecting the perception of risk sources of households. In this study, 14 risk sources were identified by fruit farmers in the area and ranked in order. Among these, the group of risk sources that can have the greatest impact on household production according to farmers' perceptions includes seedling quality, weather variability, and crop pests and diseases. After the PCA analysis, the risk sources are divided into 4 groups of basic components such as Finance, Human Resources, Production, and Market. The research results also show the important factors that explain the perception of farmers for each risk component. Notably, access to information from agricultural extension services and access to credit contributes to reducing concerns for farmers in production. Besides, producing products with good quality such as meeting VietGAP standards will contribute to reducing market risks for farmers. Therefore, policies to support agricultural production should aim to encourage farmers to produce agricultural products according to VietGAP standards. Additionally, maintaining and improving the efficiency of agricultural extension activities should be focused. In addition, increasing the level of access to credit to support agricultural production also contributes to helping farmers who lack capital to reduce risks in production and markets.
REFERENCES
Abdi, H. and Williams, LJ (2010), 'Principal component analysis', WIREs Computational Statistics, 2, 433–459. doi:10.1002/wics.101.
Akcaoz, H., & Ozkan, B. (2005), 'Determining risk sources and strategies among farmers of contrasting risk awareness: A case study for Cukurova region of Turkey', Journal of Arid Environment, 62, 661-675.
Alderman, H. (2008), 'Managing Risks to Increase Efficiency and Reduce Poverty', World Development Report 2008, World Bank, Washington, DC.
MARD, (2020), Report on Area of fruits tree in different regions of Viet Nam, http://www.cuctrongtrot.gov.vn/TinTuc/Index/4377 (accessed 22/3/2021).
Sơn La Statistical Office, (2019), Report on Area and Production of fruits tree in Sơn La province, https://sonla.gov.vn/4/469/63804/545755/thong-tin-ve-nong-san/nam-2019-tong-dien-tich-nong-san-cay-an-qua-chinh-cua-tinh-dat-147-272-ha (accessed 19/3/2021).
Drollette, SA (2009), Managing Production Risk in Agriculture, Department of Applied Economics, Utah State University, United States.
Flaten, O., Lien, G., Koesling, M., Valle, PS & Ebbesvik, M. (2005), 'Comparing risk perceptions and risk management in organic and conventional dairy farming: empirical results from Norway', Livestock Production Science, 95 (1–2): 11–25.
Gujarati, D. (2011), 'Econometrics by example', Palgrve Macmillan, Hamphire, UK, 25-81.
Jain, RCA, Parshad, M. (2007), 'Working Group on Risk Management in Agriculture for XI Five Year Plan', Government of India, Planning Commission, New Delhi.
Meuwissen, MPM, Huirne, RBM & Hardaker, JB (2001), 'Risk and risk management: An empirical analysis of Dutch livestock farmers', Livestock Production Science, 69, 43-53.
Miller, A., Dobbins, C., Pritchett, J., Boehlje, M. & Ehmke, C. (2004), Risk Management for Farmers, Staff Paper 04-11, Department of Agricultural Economics, Purdue University, 1-27.
Riwthong, S., Schreinemachers, P., Grovermann, C. & Berger, T. (2016), 'Agricultural commercialization: Risk perceptions, risk management and the role of pesticides in Thailand', Kasetsart Journal of Social Sciences (2016).
USDA. (1997), Introduction to Risk Management, United States Department of Agriculture, Risk Management Agency.