ABSTRACT
Malaysia’s recent national agendas are primed toward achieving triple-bottom line development, in-line with principles stipulated in the SDGs. Achieving this will require an agricultural sector that is robust, efficient and cost effective. Malaysia’s agricultural sector is distinguished by its dichotomous nature; primary commodities such as oil palm and rubber, and the agro-food branch with paddy and other food crops. It can also be viewed in terms of not only its obvious share of GDP, scale and value, but also in terms of its efficiency, productivity and level of sophistication and coordination. Precision agriculture (PA) techniques can be applied to address technical issues in the agriculture sector, such as reducing uncertainties, managing variabilities in farm management and allowing efficient use of resources. Its application in the palm oil industry enables temporal and spatially distributed data on trees, soil and environmental conditions of large plantation areas which are collected remotely. This aids management of plantation areas, disease detection and affirmative actions, as well as yield estimation. In the paddy industry, PA allows farmers to reduce input costs and increase planting efficiency. PA can be aligned to the needs of the palm oil industry to increase productivity and overcome its negative market image. In the paddy industry, PA technology has shown promising results, however it is plagued by high costs and an industry that is recalcitrant to new technologies.
Keywords: Precision agriculture, sustainability, food security, oil palm, paddy
INTRODUCTION
In line with Malaysia’s aspirations to achieve high-incomed nation status by 2020, a sustainable development structure has been the theme of Malaysia’s five-year economic national development plans. Within this context, sustainable development entails encompassing economic growth with a balanced and equitable growth distribution across all levels of society, ensuring societal well-being including access to education, healthcare, infrastructure and utilities, as well as emphasizing environmental conservation (EPU 2017). Furthermore, Malaysia’s New Economic Model (NEM) specifies the need and commitment to pursue sustainable development that hinges toward high income, inclusivity and sustainability, that is in-line with the triple-bottom line elements within the Sustainable Development Goals (SDGs).
Under the NEM, agriculture was identified as one of the 12 National Key Economic Areas that will spur economic growth through job creation and increasing income of farmers. In the latest Malaysian Plan (11th Malaysian Plan: 2016-2020), modernization and transformation of the agricultural sector is required to ensuring food security, increasing productivity, increasing skillsets of farmers, strengthening agro-food supply chain, as well as also enhancing related support and delivery service for all stakeholders. In 2017, the agriculture sector contributed 8.2 % of the RM1.2 trillion (USD314.5 billion) national Gross Domestic Product (GDP), with oil palm (46.6%) and other agriculture including agro-food sector (18.6%) as its main contributors (DOS 2018). Agriculture trade exports for 2017 was at a surplus of RM31 billion (USD8.2 billion), where oil palm was its main contributor at 102 million metric tons produced, follow by paddy at 2.6 million metric tons produced. The breakdown in terms of percentage share according to the type of economic activity can be seen in Figure 1.
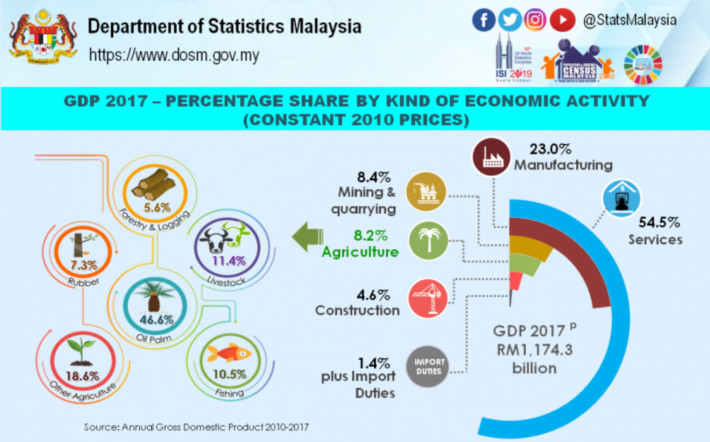
Figure 1. Malaysia 2017 GDP percentage share according to economic activity
Although the agriculture sector has witnessed a shrinkage in its GDP share, where it was more than 50% of total GDP in the 1960s, due to the nation’s industrialization emphasis through diversification of its economic drivers, this sector has always been given priority in various national policies and plans. In developing countries where the agricultural sector has large aggregate effects, there are correlations between economic growth and agricultural productivity increases, however for countries like Malaysia, agriculture influenced growth is also dependent on its feasibility and cost of importing food (Gollin 2010).
Agriculture in Malaysia can be divided into two main branches; primary commodities (oil palm, rubber) with its inherent economic value on one side, and agro-food production with its role in achieving national food security on the other. The dichotomous nature of Malaysia’s agricultural sector can also be viewed in terms of not only its obvious share of GDP, scale and value, but also in terms of its efficiency, productivity and level of sophistication and coordination. National initiatives and plans are geared towards achieving higher yields and productivity, as well as addressing issues such as volatile prices, unfavorable market outlook, pests and diseases, sustainable use of fertilizers and pesticides, and an ageing farmer demographic (Nordin 2018). The agrofood sector in particular, faces structural and supply-side challenges that are related to scarcity of land, labor, input and capital (Ahmad and Suntharalingam 2009), where without innovation and technological advancement, this sector will be uncompetitive.
With increasing public awareness and global trend towards food safety and use of hazardous chemicals in agriculture, PA also offers a more effective and efficient method to ensure accurate application of pesticides and fertilizers that are within the recommended safety limits. A recent study on sustainability practices among paddy farmers in Sungai Petani, Kedah paints an alarming picture on current farming practice that is influenced by pressure to achieve yield target, profitability and poor management practice. It was found that 80% of farmers practice unsustainable farming practices, including exceeding recommended amount of pesticide application and not following pesticide application schedule (Mohamed et al. 2016).
Precision agriculture (PA) techniques can be applied to address technical issues in the agriculture sector. When applied correctly, PA reduces uncertainties and related to managing variabilities in farm management and allows efficient use of resources. It can also be defined as an integrated, information and production-based farming system that allows increase in efficiency, productivity and profitability, as well as reducing undesirable excess chemical loading that would bring adverse effect to the environment and reduce profitability (Liaghat and Balasundram 2010). The importance of oil palm as a primary commodity to the economy, and paddy as an essential factor for food security cannot be understated. This paper aims to provide a review of recent research activities undertaken on PA in both fields that will provide a snapshot of significant findings and a general overview of the motivations, challenges and remediations related to both fields in Malaysia.
MALAYSIA’S PRECISION AGRICULTURE INITIATIVES
Salient features of precision agriculture
PA can be further elucidated by dividing it into its three main elements as depicted in Figure 2 (Schwarz, Herold, and Pölling 2011). Guidance Systems refers to the hardware and software that allows control, guidance and assistance of tractors and farm implements, either in an automated steering or automated machinery configurations. Gathering of information including condition mapping (soil, soil moisture, canopy and yield), environmental conditions and pesticide efficacy that are done with singular or an array of modules/sensors, are categorized under Recording Technologies. Finally, Reacting Technologies are implements and equipment that enable application of farming inputs at various locations and at different rates. This includes technologies such as variable rate applicators of seed, fertilizers and pesticides, as well as variable rate irrigation and weeding. To aid visualization of PA initiatives in Malaysia, Figure 2 allows visualization and characterization of the many initiatives that was carried out in both branches of the agricultural sector.
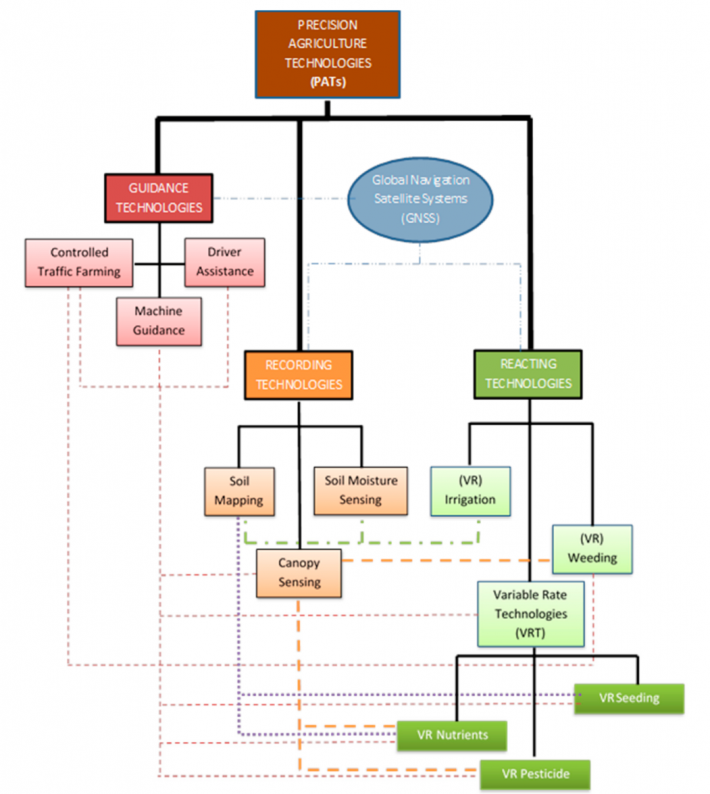
Figure 2. Overview of main elements in PA
Precision agriculture in the Malaysian oil palm industry
PA in the oil palm industry centers around the usage of remote sensing techniques, where temporal and spatially distributed data on trees, soil and environmental conditions of large plantation areas are collected remotely. Table 1 summarizes the three main technologies used in remote sensing to obtain these data. Furthermore, due to its monoculture planting practices and its unique topographical pattern (Figure 3), oil palm trees are distinguishable from other trees or forest when viewed using satellite imagery (H. Z. M. Shafri et al. 2011b). This uniformity allows operators to have a good layout of the plantation, that in turn will aid management of large oil palm plantation areas, where daily operations such as harvesting, manuring, pruning and weeding are carried out. Through remote sensing, monitoring of status and progress of oil palm development can be done in a timely, repetitive and accurately (Chong et al. 2017).
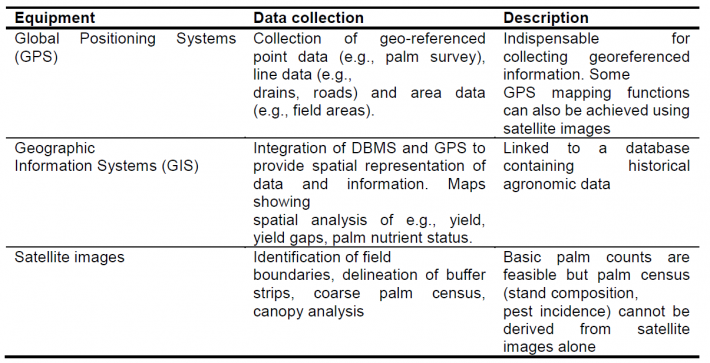
Table 1. Remote sensing equipment used in PA for palm oil industry (Adopted from Griffiths et al. 2003)
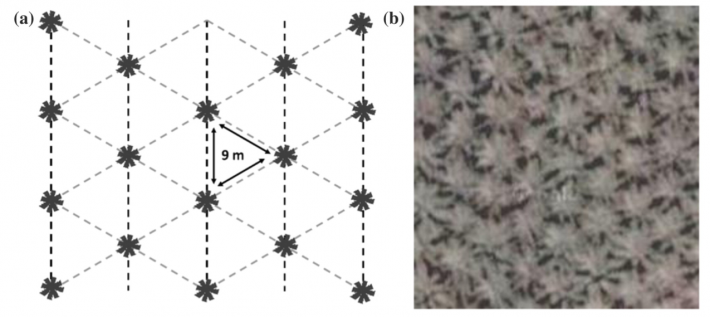
Figure 3. Oil palm plantation pattern and its Worldview-2 satellite image taken on with spatial resolution of 05m
Identification or classification of oil palm canopy from its adjacent topographical features including forests, buildings, bare land, body of water, or even other types of plants is essential in the distinction of plantation boundaries, as well as accuracy in determining temporal oil palm area coverage and related land activities (Chong et al. 2017; Nooni et al. 2014). Identification is done by analyzing distinctive spectral signatures given off by reflected energy from these different topographical features. Some promising techniques include, an L-band microwave remote sensing that is capable of mapping forested vegetation under cloud cover with increased definition of providing information on sub-canopy structures (Teng et al. 2015; Ibharim et al. 2015), and a technique that optically fuses LiDAR (light detection and ranging) and that is able to provide both information on canopy height and terrain elevation in a 3D perspective (Figure 4), facilitating plantation planning and management (Razi, Ismail, and Shafri 2013).
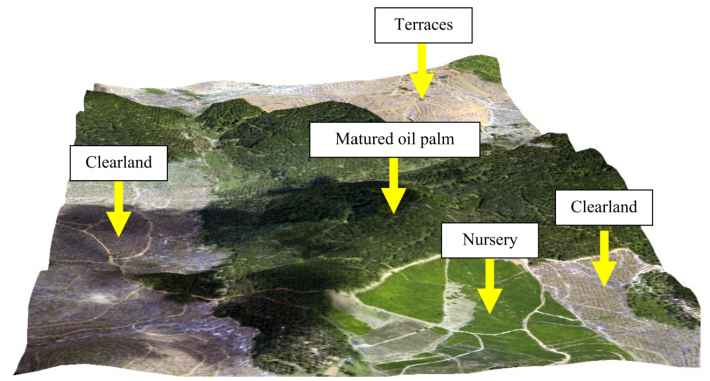
Figure 4. A 3D model obtained from fusion analysis of LiDAR, Digital Elevation Model and aerial photo (H. Shafri et al. 2012)
Using remote sensing techniques to detect pests and diseases in palm oil plantation is an area of great interest, particularly with respect to plantation management and early detection of threats. Ganoderma boninensis is a highly contagious fungal disease that causes internal rotting and subsequently leaving tree trunks structurally compromised and susceptible to collapsing during high wind (Liaghat and Balasundram 2010). Removal of infected trees through early detection and quarantine can be more efficiently done using remote sensing techniques. Early studies using portable field hyperspectral instrument to detect different spectral reflectance of leaves has shown some promise in categorizing severity of Ganoderma-infected trees (H. Z. M. Shafri et al. 2011a). Figure 5 depicts comparison of different image processing techniques to improve Ganoderma detection accuracy. Simple Ratio Index was found to give the best results in classifying infected trees, however more refined image processing and analysis are needed to improve accuracy of early detection, particularly in differentiating mild and severe infection (Izzuddin et al. 2018).
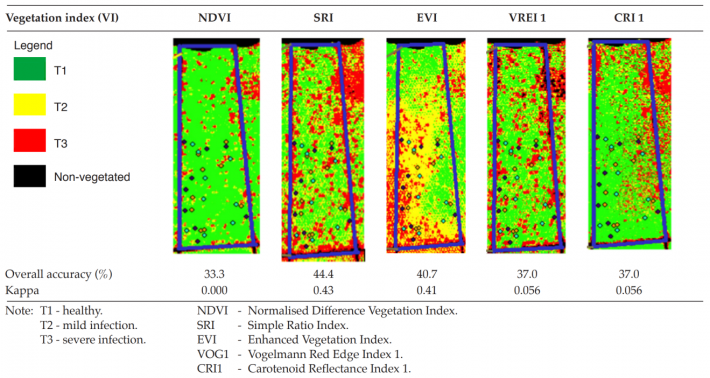
Figure 5. Detection of various stages of Ganoderma boninensis infection using airborne hyperspectral image processing
Yield and age of palm estimation in palm oil plantation are also important metrics that influence not only the overall bottom line, but also in maximizing profits by manipulating planting and replanting schedule in relation to market price. Ripened fruit bunches are not only hidden under the crown, but located at the lowest part of the bunches, making overhead imaging difficult. Thus, estimation of yield requires an analysis of various temporal parameters such as age, breed, rainfall, soil fertility, soil conditions and harvesting efficiency (Chong et al. 2017). When a 12 year time series data of yield and vegetation indices were analyzed using Ratio Vegetation Index (RVI) model, a good correlation of estimated and observed yield was obtained (Figure 6), indicating that high yielding trees have dense, robust and healthy canopies based on the positive correlation between vegetation indices value, Leaf Area Index (LAI) and yield (Chong et al. 2017; Balasundram, Memarian, and Khosla 2013).
Figure 6. RVI model used to estimate yield using vegetation indices derived from QuickBird satellite image
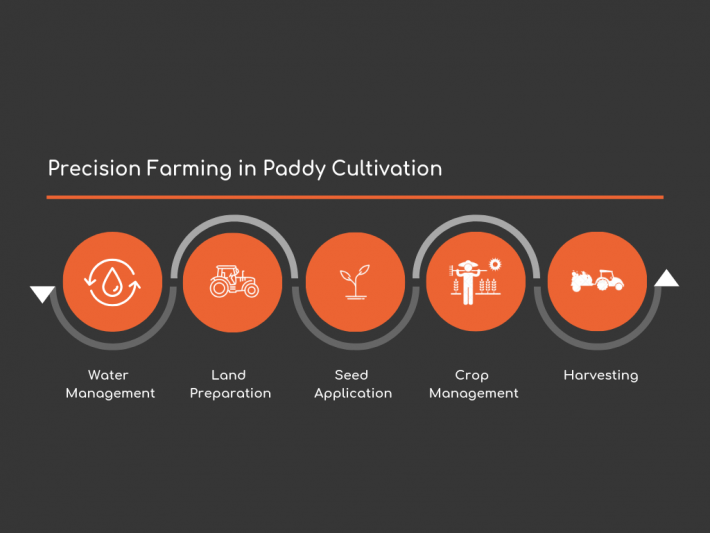
Figure 7. Essential processes of paddy cultivation
Precision agriculture in Malaysian paddy industry
Technology uptake and utilization of technology in the form of mechanization, modern varieties and farm management tools among paddy farmers was found to be at a moderate level, where the highest technology use was found in fertilizing, ploughing and seeding (Harun et al. 2015). The potential of implementing PA practices is observed to be more viable in granary areas, where it is under the management of large corporations or cooperatives.
PA elements in Figure 1 can be applied within the context of paddy farming, in which, geospatial variabilities in each process are monitored and managed. Figure 7 depicts the five main processes involved in paddy cultivation in Malaysia. PA in water management for paddy cultivation aims to find a balanced compromise of various factors such as supporting canal systems, hydraulic units, rice field conditions, field off-take, inflow and farming practices that will provide site-specific irrigation at the right amount and at the right time. By combining monitoring of hydrological, climatological and administrative parameters that are tagged to geospatial locations, a GIS-based water management model is capable of monitoring and scheduling daily crop water requirement. A GIS user-interface technique linked with water management model developed by Rowshon and Amin (2010) is capable of assisting and improving decision making process in water management (Rowshon and Amin 2010). Figure 8 illustrates the computed amount of water required (RWS) based on parameters such as Irrigation Requirement (IR), Rainfall (RF), Effective Rainfall (ER), Drainage Requirement (DR) and Total Water Depth (TWD = IR + ER) for specific locations within the observed grid.
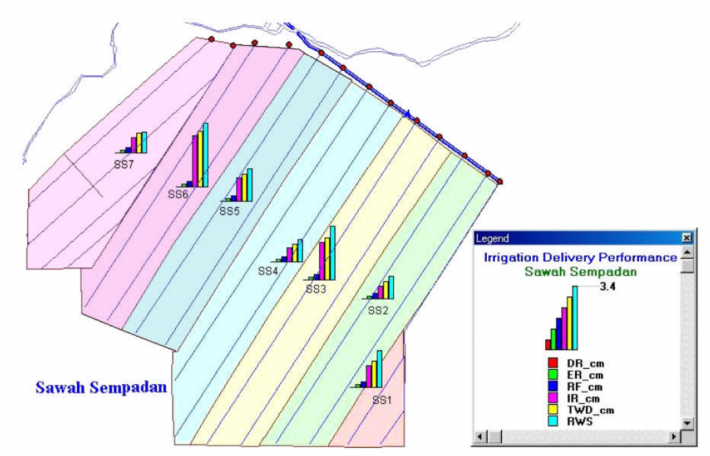
Figure 8. GIS based computer model to compute daily spatial water requirement
In land preparation, understanding the topographical features of the soil is essential to ensure good agronomic, soil and crop management practice can be carried out in the subsequent processes. Ideally, a flat and uniformly levelled land will ensure uniform crop establishment and provide adequate protection from competing weed growth when the fields are flooded. In PA, a GIS-based land survey is carried out to map out land level features and to obtain the land level index to be used in the seeding map. Based on the methods to calculate levelling index developed by Agarwal and Goel (1981), each 10 x 10m grid are designated with either ‘even’, ‘high’ or ‘low’ in the seeding map. Subsequently, seeding is done based on the land levels observed by the model, where 10 kg is added to the 120kg/ha seeding rate for grids that are designated with ‘low’ (Figure 8). When this levelling system is used in tandem with this site-specific seed application or variable rate (VRT) seed application method, it was found to produce more consistent crop establishment compared to conventional broadcast seeding (Abu Bakar et al. 2017).
Conventional soil nutrient classification requires an assumption that a sampling point represents the status of the specified area and variabilities within it are randomly distributed. PA aids soil preparation process by eliminating the need of tedious manual sampling, as well as intensive and time-consuming laboratory analysis. Quantification of site-specific nutrient status of soil will allow nutrient application to be done accurately. This can be done on a macro level where aerial images are made to map out plantation areas and subsequent sampling sites are identified and analyzed with geostatistical tools to obtain a nutrient distribution model (Amin and Wayayok 2003; Aishah et al. 2010). It can also be done on site with sensors that work in tandem with GPS enabled mapping system that will be fed into the nutrient distribution model (Aimrun et al. 2007; Aliah Baharom et al. 2015).
VRT in fertilizer application offers the capability of providing nutrients specifically to the plant’s requirement. Conventionally, this is done on the field by blanket spreading without discrimination and reference to the plant’s condition, as well as available soil nutrient content. This eventually will result in uneven application of fertilizer, either under supplying required nutrients to the plants that will adversely affect plant growth, or oversupplying it, causing increased input cost and harmful environmental effects. In relation to nutrient classification process, MARDI’s VRT Fertilizer system based on Green Area Index (GAI) model, enables specific rate of fertilizer application to be made based on soil fertility and plant condition. This system includes a GIS-based mapping system that is processed to obtain Soil Plant Analysis Development (SPAD) and GAI maps, that will be used to project a GAI treatment map (Figures 9 and 10). This map will be stored in an online server and used by farmers to apply fertilizers accordingly. This data is then compiled into a geospatial and temporal representation of soil fertility for subsequent planting seasons. This system was observed to save 17% of total fertilization cost with an increase of about 20% efficiency over conventional fertilization methods.
Way forward for PA in Malaysian oil palm and paddy industries
Jalani et al. (2002) posited that to increase production yield, issues such as inadequate agronomic inputs, shortage of skilled labor, as well as ineffective and inadequate field maintenance must be overcome. PF technologies and its application in agriculture continue to push boundaries and are capable of addressing these issues. PA technologies allow farms to increase their productivity and economics, thus translating into higher yields with lower production cost, compared to conventional practices (Balafoutis et al. 2017). Progress can be made in the form of more accurate and precise sensors to identify and monitor parameters, higher resolution and capable cameras that offer more accuracy across the spectrum, as well as more robust and dynamic algorithms to power Decision Support Systems (DSS) for it to better understand vagaries of nature and crop requirements. For the oil palm industry, PA also offers an opportunity to overcome its biggest challenge to date, where public opinion of it supposed detrimental effect on the environment continues to adversely affect palm oil and its derivative market prices. PA technologies such as VRT for fertilizers, seed and irrigation were found to contribute to the reduction of GHG emissions (Balafoutis et al. 2017). In addition, more accurate and sustainable plan of expansion and management of palm oil plantations can be carried out more efficiently, by taking into account of greenhouse gas (GHG) contribution and land use change effects.
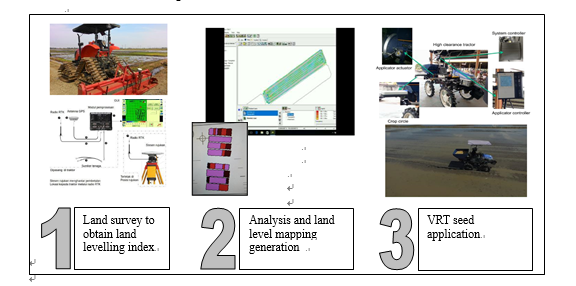
Figure 9. MARDI’s GIS-based land levelling and seed VRT system
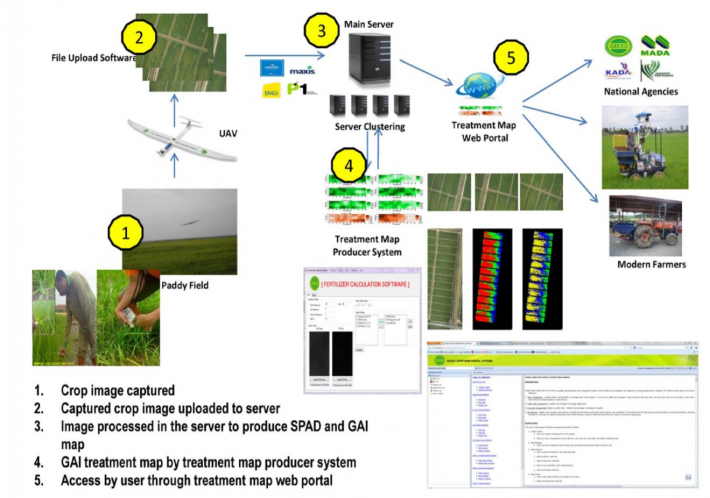
Figure 10. MARDI' s VRT fertilization system
Yield and productivity challenges seen in palm oil can also be applied in Malaysia’s paddy industry. Sustainability and lowering of GHG emissions in the paddy production system continues to rank high in national agendas. High tech equipment used in PA can provide site specific applications and deliver accurate inputs depending on the spatial and temporal requirements, thus lowering its GHG emissions. Here, a robust DSS that compiles information and facilities from all related agencies will aid farmers to better manage their farms. A dynamic system such as those developed by Norasma et al. (2013) can be upscaled and improved using mobile applications or in an autonomous farming system. Despite the promise, technology adoption continues to be challenging not only because of the high cost factor, but also due to farmers recalcitrant willingness to change or adapt to new techniques (Harun et al. 2015). National policies can aid reducing or spreading burden of high cost; however, PA innovations must continue to achieve higher accuracy and efficiency to warrant its higher cost investments.
CONCLUSION
The importance of the agriculture sector to Malaysia’s aspirations necessitates implementation of PA technologies to overcome market challenges, increase productivity, achieving food security, as well as also ensuring national development is done sustainably. Existing PA technologies are providing promising results when compared to conventional practices and emphasis on sustainable agriculture in national agendas will propel more research on PA technologies in order for it to be more accurate, efficient and feasible.
REFERENCES
Abu Bakar, B, M. A. M. Bookeri, S. S. Mohamad Ghazali, M. S. Abd. Rahman, M. T. Ahmad, and R. Abdullah, M. Z. K. Ismail. 2017. “Automated Land Leveling Index Determination for Variable Rate Seeding of Paddy.” In Proceedings of International Conference on Sustainable Development ICoSD 2017. Putrajaya, Malaysia.
Agarwal, M C, and A C Goel. 1981. “Effect of Field Levelling Quality on Irrigation.” Agric Water Manag 4: 457–64.
Ahmad, Tengku Ariff Tengku, and Chubashini Suntharalingam. 2009. “Transformation and Economic Growth of the Malaysian Agricultural Sector.” Economic and Technology Management Review 4: 1–10.
Aimrun, W, M S M Amin, Desa Ahmad, M M Hanafi, and C S Chan. 2007. “Spatial Variability of Bulk Soil Electrical Conductivity in a Malaysian Paddy Field: Key to Soil Management.” Paddy and Water Environment 5 (2): 113–21. https://doi.org/10.1007/s10333-007-0072-z.
Aishah, A W, S Zauyah, A R Anuar, and C I Fauziah. 2010. “Spatial Variability of Selected Chemical Characteristics of Paddy Soils in Sawah Sempadan , Selangor , Malaysia” 14: 27–39.
Aliah Baharom, Siti Noor, Sakae Shibusawa, Masakazu Kodaira, and Ryuhei Kanda. 2015. “Multiple-Depth Mapping of Soil Properties Using a Visible and near Infrared Real-Time Soil Sensor for a Paddy Field.” Engineering in Agriculture, Environment and Food 8 (1): 13–17. https://doi.org/https://doi.org/10.1016/j.eaef.2015.01.002.
Amin, M S M, and Aimrun Wayayok. 2003. “Spatial Variability of Total Nitrogen , and Available Phosphorus of Spatial Variability of Total Nitrogen , and Available Phosphorus of Large Rice Field In,” no. January.
Balafoutis, Athanasios, Bert Beck, Spyros Fountas, Jurgen Vangeyte, Tamme Van Der Wal, Iria Soto, Manuel Gómez-Barbero, Andrew Barnes, and Vera Eory. 2017. “Precision Agriculture Technologies Positively Contributing to Ghg Emissions Mitigation, Farm Productivity and Economics.” Sustainability (Switzerland) 9 (8): 1–28. https://doi.org/10.3390/su9081339.
Balasundram, Siva K, Hadi Memarian, and Raj Khosla. 2013. “Estimating Oil Palm Yields Using Vegetation Indices Derived from QuickBird,” no. January.
Chong, Khai Loong, Kasturi Devi Kanniah, Christine Pohl, and Kian Pang Tan. 2017. “Geo-Spatial Information Science A Review of Remote Sensing Applications for Oil Palm Studies A Review of Remote Sensing Applications for Oil Palm Studies” 20 (2): 184–200. https://doi.org/10.1080/10095020.2017.1337317.
DOS, DEPARTMENT OF STATISTICS MALAYSIA. 2018. “Malaysia Selected Agricultural Indicators.” 2018. https://www.dosm.gov.my/v1/index.php?r=column/cthemeByCat&cat=72&bul_id=....
EPU, Economic Planning Unit. 2017. “Malaysia Sustainable Development Goals Voluntary National Review 2017.”
Gollin, Douglas. 2010. “Chapter 73 Agricultural Productivity and Economic Growth.” Handbook of Agricultural Economics 4 (January): 3825–66. https://doi.org/10.1016/S1574-0072(09)04073-0.
Griffiths, William, Thomas Fairhurst, Ian Rankine, Armin Gfroerer Kerstan, Vince Mcaleer, and Clive Taylor. 2003. “A Conceptual Framework for Precision Agriculture in Oil Palm.”
Harun, Rosnani, Syahrin Suhaimee, Mohd Zaffrie, Mohd Amin, and Nurul Huda Sulaiman. 2015. “Benchmarking and Prospecting of Technological Practices in Rice Production” M: 77–88.
Ibharim, N A, M A Mustapha, T Lihan, and A G Mazlan. 2015. “Mapping Mangrove Changes in the Matang Mangrove Forest Using Multi Temporal Satellite Imageries.” Ocean & Coastal Management 114: 64–76. https://doi.org/https://doi.org/10.1016/j.ocecoaman.2015.06.005.
Izzuddin, M. A., M. N. Nisfariza, B. Ezzati, A. S. Idris, M. D. Steven, and D. Boyd. 2018. “Analysis of Airborne Hyperspectral Image Using Vegetation Indices, Red Edge Position and Continuum Removal for Detection of Ganoderma Disease in Oil Palm.” Journal of Oil Palm Research 30 (3): 416–28. https://doi.org/10.21894/jopr.2018.0037.
Jalani, BS, Yusof Basiron, Ariffin Darus, KW Chan, and N Rajanaidu. 2002. “Prospects ofElevating National Palm Productivity:A Malaysian Perspective.” Oil Palm Industry Economic Journal 2 (2): 1–9.
Liaghat, S., and S.K. Balasundram. 2010. “A Review: The Role of Remote Sensing in Precision Agriculture.” American Journal OfAgricultural and Biological Sciences 5 (1): 50–55.
Mohamed, Zainalabidin, Rika Terano, Mad Nasir Shamsudin, and Ismail Abd Latif. 2016. “Paddy Farmers ’ Sustainability Practices in Granary.” https://doi.org/10.3390/resources5020017.
Nooni, I K, A A Duker, I Van Duren, L Addae-Wireko, and E M Osei Jnr. 2014. “Support Vector Machine to Map Oil Palm in a Heterogeneous Environment.” International Journal of Remote Sensing 35 (13): 4778–94. https://doi.org/10.1080/01431161.2014.930201.
Norasma, C Y N, A R M Shariff, E Jahanshiri, M S M Amin, and A R Mahmud. 2013. “SCIENCE & TECHNOLOGY Web-Based Decision Support System for Paddy Planting Management” 21 (2).
Nordin, Khairani Afifi. 2018. “Agriculture: Addressing Food Security in Malaysia.” The Edge Markets, 2018. https://www.theedgemarkets.com/article/agriculture-addressing-food-secur....
Razi, Mohamad, Mohd Hasmadi Ismail, and Helmi Shafri. 2013. “Fusion Technique of LiDAR and Airphoto Imagery for Oil Palm Classification.” 34th Asian Conference on Remote Sensing 2013, ACRS 2013 2 (January): 1317–25.
Rowshon, MK, and MSM Amin. 2010. “GIS-Based Irrigation Water Management for Precision Farming of Rice.” Int J Agric & Biol Eng Open 3 (3): 27–35. https://doi.org/10.3965/j.issn.1934-6344.2010.01.027-035.
Schwarz, J, L Herold, and B Pölling. 2011. “Typology of PF Technologies; FP7 Project Future Farm.” http://www.futurefarm.eu/.
Shafri, Helmi, Mohd Hasmadi Ismail, Mohamad Razi, Izzuddin Anuar, and Abdul Rahman Ahmad. 2012. “Application of LiDAR and Optical Data for Oil Palm Plantation Management in Malaysia.” Proc SPIE 8526 (November): 8. https://doi.org/10.1117/12.979631.
Shafri, Helmi Z.M., Mohd I. Anuar, Idris A. Seman, and Nisfariza M. Noor. 2011a. “Spectral Discrimination of Healthy and Ganoderma-Infected Oil Palms from Hyperspectral Data.” International Journal of Remote Sensing 32 (22): 7111–29. https://doi.org/10.1080/01431161.2010.519003.
Shafri, Helmi Z M, Mohd I Anuar, Idris A Seman, and Nisfariza M Noor. 2011b. “Spectral Discrimination of Healthy and Ganoderma-Infected Oil Palms from Hyperspectral Data.” International Journal of Remote Sensing 32 (22): 7111–29. https://doi.org/10.1080/01431161.2010.519003.
Teng, K C, J Y Koay, S H Tey, K S Lim, H T Ewe, and H T Chuah. 2015. “A Dense Medium Microwave Backscattering Model for the Remote Sensing of Oil Palm.” IEEE Transactions on Geoscience and Remote Sensing 53 (6): 3250–59. https://doi.org/10.1109/TGRS.2014.2372796.
Date submitted: August 5~9, 2019
Reviewed, edited and uploaded: August 15, 2019
|
Precision Agriculture in Malaysia
ABSTRACT
Malaysia’s recent national agendas are primed toward achieving triple-bottom line development, in-line with principles stipulated in the SDGs. Achieving this will require an agricultural sector that is robust, efficient and cost effective. Malaysia’s agricultural sector is distinguished by its dichotomous nature; primary commodities such as oil palm and rubber, and the agro-food branch with paddy and other food crops. It can also be viewed in terms of not only its obvious share of GDP, scale and value, but also in terms of its efficiency, productivity and level of sophistication and coordination. Precision agriculture (PA) techniques can be applied to address technical issues in the agriculture sector, such as reducing uncertainties, managing variabilities in farm management and allowing efficient use of resources. Its application in the palm oil industry enables temporal and spatially distributed data on trees, soil and environmental conditions of large plantation areas which are collected remotely. This aids management of plantation areas, disease detection and affirmative actions, as well as yield estimation. In the paddy industry, PA allows farmers to reduce input costs and increase planting efficiency. PA can be aligned to the needs of the palm oil industry to increase productivity and overcome its negative market image. In the paddy industry, PA technology has shown promising results, however it is plagued by high costs and an industry that is recalcitrant to new technologies.
Keywords: Precision agriculture, sustainability, food security, oil palm, paddy
INTRODUCTION
In line with Malaysia’s aspirations to achieve high-incomed nation status by 2020, a sustainable development structure has been the theme of Malaysia’s five-year economic national development plans. Within this context, sustainable development entails encompassing economic growth with a balanced and equitable growth distribution across all levels of society, ensuring societal well-being including access to education, healthcare, infrastructure and utilities, as well as emphasizing environmental conservation (EPU 2017). Furthermore, Malaysia’s New Economic Model (NEM) specifies the need and commitment to pursue sustainable development that hinges toward high income, inclusivity and sustainability, that is in-line with the triple-bottom line elements within the Sustainable Development Goals (SDGs).
Under the NEM, agriculture was identified as one of the 12 National Key Economic Areas that will spur economic growth through job creation and increasing income of farmers. In the latest Malaysian Plan (11th Malaysian Plan: 2016-2020), modernization and transformation of the agricultural sector is required to ensuring food security, increasing productivity, increasing skillsets of farmers, strengthening agro-food supply chain, as well as also enhancing related support and delivery service for all stakeholders. In 2017, the agriculture sector contributed 8.2 % of the RM1.2 trillion (USD314.5 billion) national Gross Domestic Product (GDP), with oil palm (46.6%) and other agriculture including agro-food sector (18.6%) as its main contributors (DOS 2018). Agriculture trade exports for 2017 was at a surplus of RM31 billion (USD8.2 billion), where oil palm was its main contributor at 102 million metric tons produced, follow by paddy at 2.6 million metric tons produced. The breakdown in terms of percentage share according to the type of economic activity can be seen in Figure 1.
Figure 1. Malaysia 2017 GDP percentage share according to economic activity
Although the agriculture sector has witnessed a shrinkage in its GDP share, where it was more than 50% of total GDP in the 1960s, due to the nation’s industrialization emphasis through diversification of its economic drivers, this sector has always been given priority in various national policies and plans. In developing countries where the agricultural sector has large aggregate effects, there are correlations between economic growth and agricultural productivity increases, however for countries like Malaysia, agriculture influenced growth is also dependent on its feasibility and cost of importing food (Gollin 2010).
Agriculture in Malaysia can be divided into two main branches; primary commodities (oil palm, rubber) with its inherent economic value on one side, and agro-food production with its role in achieving national food security on the other. The dichotomous nature of Malaysia’s agricultural sector can also be viewed in terms of not only its obvious share of GDP, scale and value, but also in terms of its efficiency, productivity and level of sophistication and coordination. National initiatives and plans are geared towards achieving higher yields and productivity, as well as addressing issues such as volatile prices, unfavorable market outlook, pests and diseases, sustainable use of fertilizers and pesticides, and an ageing farmer demographic (Nordin 2018). The agrofood sector in particular, faces structural and supply-side challenges that are related to scarcity of land, labor, input and capital (Ahmad and Suntharalingam 2009), where without innovation and technological advancement, this sector will be uncompetitive.
With increasing public awareness and global trend towards food safety and use of hazardous chemicals in agriculture, PA also offers a more effective and efficient method to ensure accurate application of pesticides and fertilizers that are within the recommended safety limits. A recent study on sustainability practices among paddy farmers in Sungai Petani, Kedah paints an alarming picture on current farming practice that is influenced by pressure to achieve yield target, profitability and poor management practice. It was found that 80% of farmers practice unsustainable farming practices, including exceeding recommended amount of pesticide application and not following pesticide application schedule (Mohamed et al. 2016).
Precision agriculture (PA) techniques can be applied to address technical issues in the agriculture sector. When applied correctly, PA reduces uncertainties and related to managing variabilities in farm management and allows efficient use of resources. It can also be defined as an integrated, information and production-based farming system that allows increase in efficiency, productivity and profitability, as well as reducing undesirable excess chemical loading that would bring adverse effect to the environment and reduce profitability (Liaghat and Balasundram 2010). The importance of oil palm as a primary commodity to the economy, and paddy as an essential factor for food security cannot be understated. This paper aims to provide a review of recent research activities undertaken on PA in both fields that will provide a snapshot of significant findings and a general overview of the motivations, challenges and remediations related to both fields in Malaysia.
MALAYSIA’S PRECISION AGRICULTURE INITIATIVES
Salient features of precision agriculture
PA can be further elucidated by dividing it into its three main elements as depicted in Figure 2 (Schwarz, Herold, and Pölling 2011). Guidance Systems refers to the hardware and software that allows control, guidance and assistance of tractors and farm implements, either in an automated steering or automated machinery configurations. Gathering of information including condition mapping (soil, soil moisture, canopy and yield), environmental conditions and pesticide efficacy that are done with singular or an array of modules/sensors, are categorized under Recording Technologies. Finally, Reacting Technologies are implements and equipment that enable application of farming inputs at various locations and at different rates. This includes technologies such as variable rate applicators of seed, fertilizers and pesticides, as well as variable rate irrigation and weeding. To aid visualization of PA initiatives in Malaysia, Figure 2 allows visualization and characterization of the many initiatives that was carried out in both branches of the agricultural sector.
Figure 2. Overview of main elements in PA
Precision agriculture in the Malaysian oil palm industry
PA in the oil palm industry centers around the usage of remote sensing techniques, where temporal and spatially distributed data on trees, soil and environmental conditions of large plantation areas are collected remotely. Table 1 summarizes the three main technologies used in remote sensing to obtain these data. Furthermore, due to its monoculture planting practices and its unique topographical pattern (Figure 3), oil palm trees are distinguishable from other trees or forest when viewed using satellite imagery (H. Z. M. Shafri et al. 2011b). This uniformity allows operators to have a good layout of the plantation, that in turn will aid management of large oil palm plantation areas, where daily operations such as harvesting, manuring, pruning and weeding are carried out. Through remote sensing, monitoring of status and progress of oil palm development can be done in a timely, repetitive and accurately (Chong et al. 2017).
Table 1. Remote sensing equipment used in PA for palm oil industry (Adopted from Griffiths et al. 2003)
Figure 3. Oil palm plantation pattern and its Worldview-2 satellite image taken on with spatial resolution of 05m
Identification or classification of oil palm canopy from its adjacent topographical features including forests, buildings, bare land, body of water, or even other types of plants is essential in the distinction of plantation boundaries, as well as accuracy in determining temporal oil palm area coverage and related land activities (Chong et al. 2017; Nooni et al. 2014). Identification is done by analyzing distinctive spectral signatures given off by reflected energy from these different topographical features. Some promising techniques include, an L-band microwave remote sensing that is capable of mapping forested vegetation under cloud cover with increased definition of providing information on sub-canopy structures (Teng et al. 2015; Ibharim et al. 2015), and a technique that optically fuses LiDAR (light detection and ranging) and that is able to provide both information on canopy height and terrain elevation in a 3D perspective (Figure 4), facilitating plantation planning and management (Razi, Ismail, and Shafri 2013).
Figure 4. A 3D model obtained from fusion analysis of LiDAR, Digital Elevation Model and aerial photo (H. Shafri et al. 2012)
Using remote sensing techniques to detect pests and diseases in palm oil plantation is an area of great interest, particularly with respect to plantation management and early detection of threats. Ganoderma boninensis is a highly contagious fungal disease that causes internal rotting and subsequently leaving tree trunks structurally compromised and susceptible to collapsing during high wind (Liaghat and Balasundram 2010). Removal of infected trees through early detection and quarantine can be more efficiently done using remote sensing techniques. Early studies using portable field hyperspectral instrument to detect different spectral reflectance of leaves has shown some promise in categorizing severity of Ganoderma-infected trees (H. Z. M. Shafri et al. 2011a). Figure 5 depicts comparison of different image processing techniques to improve Ganoderma detection accuracy. Simple Ratio Index was found to give the best results in classifying infected trees, however more refined image processing and analysis are needed to improve accuracy of early detection, particularly in differentiating mild and severe infection (Izzuddin et al. 2018).
Figure 5. Detection of various stages of Ganoderma boninensis infection using airborne hyperspectral image processing
Yield and age of palm estimation in palm oil plantation are also important metrics that influence not only the overall bottom line, but also in maximizing profits by manipulating planting and replanting schedule in relation to market price. Ripened fruit bunches are not only hidden under the crown, but located at the lowest part of the bunches, making overhead imaging difficult. Thus, estimation of yield requires an analysis of various temporal parameters such as age, breed, rainfall, soil fertility, soil conditions and harvesting efficiency (Chong et al. 2017). When a 12 year time series data of yield and vegetation indices were analyzed using Ratio Vegetation Index (RVI) model, a good correlation of estimated and observed yield was obtained (Figure 6), indicating that high yielding trees have dense, robust and healthy canopies based on the positive correlation between vegetation indices value, Leaf Area Index (LAI) and yield (Chong et al. 2017; Balasundram, Memarian, and Khosla 2013).
Figure 6. RVI model used to estimate yield using vegetation indices derived from QuickBird satellite image
Figure 7. Essential processes of paddy cultivation
Precision agriculture in Malaysian paddy industry
Technology uptake and utilization of technology in the form of mechanization, modern varieties and farm management tools among paddy farmers was found to be at a moderate level, where the highest technology use was found in fertilizing, ploughing and seeding (Harun et al. 2015). The potential of implementing PA practices is observed to be more viable in granary areas, where it is under the management of large corporations or cooperatives.
PA elements in Figure 1 can be applied within the context of paddy farming, in which, geospatial variabilities in each process are monitored and managed. Figure 7 depicts the five main processes involved in paddy cultivation in Malaysia. PA in water management for paddy cultivation aims to find a balanced compromise of various factors such as supporting canal systems, hydraulic units, rice field conditions, field off-take, inflow and farming practices that will provide site-specific irrigation at the right amount and at the right time. By combining monitoring of hydrological, climatological and administrative parameters that are tagged to geospatial locations, a GIS-based water management model is capable of monitoring and scheduling daily crop water requirement. A GIS user-interface technique linked with water management model developed by Rowshon and Amin (2010) is capable of assisting and improving decision making process in water management (Rowshon and Amin 2010). Figure 8 illustrates the computed amount of water required (RWS) based on parameters such as Irrigation Requirement (IR), Rainfall (RF), Effective Rainfall (ER), Drainage Requirement (DR) and Total Water Depth (TWD = IR + ER) for specific locations within the observed grid.
Figure 8. GIS based computer model to compute daily spatial water requirement
In land preparation, understanding the topographical features of the soil is essential to ensure good agronomic, soil and crop management practice can be carried out in the subsequent processes. Ideally, a flat and uniformly levelled land will ensure uniform crop establishment and provide adequate protection from competing weed growth when the fields are flooded. In PA, a GIS-based land survey is carried out to map out land level features and to obtain the land level index to be used in the seeding map. Based on the methods to calculate levelling index developed by Agarwal and Goel (1981), each 10 x 10m grid are designated with either ‘even’, ‘high’ or ‘low’ in the seeding map. Subsequently, seeding is done based on the land levels observed by the model, where 10 kg is added to the 120kg/ha seeding rate for grids that are designated with ‘low’ (Figure 8). When this levelling system is used in tandem with this site-specific seed application or variable rate (VRT) seed application method, it was found to produce more consistent crop establishment compared to conventional broadcast seeding (Abu Bakar et al. 2017).
Conventional soil nutrient classification requires an assumption that a sampling point represents the status of the specified area and variabilities within it are randomly distributed. PA aids soil preparation process by eliminating the need of tedious manual sampling, as well as intensive and time-consuming laboratory analysis. Quantification of site-specific nutrient status of soil will allow nutrient application to be done accurately. This can be done on a macro level where aerial images are made to map out plantation areas and subsequent sampling sites are identified and analyzed with geostatistical tools to obtain a nutrient distribution model (Amin and Wayayok 2003; Aishah et al. 2010). It can also be done on site with sensors that work in tandem with GPS enabled mapping system that will be fed into the nutrient distribution model (Aimrun et al. 2007; Aliah Baharom et al. 2015).
VRT in fertilizer application offers the capability of providing nutrients specifically to the plant’s requirement. Conventionally, this is done on the field by blanket spreading without discrimination and reference to the plant’s condition, as well as available soil nutrient content. This eventually will result in uneven application of fertilizer, either under supplying required nutrients to the plants that will adversely affect plant growth, or oversupplying it, causing increased input cost and harmful environmental effects. In relation to nutrient classification process, MARDI’s VRT Fertilizer system based on Green Area Index (GAI) model, enables specific rate of fertilizer application to be made based on soil fertility and plant condition. This system includes a GIS-based mapping system that is processed to obtain Soil Plant Analysis Development (SPAD) and GAI maps, that will be used to project a GAI treatment map (Figures 9 and 10). This map will be stored in an online server and used by farmers to apply fertilizers accordingly. This data is then compiled into a geospatial and temporal representation of soil fertility for subsequent planting seasons. This system was observed to save 17% of total fertilization cost with an increase of about 20% efficiency over conventional fertilization methods.
Way forward for PA in Malaysian oil palm and paddy industries
Jalani et al. (2002) posited that to increase production yield, issues such as inadequate agronomic inputs, shortage of skilled labor, as well as ineffective and inadequate field maintenance must be overcome. PF technologies and its application in agriculture continue to push boundaries and are capable of addressing these issues. PA technologies allow farms to increase their productivity and economics, thus translating into higher yields with lower production cost, compared to conventional practices (Balafoutis et al. 2017). Progress can be made in the form of more accurate and precise sensors to identify and monitor parameters, higher resolution and capable cameras that offer more accuracy across the spectrum, as well as more robust and dynamic algorithms to power Decision Support Systems (DSS) for it to better understand vagaries of nature and crop requirements. For the oil palm industry, PA also offers an opportunity to overcome its biggest challenge to date, where public opinion of it supposed detrimental effect on the environment continues to adversely affect palm oil and its derivative market prices. PA technologies such as VRT for fertilizers, seed and irrigation were found to contribute to the reduction of GHG emissions (Balafoutis et al. 2017). In addition, more accurate and sustainable plan of expansion and management of palm oil plantations can be carried out more efficiently, by taking into account of greenhouse gas (GHG) contribution and land use change effects.
Figure 9. MARDI’s GIS-based land levelling and seed VRT system
Figure 10. MARDI' s VRT fertilization system
Yield and productivity challenges seen in palm oil can also be applied in Malaysia’s paddy industry. Sustainability and lowering of GHG emissions in the paddy production system continues to rank high in national agendas. High tech equipment used in PA can provide site specific applications and deliver accurate inputs depending on the spatial and temporal requirements, thus lowering its GHG emissions. Here, a robust DSS that compiles information and facilities from all related agencies will aid farmers to better manage their farms. A dynamic system such as those developed by Norasma et al. (2013) can be upscaled and improved using mobile applications or in an autonomous farming system. Despite the promise, technology adoption continues to be challenging not only because of the high cost factor, but also due to farmers recalcitrant willingness to change or adapt to new techniques (Harun et al. 2015). National policies can aid reducing or spreading burden of high cost; however, PA innovations must continue to achieve higher accuracy and efficiency to warrant its higher cost investments.
CONCLUSION
The importance of the agriculture sector to Malaysia’s aspirations necessitates implementation of PA technologies to overcome market challenges, increase productivity, achieving food security, as well as also ensuring national development is done sustainably. Existing PA technologies are providing promising results when compared to conventional practices and emphasis on sustainable agriculture in national agendas will propel more research on PA technologies in order for it to be more accurate, efficient and feasible.
REFERENCES
Abu Bakar, B, M. A. M. Bookeri, S. S. Mohamad Ghazali, M. S. Abd. Rahman, M. T. Ahmad, and R. Abdullah, M. Z. K. Ismail. 2017. “Automated Land Leveling Index Determination for Variable Rate Seeding of Paddy.” In Proceedings of International Conference on Sustainable Development ICoSD 2017. Putrajaya, Malaysia.
Agarwal, M C, and A C Goel. 1981. “Effect of Field Levelling Quality on Irrigation.” Agric Water Manag 4: 457–64.
Ahmad, Tengku Ariff Tengku, and Chubashini Suntharalingam. 2009. “Transformation and Economic Growth of the Malaysian Agricultural Sector.” Economic and Technology Management Review 4: 1–10.
Aimrun, W, M S M Amin, Desa Ahmad, M M Hanafi, and C S Chan. 2007. “Spatial Variability of Bulk Soil Electrical Conductivity in a Malaysian Paddy Field: Key to Soil Management.” Paddy and Water Environment 5 (2): 113–21. https://doi.org/10.1007/s10333-007-0072-z.
Aishah, A W, S Zauyah, A R Anuar, and C I Fauziah. 2010. “Spatial Variability of Selected Chemical Characteristics of Paddy Soils in Sawah Sempadan , Selangor , Malaysia” 14: 27–39.
Aliah Baharom, Siti Noor, Sakae Shibusawa, Masakazu Kodaira, and Ryuhei Kanda. 2015. “Multiple-Depth Mapping of Soil Properties Using a Visible and near Infrared Real-Time Soil Sensor for a Paddy Field.” Engineering in Agriculture, Environment and Food 8 (1): 13–17. https://doi.org/https://doi.org/10.1016/j.eaef.2015.01.002.
Amin, M S M, and Aimrun Wayayok. 2003. “Spatial Variability of Total Nitrogen , and Available Phosphorus of Spatial Variability of Total Nitrogen , and Available Phosphorus of Large Rice Field In,” no. January.
Balafoutis, Athanasios, Bert Beck, Spyros Fountas, Jurgen Vangeyte, Tamme Van Der Wal, Iria Soto, Manuel Gómez-Barbero, Andrew Barnes, and Vera Eory. 2017. “Precision Agriculture Technologies Positively Contributing to Ghg Emissions Mitigation, Farm Productivity and Economics.” Sustainability (Switzerland) 9 (8): 1–28. https://doi.org/10.3390/su9081339.
Balasundram, Siva K, Hadi Memarian, and Raj Khosla. 2013. “Estimating Oil Palm Yields Using Vegetation Indices Derived from QuickBird,” no. January.
Chong, Khai Loong, Kasturi Devi Kanniah, Christine Pohl, and Kian Pang Tan. 2017. “Geo-Spatial Information Science A Review of Remote Sensing Applications for Oil Palm Studies A Review of Remote Sensing Applications for Oil Palm Studies” 20 (2): 184–200. https://doi.org/10.1080/10095020.2017.1337317.
DOS, DEPARTMENT OF STATISTICS MALAYSIA. 2018. “Malaysia Selected Agricultural Indicators.” 2018. https://www.dosm.gov.my/v1/index.php?r=column/cthemeByCat&cat=72&bul_id=....
EPU, Economic Planning Unit. 2017. “Malaysia Sustainable Development Goals Voluntary National Review 2017.”
Gollin, Douglas. 2010. “Chapter 73 Agricultural Productivity and Economic Growth.” Handbook of Agricultural Economics 4 (January): 3825–66. https://doi.org/10.1016/S1574-0072(09)04073-0.
Griffiths, William, Thomas Fairhurst, Ian Rankine, Armin Gfroerer Kerstan, Vince Mcaleer, and Clive Taylor. 2003. “A Conceptual Framework for Precision Agriculture in Oil Palm.”
Harun, Rosnani, Syahrin Suhaimee, Mohd Zaffrie, Mohd Amin, and Nurul Huda Sulaiman. 2015. “Benchmarking and Prospecting of Technological Practices in Rice Production” M: 77–88.
Ibharim, N A, M A Mustapha, T Lihan, and A G Mazlan. 2015. “Mapping Mangrove Changes in the Matang Mangrove Forest Using Multi Temporal Satellite Imageries.” Ocean & Coastal Management 114: 64–76. https://doi.org/https://doi.org/10.1016/j.ocecoaman.2015.06.005.
Izzuddin, M. A., M. N. Nisfariza, B. Ezzati, A. S. Idris, M. D. Steven, and D. Boyd. 2018. “Analysis of Airborne Hyperspectral Image Using Vegetation Indices, Red Edge Position and Continuum Removal for Detection of Ganoderma Disease in Oil Palm.” Journal of Oil Palm Research 30 (3): 416–28. https://doi.org/10.21894/jopr.2018.0037.
Jalani, BS, Yusof Basiron, Ariffin Darus, KW Chan, and N Rajanaidu. 2002. “Prospects ofElevating National Palm Productivity:A Malaysian Perspective.” Oil Palm Industry Economic Journal 2 (2): 1–9.
Liaghat, S., and S.K. Balasundram. 2010. “A Review: The Role of Remote Sensing in Precision Agriculture.” American Journal OfAgricultural and Biological Sciences 5 (1): 50–55.
Mohamed, Zainalabidin, Rika Terano, Mad Nasir Shamsudin, and Ismail Abd Latif. 2016. “Paddy Farmers ’ Sustainability Practices in Granary.” https://doi.org/10.3390/resources5020017.
Nooni, I K, A A Duker, I Van Duren, L Addae-Wireko, and E M Osei Jnr. 2014. “Support Vector Machine to Map Oil Palm in a Heterogeneous Environment.” International Journal of Remote Sensing 35 (13): 4778–94. https://doi.org/10.1080/01431161.2014.930201.
Norasma, C Y N, A R M Shariff, E Jahanshiri, M S M Amin, and A R Mahmud. 2013. “SCIENCE & TECHNOLOGY Web-Based Decision Support System for Paddy Planting Management” 21 (2).
Nordin, Khairani Afifi. 2018. “Agriculture: Addressing Food Security in Malaysia.” The Edge Markets, 2018. https://www.theedgemarkets.com/article/agriculture-addressing-food-secur....
Razi, Mohamad, Mohd Hasmadi Ismail, and Helmi Shafri. 2013. “Fusion Technique of LiDAR and Airphoto Imagery for Oil Palm Classification.” 34th Asian Conference on Remote Sensing 2013, ACRS 2013 2 (January): 1317–25.
Rowshon, MK, and MSM Amin. 2010. “GIS-Based Irrigation Water Management for Precision Farming of Rice.” Int J Agric & Biol Eng Open 3 (3): 27–35. https://doi.org/10.3965/j.issn.1934-6344.2010.01.027-035.
Schwarz, J, L Herold, and B Pölling. 2011. “Typology of PF Technologies; FP7 Project Future Farm.” http://www.futurefarm.eu/.
Shafri, Helmi, Mohd Hasmadi Ismail, Mohamad Razi, Izzuddin Anuar, and Abdul Rahman Ahmad. 2012. “Application of LiDAR and Optical Data for Oil Palm Plantation Management in Malaysia.” Proc SPIE 8526 (November): 8. https://doi.org/10.1117/12.979631.
Shafri, Helmi Z.M., Mohd I. Anuar, Idris A. Seman, and Nisfariza M. Noor. 2011a. “Spectral Discrimination of Healthy and Ganoderma-Infected Oil Palms from Hyperspectral Data.” International Journal of Remote Sensing 32 (22): 7111–29. https://doi.org/10.1080/01431161.2010.519003.
Shafri, Helmi Z M, Mohd I Anuar, Idris A Seman, and Nisfariza M Noor. 2011b. “Spectral Discrimination of Healthy and Ganoderma-Infected Oil Palms from Hyperspectral Data.” International Journal of Remote Sensing 32 (22): 7111–29. https://doi.org/10.1080/01431161.2010.519003.
Teng, K C, J Y Koay, S H Tey, K S Lim, H T Ewe, and H T Chuah. 2015. “A Dense Medium Microwave Backscattering Model for the Remote Sensing of Oil Palm.” IEEE Transactions on Geoscience and Remote Sensing 53 (6): 3250–59. https://doi.org/10.1109/TGRS.2014.2372796.
Date submitted: August 5~9, 2019
Reviewed, edited and uploaded: August 15, 2019