ABSTRACT
This study aims to review the approaches and policies on smart farming for small-scale farming in Thailand. The opportunities and challenges of smart farming as well as initiatives policies are also discussed. Under the challenges in many aspects on the agricultural sector, such as declining of average farm size, an increasing number of aging farmers, labor shortage in the sector, while the demand of global food is increasing, thus, initiatives of smart farming system for small farmers will inevitably be cascaded to farmers in the future. Thus, raising the awareness of technology acceptance by farmers and also the cooperation on working on researches, invention and development of smart farming technologies that are suitable for farmers from all related agencies are needed. However, by concerning the constraint of smart farming that would arise from technical unemployment and the knock-on impact on rural populations, government also needs a long-term plan to deal with the problem of unemployment that may occur as a result of the decrease in both working hours and labors. Moreover, to take smart farming into practice, the government should support smart farmers who settle throughout the country and have a strong network for conducting pilot systems and contributing the lessons to other farms.
Keywords: small-scale farming, smart farming, IoT platform, IoT devices
INTRODUCTION
Agriculture plays a crucial role in economic growth and development of Thailand as it is comprised of production resources, and raw material of continuous manufacturing by both processing and product industry. Agriculture also creates employment and income of most population in Thailand. The areas over 23.88 million hectares of the country which accounted for 46.54 % of total areas are used for agricultural activities in planting, livestock and fisheries. For decades, most farmers get used to do traditional farming by following the approach of their parents and ancestors for a long time. This implies that they rarely take an advantage of technology, digital innovation and information for farming.
The agriculture sector in Thailand is facing many challenges, both from structural factors and external changes that directly affect productivity and competitiveness of farmers in the country.
A decrease in the average size of small-scale farms is one of agriculture’s concerns. Half of the total land (46.54%), or 23.88 million hectares, is used for agriculture, and the vast majority of agricultural production in Thailand occurs on small-scale farms (Office of Agricultural Economics, 2016). Family-run farms have an average farm size of 4.04 hectares. Even though this farm size is larger than many other Asian countries such as Japan, China, India, South Korea (Fan and Chan-Kang, 2005), Bangladesh, Indonesia, and the Philippines (Otsuka et al., 2016), however, in common with most of these Asian countries (except Japan and South Korea), Thailand has been experiencing a decline in average farm size over the past number of years. From 1993 to 2016, the annual average farm size per household decreased by 0.66% across the country. This phenomenon of gradual decline in average farm size has been found in every region of Thailand.
There are 5.9 million farms with an average farm size of 4.04 hectares (or 25.26 rais). Moreover, of the 5.9 million total farm households, 26.5% of farm households had less than 1.6 hectares (or 10 rais, the smallest size of land) in 2012. And almost half of farms (43.6%) had land areas from 1.6 to < 4.8 hectares (or 10 to < 30 rais). Additionally, the share of farm holdings of the land less than 1.6 hectares or of the smallest size increased from 22.5% in 1996 to 26.5% in 2012. But in the same period, the share of large-sized farms over 8 hectares (i.e., 50 rai) decreased from 11.4% to 8.8% (Bureau of Agricultural Economics Research, 2015). This evidence showed the increasing of the share of farm households holding the smallest-sized farms (less than 1.6 hectares) (Kwanmuang, 2018).
There are many factors that influenced this declining average size, including industrialization, urbanization, institutionalization, land policy, property rights, and land and labor markets (Fan and Chan-Kang, 2005). In Thailand’s case, in addition to these factors, the custom of dividing the land for inheritance led to the reduction in farm size for the next generation. Whether the household has a successor or not, farm size per child was not significantly different between the households (Kwanmuang, 2015; Kwanmuang, 2018). The findings increased awareness of concerns, especially for successors who will inherit small farms. In other words, in the agriculture sector, the average scale of farming will decrease. This phenomenon may increase the unbalanced growth between agriculture and non-agriculture lands and increases more concerns regarding the sustainability of agriculture. Thus, the program for supporting and enhancing ability for small-scale farms is needed in the future (Kwanmuang, 2018).
Although many studies revealed that small farms were more productive than larger ones due to the advantages of small farms using farm labor or labor-intensive processes (Fan S., et al., 2005), including the reverse relationship between farm size and productivity still exists in Thailand (Wattanutchariya and Jitsanguan, 1992; Bureau of Agricultural Economic Research, 1997, 2007, and 2015), they may be affected by increasing labor wages. Thus, small farm sizes may lose their competitive advantages due to the decline of small farm production efficiency relative to large ones (Otsuka et al., 2016).
Moreover, the future concern is that the average age of the head of the farm household was 56 years (Office of Agricultural Economics, 2016). Almost half of farm households are aging farmers who lack access to knowledge and new technologies. The aging problem will become serious and affect the productivity of farms in the next 10 years (Logindarat, 2011). Meanwhile, the decline in the number of younger people who want to work on farms (Poapongsakorn et al., 1998) raises concerns about the success to farms and the agricultural sectors in the near future.
Furthermore, another concern is the lack of farm labor. The number of agricultural laborers has shown a gradual decline in every region. From 1998 to 2014, the farm labor force decreased from 22.80 million to 17.78 million, decreasing by an annual rate of 1.18% (Office of Agricultural Economics, 2015), and this trend occurred across all regions.
Additionally, most farmers are owners of small farms and have constraints in terms of economies of scales for getting into resources and technologies. There is also lack of bargaining power in the market that has a long supply chain as well as the implementation of agricultural policies that may not be effective and widespread to farmers. The impact of aforementioned challenges together with climate change and higher competitiveness of global market all affect most of farmers to have low productivity and profit, and heavily depend on the government policy supports (Attavanich et al., 2019).
With these challenges, the government focuses on the development of agricultural sector and aims that agriculture, farmers and farmer institutions need to have security, prosperity and sustainability within the next 20 years by driving relevant projects through the 20-year national strategic plan (2017 to 2036) and 20-year Agriculture and Cooperatives Strategy (2017-2036) and the agricultural development Plan in the 12th national economic and social development plan (2017-2021). These three plans are related and intend to transform traditional agriculture into smart agriculture especially as changes of consumer behavior affected by the impact of COVID-19 leading to the new normal, small farmers will have to change and inevitably shift and do smart agriculture.
Hence, this research aims to mainly review the approaches and policies on smart farming for small-scale farming in Thailand in order to understand the state of development of the system which is one of the ways to solve agricultural problems in the future.
LITERATURE REVIEW: SMART FARMING AS WORLDWILD PERSPECTIVE
Definitions of smart farming
There is no single definition of smart farming, one is the integration of data and communication technology into agricultural production system. Another defines Smart farming as the use of information transfer and concentration in remote storage systems (servers), to allow different farm data to be combined and evaluated for decision-making. Beecham defines Smart farming as a software engineering and computer science concept that came about by adding computer techniques and by transmitting data from agriculture in a virtual environment (Beecham research, 2014). Farm Management Information System (FMIS), is one of the first academic definitions of smart farming. FMIS is defined as a system for gathering, sorting, preserving, and disseminating data in an acceptable format for the success of agricultural land activities and functions (Sorensen et al., 2010).
Due to technology advancement, two factors make Smart farming possible: data collection and data storage and analysis. Data collection is guaranteed by using sensors in agriculture. A sensor is an electrotechnical system that detects environmental and physical quantities (temperature, humidity, light, pressure, presence or absence of certain types of artifacts, mechanical stress levels, and object size) then transforms them into a signal that can be interpreted and collected as data (Lehmann et al., 2012).
Likewise, the Internet of Things (IoT) is a smart farming-related invention. IoT shares with FMIS the idea of an intelligent environment (Gubbi et al., 2013). The Internet of Things enables objects to be remotely operated over existing network infrastructure. However, the use of IoT relies on the internet infrastructure and this poses a variety of weaknesses, in particular when a vast number of devices are connected and when combined with other systems (Kaloxylos A et al., 2012).
Smart farming instruments implement a new level of agricultural technologies, including automation, mapping and geomatics, decision-making, and mathematical processes. The most promising smart farming developments include advancements in sensors, data processing, telemetry, and positioning technologies, but it may take time and effort to evolve and disseminate these technologies.
Opportunities of smart farming
Schumpeter who focused on the relation between innovation and economic growth mentioned that technical innovation modifies manufacturing habits and can distinguish between regions and countries’ economic development (Schumpeter and Garcia, 1983). Likewise, Freeman and Perez, introduced the concept of a techno-economic pattern as a way of explaining how technology and innovation are progressing. They stated that interruption periods of technological standards produce new products and approaches, leading to important behavioral changes (Freeman and Perez, 1988). As a result of introducing mechanization and chemistry, deep shifts have arisen in the agriculture industry, which leads to the usage of the Internet of Things, in smart environments, and the use of cloud computing.
The previous innovation-economic growth relation should be investigated, to do so, development organizations (R&D) are required to change the techno-economic context (Penrose, 2009). R&D investment is needed, as there are degrees of accumulation of technologies and advanced development processes when comparing different regions and countries (Cohen and Levinthal, 1999). According to the World Bank, R&D investment was concentrated in 2013 for both public and private in some countries, including South Korea (4.15 % of the gross domestic product), Japan (3.47 %), Denmark (3.60 %), Germany (2.85 %), and the USA (2.81 %) (World Bank, 2017).
The economic potential of smart technologies applied to agricultural production is clearly understood by companies and governments. As an example, Cropio is a satellite field management system developed by New Science Developments New York in 2013 (Verónica Saiz-Rubio et al, 2020). And purchased by Syngenta in 2019, the first agricultural firm to have access to leading management platforms in the top four agricultural markets, USA, China, Brazil, and Eastern Europe. These 4 countries market combined 98 million acres and it will be handled internationally using the Syngenta Automated Platform, to double by the end of 2020 (Agriculture and commodity trade chamber, 2019).
As a platform for crop management, health, and vegetation control, Cropio allows its users to plan and carry out agricultural operations effectively. It offers real-time updates on current field and crop conditions, describes vegetation levels, highlights problem areas, and provides accurate weather forecasts and an individual commodity market report.
The key purpose of Cropio and its functions is to maximize the use of resources and improve the efficiency of the farming sector. Besides, Cropio expands its cloud solutions to various vendors to gather all data required for the farmer in a single location and in a convenient way. Farmers do not need to download new programs and data is converted from one device to another or from one format to another.
The advantages of these emerging ICT technologies for agriculture are diverse: farmers are offered with improved production and benefits (Bronson and Knezevic, 2016; Ge and Bogaardt, 2015), while these technologies will help solve concerns such as food security and sustainability at the social level (Carolan, 2016). At the same time, the transformative nature of digitization means that social disturbance is expected; it can affect economies, corporate structures, and supply chains, agriculture and rural societies, ownership, and privacy. Such impacts will be beneficial, but there is also the possibility of surprising harmful results when emerging innovations are incorporated into society (Bronson, 2018).
Challenges of smart farming
Fleming has pointed out possible tensions or concerns about the digital divide and unequal development between different farm sizes (e.g. small and large), territories (e.g. urban and rural), and persons (e.g. age, socio-economic or educational disparities) (Fleming et al., 2018). Likewise, Bronson has raised awareness about technical unemployment and the knock-on impact on rural populations (Bronson and Knezevic, 2016). While, there are undeniable benefits beyond economic gains associated with smart farming advances (for example environmental gains by decreased input usage), there are potentially negative effects, especially socio-ethical consequences for humans and non-humans (Wolfert et al., 2017; Carbonell, 2016; Driessen and Heutinck, 2015; Millar, 2000). To date, these possible effects of smart farming have been investigated by just a few reports. However, it is important to draw implications from the use of intelligent inspiration in other fields. In this sense, the World Economic Forum expects a wide-scale “technological unemployment” rising from the application of automation in agriculture. This was possible by extrapolating the automotive industry mechanization to the automation of agriculture (World Economic Forum, 2016). In many communities, shifts in rural workplaces could have a significant effect on social stability and the livelihoods of many workers (Carolan, 2016). In Australia for example, analyses of the application of smart technologies to the dairy industry show a reshaping of farming activity, with less labor force and more data-driven choices (Eastwood et al., 2013).
Ethical problems (such as animal abuse, unemployment-economic benefit relation) would raise challenges in deciding which data to collect to accomplish social objectives, and how much access to socially relevant data sets to be allowed. Indeed, the Global Open Data for Agriculture and Nutrition (GODAN) initiative of the United Nations, calls for an open access to agricultural data as a constitutional right, they claim that it would lead to solve serious humanitarian problems of global food insecurity. The majority of literature on innovation and community indicates that GODAN will profit both advantages and costs, regardless of what we know about smart farming in particular (Beck, 1992). However, besides the increased efficiency and favorable environment, smart farming could have negative consequences, too. The socio-ethical consequences have been explored by several scholars. It has been proposed that smart farming, with less ‘hands-on’ management and a more data-driven approach, would reshape farming practices (Eastwood et al., 2013).
To enable and integrate smart farming technologies, a range of skills and advisory structures would be required around the farming team, in order to relocate farm labor force and service providers. By integrating detailed monitoring, agricultural machinery manufacturers, input vendors, processors, and retailers, several changes can have a significant impact on what it means to be a farmer, since a more formal and assessed approach in smart farming will replace the old concept of “your land, your way” (Bronson and Knezevic, 2016; Carolan, 2016).
Challenges of smart farming in Thailand
The agriculture sector in Thailand are challenged in many aspects. As population ages, labor shortage in the agriculture sector tends to rise from the past, while the demand of global food is increasing. In the past, farmers always faced the problem of price falling while the production cost was high and the weather was unpredictable. In addition, farmers also faced the lack of capital access and agricultural technology as well as the inconsistent implementation of government policies and concentrated on the short-run (Pattama, 2020). This shows the urgent need for improvement of agriculture sector to help farmers increase their productivity, reduce the costs of production and improve the living standards.
Although smart farming is considered as one of the management approaches that help increase productivity and economic returns while reducing the environmental impact, the implementation of Thai farms is uncertain. In Thailand, smart farming which precisely managed is considered as a new thing for small-scale farmers because there are constraints in terms of the technological readiness to apply technologies and innovations in their farms accurately and properly. The significant factors that have hindered the adoption of new technologies and innovations in smart farming are technological, economic and social (Manwicha, 2015).
To adopt technologies in smart farming, farmers needto have the ability to get access and exploit the benefit of the advent of technologies and innovations to make use of data for planning and managing their farms (Pattama ,2020). A research conducted by Kuhasak and Srichampa (2018) on management pattern for smart farming business indicated that entrepreneurs needed to learn, understand, and use technologies that was suitable and useful for their farm to get the proper management pattern of smart agriculture for small and medium enterprise (SMEs), and to solve problems of uncontrolled pests and diseases in farms. A way for training technology is by educating farmers to understand what the technology was and how it could be beneficial to them. As a result, policymakers should provide resources for learning or develop programs that suit for farmers to find those resources (Duang-Ek-Anong et al., 2019). Government also launched the project that encourages young farmers to be “Smart farmers”. This project intends to develop young farmers to learn to manage their farms by using technologies and innovations so that they can become a self-reliant entrepreneurs and local agricultural leaders in their regions (Yungyuen, 2020). The study of Kraipinit (2018) on development program for smart farmers of agribusiness students also supported that people who attended the program had higher skills for doing smart agriculture. This program developed from the idea of cognitive thinking approach consisting of stimulation, thinking, perception, and memorizing. The results showed that the important skills for smart farmers were thought leadership and empowerment skills. The knowledge gained from this agricultural model could be used for developing and building supply chain of farming and used as a guideline to improve skills of smart farming as well.
The investment on new technology is one of the challenges for the adopting of smart farming. In order to use technologies and innovations, the investment is needed to plan and build new farming systems that are suitable for their farms. As farmers in Thailand are normally small-scale farmers, changing to do smart farming required a lot of money and the return on investment is uncertain for small farms. Additionally, as agricultural process takes many steps and different methods, technologies from different farms could have different standards and difficult to work together effectively. This may also increase the cost of investments, therefore, farmers are not affordable to those smart farming technologies (ARDA, 2020). To support smart farming, Thailand Board of investment (BOI) offers promotion investment scheme which provides tax exemption for the smart farming investors. However, if Thailand can create and develop technologies in smart farming with lower cost, smart farming would be widely used.
THAILAND POLICY ON SMART FARMING
Smart farming technology in Thailand
Smart farming technology is a new agricultural innovation in the digital era that adopted various information and communication technologies in farms such as GPS, GIS, remote-sensing, proximal-sensing, variable rate technology (VRT) and decision support system (DSS) through the wireless network for receiving, sending, and evaluating by package software. The processes for doing smart farming consist of data collection, data diagnosis, data analysis, implementation of the plan, and evaluation. These are operated with farm management in every step by mapping of soil conditions, watering, fertilization, pest control, harvesting, cost-profit calculation, and planning for the next planting season. As a result, smart farming leads to the efficient use of production factors, the increase in quality of farm production, cost-effectiveness, reducing environmental impact, cost of production, and labor, saving time and leading to sustainable agriculture (Manwicha, 2015)
In response to the development of Thai agriculture, the Ministry of Agriculture and Cooperatives (MOAC) intends to transform traditional farming into “Smart Farming” by using advanced information technology in the production of crops, livestock and fisheries as well as using the intelligent sensor systems to monitor and evaluate their production in real-time.
Smart farming is considered as one of the important strategic goals in Thailand which aims to enhance Thailand’s potential by focusing on the increase in production both in terms of quality and quantity by applying new technologies and innovations in the production process, while decreasing a number of labors and cost of production. Smart farming combines environmental sensor networks and artificial intelligence – based analysis such as digital technology, biotechnology, modern agricultural machines, Internet of Things and intelligent sensor systems in assisting, planting, and production. To implement smart farming in Thailand, MOAC prepares agricultural field demonstration of smart farming which enables farmers to gain knowledge and improve skills from doing smart farming and be ready for agricultural transformation of Thailand in the future (MOAC, 2019).
Smart agricultural development of the Ministry of Agriculture and Cooperatives
With regard to the Thailand 4.0 policy, Thailand’s 20-Year National Strategy Plan, the Ministry of Agriculture and Cooperatives (MOAC) intends to transform traditional farming into smart farming or Agriculture 4.0 by using technologies and innovations. The MOAC appoints the smart farming-driven committee which are responsible for imposing plans and policies of smart farming, and driving smart agricultural development of MOAC through the process of agricultural field demonstration of smart farming, development in Big Data platform, Artificial Intelligence (AI), robotics and machine development, agricultural research and development of remote sensing and the potential development of agricultural manpower. The details and progress of smart farming are as follows.
Agricultural field demonstration of smart farming
The smart farming-driven committee has cooperated with domestic and foreign organizations to make use of technologies for trails in the agricultural field. These field demonstrations are tested with various crops such as rice, sugarcane, maize, cassava, pineapple, and tomato by using technologies applied in the field demonstration such as unmanned aerial vehicle (UAV), multispectral camera technology, auto steering, laser land leveler, weather station, autonomous water monitoring system, and autonomous harvesting system. Agricultural field demonstration also records and collects data for linking to Big Data platform. This data can be used as a tool for decision making and set guidelines for the future work plan of smart agriculture.
Smart farming Big Data Platform
The smart farming-driven committee attempted to make use of data obtained from the application of smart farming technology to help support planning and decision making at the war room of the MOAC. The committee also cooperated with other organizations in order to link data both inside and outside of the MOAC to agricultural Big Data platform for analyzing and monitoring plant growth such as data of crop situation from satellite images, weather detection, and weather forecast. The work plan includes two parts which are the estimation of production and pest monitoring, both of which are the key factors for balancing the supply of production with the market demand and decreasing production cost. Big data platform is used for estimating and planning supply of production in order to deal with market conditions which affect crop prices. In the farm level, the MOAC develops crop monitoring system by taking advantages of sensor technology to collect data from the field for analyzing, and monitoring crop situation in farms. This data can help predict crop situation and control damages in farms. In the country level, the MOAC uses data from the survey of farmers’ field, satellite images, and data of weather and natural disasters to manage crop production to the market. Additionally, the MOAC has developed a prototype of pest recognition that collected data via web application to manage and prevent damages occurred to farm and productions effectively.
IMPLEMENTATION OF SMART FARMING FOR SMALL-SCALE FARMING IN THAILAND
Transforming the agriculture sector to smart agriculture is very challenging because most of Thai farmers are aged, operating in small-scale basis and lack of skills and resources. In the past, smart agriculture technologies were adopted by mostly large-scale farmers due to economies of scale and financial viability. As the advance of information and digital technology, the cost of developing and implementation of smart farming technologies become more affordable. In addition, smart farming technologies are extended to small-scale farming system. This section provides an overview on the current situation of smart farming implementation in Thailand.
Various organizations including government agencies, educational institutions, and private companies have launched projects and initiatives in an attempt to make smart farming technologies available to small farmers. The Ministry of Agricultural and Cooperatives has placed high priority on smart farming. The ministry promotes smart farming technologies through field demonstrations.
In 2019, field demonstrations were focused on six crops: paddy, sugarcane, maize, cassava and pineapple and tomatoes (Ministry of Agriculture and Cooperatives, 2019). National Science and Technology Development Agency (NSTDA), a government research center, developed a smart agricultural system for greenhouse called “Handy Sense” which is able to monitor key environmental variables including light intensity, soil moisture and temperature, and air humidity and temperature in real time and also able to control i.e. irrigation (NECTEC, 2020a). A research conducted by Phonpho and Phonpho (2013) has studied the development of automatic nursery seeding machine which is controlled by a programmable logic controller. The results showed that seeding machine were highly effective for sowing up to 91.98 % of needle head number 18 and able to sow seeds into seedling tray for 94.7 trays per hour which saved time than using human labor for 3.45 times. In addition, Samseemoung and Sangpradit (2014) designed and fabricated a high-performance vegetable seeder of Brassica alboglabra for seeding trays. This machine was invented to meet farmers’ need for replacing human labor, reducing seed loss, saving time, and decreasing human fatigue for seeding.
Not only public sectors, private companies ranging from telecommunication companies, agricultural machinery manufacturers, agricultural input suppliers and agri-tech startups also put serious efforts on smart farming technologies. Total Access Communication (DTAC), a big telecommunication company, joined with Samnuek Rak Ban Kerd, a social enterprise and Ricult, an agritech startup, to develop a service named “Farm Man Yum”. The service use Artificial Intelligence (AI) to provide weather forecast (temperature, probability of precipitation, and rainfall amount) at specific location, provide a satellite image for monitoring crop health and give advice, tips and knowledge from planting to harvest. In addition, DTAC, the Department of Agricultural Extension and National Electronics and Computer Technology Center (NECTEC) collaborated and developed a greenhouse IoT farming platform. A greenhouse is equipped with sensors to measure air, soil and light condition where farmer can monitor via smartphone or smart device in real time (DTAC, n.d.). Similar IoT platforms are developed by other enterprises for example Intelligence Farm or i-Farm platform developed by Advanced Info Service (AIS) (Sentangsedtee, 2019) or an IoT platform for vegetable greenhouses developed by CAT Telecom (CAT) (CAT, 2019). The concept of internet of things was also applied on the design of smart farming in lime farms in Phetchaburi Province to increase lime production efficiency by using a sensor to measure data of four parameters which are temperature, humidity, moisture and pH. This design by connecting IoT devices (Node MCU) to Arduino board and sensor system as well as the development of application for connecting IoT devices could display the standard values with various mobile applications which supported both in IOS and Android operating system (Sriamnouy et al., 2019).
IoT platform is not only limited to crop production, True Corporation (TRUE) joined with Khon Kaen University, and Charoen Pokphand Foods (CPF) to apply the 5G technology to smart agriculture. The plan involves subprojects, for example, to apply CCTV cameras and sensors to get information and to use AI technology to analyze and monitor chicken health. (The Reporter Asia, 2020)
Agricultural machinery manufactures also developed a new generation of agricultural machineries incorporating IoT and robotics technology enabling agricultural machineries to send data from the field while it can be controlled from distance, for example (Yanmar, n.d). Beside IoT machinery, some manufactures also offer a digital solution service such as KUBOTA Agri Solutions (KAS) by Siam Kubota (Siam Kubota Corporation, n.d.)
SUCCESSFUL CASE OF IMPLEMENTATION
We conducted a field study of successful case of using smart farming system, this farm belongs to Mr. Somboon Ngamsangiam. With cooperation and help from National Science and Technology Development Agency (NSTDA) collaborated with a Thai farmer, Mr. Somboon Ngamsangiam has adopted technology of plant watering control system in the field experiment of durian farm in Rayong Province.
The entering point of using this smart technology started with the experience of durian growers. They knew that water and weather are two important factors for durian cultivation, but they did not notice that what they observed can be measured scientifically. Moreover, in the past, Mr. Somboon recorded revenues and expenses on his farm and he found that the cost of the common water pumping system using diesel fuel for durian trees each year was quite high. The common system for 8 hectares (50 rais) of durian farm consumed approximately 18,667 USD per year (or 2,334 USD per hectares). This is because he did not know whether the soil needed water at that moment or not, resulting in unnecessarily pumping water for durian trees. Moreover, Mr. Somboon attended a meeting which explained and introduced technology of automatic water control system developed by NSTDA. He was interested to apply this technology in his own farm as this technology helped reduce water supply, fertilizer loss, and production damage. It also helped save electrical energy and the investment cost was low due to the use of small water pump.
Thus, in the beginning, Mr. Somboon and NSTDA started the field experiment using technology of automatic water control system that used the sensor to measure soil moisture, air humidity, light intensity and temperature in the field experiment in a durian farm for 0.32 hectares (2 rais).
For this first year of experiment, data on soil moisture in every stage of planting was collected. During the growth stage, the percentage of soil moisture was not below 28, while the percentage of soil moisture in flowering stage was between 28 and 34. For the fruiting stage, the percentage of soil moisture was around 32 to 40. These results were obtained by collecting data of soil moisture that was suitable for each growing stage from the system as well as observing by converting his sense to number. Although the sensor was not stable in the first time, it was developed until now to be acceptable and useful for collecting data to manage farms and plan for the next year cultivation (NSTDA, 2020).
As the result of field experiment is satisfied, Mr. Somboon has invested the automatic water control system in the farm up to 8 hectares (50 rais). By applying the automatic water control system, he significantly decreased the production cost from using common pumping machine using diesel fuel which costed approximately 18,667 USD per year to 8,333 USD per year. This implied that he could save cost of production approximately 10,334 USD per year.
However, in terms of investment in this system, in the first year, Mr. Somboon had to invest in electric pumps and electrical installations which accounted for 23,333 USD. The system also included two sets of agricultural weather stations fully supported by NECTEC. Since it was still in the development stage, the cost must be estimated and it would cost approximately 3,333 USD for two sets. Thus, the total investment costs of automatic water control system were approximately 26,666 USD. In comparison to the cost he could save, this showed that the investment in automatic water control system could reach the breakeven point within three years. Moreover, this system could also be applied to a smaller farm or orchards where one set of equipment including one electrical pump and one weather station could optimally operate 4 hectares (25 rais).
In conclusion, although in the first year he had to invest in technological equipment and machine which accounted for approximately 26,666 USD, it could reach the breakeven point within three years. The parameters of those measurements of this system are used not only for management of durian farm especially the water input according to the needs of durian trees during growth stage, flowering, and fruiting which help farmers to eliminate the problem of overwatering, but also helped reduce the number of labors worked in his farm or reduce labor costs, fertilizer loss, production damage, and control quality of fruit yield, resulting in the increase in farm revenue more than twice (Eastern Economic Corridor (EEC) Office, 2020). However, the barriers of small-scale farmers to apply this system are the lack of capital and knowledge. As a result, Mr. Somboon suggested this system will be efficient if government starts with the large-scale farming (called “ka-set prang yai”) by gathering small-scale farming to collaborate into large-scale farming for reducing the production cost and sharing knowledge with each other.
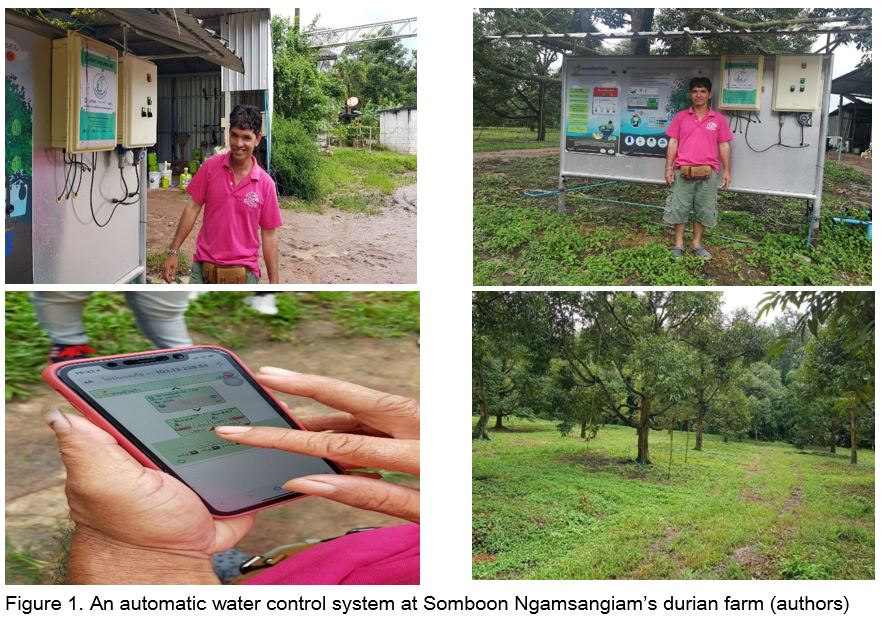
Another example of successful case is the implementation of "Handy Sense" system by NSTDA mentioned earlier in previous section (NECTEC, 2020a). The system uses sensors to monitor important environmental factors in a greenhouse where farmer can access the information via smart devices in real-time. Moreover, the farmer is able to manually control irrigation or pre-defined schedule watering time. The system also provides optimal environmental values for various crops such as melon tomatoes mango rice and hydroponics vegetables. NSTDA promoted the Handy Sense system throughout the country. One example is Mr. Jittakorn Padetsuk, a vegetable grower in Chachoengsao province. He applied the Handy Sense system in one of his greenhouses. Prior to implementation, he watered vegetables using rubber tube and had problems with fungi due to inconsistency. After using the Handy Sense system, he found that productivity increased by 20 percent while he could save water usage by 5-10 percent and reduce labor force (NECTEC, 2020a). Another example that the authors visited in the field is Mr. Surapol Jarupong, a farmer in Chachoengsao province. He installed the Handy Sense system in tomatoes and melon greenhouses where he also found an increase in productivity. The cost of implementing this Handy Sense system was fully funded by local government as demonstration farms. However, it was estimated to cost around 1,667 USD per greenhouse project. This smart greenhouse system like Handy Sense could be more applicable to small-scale farmers i.e. less than 4 hectares (25 rais).
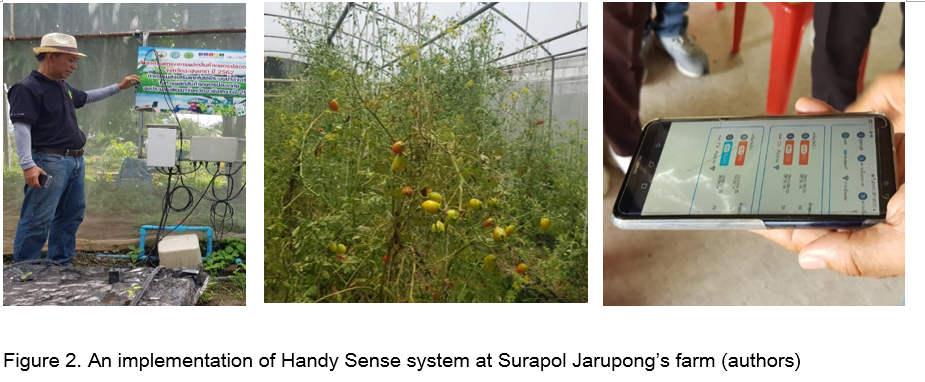
CONCLUSION
The agriculture sector in Thailand is challenged in many aspects. As a declining average farm size and an increasing number of aging farmers, labor shortage in the agricultural sector tends to rise from the past, while the demand for global food is increasing. Small farmers will have to inevitably switch to smart farming in the future.
Smart farming is a new approach to agriculture which helps solve problems and limitations of population age and farm labor shortage. Smart farming does not only have various advantages to farmers but also have constraints when applying or implementing to the large number of small-scale farmers who are facing aging and lack of technical knowledge as well as lack of capital. Thus, raising the awareness of technology acceptance of farmers is needed. The need of cooperation on the researches, invention, and development of smart farming technologies that are suitable for farmers should be supported by all related agencies of the government, private sectors, and educational institutions. Since small-scale farmers have different characteristics in terms of farm resource endowment and skill of farm managers, the transferring and training should be of major concern.
Moreover, to take smart farming into practice, the government should support farms or farmers that have high and top capability as a priority. In Thailand, there are many smart farmers throughout the country that have a strong network for conducting pilot systems and contributing the lesson to other farms. Besides, technology development should be easy to use for farmers with low cost, and suitable for their knowledge and age.
However, smart farming also faces constraints that could arise like technical unemployment and the knock-on impact on rural populations. The government, therefore, also needs a long-term plan to deal with the problem of unemployment that may occur as a result of the decrease both in working hours and labors.
ACKNOWLEDGEMENT
The authors express gratitude to Mr. Somboon Ngamsangiam and Mr. Surapol Jarupong for field visited and precious information and knowledge of example of smart farming and responses to our questions. Every statement expressed in this article is that of the author and does not reflect any opinions of anyone affiliated to the authors.
REFERENCES
Agriculture and commodity trade chamber. 2019. Sygenta buys agricultural technology firm cropio. https://www.actc.life/syngenta-buys-agricultural-technology-firm-cropio/ Access on 23 November 2020.
Agricultural Research Development Agency (Public Organization) (ARDA). 2020. Smart Farming: Success and challenges of the era. [Online]. Available from http://www.arda.or.th/knowledge_detail.php?id=6. [in Thai]
Attavanich, W., Chantarat, S., Chenphuengpawn, J., Mahasuweerachai, P., & Thampanishvong, K. 2019.
Farms, Farmers and Farming: a Perspective through Data and Behavioral Insights. BOT Symposium Discussion Paper.
Beck, U. 1992. Risk Society: Towards a New Modernity. London, UK: Sage Publications.
Beecham Research.2014. Towards smart farming: agriculture embracing the IoT vision. http://www.beechamresearch.com/files/BRL%20Smart%20Farming%20Executive%2... 2014.
Bronson, K., 2018. Smart Farming: including rights holders for responsible agricultural innovation. Technol. Innov. Manage. Rev. 8 (2), 7–14.
Bronson, K., Knezevic, I., 2016. Big data in food and agriculture. Big Data Soc. 3. https://doi.org/10.1177/2053951716648174
Bureau of Agricultural Economic Research. 2015. Farm efficiency by farm size and farm land holding and income inequality office of Agricultural Economics in crop year 2011/12. Office of Agricultural Economic, Bangkok. [in Thai]
Bureau of Agricultural Economic Research. 1997. Production efficiency by farm type and farm size in crop year 1995/96. (Research report). Bangkok, Thailand: Office of Agricultural Economics. [in Thai]
Bureau of Agricultural Economic Research. 2007. Factor affects labor efficiency in crop year 2004/2005. (Research report). Bangkok, Thailand: Office of Agricultural Economics. [in Thai]
Bureau of Agricultural Economic Research. 2015. Farm efficiency by farm size and farmland holding and income inequality office of Agricultural Economics in crop year 2011/12. (Research report). Bangkok, Thailand: Office of Agricultural Economics. [in Thai]
Carbonell, I. 2016. The Ethics of Big Data in Agriculture. Internet Policy Review, 5. https://doi.org/10.14763/2016.1.405
Carolan, M., 2016. Publicising Food: big data, precision agriculture, and co-experimental techniques of addition. Sociol. Ruralis, 57.
CAT. 2019. CAT brings IoT smart agriculture to Saraburi Wittayakhom School to become a learning center for modern agriculture. [Online]. Available from https://www.cattelecom.com/cat/content/3538/216/CAT+นำ+IoT+หนุนการพัฒนาเกษตรอัจฉริยะให้กับ+รร+สระบุรีวิทยาคม+ก้าวสู่ต้นแบบศูนย์การเรียนรู้เกษตรสมัยใหม่ ?accesEye=on&lang=th_TH. Access on 20 October 2020. [in Thai]
Cohen WM, Levinthal DA. 1999. Absorptive capacity: a new perspective on learning and innovation. Adm Sci Q 1999;35: 128–52.
Driessen, C., and Heutinck, L. F. M. 2015. Cows Desiring to Be Milked? Milking Robots and the Co-Evolution of Ethics and Technology on Dutch Dairy Farms. Agriculture and Human Values, 32: 3–20. https://doi.org/10.1007/s10460-014-9515-5
DTAC. (nd). Smart Farmer program. Available from https://www.dtac.co.th/sustainability/en/project/Project-SmartFarmer. Access on 20 October 2020.
Duang-Ek-Anong.S.,Pibulcharoensit,S.,and Phongsatha, T. 2019. Technology Readiness for Internet of Things (IoT) Adoption in Smart Farming in Thailand. International Journal of Simulation -- Systems, Science & Technology. 2019. Vol. 20 Issue 5, p1-6.
Eastern Economic Corridor (EEC) Office. 2020. EECi: An Innovation City to Transform Country’s innovation into a commerce. Electricity and Industry Magazine. Vol. 5, 2020.
Eastwood. Osipov and L. Riliskis.2013. "Educating innovators of future Internet of Things". IEEE Frontiers in Education Conference. OK, USA, 2013.
Fan S., and Chan-Kang C., 2005. Is small beautiful? Farm size, productivity, and poverty in Asian agriculture. The journal of the international Association of agricultural Economists. 32 (135-146).
Fleming, A., Jakku, E., Lim-Camacho, L., Taylor, B., Thorburn, P., 2018a. Is big data for big farming or for everyone? Perceptions in the Australian grains industry. Agron. Sustain. Dev. 38.
Freeman C, Perez C.1988. Structural crisis of adjustment, business cycles and investment behaviour. In: Dosi G, Freeman C, Nelson R, Silverberg G, Soete L, editors. Technical change and economic theory. London: Pinter; 1988. p. 38–66.
Ge, L., Bogaardt, M.J., 2015. Bites into bits: governance of data harvesting initatives in agrifood chains. Paper Presented at the European Association of Agricultural Economists, The Hague, The Netherlands.
Gubbi J, Buyya B, Marusic S, Palaniswami M. 2013. Internet of Things (IoT): a vision, architectural elements, and future directions. Future Gen Comput Syst 2013;29(7):1645–60.
Kaloxylos A, Eigemann R, Teye F, Politopoulou Z, Wolfert S, Shrank CS, et al. 2012. Farm management systems and the future internet era. Comput Electron Agric 2012; 89:130–44.
Kraipinit, Y. 2018. Study of Program Development for Smart Farmers of Agribusiness Students. [Online]. Available from http://www.polsci-law.buu.ac.th/journal/document/
11-1/22.pdf. Access on 8 October 2020.
Kuhasak, N. and Srichampa, R. 2018. Study of Appropriate Smart Farm Business Model for Small and Medium Entrepreneurs (SMEs) of Melon. [Online]. Available from http://www.journalgrad.ssru.ac.th/index.php/miniconference/article/view/.... Access on 9 October 2020.
Kwanmuang K. 2015. Farmland Inheritance, Transaction and Transference: A Case Study in the Southern Region of Thailand, The Japanese Journal of Rural Economics 17: 46-51.
Kwanmuang, K. 2018. Succession Decisions and Inherited land Size: An Evidence of Family Farms, in Nakhon Si Thammarat Province, Thailand. Applied Economics Journal Vol. 25 No. 2 (Demember 2018): 70-88.
Lehmann RJ, Reiche R, Schiefer G. 2012.Future internet and the agri-food sector: state-of-the-art in literature and research. Comput Electron Agric 2012; 89:158–74.
Logindarat, P. 2011. “pon kratob kon kan pranprang krongsart pracharkon pakkasart teme tor kwammunkong nai kan palit arhan kon pakkasartthai (Effecting of changing agricultural population structure on food security research project in Thailand),” TRF Policy Brief journal, Vol.4, 2011.
Manwicha J. 2015. Smart Farm Technology. Hatyai Academic Journal 14(2): 201-210. [in Thai]
Millar, K. M. 2000. Respect for Animal Autonomy in Bioethical Analysis: The Case of Automatic Milking Systems (AMS). Journal of Agricultural and Environmental Ethics, 12(1): 41–50. https://doi.org/10.1023/A:1009548025408
Ministry of Agriculture and Cooperatives (MOAC). 2019. Smart Farming Action Plan during 2020 to 2022. [Online]. Available from https://www.alro.go.th/research_plan/
ewt_dl.php?nid=1218&filename=index. [in Thai]
Ministry of Agriculture and Cooperatives. 2019. Transforming agriculture Ministry of Agriculture and Cooperatives push “smart tomatoes greenhouse”. [Online]. Available from https://www.opsmoac.go.th/datacenter-news-preview-411691791322. Access on 20 October 2020.
National Electronics and Computer Technology Center (NECTEC). 2020. Handy Sense precision farming system - Smart Farm from Thai farmers’ understanding. [Online]. Available from https://www.nectec.or.th/news/news-article/handy-sense-interview.html. Access on 17 October 2020.
National Science and Technology Development Agency (NSTDA). 2020. Technology demonstration and showcase of smart farming technologies at Maejo University. [Online]. Available from https://www.nstda.or.th/en/news/news-years-2020/971-technology-demonstration-and-showcase-of-smart-farming-technologies-at-maejo-university.html. Access on 20 October 2020.
National Science and Technology Development Agency (NSTDA). 2020. Translating “Sense” to “Data” to improve quality of durian farm. [Online]. Available from https://www.nstda.or.th/agritec/durian-smart-tech/. Access on 20 October 2020.
Office of Agricultural Economics 2016. Agricultural economics indicator of Thailand. Bangkok, Thailand: Office of Agricultural Economics. [in Thai]
Office of Agricultural Economics. 2015. Agricultural economics indicator of Thailand. Bangkok, Thailand: Office of Agricultural Economics (in Thai).
Otsuka K., Liu Y., and Yamauchi F. 2016. Growing advantage of large farms in Asia and its implications for global food security. Development of Policy Review, 34(3):441-461.
Pattama N. 2020. The development of Smart Farming Systems in Thailand. [Online]. Available from https://www.senate.go.th/document/Ext23700/23700529_0008.PDF.
Penrose ET.2009. The theory of the growth of the firm. Oxford: Oxford University Press; 2009.
Perez C. 1988. New technologies and development. In: Freeman C, Lunvall BA, editors. Small countries facing the technological revolution. London: Pinter Publishers; 1988. p. 85–97.
Phonpho, T. and Phonpho, K. 2013. Development of automatic nursery seeding machine. [Online]. Available from http://www.repository.rmutt.ac.th/bitstream/handle/123456789/1778/. Access on 9 October 2020.
Poapongsakorn, N., Ruhs, M. and Tangjitwisuth, S.1998. “Problems and outlook of agriculture in Thailand,” TDRI Quarterly Review, Vol.13, No.2, 1998, pp.3-14.
Samseemoung, K. and Sangpradit, K. 2014. Design and Fabrication of high performance vegetable seeder of Brassica alboglabra for seeding trays. [Online]. Available from https://li01.tci-thaijo.org/index.php/thaiagriculturalresearch/article/v.... Access on 8 October 2020.
Schumpeter JA, Garcia JD. 1983. Capitalismo, socialismo y democracia. Barcelona: Orbis; 1983.
Sentangsedtee. 2019. AIS partners with Uncle Ree Farm to apply IoT platform i-Farm for urban farming. [Online]. Available from https://www.sentangsedtee.com/today-news/article_134891. Access on 20 October 2020.
Siam Kubota Corporation. n.d. About KUBOTA (Agri) Solutions. [Online]. Available from https://www.kubotasolutions.com/about/definition. Access on 20 October 2020.
Sorensen CG, Fountas S, Nash E, Pesonen L, Bochtis D,Pedersen SM, et al. 2010. Conceptual model of a future farm management information system. Comput Electron Agric 2010;72(1):37–47.
Sriamnouy,B. Plangsorn,S. Piticharoenpon,V., Srihanam,M. 2019. Study of Smart Farming Design by Using Internet of Things for Lime Farm in Phetchaburi Province. The 6th National Conference Nakhonratchasima College. [Online]. Available from http://journal.nmc.ac.th/th/admin/Journal/2562Vol9No1_89.pdf. Access on 8 October 2020.
The Reporter Asia. 2020. TRUE Cooperation joins Khon Kaen University to develop smart agriculture [Online.] Available from https://www.thereporter.asia/th/2020/02/03/true-5g-2/. Access on 18 October 2020.
Verónica Saiz-Rubio and Francisco Rovira-Más .2020. From Smart Farming towards Agriculture 5.0: A Review on Crop Data Management. Mdpi agronomy review.
Wattanutchariya, S. and Jitsanguan, T. 1992. Increasing the scale of small-farm operations I. Thailand. Retrieved from http://www.agnet.org/library.php?func=view&id=20110726143050.
World Economic Forum. 2016. The Future of Jobs. Geneva: World Economic Forum (WEF). [Online]. Available from https://www.weforum.org/reports/the-future-of-jobs
Wolfert, S., Ge, L., Verdouw, C., Bogaardt, M.J., 2017b. Big data in smart farming – a review. Agric. Syst. 153, 69–80.
World Bank. 2017. Research and development expenditure (% of GDP). [Online]. Available from http://data.worldbank.org/indicator/GB.XPD. RSDV.GD.ZS; 2017.
Yanmar. n.d. SMARTASSIST (SA-R) system by Yanmar. [Online]. Available from https://www.yanmar.com/th/news/2020/05/29/74726.html. Access on 20 October 2020.
Yungyuen, K. 2020. The Responsible Agriculture Investment Policy to create Social Welfare and Food Security. Journal of Social Synergy, 11(1), 32-61. [Online] Available from https://so04.tci-thaijo.org/index.php/thaijss/article/view/242029.
Small-Scale Farmers under Thailand’s Smart Farming System
ABSTRACT
This study aims to review the approaches and policies on smart farming for small-scale farming in Thailand. The opportunities and challenges of smart farming as well as initiatives policies are also discussed. Under the challenges in many aspects on the agricultural sector, such as declining of average farm size, an increasing number of aging farmers, labor shortage in the sector, while the demand of global food is increasing, thus, initiatives of smart farming system for small farmers will inevitably be cascaded to farmers in the future. Thus, raising the awareness of technology acceptance by farmers and also the cooperation on working on researches, invention and development of smart farming technologies that are suitable for farmers from all related agencies are needed. However, by concerning the constraint of smart farming that would arise from technical unemployment and the knock-on impact on rural populations, government also needs a long-term plan to deal with the problem of unemployment that may occur as a result of the decrease in both working hours and labors. Moreover, to take smart farming into practice, the government should support smart farmers who settle throughout the country and have a strong network for conducting pilot systems and contributing the lessons to other farms.
Keywords: small-scale farming, smart farming, IoT platform, IoT devices
INTRODUCTION
Agriculture plays a crucial role in economic growth and development of Thailand as it is comprised of production resources, and raw material of continuous manufacturing by both processing and product industry. Agriculture also creates employment and income of most population in Thailand. The areas over 23.88 million hectares of the country which accounted for 46.54 % of total areas are used for agricultural activities in planting, livestock and fisheries. For decades, most farmers get used to do traditional farming by following the approach of their parents and ancestors for a long time. This implies that they rarely take an advantage of technology, digital innovation and information for farming.
The agriculture sector in Thailand is facing many challenges, both from structural factors and external changes that directly affect productivity and competitiveness of farmers in the country.
A decrease in the average size of small-scale farms is one of agriculture’s concerns. Half of the total land (46.54%), or 23.88 million hectares, is used for agriculture, and the vast majority of agricultural production in Thailand occurs on small-scale farms (Office of Agricultural Economics, 2016). Family-run farms have an average farm size of 4.04 hectares. Even though this farm size is larger than many other Asian countries such as Japan, China, India, South Korea (Fan and Chan-Kang, 2005), Bangladesh, Indonesia, and the Philippines (Otsuka et al., 2016), however, in common with most of these Asian countries (except Japan and South Korea), Thailand has been experiencing a decline in average farm size over the past number of years. From 1993 to 2016, the annual average farm size per household decreased by 0.66% across the country. This phenomenon of gradual decline in average farm size has been found in every region of Thailand.
There are 5.9 million farms with an average farm size of 4.04 hectares (or 25.26 rais). Moreover, of the 5.9 million total farm households, 26.5% of farm households had less than 1.6 hectares (or 10 rais, the smallest size of land) in 2012. And almost half of farms (43.6%) had land areas from 1.6 to < 4.8 hectares (or 10 to < 30 rais). Additionally, the share of farm holdings of the land less than 1.6 hectares or of the smallest size increased from 22.5% in 1996 to 26.5% in 2012. But in the same period, the share of large-sized farms over 8 hectares (i.e., 50 rai) decreased from 11.4% to 8.8% (Bureau of Agricultural Economics Research, 2015). This evidence showed the increasing of the share of farm households holding the smallest-sized farms (less than 1.6 hectares) (Kwanmuang, 2018).
There are many factors that influenced this declining average size, including industrialization, urbanization, institutionalization, land policy, property rights, and land and labor markets (Fan and Chan-Kang, 2005). In Thailand’s case, in addition to these factors, the custom of dividing the land for inheritance led to the reduction in farm size for the next generation. Whether the household has a successor or not, farm size per child was not significantly different between the households (Kwanmuang, 2015; Kwanmuang, 2018). The findings increased awareness of concerns, especially for successors who will inherit small farms. In other words, in the agriculture sector, the average scale of farming will decrease. This phenomenon may increase the unbalanced growth between agriculture and non-agriculture lands and increases more concerns regarding the sustainability of agriculture. Thus, the program for supporting and enhancing ability for small-scale farms is needed in the future (Kwanmuang, 2018).
Although many studies revealed that small farms were more productive than larger ones due to the advantages of small farms using farm labor or labor-intensive processes (Fan S., et al., 2005), including the reverse relationship between farm size and productivity still exists in Thailand (Wattanutchariya and Jitsanguan, 1992; Bureau of Agricultural Economic Research, 1997, 2007, and 2015), they may be affected by increasing labor wages. Thus, small farm sizes may lose their competitive advantages due to the decline of small farm production efficiency relative to large ones (Otsuka et al., 2016).
Moreover, the future concern is that the average age of the head of the farm household was 56 years (Office of Agricultural Economics, 2016). Almost half of farm households are aging farmers who lack access to knowledge and new technologies. The aging problem will become serious and affect the productivity of farms in the next 10 years (Logindarat, 2011). Meanwhile, the decline in the number of younger people who want to work on farms (Poapongsakorn et al., 1998) raises concerns about the success to farms and the agricultural sectors in the near future.
Furthermore, another concern is the lack of farm labor. The number of agricultural laborers has shown a gradual decline in every region. From 1998 to 2014, the farm labor force decreased from 22.80 million to 17.78 million, decreasing by an annual rate of 1.18% (Office of Agricultural Economics, 2015), and this trend occurred across all regions.
Additionally, most farmers are owners of small farms and have constraints in terms of economies of scales for getting into resources and technologies. There is also lack of bargaining power in the market that has a long supply chain as well as the implementation of agricultural policies that may not be effective and widespread to farmers. The impact of aforementioned challenges together with climate change and higher competitiveness of global market all affect most of farmers to have low productivity and profit, and heavily depend on the government policy supports (Attavanich et al., 2019).
With these challenges, the government focuses on the development of agricultural sector and aims that agriculture, farmers and farmer institutions need to have security, prosperity and sustainability within the next 20 years by driving relevant projects through the 20-year national strategic plan (2017 to 2036) and 20-year Agriculture and Cooperatives Strategy (2017-2036) and the agricultural development Plan in the 12th national economic and social development plan (2017-2021). These three plans are related and intend to transform traditional agriculture into smart agriculture especially as changes of consumer behavior affected by the impact of COVID-19 leading to the new normal, small farmers will have to change and inevitably shift and do smart agriculture.
Hence, this research aims to mainly review the approaches and policies on smart farming for small-scale farming in Thailand in order to understand the state of development of the system which is one of the ways to solve agricultural problems in the future.
LITERATURE REVIEW: SMART FARMING AS WORLDWILD PERSPECTIVE
Definitions of smart farming
There is no single definition of smart farming, one is the integration of data and communication technology into agricultural production system. Another defines Smart farming as the use of information transfer and concentration in remote storage systems (servers), to allow different farm data to be combined and evaluated for decision-making. Beecham defines Smart farming as a software engineering and computer science concept that came about by adding computer techniques and by transmitting data from agriculture in a virtual environment (Beecham research, 2014). Farm Management Information System (FMIS), is one of the first academic definitions of smart farming. FMIS is defined as a system for gathering, sorting, preserving, and disseminating data in an acceptable format for the success of agricultural land activities and functions (Sorensen et al., 2010).
Due to technology advancement, two factors make Smart farming possible: data collection and data storage and analysis. Data collection is guaranteed by using sensors in agriculture. A sensor is an electrotechnical system that detects environmental and physical quantities (temperature, humidity, light, pressure, presence or absence of certain types of artifacts, mechanical stress levels, and object size) then transforms them into a signal that can be interpreted and collected as data (Lehmann et al., 2012).
Likewise, the Internet of Things (IoT) is a smart farming-related invention. IoT shares with FMIS the idea of an intelligent environment (Gubbi et al., 2013). The Internet of Things enables objects to be remotely operated over existing network infrastructure. However, the use of IoT relies on the internet infrastructure and this poses a variety of weaknesses, in particular when a vast number of devices are connected and when combined with other systems (Kaloxylos A et al., 2012).
Smart farming instruments implement a new level of agricultural technologies, including automation, mapping and geomatics, decision-making, and mathematical processes. The most promising smart farming developments include advancements in sensors, data processing, telemetry, and positioning technologies, but it may take time and effort to evolve and disseminate these technologies.
Opportunities of smart farming
Schumpeter who focused on the relation between innovation and economic growth mentioned that technical innovation modifies manufacturing habits and can distinguish between regions and countries’ economic development (Schumpeter and Garcia, 1983). Likewise, Freeman and Perez, introduced the concept of a techno-economic pattern as a way of explaining how technology and innovation are progressing. They stated that interruption periods of technological standards produce new products and approaches, leading to important behavioral changes (Freeman and Perez, 1988). As a result of introducing mechanization and chemistry, deep shifts have arisen in the agriculture industry, which leads to the usage of the Internet of Things, in smart environments, and the use of cloud computing.
The previous innovation-economic growth relation should be investigated, to do so, development organizations (R&D) are required to change the techno-economic context (Penrose, 2009). R&D investment is needed, as there are degrees of accumulation of technologies and advanced development processes when comparing different regions and countries (Cohen and Levinthal, 1999). According to the World Bank, R&D investment was concentrated in 2013 for both public and private in some countries, including South Korea (4.15 % of the gross domestic product), Japan (3.47 %), Denmark (3.60 %), Germany (2.85 %), and the USA (2.81 %) (World Bank, 2017).
The economic potential of smart technologies applied to agricultural production is clearly understood by companies and governments. As an example, Cropio is a satellite field management system developed by New Science Developments New York in 2013 (Verónica Saiz-Rubio et al, 2020). And purchased by Syngenta in 2019, the first agricultural firm to have access to leading management platforms in the top four agricultural markets, USA, China, Brazil, and Eastern Europe. These 4 countries market combined 98 million acres and it will be handled internationally using the Syngenta Automated Platform, to double by the end of 2020 (Agriculture and commodity trade chamber, 2019).
As a platform for crop management, health, and vegetation control, Cropio allows its users to plan and carry out agricultural operations effectively. It offers real-time updates on current field and crop conditions, describes vegetation levels, highlights problem areas, and provides accurate weather forecasts and an individual commodity market report.
The key purpose of Cropio and its functions is to maximize the use of resources and improve the efficiency of the farming sector. Besides, Cropio expands its cloud solutions to various vendors to gather all data required for the farmer in a single location and in a convenient way. Farmers do not need to download new programs and data is converted from one device to another or from one format to another.
The advantages of these emerging ICT technologies for agriculture are diverse: farmers are offered with improved production and benefits (Bronson and Knezevic, 2016; Ge and Bogaardt, 2015), while these technologies will help solve concerns such as food security and sustainability at the social level (Carolan, 2016). At the same time, the transformative nature of digitization means that social disturbance is expected; it can affect economies, corporate structures, and supply chains, agriculture and rural societies, ownership, and privacy. Such impacts will be beneficial, but there is also the possibility of surprising harmful results when emerging innovations are incorporated into society (Bronson, 2018).
Challenges of smart farming
Fleming has pointed out possible tensions or concerns about the digital divide and unequal development between different farm sizes (e.g. small and large), territories (e.g. urban and rural), and persons (e.g. age, socio-economic or educational disparities) (Fleming et al., 2018). Likewise, Bronson has raised awareness about technical unemployment and the knock-on impact on rural populations (Bronson and Knezevic, 2016). While, there are undeniable benefits beyond economic gains associated with smart farming advances (for example environmental gains by decreased input usage), there are potentially negative effects, especially socio-ethical consequences for humans and non-humans (Wolfert et al., 2017; Carbonell, 2016; Driessen and Heutinck, 2015; Millar, 2000). To date, these possible effects of smart farming have been investigated by just a few reports. However, it is important to draw implications from the use of intelligent inspiration in other fields. In this sense, the World Economic Forum expects a wide-scale “technological unemployment” rising from the application of automation in agriculture. This was possible by extrapolating the automotive industry mechanization to the automation of agriculture (World Economic Forum, 2016). In many communities, shifts in rural workplaces could have a significant effect on social stability and the livelihoods of many workers (Carolan, 2016). In Australia for example, analyses of the application of smart technologies to the dairy industry show a reshaping of farming activity, with less labor force and more data-driven choices (Eastwood et al., 2013).
Ethical problems (such as animal abuse, unemployment-economic benefit relation) would raise challenges in deciding which data to collect to accomplish social objectives, and how much access to socially relevant data sets to be allowed. Indeed, the Global Open Data for Agriculture and Nutrition (GODAN) initiative of the United Nations, calls for an open access to agricultural data as a constitutional right, they claim that it would lead to solve serious humanitarian problems of global food insecurity. The majority of literature on innovation and community indicates that GODAN will profit both advantages and costs, regardless of what we know about smart farming in particular (Beck, 1992). However, besides the increased efficiency and favorable environment, smart farming could have negative consequences, too. The socio-ethical consequences have been explored by several scholars. It has been proposed that smart farming, with less ‘hands-on’ management and a more data-driven approach, would reshape farming practices (Eastwood et al., 2013).
To enable and integrate smart farming technologies, a range of skills and advisory structures would be required around the farming team, in order to relocate farm labor force and service providers. By integrating detailed monitoring, agricultural machinery manufacturers, input vendors, processors, and retailers, several changes can have a significant impact on what it means to be a farmer, since a more formal and assessed approach in smart farming will replace the old concept of “your land, your way” (Bronson and Knezevic, 2016; Carolan, 2016).
Challenges of smart farming in Thailand
The agriculture sector in Thailand are challenged in many aspects. As population ages, labor shortage in the agriculture sector tends to rise from the past, while the demand of global food is increasing. In the past, farmers always faced the problem of price falling while the production cost was high and the weather was unpredictable. In addition, farmers also faced the lack of capital access and agricultural technology as well as the inconsistent implementation of government policies and concentrated on the short-run (Pattama, 2020). This shows the urgent need for improvement of agriculture sector to help farmers increase their productivity, reduce the costs of production and improve the living standards.
Although smart farming is considered as one of the management approaches that help increase productivity and economic returns while reducing the environmental impact, the implementation of Thai farms is uncertain. In Thailand, smart farming which precisely managed is considered as a new thing for small-scale farmers because there are constraints in terms of the technological readiness to apply technologies and innovations in their farms accurately and properly. The significant factors that have hindered the adoption of new technologies and innovations in smart farming are technological, economic and social (Manwicha, 2015).
To adopt technologies in smart farming, farmers needto have the ability to get access and exploit the benefit of the advent of technologies and innovations to make use of data for planning and managing their farms (Pattama ,2020). A research conducted by Kuhasak and Srichampa (2018) on management pattern for smart farming business indicated that entrepreneurs needed to learn, understand, and use technologies that was suitable and useful for their farm to get the proper management pattern of smart agriculture for small and medium enterprise (SMEs), and to solve problems of uncontrolled pests and diseases in farms. A way for training technology is by educating farmers to understand what the technology was and how it could be beneficial to them. As a result, policymakers should provide resources for learning or develop programs that suit for farmers to find those resources (Duang-Ek-Anong et al., 2019). Government also launched the project that encourages young farmers to be “Smart farmers”. This project intends to develop young farmers to learn to manage their farms by using technologies and innovations so that they can become a self-reliant entrepreneurs and local agricultural leaders in their regions (Yungyuen, 2020). The study of Kraipinit (2018) on development program for smart farmers of agribusiness students also supported that people who attended the program had higher skills for doing smart agriculture. This program developed from the idea of cognitive thinking approach consisting of stimulation, thinking, perception, and memorizing. The results showed that the important skills for smart farmers were thought leadership and empowerment skills. The knowledge gained from this agricultural model could be used for developing and building supply chain of farming and used as a guideline to improve skills of smart farming as well.
The investment on new technology is one of the challenges for the adopting of smart farming. In order to use technologies and innovations, the investment is needed to plan and build new farming systems that are suitable for their farms. As farmers in Thailand are normally small-scale farmers, changing to do smart farming required a lot of money and the return on investment is uncertain for small farms. Additionally, as agricultural process takes many steps and different methods, technologies from different farms could have different standards and difficult to work together effectively. This may also increase the cost of investments, therefore, farmers are not affordable to those smart farming technologies (ARDA, 2020). To support smart farming, Thailand Board of investment (BOI) offers promotion investment scheme which provides tax exemption for the smart farming investors. However, if Thailand can create and develop technologies in smart farming with lower cost, smart farming would be widely used.
THAILAND POLICY ON SMART FARMING
Smart farming technology in Thailand
Smart farming technology is a new agricultural innovation in the digital era that adopted various information and communication technologies in farms such as GPS, GIS, remote-sensing, proximal-sensing, variable rate technology (VRT) and decision support system (DSS) through the wireless network for receiving, sending, and evaluating by package software. The processes for doing smart farming consist of data collection, data diagnosis, data analysis, implementation of the plan, and evaluation. These are operated with farm management in every step by mapping of soil conditions, watering, fertilization, pest control, harvesting, cost-profit calculation, and planning for the next planting season. As a result, smart farming leads to the efficient use of production factors, the increase in quality of farm production, cost-effectiveness, reducing environmental impact, cost of production, and labor, saving time and leading to sustainable agriculture (Manwicha, 2015)
In response to the development of Thai agriculture, the Ministry of Agriculture and Cooperatives (MOAC) intends to transform traditional farming into “Smart Farming” by using advanced information technology in the production of crops, livestock and fisheries as well as using the intelligent sensor systems to monitor and evaluate their production in real-time.
Smart farming is considered as one of the important strategic goals in Thailand which aims to enhance Thailand’s potential by focusing on the increase in production both in terms of quality and quantity by applying new technologies and innovations in the production process, while decreasing a number of labors and cost of production. Smart farming combines environmental sensor networks and artificial intelligence – based analysis such as digital technology, biotechnology, modern agricultural machines, Internet of Things and intelligent sensor systems in assisting, planting, and production. To implement smart farming in Thailand, MOAC prepares agricultural field demonstration of smart farming which enables farmers to gain knowledge and improve skills from doing smart farming and be ready for agricultural transformation of Thailand in the future (MOAC, 2019).
Smart agricultural development of the Ministry of Agriculture and Cooperatives
With regard to the Thailand 4.0 policy, Thailand’s 20-Year National Strategy Plan, the Ministry of Agriculture and Cooperatives (MOAC) intends to transform traditional farming into smart farming or Agriculture 4.0 by using technologies and innovations. The MOAC appoints the smart farming-driven committee which are responsible for imposing plans and policies of smart farming, and driving smart agricultural development of MOAC through the process of agricultural field demonstration of smart farming, development in Big Data platform, Artificial Intelligence (AI), robotics and machine development, agricultural research and development of remote sensing and the potential development of agricultural manpower. The details and progress of smart farming are as follows.
Agricultural field demonstration of smart farming
The smart farming-driven committee has cooperated with domestic and foreign organizations to make use of technologies for trails in the agricultural field. These field demonstrations are tested with various crops such as rice, sugarcane, maize, cassava, pineapple, and tomato by using technologies applied in the field demonstration such as unmanned aerial vehicle (UAV), multispectral camera technology, auto steering, laser land leveler, weather station, autonomous water monitoring system, and autonomous harvesting system. Agricultural field demonstration also records and collects data for linking to Big Data platform. This data can be used as a tool for decision making and set guidelines for the future work plan of smart agriculture.
Smart farming Big Data Platform
The smart farming-driven committee attempted to make use of data obtained from the application of smart farming technology to help support planning and decision making at the war room of the MOAC. The committee also cooperated with other organizations in order to link data both inside and outside of the MOAC to agricultural Big Data platform for analyzing and monitoring plant growth such as data of crop situation from satellite images, weather detection, and weather forecast. The work plan includes two parts which are the estimation of production and pest monitoring, both of which are the key factors for balancing the supply of production with the market demand and decreasing production cost. Big data platform is used for estimating and planning supply of production in order to deal with market conditions which affect crop prices. In the farm level, the MOAC develops crop monitoring system by taking advantages of sensor technology to collect data from the field for analyzing, and monitoring crop situation in farms. This data can help predict crop situation and control damages in farms. In the country level, the MOAC uses data from the survey of farmers’ field, satellite images, and data of weather and natural disasters to manage crop production to the market. Additionally, the MOAC has developed a prototype of pest recognition that collected data via web application to manage and prevent damages occurred to farm and productions effectively.
IMPLEMENTATION OF SMART FARMING FOR SMALL-SCALE FARMING IN THAILAND
Transforming the agriculture sector to smart agriculture is very challenging because most of Thai farmers are aged, operating in small-scale basis and lack of skills and resources. In the past, smart agriculture technologies were adopted by mostly large-scale farmers due to economies of scale and financial viability. As the advance of information and digital technology, the cost of developing and implementation of smart farming technologies become more affordable. In addition, smart farming technologies are extended to small-scale farming system. This section provides an overview on the current situation of smart farming implementation in Thailand.
Various organizations including government agencies, educational institutions, and private companies have launched projects and initiatives in an attempt to make smart farming technologies available to small farmers. The Ministry of Agricultural and Cooperatives has placed high priority on smart farming. The ministry promotes smart farming technologies through field demonstrations.
In 2019, field demonstrations were focused on six crops: paddy, sugarcane, maize, cassava and pineapple and tomatoes (Ministry of Agriculture and Cooperatives, 2019). National Science and Technology Development Agency (NSTDA), a government research center, developed a smart agricultural system for greenhouse called “Handy Sense” which is able to monitor key environmental variables including light intensity, soil moisture and temperature, and air humidity and temperature in real time and also able to control i.e. irrigation (NECTEC, 2020a). A research conducted by Phonpho and Phonpho (2013) has studied the development of automatic nursery seeding machine which is controlled by a programmable logic controller. The results showed that seeding machine were highly effective for sowing up to 91.98 % of needle head number 18 and able to sow seeds into seedling tray for 94.7 trays per hour which saved time than using human labor for 3.45 times. In addition, Samseemoung and Sangpradit (2014) designed and fabricated a high-performance vegetable seeder of Brassica alboglabra for seeding trays. This machine was invented to meet farmers’ need for replacing human labor, reducing seed loss, saving time, and decreasing human fatigue for seeding.
Not only public sectors, private companies ranging from telecommunication companies, agricultural machinery manufacturers, agricultural input suppliers and agri-tech startups also put serious efforts on smart farming technologies. Total Access Communication (DTAC), a big telecommunication company, joined with Samnuek Rak Ban Kerd, a social enterprise and Ricult, an agritech startup, to develop a service named “Farm Man Yum”. The service use Artificial Intelligence (AI) to provide weather forecast (temperature, probability of precipitation, and rainfall amount) at specific location, provide a satellite image for monitoring crop health and give advice, tips and knowledge from planting to harvest. In addition, DTAC, the Department of Agricultural Extension and National Electronics and Computer Technology Center (NECTEC) collaborated and developed a greenhouse IoT farming platform. A greenhouse is equipped with sensors to measure air, soil and light condition where farmer can monitor via smartphone or smart device in real time (DTAC, n.d.). Similar IoT platforms are developed by other enterprises for example Intelligence Farm or i-Farm platform developed by Advanced Info Service (AIS) (Sentangsedtee, 2019) or an IoT platform for vegetable greenhouses developed by CAT Telecom (CAT) (CAT, 2019). The concept of internet of things was also applied on the design of smart farming in lime farms in Phetchaburi Province to increase lime production efficiency by using a sensor to measure data of four parameters which are temperature, humidity, moisture and pH. This design by connecting IoT devices (Node MCU) to Arduino board and sensor system as well as the development of application for connecting IoT devices could display the standard values with various mobile applications which supported both in IOS and Android operating system (Sriamnouy et al., 2019).
IoT platform is not only limited to crop production, True Corporation (TRUE) joined with Khon Kaen University, and Charoen Pokphand Foods (CPF) to apply the 5G technology to smart agriculture. The plan involves subprojects, for example, to apply CCTV cameras and sensors to get information and to use AI technology to analyze and monitor chicken health. (The Reporter Asia, 2020)
Agricultural machinery manufactures also developed a new generation of agricultural machineries incorporating IoT and robotics technology enabling agricultural machineries to send data from the field while it can be controlled from distance, for example (Yanmar, n.d). Beside IoT machinery, some manufactures also offer a digital solution service such as KUBOTA Agri Solutions (KAS) by Siam Kubota (Siam Kubota Corporation, n.d.)
SUCCESSFUL CASE OF IMPLEMENTATION
We conducted a field study of successful case of using smart farming system, this farm belongs to Mr. Somboon Ngamsangiam. With cooperation and help from National Science and Technology Development Agency (NSTDA) collaborated with a Thai farmer, Mr. Somboon Ngamsangiam has adopted technology of plant watering control system in the field experiment of durian farm in Rayong Province.
The entering point of using this smart technology started with the experience of durian growers. They knew that water and weather are two important factors for durian cultivation, but they did not notice that what they observed can be measured scientifically. Moreover, in the past, Mr. Somboon recorded revenues and expenses on his farm and he found that the cost of the common water pumping system using diesel fuel for durian trees each year was quite high. The common system for 8 hectares (50 rais) of durian farm consumed approximately 18,667 USD per year (or 2,334 USD per hectares). This is because he did not know whether the soil needed water at that moment or not, resulting in unnecessarily pumping water for durian trees. Moreover, Mr. Somboon attended a meeting which explained and introduced technology of automatic water control system developed by NSTDA. He was interested to apply this technology in his own farm as this technology helped reduce water supply, fertilizer loss, and production damage. It also helped save electrical energy and the investment cost was low due to the use of small water pump.
Thus, in the beginning, Mr. Somboon and NSTDA started the field experiment using technology of automatic water control system that used the sensor to measure soil moisture, air humidity, light intensity and temperature in the field experiment in a durian farm for 0.32 hectares (2 rais).
For this first year of experiment, data on soil moisture in every stage of planting was collected. During the growth stage, the percentage of soil moisture was not below 28, while the percentage of soil moisture in flowering stage was between 28 and 34. For the fruiting stage, the percentage of soil moisture was around 32 to 40. These results were obtained by collecting data of soil moisture that was suitable for each growing stage from the system as well as observing by converting his sense to number. Although the sensor was not stable in the first time, it was developed until now to be acceptable and useful for collecting data to manage farms and plan for the next year cultivation (NSTDA, 2020).
As the result of field experiment is satisfied, Mr. Somboon has invested the automatic water control system in the farm up to 8 hectares (50 rais). By applying the automatic water control system, he significantly decreased the production cost from using common pumping machine using diesel fuel which costed approximately 18,667 USD per year to 8,333 USD per year. This implied that he could save cost of production approximately 10,334 USD per year.
However, in terms of investment in this system, in the first year, Mr. Somboon had to invest in electric pumps and electrical installations which accounted for 23,333 USD. The system also included two sets of agricultural weather stations fully supported by NECTEC. Since it was still in the development stage, the cost must be estimated and it would cost approximately 3,333 USD for two sets. Thus, the total investment costs of automatic water control system were approximately 26,666 USD. In comparison to the cost he could save, this showed that the investment in automatic water control system could reach the breakeven point within three years. Moreover, this system could also be applied to a smaller farm or orchards where one set of equipment including one electrical pump and one weather station could optimally operate 4 hectares (25 rais).
In conclusion, although in the first year he had to invest in technological equipment and machine which accounted for approximately 26,666 USD, it could reach the breakeven point within three years. The parameters of those measurements of this system are used not only for management of durian farm especially the water input according to the needs of durian trees during growth stage, flowering, and fruiting which help farmers to eliminate the problem of overwatering, but also helped reduce the number of labors worked in his farm or reduce labor costs, fertilizer loss, production damage, and control quality of fruit yield, resulting in the increase in farm revenue more than twice (Eastern Economic Corridor (EEC) Office, 2020). However, the barriers of small-scale farmers to apply this system are the lack of capital and knowledge. As a result, Mr. Somboon suggested this system will be efficient if government starts with the large-scale farming (called “ka-set prang yai”) by gathering small-scale farming to collaborate into large-scale farming for reducing the production cost and sharing knowledge with each other.
Another example of successful case is the implementation of "Handy Sense" system by NSTDA mentioned earlier in previous section (NECTEC, 2020a). The system uses sensors to monitor important environmental factors in a greenhouse where farmer can access the information via smart devices in real-time. Moreover, the farmer is able to manually control irrigation or pre-defined schedule watering time. The system also provides optimal environmental values for various crops such as melon tomatoes mango rice and hydroponics vegetables. NSTDA promoted the Handy Sense system throughout the country. One example is Mr. Jittakorn Padetsuk, a vegetable grower in Chachoengsao province. He applied the Handy Sense system in one of his greenhouses. Prior to implementation, he watered vegetables using rubber tube and had problems with fungi due to inconsistency. After using the Handy Sense system, he found that productivity increased by 20 percent while he could save water usage by 5-10 percent and reduce labor force (NECTEC, 2020a). Another example that the authors visited in the field is Mr. Surapol Jarupong, a farmer in Chachoengsao province. He installed the Handy Sense system in tomatoes and melon greenhouses where he also found an increase in productivity. The cost of implementing this Handy Sense system was fully funded by local government as demonstration farms. However, it was estimated to cost around 1,667 USD per greenhouse project. This smart greenhouse system like Handy Sense could be more applicable to small-scale farmers i.e. less than 4 hectares (25 rais).
CONCLUSION
The agriculture sector in Thailand is challenged in many aspects. As a declining average farm size and an increasing number of aging farmers, labor shortage in the agricultural sector tends to rise from the past, while the demand for global food is increasing. Small farmers will have to inevitably switch to smart farming in the future.
Smart farming is a new approach to agriculture which helps solve problems and limitations of population age and farm labor shortage. Smart farming does not only have various advantages to farmers but also have constraints when applying or implementing to the large number of small-scale farmers who are facing aging and lack of technical knowledge as well as lack of capital. Thus, raising the awareness of technology acceptance of farmers is needed. The need of cooperation on the researches, invention, and development of smart farming technologies that are suitable for farmers should be supported by all related agencies of the government, private sectors, and educational institutions. Since small-scale farmers have different characteristics in terms of farm resource endowment and skill of farm managers, the transferring and training should be of major concern.
Moreover, to take smart farming into practice, the government should support farms or farmers that have high and top capability as a priority. In Thailand, there are many smart farmers throughout the country that have a strong network for conducting pilot systems and contributing the lesson to other farms. Besides, technology development should be easy to use for farmers with low cost, and suitable for their knowledge and age.
However, smart farming also faces constraints that could arise like technical unemployment and the knock-on impact on rural populations. The government, therefore, also needs a long-term plan to deal with the problem of unemployment that may occur as a result of the decrease both in working hours and labors.
ACKNOWLEDGEMENT
The authors express gratitude to Mr. Somboon Ngamsangiam and Mr. Surapol Jarupong for field visited and precious information and knowledge of example of smart farming and responses to our questions. Every statement expressed in this article is that of the author and does not reflect any opinions of anyone affiliated to the authors.
REFERENCES
Agriculture and commodity trade chamber. 2019. Sygenta buys agricultural technology firm cropio. https://www.actc.life/syngenta-buys-agricultural-technology-firm-cropio/ Access on 23 November 2020.
Agricultural Research Development Agency (Public Organization) (ARDA). 2020. Smart Farming: Success and challenges of the era. [Online]. Available from http://www.arda.or.th/knowledge_detail.php?id=6. [in Thai]
Attavanich, W., Chantarat, S., Chenphuengpawn, J., Mahasuweerachai, P., & Thampanishvong, K. 2019.
Farms, Farmers and Farming: a Perspective through Data and Behavioral Insights. BOT Symposium Discussion Paper.
Beck, U. 1992. Risk Society: Towards a New Modernity. London, UK: Sage Publications.
Beecham Research.2014. Towards smart farming: agriculture embracing the IoT vision. http://www.beechamresearch.com/files/BRL%20Smart%20Farming%20Executive%2... 2014.
Bronson, K., 2018. Smart Farming: including rights holders for responsible agricultural innovation. Technol. Innov. Manage. Rev. 8 (2), 7–14.
Bronson, K., Knezevic, I., 2016. Big data in food and agriculture. Big Data Soc. 3. https://doi.org/10.1177/2053951716648174
Bureau of Agricultural Economic Research. 2015. Farm efficiency by farm size and farm land holding and income inequality office of Agricultural Economics in crop year 2011/12. Office of Agricultural Economic, Bangkok. [in Thai]
Bureau of Agricultural Economic Research. 1997. Production efficiency by farm type and farm size in crop year 1995/96. (Research report). Bangkok, Thailand: Office of Agricultural Economics. [in Thai]
Bureau of Agricultural Economic Research. 2007. Factor affects labor efficiency in crop year 2004/2005. (Research report). Bangkok, Thailand: Office of Agricultural Economics. [in Thai]
Bureau of Agricultural Economic Research. 2015. Farm efficiency by farm size and farmland holding and income inequality office of Agricultural Economics in crop year 2011/12. (Research report). Bangkok, Thailand: Office of Agricultural Economics. [in Thai]
Carbonell, I. 2016. The Ethics of Big Data in Agriculture. Internet Policy Review, 5. https://doi.org/10.14763/2016.1.405
Carolan, M., 2016. Publicising Food: big data, precision agriculture, and co-experimental techniques of addition. Sociol. Ruralis, 57.
CAT. 2019. CAT brings IoT smart agriculture to Saraburi Wittayakhom School to become a learning center for modern agriculture. [Online]. Available from https://www.cattelecom.com/cat/content/3538/216/CAT+นำ+IoT+หนุนการพัฒนาเกษตรอัจฉริยะให้กับ+รร+สระบุรีวิทยาคม+ก้าวสู่ต้นแบบศูนย์การเรียนรู้เกษตรสมัยใหม่ ?accesEye=on&lang=th_TH. Access on 20 October 2020. [in Thai]
Cohen WM, Levinthal DA. 1999. Absorptive capacity: a new perspective on learning and innovation. Adm Sci Q 1999;35: 128–52.
Driessen, C., and Heutinck, L. F. M. 2015. Cows Desiring to Be Milked? Milking Robots and the Co-Evolution of Ethics and Technology on Dutch Dairy Farms. Agriculture and Human Values, 32: 3–20. https://doi.org/10.1007/s10460-014-9515-5
DTAC. (nd). Smart Farmer program. Available from https://www.dtac.co.th/sustainability/en/project/Project-SmartFarmer. Access on 20 October 2020.
Duang-Ek-Anong.S.,Pibulcharoensit,S.,and Phongsatha, T. 2019. Technology Readiness for Internet of Things (IoT) Adoption in Smart Farming in Thailand. International Journal of Simulation -- Systems, Science & Technology. 2019. Vol. 20 Issue 5, p1-6.
Eastern Economic Corridor (EEC) Office. 2020. EECi: An Innovation City to Transform Country’s innovation into a commerce. Electricity and Industry Magazine. Vol. 5, 2020.
Eastwood. Osipov and L. Riliskis.2013. "Educating innovators of future Internet of Things". IEEE Frontiers in Education Conference. OK, USA, 2013.
Fan S., and Chan-Kang C., 2005. Is small beautiful? Farm size, productivity, and poverty in Asian agriculture. The journal of the international Association of agricultural Economists. 32 (135-146).
Fleming, A., Jakku, E., Lim-Camacho, L., Taylor, B., Thorburn, P., 2018a. Is big data for big farming or for everyone? Perceptions in the Australian grains industry. Agron. Sustain. Dev. 38.
Freeman C, Perez C.1988. Structural crisis of adjustment, business cycles and investment behaviour. In: Dosi G, Freeman C, Nelson R, Silverberg G, Soete L, editors. Technical change and economic theory. London: Pinter; 1988. p. 38–66.
Ge, L., Bogaardt, M.J., 2015. Bites into bits: governance of data harvesting initatives in agrifood chains. Paper Presented at the European Association of Agricultural Economists, The Hague, The Netherlands.
Gubbi J, Buyya B, Marusic S, Palaniswami M. 2013. Internet of Things (IoT): a vision, architectural elements, and future directions. Future Gen Comput Syst 2013;29(7):1645–60.
Kaloxylos A, Eigemann R, Teye F, Politopoulou Z, Wolfert S, Shrank CS, et al. 2012. Farm management systems and the future internet era. Comput Electron Agric 2012; 89:130–44.
Kraipinit, Y. 2018. Study of Program Development for Smart Farmers of Agribusiness Students. [Online]. Available from http://www.polsci-law.buu.ac.th/journal/document/
11-1/22.pdf. Access on 8 October 2020.
Kuhasak, N. and Srichampa, R. 2018. Study of Appropriate Smart Farm Business Model for Small and Medium Entrepreneurs (SMEs) of Melon. [Online]. Available from http://www.journalgrad.ssru.ac.th/index.php/miniconference/article/view/.... Access on 9 October 2020.
Kwanmuang K. 2015. Farmland Inheritance, Transaction and Transference: A Case Study in the Southern Region of Thailand, The Japanese Journal of Rural Economics 17: 46-51.
Kwanmuang, K. 2018. Succession Decisions and Inherited land Size: An Evidence of Family Farms, in Nakhon Si Thammarat Province, Thailand. Applied Economics Journal Vol. 25 No. 2 (Demember 2018): 70-88.
Lehmann RJ, Reiche R, Schiefer G. 2012.Future internet and the agri-food sector: state-of-the-art in literature and research. Comput Electron Agric 2012; 89:158–74.
Logindarat, P. 2011. “pon kratob kon kan pranprang krongsart pracharkon pakkasart teme tor kwammunkong nai kan palit arhan kon pakkasartthai (Effecting of changing agricultural population structure on food security research project in Thailand),” TRF Policy Brief journal, Vol.4, 2011.
Manwicha J. 2015. Smart Farm Technology. Hatyai Academic Journal 14(2): 201-210. [in Thai]
Millar, K. M. 2000. Respect for Animal Autonomy in Bioethical Analysis: The Case of Automatic Milking Systems (AMS). Journal of Agricultural and Environmental Ethics, 12(1): 41–50. https://doi.org/10.1023/A:1009548025408
Ministry of Agriculture and Cooperatives (MOAC). 2019. Smart Farming Action Plan during 2020 to 2022. [Online]. Available from https://www.alro.go.th/research_plan/
ewt_dl.php?nid=1218&filename=index. [in Thai]
Ministry of Agriculture and Cooperatives. 2019. Transforming agriculture Ministry of Agriculture and Cooperatives push “smart tomatoes greenhouse”. [Online]. Available from https://www.opsmoac.go.th/datacenter-news-preview-411691791322. Access on 20 October 2020.
National Electronics and Computer Technology Center (NECTEC). 2020. Handy Sense precision farming system - Smart Farm from Thai farmers’ understanding. [Online]. Available from https://www.nectec.or.th/news/news-article/handy-sense-interview.html. Access on 17 October 2020.
National Science and Technology Development Agency (NSTDA). 2020. Technology demonstration and showcase of smart farming technologies at Maejo University. [Online]. Available from https://www.nstda.or.th/en/news/news-years-2020/971-technology-demonstration-and-showcase-of-smart-farming-technologies-at-maejo-university.html. Access on 20 October 2020.
National Science and Technology Development Agency (NSTDA). 2020. Translating “Sense” to “Data” to improve quality of durian farm. [Online]. Available from https://www.nstda.or.th/agritec/durian-smart-tech/. Access on 20 October 2020.
Office of Agricultural Economics 2016. Agricultural economics indicator of Thailand. Bangkok, Thailand: Office of Agricultural Economics. [in Thai]
Office of Agricultural Economics. 2015. Agricultural economics indicator of Thailand. Bangkok, Thailand: Office of Agricultural Economics (in Thai).
Otsuka K., Liu Y., and Yamauchi F. 2016. Growing advantage of large farms in Asia and its implications for global food security. Development of Policy Review, 34(3):441-461.
Pattama N. 2020. The development of Smart Farming Systems in Thailand. [Online]. Available from https://www.senate.go.th/document/Ext23700/23700529_0008.PDF.
Penrose ET.2009. The theory of the growth of the firm. Oxford: Oxford University Press; 2009.
Perez C. 1988. New technologies and development. In: Freeman C, Lunvall BA, editors. Small countries facing the technological revolution. London: Pinter Publishers; 1988. p. 85–97.
Phonpho, T. and Phonpho, K. 2013. Development of automatic nursery seeding machine. [Online]. Available from http://www.repository.rmutt.ac.th/bitstream/handle/123456789/1778/. Access on 9 October 2020.
Poapongsakorn, N., Ruhs, M. and Tangjitwisuth, S.1998. “Problems and outlook of agriculture in Thailand,” TDRI Quarterly Review, Vol.13, No.2, 1998, pp.3-14.
Samseemoung, K. and Sangpradit, K. 2014. Design and Fabrication of high performance vegetable seeder of Brassica alboglabra for seeding trays. [Online]. Available from https://li01.tci-thaijo.org/index.php/thaiagriculturalresearch/article/v.... Access on 8 October 2020.
Schumpeter JA, Garcia JD. 1983. Capitalismo, socialismo y democracia. Barcelona: Orbis; 1983.
Sentangsedtee. 2019. AIS partners with Uncle Ree Farm to apply IoT platform i-Farm for urban farming. [Online]. Available from https://www.sentangsedtee.com/today-news/article_134891. Access on 20 October 2020.
Siam Kubota Corporation. n.d. About KUBOTA (Agri) Solutions. [Online]. Available from https://www.kubotasolutions.com/about/definition. Access on 20 October 2020.
Sorensen CG, Fountas S, Nash E, Pesonen L, Bochtis D,Pedersen SM, et al. 2010. Conceptual model of a future farm management information system. Comput Electron Agric 2010;72(1):37–47.
Sriamnouy,B. Plangsorn,S. Piticharoenpon,V., Srihanam,M. 2019. Study of Smart Farming Design by Using Internet of Things for Lime Farm in Phetchaburi Province. The 6th National Conference Nakhonratchasima College. [Online]. Available from http://journal.nmc.ac.th/th/admin/Journal/2562Vol9No1_89.pdf. Access on 8 October 2020.
The Reporter Asia. 2020. TRUE Cooperation joins Khon Kaen University to develop smart agriculture [Online.] Available from https://www.thereporter.asia/th/2020/02/03/true-5g-2/. Access on 18 October 2020.
Verónica Saiz-Rubio and Francisco Rovira-Más .2020. From Smart Farming towards Agriculture 5.0: A Review on Crop Data Management. Mdpi agronomy review.
Wattanutchariya, S. and Jitsanguan, T. 1992. Increasing the scale of small-farm operations I. Thailand. Retrieved from http://www.agnet.org/library.php?func=view&id=20110726143050.
World Economic Forum. 2016. The Future of Jobs. Geneva: World Economic Forum (WEF). [Online]. Available from https://www.weforum.org/reports/the-future-of-jobs
Wolfert, S., Ge, L., Verdouw, C., Bogaardt, M.J., 2017b. Big data in smart farming – a review. Agric. Syst. 153, 69–80.
World Bank. 2017. Research and development expenditure (% of GDP). [Online]. Available from http://data.worldbank.org/indicator/GB.XPD. RSDV.GD.ZS; 2017.
Yanmar. n.d. SMARTASSIST (SA-R) system by Yanmar. [Online]. Available from https://www.yanmar.com/th/news/2020/05/29/74726.html. Access on 20 October 2020.
Yungyuen, K. 2020. The Responsible Agriculture Investment Policy to create Social Welfare and Food Security. Journal of Social Synergy, 11(1), 32-61. [Online] Available from https://so04.tci-thaijo.org/index.php/thaijss/article/view/242029.