ABSTRACT
Smart agriculture is used to enhance agricultural productivity, address agricultural-based issues, such as food demand, and make farms more connected and intelligent. The market value of smart agriculture worldwide is forecasted to reach around 26.76 billion U.S. dollars by 2020. Smart agriculture incorporates Internet of Things (IoT) technology and solutions in order to attain improved operational efficiency, maximized yield, and minimized wastage through the use of real-time field data collection, data analysis, and the deployment of control mechanisms. Smart agriculture employs a range of IoT-based applications such as precision farming, variable rate technology, smart irrigation, as well as smart greenhouses. Other applications of IoT in smart agriculture include: Variable Rate Technology (VRT), agriculture drones, soil monitoring systems, and precision livestock farming. Telecom operators benefit a lot from smart agriculture as cellular connections account for by far the largest share of communications systems in smart agriculture worldwide. Precision farming is set to become the most influential agricultural trend in developed countries. The market value of precision farming is also expected to grow from 730 million U.S. dollars in 2015 to 2.42 billion U.S. dollars by 2020. A look at the distribution of precision farming worldwide shows North America as the major player with market value estimated at to 1.2 billion Euros in 2014. The main drivers of smart agriculture include: the realities of a changing climate, a need to conserve water, and its emphasis on the enhancement of efficiency through resource optimization and cost minimization.
Keywords: Smart agriculture, Internet of Things (IoT), Precision farming, Variable Rate Technology (VRT), Agriculture drones
INTRODUCTION
Smart agriculture is a farming management concept using modern technology to increase the quantity and quality of agricultural products. Farmers in the 21st century have access to Global Positioning System (GPS), soil scanning, data management, and IoT technologies. By precisely measuring variations within a field and adapting the strategy accordingly, farmers can greatly increase the effectiveness of pesticides and fertilizers, and use them more selectively. Similarly, using smart agriculture techniques, farmers can monitor better the needs of individual animals and adjust their nutrition correspondingly, thereby preventing disease and enhancing herd health.
Knowledge and capital are essential for any innovation. New farming technologies require more and more professional skills. A farmer today is not only a person with a passion for agriculture, he or she is also a legal expert (to find their way through a growing maze of regulations) and a part-time data analyst, economist and accountant (making a living from selling agricultural produce requires bookkeeping skills and an in-depth knowledge of market chains and price volatility).
Furthermore, smart agriculture requires capital. Thankfully, there are a wide range of options available. From using low capital investment smart phone applications that track your livestock to a capital-intensive automated combine. In principle, implementing smart agriculture technologies can be easily upscaled.
Modern farms and agricultural operations work far differently than those a few decades ago, primarily because of advancements in technology, including sensors, devices, machines, and information technology. Today’s agriculture routinely uses sophisticated technologies such as robots, temperature and moisture sensors, aerial images, and GPS technology. These advanced devices and precision agriculture and robotic systems allow businesses to be more profitable, efficient, safer, and more environmentally friendly.
Farmers no longer have to apply water, fertilizers, and pesticides uniformly across entire fields. Instead, they can use the minimum quantities required and target very specific areas, or even treat individual plants differently. Benefits include higher crop productivity, decreased use of water, fertilizer, and pesticides, which in turn keeps food prices down, reduced impact on natural ecosystems, less runoff of chemicals into rivers and groundwater and increased worker safety
In addition, robotic technologies enable more reliable monitoring and management of natural resources, such as air and water quality. It also gives producers greater control over plant and animal production, processing, distribution, and storage, which results in greater efficiencies and lower prices, safer growing conditions and safer foods and reduced environmental and ecological impact.
IoT IN SMART AGRICULTURE
ITU (International Telecommunication Union) defined the Internet of Things as: “A technology that mainly resolves the interconnection between human to a thing, thing to thing, and human to human.” IoT is a world-shattering technology that signifies the future of computing and information interchange (Patil et al. 2012). It is based on the communication between intelligent sensors, RFID (radio-frequency identification), GPS, infrared sensors, remote sensing (RS), mobile communication, and other communication networks (Dlodlo and Kalezhi 2015). It refers to a network of objects and is often a self-configurable wireless network (Patil et al. 2012). The basic purpose of IoT is to make a huge network by the combination of diverse sensor devices such as GPS, RS, RFID, laser scanner, and networks to comprehend the information sharing of global things. IoT can encompass millions of networked embedded smarts devices also called smart things; these smart things are capable of accumulating information about themselves, their environment, and associated smart devices and interconnect this information to other devices and systems via the all connecting Internet (Fig. 1) (Yan 2011).
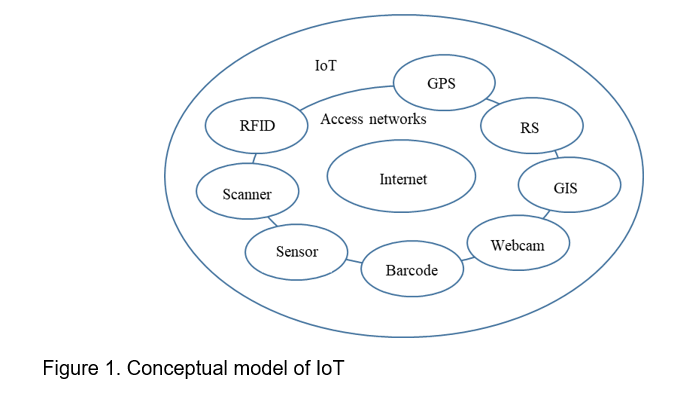
Precision agriculture
Precision agriculture refers to a suite of technologies that may reduce input costs by providing the farm operator with detailed spatial information that can be used to optimize field management practices (National Research Council 1997; Schimmelpfennig 2016). The adoptions of precision agriculture technologies have, so far, mostly been limited to parts of developed countries. In Australia, by 2013, 90
%of farmers had been using some type of precision agriculture, and 20 % of farmers had been using yield mapping and variable rate fertilizer application (Bramley and Trengove 2013). In Germany, about 10-30 % of farmers were using some form of precision agriculture in 2016 (Paustian and Theuvsen 2017 - cited in Taylor 2018). Keskin & Sekerli (2016) provides related statistics in some other developed countries. In the United States, the use of precision agriculture started growing in the 2000s, and spread considerably, although at varying rates across crops and the types of precision agriculture (Schimmelpfennig 2016). In 2010 in the United States, yield monitoring has spread to almost 70 % of maize and soybean areas, while the adoptions of variable-rate technology have also reached about 30 % of the areas.
In the United States, the progress on precision agriculture has varied across crops. Yield monitoring for cotton lagged behind grain yield monitoring (Watcharaanantapong et al. 2014). This is partly because of equipment problems associated with accuracy, calibration and maintenance of optical sensors; for example, compared to those for maize, development of reliable and accurate cotton yield monitors did not occur until 2000 when yield monitors had already been used on about one-third of corn and soybean area in the Corn Belt, respectively (Schimmelpfennig and Ebel 2011; Watcharaanantapong et al. 2014). Furthermore, even in the US, adoption rates are positively correlated with farm size. For example, the largest corn farms in the country, those over 2,900 acres, are twice as likely to adopt precision agriculture techniques as the average farm (Schimmelpfennig 2016; Taylor 2018). Precision-agriculture technologies for corns, which have spread substantially in the United States recently, are still not adopted by 88% of farmers cultivating less than 600 acres (Schimmelpfennig 2016).
In developing countries, the use of precision agriculture has been limited. In Malaysia, site-specific fertilization is being applied to rubber plantations, and other precision technologies have started being applied for oil palm, although not to rice fields (Mondal and Basu 2009). In Turkey, recently, international companies like Topcon sold about 60 auto-steering system in the Southern part of the countries along Mediterranean regions (Keskin and Sekerli 2016). The service providers of auto-steering service set up own reference station networks for positioning correction, and some farmers owning automatic steering use them to serve other farmers mainly in ridge tillage in cotton and corn cultivation (Keskin and Sekerli 2016). Similar service dealership related to precision agriculture is observed in Brazil as well (Borghi et al. 2016). Similarly in Turkey, an international company (New Holland) is planning to install yield monitors on about 500 combine harvesters countrywide in the last few years (Keskin and Sekerli 2016). In India, technologies related to precision agriculture that have been adopted include drip and sprinkler irrigation, which have been growing steadily, although still accounting for only a fraction of irrigated areas in India (Kalubhai 2015). Similarly, while similarly accounting for a tiny fraction of areas within India, adoptions of laser-land-leveling technologies have been growing in parts of India, including Western Uttar Pradesh and Haryana (Tiwari and Jaga 2012).
Precision livestock farming
“Monitoring animals within groups is challenging, even for the best herdsmen” NPB—Animal Science Committee” (Hostetler et al. 2018). Precision livestock farming implies automated remote detection and monitoring of identified individuals for animal health and welfare using real-time analysis of images, sounds, tracking data, weight and body condition, and biological metrics in livestock (Berckmans 2014, Neethirajan 2017, De Montis 2017). With that, there is the capacity for early detection of illness or physiological status at the farm level. While not a new science (DeShazer et al. 1988), the information, applications, and availability of precision livestock farming has greatly increased due to computer science expertise and inexpensive sensors off-labelled from the video gaming industry (Xbox, PlayStation R, etc.) combined with the growing computer capacity for capturing and processing data (Nasirahmadi et al. 2017, Berckmans 2014, Banhazi et al. 2012).
In order to better understand how current technology may impact the world of precision livestock farming, a basic understanding of remote monitoring sensors, the process of algorithm development and machine learning is required. Remote sensors such as cameras, microphones, thermometers and accelerometers monitor or capture information such as images, sound, heat or motion from groups or individual animals. The data from the sensors, stored in external drives or sent directly to a processing node (analogous to transferring photos from a digital camera to a computer) are then processed by algorithms. An algorithm is a formula, or step-by-step set of operations, used to solve a specific problem or class of problems. A programming algorithm is a computer procedure that tells a computer precisely what steps to take to solve a problem utilizing inputs to determine the outputs. Programmers initiate the process by writing the algorithm that instructs the computer how to perform the specific operations necessary to solve a problem. An algorithm’s value to farmers is dependent on its ability to transform the sensor data or “feature variable” to a biological outcome. Examples of feature variables include percentage of time livestocks are lying down to determine the biological outcome “lameness” or the number of coughs to detect the biological outcome “respiratory disease”. Machine learning is a family of computational methods that allows an algorithm to program itself using large sets of examples. Because the computer “learns” from these example sets of existing data, a system can become highly adept at processing and analyzing large data sets to track variables and produce estimates at a rate that would not be possible for humans or traditional statistical methods (Puri et al. 2015). Taken together, data from remote monitoring sensors is combined with individual animal identification, referenced observations and production data, and then integrated in algorithms to provide credible information and alerts regarding livestock welfare, health and productivity.
Individual identification methods, either currently used in the swine industry or research, include radio frequency identification, optical character recognition, and facial recognition. A well-established technology for livestock identification, health purposes and management on farms is the radio-frequency identification (RFID) chip (Ariff et al. 2014). The device is primarily implanted in ear tags; it stores information such as animal and farm records. The radio wave (low, high or ultra-high frequency) is the medium of communication between the transponder circuit within the tag and an RFID reader to wirelessly read and write data. The working principle of such a device is that when an RFID tag comes within range of an RFID reader, it receives a signal. Furthermore, a second radio frequency signal is induced, carrying data that travels to the reader (Ariff et al. 2014). These data can be stored and analyzed later, or the RFID chips can be used immediately to identify individual animals. Low frequency (LF)—RFID is a valuable component of groups housing electronic sow feeders; these small feeder enclosures fit one sow at a time and dispense a specific limited diet to the sow while also collecting identification data and feeding frequency via RFID (Maselyne et al. 2015). Infrequent visits of certain sows could raise an alert to producers so that health issues or undesirable social behaviors may be addressed early in their progression (Cornou et al. 2008). Nevertheless, LF-RFID has two major disadvantages: a low read range (<1 m), and the inability to identify more than one animal (Adrion et al. 2017) within range. In order to track multiple animals at a greater range (3 to 10 m), researchers have investigated the commercial feasibility of Ultra-High Frequency (UHF) readers (Sales et al. 2015). While promising, the UHF-RFID transponder ear tags are sensitive to interference from ear tissue, leading to false registrations (Brown-Brandl 2018), thus requiring further research to adapt the technology for consistent use in animal identification. Other drawbacks to RFID include loss or attrition of tags, pain and stress of the animal during tagging and required removal from the animal prior to slaughter processing.
A low-cost identification system, optical character recognition, is the recognition of printed, stamped, or written text characters (e.g., license plates, barcodes, and QR codes) by a computer. In livestock production, optical recognition includes characters on ear tags or painted symbols and numbers. Optical character recognition is performed with a digital camera and data is developed with machine learning to provide remote identification (Mittek et al. 2017). Depending on the clarity and color of the markings, there is the capacity to identify large permutations of animals and, with the exception of optical character recognition on tags, the identification may not need to be removed from livestocks prior to slaughter (Mittek et al. 2016). When the characters are painted, visual identification patterns can fade within a day and when livestocks lie close to or on one another, pattern recognition is occluded (Lancaster et al. 2012).
The development of a new technology for precision livestock farming requires a deep understanding of the specific task to identify what type of sensor best suits those needs. There are various ways in which to design and implement such new devices. Single sensor designs are easier to integrate and develop, but are restricted within sensor capabilities. Implementations which include multiple sensor types come with an added benefit of higher accuracy and robustness, but at the cost of the design complexity of sensor fusion to create a joint signal for processing. Validation of data and how to identify the accuracy or precision of such measurement or prediction requires background knowledge in statistics and probability (Kay 1993). In a practical setting, human observations can be taken from well-trained (experts) individuals and can be compared to device results to quickly assess performance. For fine accuracy, statistical methodologies such as least squares and maximum likelihood estimation can be performed on the data to evaluate accuracy. Standard engineering design processes such as preliminary hazard analysis, design failure mode and affect analysis, and design reviews should be utilized especially within farm settings where possible hazards are not accounted for, resulting in performance loss. Having close communication with farms and being able to test and prototype designs will help identify what design constraints are necessary for a successful device.
Variable rate technology
One of the components of precision agriculture concept is Variable Rate Control (VRC) that implements management decisions at right time and place. VRC is a process of applying varying rates of inputs (water, fertilizer, pesticides etc.) at proper zones throughout the field in an optimized way. This optimum utilization of resources helps to maintain economic profit ensuring sustainability and environmental safety. The technology used for variable rate control is known as Variable Rate Technology (VRT) (Council 1997). VRT are briefed in Table 1. From this table it is clear that most of the literatures concentrate on Variable Rate Fertigation (VRF). Very little work is done on the combination of four types of VRT – Variable Rate Irrigation (VRI), VRF, Variable Rate Pesticide (VRP), and Variable Rate Herbicide (VRH). It inspires the researchers to develop a single control unit that considers the correlation among different field and weather parameters and develop a combined VRT. This combined VRT can apply water, fertilizer, pesticide, and herbicide at a prescribed rate with a centralized controller.
Table 1. Technology used in reviewed literature for variable rate control.
References
|
|
Technologies
|
|
VRF
|
VRP
|
VRH
|
VRI
|
(Zheng et al. 2013, Chattha et al. 2014, Reyes et al. 2015, Ying-zi et al. 2015)
|
√
|
X
|
X
|
X
|
(Jones et al. 2016)
|
X
|
√
|
√
|
X
|
(Kargar and Shirzadifar 2013)
|
X
|
X
|
X
|
X
|
(Zhang and Song 2014)
|
√
|
X
|
X
|
√
|
(Partel et al. 2019)
|
X
|
X
|
√
|
X
|
(Weber et al. 2018)
|
X
|
√
|
X
|
X
|
Soil monitoring systems
Soil moisture and climatic conditions are the two most important factors in agriculture productivity. During irrigation, water wastage should be avoided. The plants or crop should be irrigated only when they need to be. When plants transpire more amount of water, the relative humidity of atmosphere increases. This presence of large amount of relative humidity increases the chances of disease attack. So, soil moisture levels in the field require periodic inspection, from where one can determine, when the next irrigation should be done and how much amount of water should be applied. Current developments in the miniaturization of electronic devices and wireless communication technology have resulted in the emergence of an energy efficient WSN. This makes it possible to acquire the field information more timely, accurately and conveniently. Monitoring parameters of temperature and humidity is an important means for obtaining high-quality environment. Remote monitoring is an effective method in order to avoid interference environment and improve efficiency. Today, Ethernet network and ZigBee wireless networks are used to transmit data in remote monitoring System. The IoT is a system which integrates the computing devices, possessing the ability of transferring the data over the network via the better route. (Dhumane et al. 2017). A review of remote control and monitoring systems based on existing technologies and a ZigBee based IoT network to allow efficient routing in the network was given.
The opportunity to design a device that monitors soil moisture, temperature and humidity of field atmosphere, and transmit the information to the remote receiver at the farmhouse or outside the field was taken here. The remote receiver is a laptop connected to the Zigbee transceiver. (Zhang et al. 2009) The proposed system consists of Arduino as processing unit and WSN base station. A soil moisture and temperature humidity sensor is connected to the WSN data collection node. The sensor node also consists of a LCD module, where the sensor output is displayed in real time. The sensor node is building block of the WSN. The task of sensor node is to achieve the perception, collection, processing and wireless transmission. The sensor node converts the physical quantity to the voltage signal and Arduino UNO board controls the processing, and manages the communication protocol. The sensor node communicates with the base station using transceiver. The base station collects all the data send by sensor node. The whole system is made with the collaboration of hardware and software components. The hardware components include Arduino UNO board, soil moisture sensor, DHT11 temperature humidity sensor, LCD module and Zigbee transceivers. The software includes Arduino IDE and X-CTU.
Smart irrigation
With the changes in technologies and rapid consumption of natural resources by humans, it is vital to implement smart irrigation techniques to overcome the scarcity of water resources in near future. Smart irrigation using wireless sensors for precision agriculture, drip irrigation automation system for water level monitoring, are discussed by considering various factors like soil moisture, humidity, and temperature in (Gajendran et al. 2017). In (Al-Ghobari et al. 2017), couple of irrigation tools, SmartLine and Hunter Pro-C2, are used to achieve water savings and better crop yield. Here, Hunter Pro-C2 has realized better economic benefits and large amount of irrigation water is saved. Unplanned usage of water and irrigation with automatic valve mechanism based on the soil moisture is illustrated in (Tyagi et al. 2017, Akubattin et al. 2016) which minimizes manual intervention of farmers for crop irrigation. In (Ranade and Takale 2016), a framework for efficient water distribution management is defined using wireless sensor network (WSN) and field-programmable gate array (FPGA), where data is transmitted using ZigBee and based on which control of motor on/off conditions is determined.
The above mentioned research efforts majorly focused on illustrating smart irrigation techniques by using various irrigation tools, automating irrigation systems using actuators, and sensors for minimizing the water consumption in crop irrigation and to achieve better crop yield. But it is vital that these smart irrigation techniques must be extended for preserving water generated from rainfall which will help to increase the groundwater levels in agricultural farms and to enable farmers to save crops from unforeseen rainfall by integrating farms with real-time weather conditions. A framework based on cloud and Internet of things to implement a smart irrigation system that saves crops during unforeseen rainfall, increases groundwater levels
with a competent mechanism, and to reuse excessive water generated during rainfall for crop irrigation was proposed. Based on the defined framework, a use case for smart irrigation system is developed which extends the features depicted in (Tyagi et al. 2017, Akubattin et al. 2016, Ranade and Takale 2016) where agricultural farms are closely monitored based on real-time weather conditions and soil moisture. Also, a competent mechanism is defined to avoid rainwater depletion, utilize excessive water generated from rainfall for crop irrigation, and save farms during unforeseen rainfall.
Early warning system
There is a great deal of the Android applications alert system and agriculture in the literature. The system in (Sharma 2013) uses a mobile phone to detect the activities around the farm. The system will collect the data through the mobile phone (GPS, accelerometer and microphone sensor) carried by the farmers. By obtaining the GPS coordinates, the system can obtain the statistics of the working pattern of any farm worker and frequency of visit to a certain area (different plots) of the farm. The work in (Rachana and Suhas 2014) uses of Android mobile to increase productivity of crops and indirectly increase the knowledge of the farmer society.
In (Dominic 2010), the Android apps make use of the alerts published by the Global Disaster Alert and Coordination System (GDACS). The app shows icons on the map with color green, orange and red, each mapping the severity of the calamities. StormTracker (WSI 2008) makes use of the NOWrad (National Operational Weather radar) which gives one of the best resolution cloud imagery with color codes. It also gives 10-day forecasts with both daily and hourly details. Users can also lock their favorite locations to keep track of the local area. WebGIS (Geographic Information System) Expert System for Rice Brown Hopper Disaster Early-Warning (Chen et al. 2008) can perform disaster evaluation and disaster early-warning. The WebGIS is known for its features to locate and mark area in large scale. It is used to relate how the insects are spreading on the fields.
Most importantly, the farmers need to know about the current situation so that they can react before the insects spread out. Early Detection and Distribution Mapping System for the Southeast Exotic Pest Plant Council (Bargeron and Moorhead, 2007) was developed to fill up the species distribution of invasive plant throughout South America. The location of the data are various, in different formats, and unfitting to produce an overall map. The use of Google Map, instead of the flash map Area Selector and Adobe Flash technology has solved the problem of combining the available data from different locations.
Agriculture drones
Remote sensing techniques utilizing Unmanned Aerial Vehicles (UAVs) are in constant development and the use of tiny MEMS sensors (accelerometers, gyros, magnetometers, and often pressure sensors), small GPS modules, powerful processors, and a range of digital radios makes drones small, low cost and quite easy to use. In just under five years, UAVs have gone from a toy for gadget junkies to an essential tool in many application fields; today, agriculture is one of the fastest growing markets for the commercial UAVs industry. In 2014, the Massachusetts Institute of Technology classified agricultural drones at the primary position among the ten breakthrough technologies (Anderson 2014), helping the farmer in a better utilization of the land. As an example of drone utilization in farming in the wine production sector, multispectral cameras mounted on drones have highlighted the close correlation between the quality of the grapes and the health of the plants. Another example of drone utilization is the reduction in waste of water around a farm: drones can be fitted with remote sensing equipment (multispectral, hyperspectral or thermal sensing systems) in order to quickly and easily identify the driest sections of a field, thus allowing farmers to allocate their water resources more economically. Even the amount of chemicals ground penetration for fighting pest and fungal infestations can be reduced if drones effectively scan the ground of a farm and spray the correct amount of chemical substance modulating the distance from the ground. Moreover, a drone can survey a crop at any requirement of the farmer, thus showing any dangerous changes or other events of interest.
Drones, or UAVs, were first introduced during WWI for enemy surveillance. They are small, radio-controlled airplanes that can be used to take pictures. Nowadays, their use has expanded to small-time hobbyists and is quickly expanding to use in agriculture. Military drones are much larger than ones used by civilians. Crop scouting drones are small enough to be brought to the field in the back of a pickup, often under 15 pounds. The economic impact in the few years after regulations are implemented is predicted to be around $13 billion and 7500 commercial drones by The Association for Unmanned Vehicle Systems International, with 80 to 90% of the growth in the market coming from agricultural use (Karst 2012). The two main designs for UAVs are fixed-wing airplanes and rotary motor helicopters. Each of these has its own benefits. The fixed-wing drones are able to fly at a higher speed, 25 to 35 mph, while still taking pictures. It can cover 600 acres per hour (Patrico 2013), which is how long the charge on the battery lasts. Fixed-wing airplanes have to be thrown or launched into the air and need a large flat area to land upon. A parachute can be used in confined conditions, but that will severely reduce the life of the airplane. Luckily, replacement parts are easily available if the fuselage gets cracked. Rotary motors are able to hover and focus in on specific problem spots in a field while sacrificing maximum speed. They have a little less battery life because they often have to run four engines or more. The takeoffs and landings can be done in more confined areas, so they are better for beginner flyers.
Another aspect of the system is the ground control station. This is where the operator stands and monitors the flight and data coming from the drone. Some systems are simple enough to be operated from a smart phone. A number of different software programs are available to analyze the data. More important ones stitch together the separate images into one smooth picture and then can incorporate the image into GIS systems. Afterward, variable-rate applicators can use those pictures to find portions of the field that are low on nutrients or pressured by weeds. The drones can be manually flown, or pre-determined flight paths can be programed with GPS data. Learning to pilot the planes takes only a few hours, or they can be programed with one touch takeoff and will fly without any steering from the ground. Self-leveling programming can be installed to adjust for wind and to stabilize the images.
Drones are fairly cost effective and can pay for themselves in just a few uses. The cost of these UAVs can range from as little as $1000 for a starter system and can go up to $10,000 or $20,000, depending on the size of the machine and any extra cameras or features added. These systems are designed to have easily replaceable parts in case of crashes. Compare that to the several hundred dollars per hour it costs to have a piloted airplane fly over a field and it does not take long to cover the cost (Anderson 2014). The money saved by targeting areas for fertilizer and pesticide applications can be figured into the price as well. Plus, it benefits the environment to not over apply chemicals to areas that do not need it.
CLIMATE-SMART AGRICULTURE
Climate-Smart Agriculture (CSA) is an approach to help the people who manage agricultural systems respond effectively to climate change. The CSA approach pursues the triple objectives of sustainably increasing productivity and incomes, adapting to climate change and reducing greenhouse gas emissions where possible. This does not imply that every practice applied in every location should produce “triple wins”. Rather the CSA approach seeks to reduce trade-offs and promote synergies by taking these objectives into consideration to inform decisions from the local to the global scales and over short and long time horizons, to derive locally-acceptable solutions.
The majority of the world’s poor live in rural areas and agriculture is their most important income source. Developing the potential to increase the productivity and incomes from smallholder crop, livestock, fish and forest production systems will be the key to achieving global food security over the next twenty years. Climate change is expected to hit developing countries the hardest. Its effects include higher temperatures, changes in precipitation patterns, rising sea levels and more frequent extreme weather events. All of these pose risks for agriculture, food and water supplies. Resilience is therefore a predominant concern. Agriculture is a major source of greenhouse gas emissions. Mitigation can often be a significant co-benefit of actions to strengthen adaptation and enhance food security, and thus mitigation action compatible with national development priorities for agriculture is an important aspect of CSA.
Smart greenhouses
Greenhouses are widely used to produce food in USA, UK, and the Netherlands, but in India, greenhouse technology is still at a nascent stage. By employing greenhouses, food production can be increased by utilizing even the arid and non-fertile lands, thus increasing the yield. Having better control over the processes and maintaining a favorable atmosphere for the crops leads to high quality yield by reducing the wastages. Many systems were proposed for automating certain processes of the greenhouse and to provide remote monitoring of the parameters. Global System for Mobile Communications (GSM)-based systems that provide notifications through short message service (SMS) (Huang et al. 2011) and Zigbee-based wireless sensor network (WSN) (Li et al. 2014) were explored. Certain other systems used android application to provide remote monitoring (Xiaofeng et al. 2015). Some previous studies were also based on Bluetooth technology (Gang 2006).
A relatively new technology, LoRa, is studied and implemented in a farming scenario. Though the concept of greenhouse farming is not adopted on a large scale in India, several countries have benefitted immensely from its usage. Such modern farms can sprawl for hundreds of acres. But the system can also be implemented for small-scale farms. Between efforts to eat more food grown locally, a younger generation of farmers and cheaper component-farming is getting an infusion of data and technology. As the concept of the ‘Internet of Things’ becomes increasingly prevalent, many systems are being devised to allow all manner of data to be gathered and analyzed, and devices controlled via wireless data networks. Connected devices such as smart thermostats and lighting systems are making their way into homes, but another big opportunity for the IoT could be outdoors, in agriculture.
Greenhouse though concept up to speed internationally, the Indian farming economy is only actively enrolling itself in the recent times. Initial automation efforts in the farming sector include the automatic drip irrigation systems, which were developed to water the crops automatically based on the soil moisture level. But such systems automated only one process, and other processes were still monitored and controlled manually. Important parameters to be monitored and controlled in a greenhouse include soil moisture, temperature and humidity and lighting, and it is vital that all the factors are considered. Some of the previous systems developed include an android application to monitor the greenhouse but did not include mechanisms to control it (Luo et al. 2012, Xu et al. 2016). One of them was based on GSM in which notifications are sent via SMS. The drawback to this system was that human presence is required to control the parameters. Other systems using AT8051 microcontroller core were proposed which led to some automation and automatic control, but it was complicated to interface the microcontroller to a wireless network for cloud integration. Bluetooth-based systems had a short range and could not be used for long-distance communication.
Another option explored in this context was the Zigbee-based WSN. This has been widely adopted for farm monitoring and irrigation systems. WSN employing Zigbee protocol stack was efficient and led to complete automation of the process but was often too expensive. Though the WSN-based model boasts diverse nature and scalability, it poses limitations in terms of large area deployment and cost, for farming solutions. A system designed using WSN has a shorter range of coverage. A Zigbee coordinator can support only a few hundred nodes. A possible solution to overcome the above-discussed downfalls of the existing systems in agriculture was proposed. This system comprises of sensors and actuators which would be programmed by using an Mbed board which can aid the overall process of agriculture by automating it and helps keep the farmer updated by sending live feeds via the Internet. The technology of LoRaWAN is used here, as it provides features such as low power and wide range which are desired for this application. The LoRa technology is to provide ranges between 5 and 10 km, and its average battery life is about 5–11 years. This is highly desirable in farming considering the vast area of implementation of the system. LoRaWAN allows around thousand nodes to be attributed to one gateway (Georgiou and Raza 2017). The battery life and its low power consumption also make the system implementation more economical.
Smart fertilizers
Slow-/controlled-release fertilizers
According to Trenkel (1997), slow- or controlled-release fertilizers are those containing a plant nutrient in a form, which either (a) delays its availability for plant uptake and use after application, or (b) is available to the plant significantly longer than a reference “rapidly available nutrient fertilizer” such as ammonium NO - or urea, ammonium phosphate or potassium chloride (AAPFCO, 1995). There is no official differentiation between slow release and controlled-release fertilizers. However, the microbially decomposed N products, such as urea-formaldehydes, are commonly referred to as slow-release fertilizers, and coated or encapsulated products as controlled-release fertilizers (Trenkel, 1997). Delayed availability of nutrients or consistent supply for extended time periods can be achieved through a number of mechanisms. These include semipermeable coatings for controlled solubility of the fertilizer in water, protein materials, occlusion, chemicals, slow hydrolysis of water-soluble compounds of lower molecular weights, and some other unknown means (Naz and Sulaiman 2016).
Other options include utilization of semipermeable materials and sensors of chemical or biological origin within the fertilizer. These are advanced materials, whose physical or chemical properties can change in response to an external stimulus such as temperature, pH, and electric or magnetic fields (Foster, 2013; Mastronardi et al., 2015; Roy and Gupta, 2003). Many coating materials can be used to slow nutrient release, including natural materials such as clays and nanoclays (e.g., allophane), and nondegradable (polysulfone) and biodegradable polymers (e.g., alginate beads). Several studies have shown that urea-based coatings can have variable efficiencies depending on the material used (Du et al., 2006; Naz and Sulaiman, 2016; Shaviv, 2005).
In addition, they can be highly expensive in some cases, pollutants, or toxic (i.e., polymer-coated urea) and mostly difficult to degrade with potential environmental impacts (accumulation) as the major concern. As a consequence, formulation with environmentally safe and biodegradable coating materials seems to be necessary (Ni et al., 2009). In this sense, natural polymeric carbohydrates appear as an alternative to nonbiodegradable materials acting as permeable or impermeable membranes with tiny pores in slow-release fertilizers (e.g., urea) (Butzen 2013). These highly degradable materials have also received attention because of their low cost and low environmental damage due to biodegradability and low accumulation in the environment (Naz and Sulaiman, 2016). However, these polymers may need some modifications to be included into the coating design because of their hydrophilic properties and their weak coating barrier, for example, in the case of starch.
Biofertilizers
One group of microorganisms beneficial for plant growth is PGPR, a heterogeneous group of bacteria that can be found in the rhizosphere, at root surfaces, and in association with roots (Ahmad et al., 2008). These bacteria have several functions, including production and regulation of phytohormones, release of nutrients to plants (e.g., P-, N-fixation, siderophores, among others), and control of phytopathogens (production of antibiotics and siderophores) (Egamberdieva and Adesemoye 2016; Martı´nez-Viveros et al. 2010; Zahid et al. 2015). Phosphobacteria, phytate-mineralizing bacteria, and phosphatesolubilizing bacteria have been commonly isolated from soil and proposed as inoculants for agricultural improvement (Jorquera et al. 2008). For example, a large diversity of microbes in Andisols under pastures and cereal crops are capable of mineralizing phytate (Jorquera et al. 2008; Martı´nez-Viveros et al. 2010; Menezes-Blackburn et al. 2014). They may be used to develop bacterial or enzyme systems as biofertilizers to overcome the limitations of conventional fertilizers in acidic soils, as well as for developing added value products from agricultural wastes. To this end, Calabi-Floody et al. (2012) studied the effect of enzyme–nanoclay complexes on P availability of composted cattle dung and showed that it increased the inorganic P content. Moreover, Menezes-Blackburn et al. (2014) suggest that inoculation of cattle manure with enzyme–nanoclay complexes enhances the organic P cycling and P nutrition of plants grown in P-deficient soils. Low N acquisition by plants is a limiting factor in agricultural ecosystems, and there is interest in using N2-fixing bacteria as an alternative to conventional fertilization.
Diazotrophic bacteria are capable of converting atmospheric dinitrogen (N2) into NH3, which can be used by plants (Islam et al. 2009). Among them, a number of free-living soil bacteria are considered to be PGPR because of their competitive advantage in C-rich and N-poor environments (Kennedy et al. 2004). Free-living N2-fixing bacteria have been considered as an alternative to conventional N fertilizer for promoting plant growth, and several research studies reported significant increases in grain and shoot biomass yield from plants inoculated with freeliving diazotrophic bacteria (Andrade et al. 2013; Barua et al. 2012; Kennedy et al. 2004; Park et al. 2005). Moreover, Vadakattu and Paterson (2006) reported that under intensive wheat rotation at Avon, South Australia, free-living N2-fixing bacteria contributed 20 kg N per ha per year, which represented 30%–50% of total crop requirements. This response was attributed to a combination of factors including enhancement of root development, production of growth regulators, and N2-fixation (Naiman et al. 2009). However, it is well known that bacteria directly inoculated in the soil system could be adversely affected by competition with native microorganisms, unfavorable physicochemical conditions, and fluctuating pH and temperature (Bréant et al. 2002). Encapsulating microorganisms in carrier materials (bioformulation) is designed to protect them during storage and from adverse environmental condition (pH, temperature, etc.), thus ensuring a gradual and prolonged release (Bashan 1986; Kim et al. 2012).
A wide range of microorganisms have been investigated, and a framework for selecting suitable organisms for specific purposes has been developed. Materials suitable for immobilization and preservation of bacteria include alginate gels, synthetic gels (Sol–Gel), polyacrylamide, agar and agarose, polyurethane, vermiculite, and polysaccharides (Bashan 1998; Liu et al. 2008). In addition, composite materials based on biodegradable polymer clay or nanoclays are being studied, including nanocomposites (Calabi-Floody et al. 2009). For example, encapsulation of free-living diazotrophic bacteria has been considered as one of the possible alternatives for inorganic N fertilizer for promoting plant growth and crop yield (Ivanova et al. 2005).
Plant factory
Plant Factories (also known as indoor farms or vertical farms) are gaining widespread attention over the last few years. Pioneered in Japan, the last few years show an acceleration in the adoption of plant factories in other regions such as the United States, Canada, Europe, and Southeast Asia. The differences between regions are becoming apparent: whereas the United States and Canada focus mainly on the local perspective, in Europe the focus is on optimizing yield and quality, while in Japan it is on food safety and maturing the production technology. Recent advancements in technology, knowledge of plant growth, and market development have resulted in plant factories becoming economically viable as a facility for the year-round production of high-quality leafy vegetables and herbs. Especially LED lighting has proven to be an enabler in this respect.
In plant factories, the plants are grown in multiple layers, in a fully controlled and closed environment, without daylight, and close to the consumer. Mostly, LED lighting is used for growing light as well as steering light. With LED lighting, the light level, spectrum, and photoperiod can be tuned to result in optimum growth in terms of yield and quality (Goto 2012 and Kozai et al. 2015). LEDs have shown to be suitable for multilayer growth because of their form-factor, cool temperature, and high energy efficiency. As a result, they can be located close to the plants (Stutte 2015). The economic viability of plant factories is influenced by a number of factors. Compared to greenhouses, plant factories require large investments. Also, the cost of operation of a plant factory is relatively high (Banerjee and Adenaeuer 2014). However, plant factories are very efficient production facilities: the yearly production per unit of growth area easily exceeds the production in the most advanced greenhouses.
To further improve the viability of plant factories and to be able to monitor the effectiveness of advances in systems and knowledge relevant for growth, it helps to define key performance indicators (KPIs). An important KPI for the efficiency of growth is the one relating biomass production to the integral amount of light used for the production (this KPI is hereafter called light-useefficiency or LUE, expressed in gmol–1). This KPI which can be maximized by an optimal lighting design in combination with a transplanting strategy that maximizes the interception of light by the plants is shown. A model to estimate the theoretical maximum LUE achievable was presented. The approach with specific examples of what had been achieved for lettuce growth and the growth of several types of herbs was illustrated. Since the use of energy in a plant factory represents a considerable fraction of the cost of operation, some detail of a KPI related to the energy efficiency of LED lighting performance indicators for the efficiency of other resources are out of scope (Kozai 2013). Next to a high growth efficiency, resulting in a high production, also crop quality is important. It is not obvious that a high quality can be achieved without compromising a high production. As an example, it was shown that the coloration of lettuce and the reduction of nitrate in lettuce can be achieved by a preharvest lighting strategy such as not to compromise the overall growth efficiency.
Vertical farming
Increase in the worldwide population combined with the rise in per capita consumption of modern man has led to increased demand for food. To meet the growing demands and to feed the masses, more agricultural land is required. This has led to increased pressure on existing farmlands (which are being harvested multiple times in a year) as well as necessitated the acquisition of more land for agriculture. But an increased understanding of the adverse effects of deforestation has led man to think of alternative ways to optimize land use in agriculture. One such solution is vertical farming. This technique involves growing crops in a vertically stacked manner in an indoor controlled environment.
Vertical farming is environmentally friendly and pesticide free. But this technique is highly critical as it requires constant monitoring of the ambient parameters. Vertical farming is usually practiced in large warehouses which can be several storey’s tall. The sunlight is replaced by LED lights. The controlling parameters for vertical farming are mainly ambient light, ambient temperature, soil moisture, and humidity. Several automation techniques have been previously suggested for optimizing resource use, such as accommodation of actuation by automated irrigation systems (Kotamaki et al. 2009, Gutiérrez et al. 2014), but wireless monitoring using IoT in the field of vertical agriculture has not been previously suggested. The ambient parameters must be monitored for each stack along the vertical columns. This requires a set of sensors for each vertical stack. The large amounts of data generated by these sensors need an efficient data management system. Using the IoT, we can efficiently manage the sensor data, identify redundant sensor readings, and visualize it with the help of web-based applications (Gondchawar and Kawitkar 2016). Thus, a convenient IoT-based system may be developed for vertical farming.
CONCLUSION
For precision farming, recent research has focused on the spatial variability of production environments, development of efficient and suitable data management systems, efficiency of various types of image analyses and optical sensing, efficiency of sensors and related technologies, designs of precision agriculture equipment, optimal inputs and service uses and their spatial allocations, potentials of UAV and nano-technologies. For precision livestock farming, by collecting and analyzing vast quantities of data that no person would be able to complete on their own, precision livestock farming can provide producers with information about the welfare of the whole herd as well as individual animals. The continued development of precision livestock farming and the possibilities for interconnection in the livestock value chain, ultimately links consumers and farmers. Consumers will make decisions based on farm practices, and farms can make decisions based on consumer practices. For vertical farming, research has often highlighted the variations in technologies based on out-door / indoor systems, ways to improve plants’ access to light (natural or artificial), growing medium and nutrient / water supply, advanced features like electricity generation and integration of production space into office / residential space, and water treatment methods. As for soil monitoring system, Zigbee-based agriculture monitoring system serves as a reliable and efficient system for monitoring agricultural parameters. Wireless monitoring of field will not only allow user to reduce the manual labour, but also to see accurate changes in it. It is cheaper in cost and consumes less power. Such a system can be easily installed and maintained. For low-income South-Asian countries like India, these may be some of the key areas that the country can draw on from other more advanced countries in Asia, or focus in investing further research to improve the relevance of the technologies for the country.
REFERENCES
AAPFCO, 1995. Official Publication No. 48. Published by Association of American Plant Food Control Officials. Association of American Plant Food Control Officials (AAPFCO). Inc., West Lafayette, Indiana, USA.
Ahmad, F., Ahmad, I. and Khan, M.S., 2008. Screening of free-living rhizospheric bacteria for their multiple plant growth promoting activities. Microbiological Research 163: 173–181.
Akubattin, V.L., Bansode, A.P., Ambre, T., Kachroo, A. and SaiPrasad, P., 2016. Smart Irrigation System.
International Journal of Scientific Research in Science and Technology 2: 343-345.
Al-Ghobari, H.M., Mohammad, F.S. and El Marazky, M.S.A., 2017. Evaluating two irrigation controllers under subsurface drip irrigated tomato crop. Spanish Journal of Agricultural Research 14(4): 1206 Anderson, C., 2014. “Agricultural Drones, MIT Technology Review,” [Online]. Available:
https://www.technologyreview.com/featuredstory/526491/agricultural-drones
Andrade, M.M.M., Stamford, N.P., Santos, C.E.R.S., Freitas, A.D.S., Sousa, C.A. and Lira, J.M.A., 2013. Effects of biofertilizer with diazotrophic bacteria and mycorrhizal fungi in soil attribute, cowpea nodulation yield and nutrient uptake in field conditions. Scientia Horticulturae 162: 374–379.
Ariff, M.H., Ismarani, I. and Shamsuddin, N. 2014. RFID based systematic livestock health management system. In Proceedings of the 2014 IEEE Conference on Systems Process Control (ICSPC), Kuala Lumpur, Malaysia,12–14 December 2014, pp. 111–116.
Banerjee, C. and Adenaeuer, L., 2014. Up, up and away! The economics of vertical farming. Journal of Agricultural Studies 2:40–60.
Banhazi, T.M., Lehr, H., Black, J.L., Crabtree, H., Schofield, P., Tscharke, M. and Berckmans, D., 2012. Precision Livestock Farming: An international review of scientific and commercial aspects. International Journal of Agricultural and Biological Engineering 5: 1–9.
Bargeron, C.T., and Moorhead, D.J., 2007. EDDMapS- Early Detection and Distribution Mapping System for the Southeast Exotic Pest Plant Council. Wildland Weeds 10(4):4-8.
Barua, S., Tripathi, S., Chakraborty, A., Ghosh, S. and Chakrabarti, K., 2012. Characterization and crop production efficiency of diazotrophic bacterial isolates from coastal saline soils. Microbiological Research 167: 95–102.
Bashan, Y., 1986. Alginate beads as synthetic inoculant carriers for slow release of bacteria that affect plant growth. Applied and Environmental Microbiology 51(5): 1089–1098.
Bashan, Y., 1998. Inoculants for plant growth promoting bacteria for use in agriculture. Biotechnology Advances 16: 729–770.
Borghi, E., Avanzi, J.C., Bortolon, L., Junior, A.L. and Bortolon, E.S. 2016. Adoption and use of precision agriculture in Brazil: Perception of growers and service dealership. Journal of Agricultural Science 8 (11): 89.
Boselin, P.S.R., Pradeep, M. and Gajendran, E., 2017. An analysis of smart irrigation system using wireless sensor network. Star International Journal 5 3(3): 12-17
Bramley, R., and Trengove, S.A.M., 2013. Precision agriculture in Australia: Present status and recent developments. Engenharia Agrícola 33 (3): 575-588.
Bréant, D., Jézéquel, K. and Lebeau, T., 2002. Optimization of the cell release from immobilized cells of Bacillus simplex cultivated in culture media enriched with Cd2+: influence of Cd2+, inoculum size, culture medium and alginate beads characteristics. Biotechnology Letters 24: 1237–1241.
Butzen, S., 2013. Common nitrogen fertilizers and stabilizers for corn production. In: Crop Insights. vol. 23.
DuPont Pioneer, Johnston, Iowa. No. 2.
Calabi-Floody, M., Theng, B.K.G., Reyes, P. and Mora, M.L., 2009. Natural nanoclays: applications and future trends—a Chilean perspective. Clay Minerals 44 (16): 1–176.
Calabi-Floody, M., Vela´squez, G., Gianfreda, L., Sa ggar, S., Bolan, N., Rumpel, C. and Mora, M.L., 2012. Improving bioavailability of phosphorous from cattle dung by using phosphatase immobilized on natural clay and nanoclay. Chemosphere 89: 644–655.
Chen, X., Luo, Q., Jiang, Y., Lv, Z., and Wu, S. 2008. A WebGIS Expert System for Rice Brown Planthopper Disaster Early-Warning in China's Shanghai. Bioinformatics and Biomedical Engineering, 2485-2488.
De Montis, A., Modica, G. and Arcidiacono, C., 2017. Aginformatics. In Encyclopedia of Big Data, AG2017 ed.; Schintler, L.A.; McNeely, C.L., Eds.; Springer: New York, New York, USA, pp. 1–4. Doi: 10.1007/978-3-319-32001-4.
DeShazer, J.A., Moran, P., Onyango, C.M., Randall, J.M. and Schofield, C.P. 1988. Imaging Systems to improve Stockmanship in Pig Production; AFRC Institute of Engineering Research: Bedfordshire, UK.
Dhumane, A. V., Prasad, R. S. and Prasad, J. R., 2017. An Optimal Routing Algorithm for Internet of Things Enabling Technologies. International Journal of Rough Sets and Data Analysis 4(3):1-16. doi:10.4018/IJRSDA.2017070101
Dlodlo, N. and Kalezhi, J. 2015. The internet of things in agriculture for sustainable rural development. In: 2015 International Conference on Emerging Trends in Networks and Computer Commununication (ETNCC), pp 13–18.
Dominic, 2010. Natural Disaster Monitor. Retrieved from Android 15 November 2010.
Du, C.W., Zhou, J.M. and Shaviv, A., 2006. Release characteristics of nutrients from polymer coated compound controlled release fertilizers. Journal of Polymers and the Environment 14: 223–230.
Egamberdieva, D. and Adesemoye, A.O., 2016. Improvement of crop protection and yield in hostile agroecological conditions with PGPR-based biofertilizer formulations. In: -Arora, N.K., Mehnaz, S., Balestrini, R. (Eds.), Bioformulations: For Sustainable Agriculture. Springer, India, pp. 199–211.
Foster, A., 2013. Development of Biocompatible Aptamer Films as Smart Materials for Novel Fertilizer Systems. Master Thesis, Carleton University, Ottawa, Canada.
Gang, L.L.L. 2006. Design of greenhouse environment monitoring and controlling system based on bluetooth technology. Transactions of the Chinese Society for Agricultural Machinery 10: 97–100
Georgiou, O. and Raza, U., 2017. Low Power Wide Area Network Analysis: Can LoRa Scale? IEEE Wireless Communication Letters PP. 10.1109/LWC.2016.2647247.
Gondchawar, N. and Kawitkar, R.S., 2016. IoT based smart agriculture. International Journal of Advanced Research in Computer and Communication Engineering 5(6): 838-842
Goto, E., 2012. Plant production in a closed plant factory with artificial lighting, VII International Symposium on Light in Horticultural Systems, Vol. 956, pp. 37–49.
Gutiérrez, J., Villa-Medina, J.F., Nieto-Garibay, G. and Porta-Gándara, M.A., 2014. Automated irrigation system using a wireless sensor network and GPRS module. IEEE Transactions on Instrumentation and Measurement 63:166–176
Hostetler, C., Stokes, A. and Becton, L., 2018. National Pork Board Request for Proposals General Call 2018 Writing Research Proposals for the NPB The National Pork Board Is Soliciting Research Proposals Dealing with These Categories; National Pork Board: Ames, Iowa, USA; pp. 1–19.
Huang, H., Bian, H., Zhu, S. and Jin, J., 2011 A greenhouse remote monitoring system based on GSM. In: 2011 International Conference on Information Management, Innovation Management and Industrial Engineering (ICIII), Vol. 2, pp. 357–360.
Islam, M.R., Madhaiyan, M., Boruah, H.P.D., Yim, W., Lee, G., Saravanan, V.S., Fu, Q., Hu, H. and Sa, T., 2009. Characterization of plant growth-promoting traits of free-living diazotrophic bacteria and their inoculation effects on growth and nitrogen uptake of crop plants. Journal of Microbiology and Biotechnology 19 (10): 1213–1222.
Ivanova, E., Teunou, E. and Poncelet, D., 2005. In: Gruev, B., Nikolova, M., Donev, A. (Eds.), Alginate based macrocapsules as inoculants carriers for production of nitrogen biofertilizers. Proceedings of the Balkan Scientific Conference of Biology in Plovdiv (Bulgaria), pp. 90–108.
Jorquera, M., Herna´ndez, M., Rengel, Z., Marschner, P. and Mora, M.L., 2008. Isolation of culturable phosphobacteria with both phytatemineralization and phosphatesolubilization activity from the rhizosphere of plants grown in a volcanic soil. Biology and Fertility of Soils 44: 1025–1034.
Kalubhai, P., 2015. Present Status and Prospects of Micro Irrigation System in Mahisagar District of Gujarat. Doctoral dissertation, Anand Agricultural University, Anand, India.
Kennedy, I.R., Choudhury, A.T.M.A. and Kecske´s, M.L., 2004. Non-symbiotic bacterial diazotrophs in crop-farming systems: can their potential for plant growth promotion be better exploited? Soil Biology and Biochemistry 36: 1229–1244.
Keskin, M., and Sekerli, Y.E., 2016. Awareness and adoption of precision agriculture in the Cukurova region of Turkey. Agronomy Research 14 (4): 1307-1320.
Kim, S.Y., Kim, M., Lee, S., Lee, J. and Ko, G., 2012. Survival of microorganisms on antimicrobial filters and the removal efficiency of bioaerosols in an environmental chamber. Journal of Microbiology and Biotechnology 22 (9): 1288–1295.
Kotamaki, N., Thessler, S., Koskiaho, J., Hannukkala, A.O., Huitu, H., Huttula, T., Havento, J., Jarvenpaa,
M. 2009. Wireless in-situ sensor network for agriculture and water monitoring on a river basin scale
in Southern Finland: evaluation from a data user’s perspective. Sensors 4:2862–2883
Kozai, T., Niu, G. and Takagaki, M., (Eds.), 2015. Plant Factory: An Indoor Vertical Farming System for Efficient Quality Food Production, Elsevier, Amsterdam.
Kozai, T. 2013. Resource use efficiency of closed plant production system with artificial light: concept, estimation and application to plant factory, Proceedings of the Japan Academy Series B 89: 447–461.
Li, R.A., Sha, X. and Lin, K., 2014. Smart greenhouse: a real-time mobile intelligent monitoring system based on WSN. In: Wireless Communications and Mobile Computing Conference (IWCMC) 2014 International, pp. 1152–1156.
Liu, C.H., Wu, J.Y. and Chang, J.S., 2008. Diffusion characteristic and controlled release of bacterial fertilizer from modified calcium alginate capsules. Bioresource Technology 99: 1904–1910.
Luo, H., Yang, P., Li, Y., Xu, F., 2012. An intelligent controlling system for greenhouse environment based on the architecture of the internet of things. Sensor Letters 10(1–2): 514–522
Martı´nez-Viveros, O., Jorquera, M.A., Crowley, D.E., Gajardo, G. and Mora, M.L., 2010. Mechanisms and practical considerations involved in plant growth promotion by rhizobacteria. Journal of Soil Science and Plant Nutrition 10(3): 310–339.
Mastronardi, E., Tsae, P., Zhang, X., Monreal, C.M. and DeRosa, M.C., 2015. Strategic role of nanotechnology in fertilizers: potential and limitations. In: Rai, M., Ribeiro, C., Mattoso, L., Duran, N. (Eds.), Nanotechnologies in Food and Agriculture. Springer, Cham, Switzerland, pp. 25–67.
Menezes-Blackburn, D., Jorquera, M.A., Gianfreda, L., Greiner, R.L. and Mora, M.L., 2014. A novel phosphorus biofertilization strategy using cattle manure treated with phytase–nanoclay complexes. Biology and Fertility of Soils 50: 583–592.
Mondal, P., and Basu, M., 2009. Adoption of precision agriculture technologies in India and in some developing countries: Scope, present status and strategies. Progress in Natural Science 19(6): 659-666. Moorhead, C. T., 2007. Early Detection and Distribution Mapping System for the Southeast Exotic Pest
Plant Council
Naiman, A.D., Latro´nico, A. and Garcı´a de Salamone, I.E., 2009. Inoculation of wheat with Azospirillum brasilense and Pseudomonas fluorescens: impact on the production and culturable rhizosphere microflora. European Journal of Soil Biology 45: 44–51.
National Research Council. 1997. Precision Agriculture in the 21st Century: Geospatial and Information Technologies in Crop Management. Committee on Assessing Crop Yield: Site-Specific Farming, Information Systems, and Research Opportunities. Washington DC: National Academies Press. http://www.nap.edu/catalog/5491/precision-agriculturein-the-21st-century-geospatial-and-informatio n-technologies
Naz, M.Y., Sulaiman, S.A., 2016. Slow release coating remedy for nitrogen loss from conventional urea: a review. Journal of Controlled Release 225(10): 109–120.
Neethirajan, S., 2017. Recent advances in wearable sensors for animal health management. Sensing and Bio-Sensing Research 12: 15–29. doi:10.1016/j.sbsr.2016.11.004.
Ni, B., Liu, M. and Lu, S., 2009. Multifunctional slow-release urea fertilizer from ethylcellulose and superabsorbent coated formulations. Chemical Engineering Journal 155: 892–898.
Park, M., Kim, C., Yang, J., Lee, H., Shin, W., Kim, S. and Sa, T., 2005. Isolation and characterization of diazotrophic growth promoting bacteria from rhizosphere of agricultural crops of Korea. Microbiological Research 160: 127–133.
Paustian, M., and Theuvsen, L., 2017. Adoption of precision agriculture technologies by German crop farmers. Precision Agriculture 18(5): 701–716.
Puri, M., Solanki, A., Padawer, T., Tipparaju, S.M., Moreno, W.A. and Pathak, Y., 2015. Introduction to Artificial Neural Network (ANN) as a Predictive Tool for Drug Design, Discovery, Delivery, and Disposition: Basic Concepts and Modeling; Elsevier Inc.: Amsterdam, the Netherlands, pp. 3–13. doi:10.1016/B978-0-12-801559-9.00001-6.
Rachana, P.K. and Suhas, D.R., 2014. Android Application Agriculture Decision Support System. Int.
Journal of Engineering Research Applications, 63-66.
Ranade, P. and Takale, S.B., 2016. Smart irrigation system using FPGA based wireless sensor network.
IEEE Proceeding 15: 223–224
Roy, I., Gupta, M.N., 2003. Smart polymeric materials: emerging biochemical applications. Chemistry & Biology 10(12): 1161–1171.
Schimmelpfennig, D., 2016. Farm Profits and Adoption of Precision Agriculture (No. 249773). Washington, DC: United States Department of Agriculture, Economic Research Service.
Sharma, S., Raval, J., and Jagyasi, B., 2013. Mobile sensing for agriculture activities detection. 2013 IEEE Global Humanitarian Technology Conference (GHTC).
Shaviv, A., 2005. Controlled Release Fertilizers, IFA International Workshop on Enhanced-Efficiency Fertilizers, Frankfurt. International Fertilizer Industry Association, Paris, France.
Stutte, G.W., 2015 Commercial transition to LEDs: a pathway to high-value products. Hortscience 50: 1297–1300
Taylor, J., 2018. Capital Growth: Precision Agriculture and Vertical Farming in the Corporate Food Regime. New York: CUNY Academic Works.
Trenkel, M.E., 1997. Improving Fertilizer Use Efficiency: Controlled-Release and Stabilized Fertilizers in Agriculture, the International Fertilizer Industry Association, Paris. International Fertilizer Industry Association (IFA), Paris, France, p. 151.
Tyagi, A., Gupta, N., Navani, J.P., Tiwari, M.R. and Gupta, M.A., 2017. Smart Irrigation System.
International Journal for Innovative Research in Science & Technology 3: 9-12.
Vadakattu, G. and Paterson, J., 2006. Free-living bacteria lift soil nitrogen supply. Farming Ahead 169: 40 Watcharaanantapong, P., Roberts, R.K., Lambert, D.M., Larson, J.A., Velandia, M., English, B.C., Rejesus,
R.M. and Wang, C., 2014. Timing of precision agriculture technology adoption in US cotton production. Precision Agriculture 15(4): 427-446
WSI, 2008. Stormtracker 2.8.3. Android 2.3.3
Xiaofeng, L., Linlin, Q., Linjian, L. and Gang, W. 2015. Design and implementation of modern greenhouse remote monitoring system based on the Android system. In: 34th Chinese Control Conference (CCC), pp. 5742–5746.
Xu, Z., Chen, J., Wang, Y. and Fan, Z., 2016. A remote monitoring system for greenhouse based on the internet of things. In: MATEC Web of Conferences, EDP Sciences 77:1-4
Yan-E, D., 2011. Design of intelligent agriculture management information system based on IoT. In: Proceedings of the 4th International Conference on Intelligent Computation Technology Automation ICICTA 2011, Vol. 1, pp 1045–1049
Zahid, M., Abbasi, M.K., Hameed, S. and Rahim, N., 2015. Isolation and identification of indigenous plant growth promoting rhizobacteria from Himalayan region of Kashmir and their effect on improving growth and nutrient contents of maize (Zea mays L.). Frontiers in Microbiology 6(207): 1–10.
Zhang, X., Zhang, C., Fang, J., Yu, X. and Liang, J., 2009. Smart sensor nodes for wireless soil temperature monitoring systems in precision agriculture. Transactions of the Chinese Society for Agricultural Machinery 40: 237-240.
The Current Technologies That Can Be Used for Smart Agriculture
ABSTRACT
Smart agriculture is used to enhance agricultural productivity, address agricultural-based issues, such as food demand, and make farms more connected and intelligent. The market value of smart agriculture worldwide is forecasted to reach around 26.76 billion U.S. dollars by 2020. Smart agriculture incorporates Internet of Things (IoT) technology and solutions in order to attain improved operational efficiency, maximized yield, and minimized wastage through the use of real-time field data collection, data analysis, and the deployment of control mechanisms. Smart agriculture employs a range of IoT-based applications such as precision farming, variable rate technology, smart irrigation, as well as smart greenhouses. Other applications of IoT in smart agriculture include: Variable Rate Technology (VRT), agriculture drones, soil monitoring systems, and precision livestock farming. Telecom operators benefit a lot from smart agriculture as cellular connections account for by far the largest share of communications systems in smart agriculture worldwide. Precision farming is set to become the most influential agricultural trend in developed countries. The market value of precision farming is also expected to grow from 730 million U.S. dollars in 2015 to 2.42 billion U.S. dollars by 2020. A look at the distribution of precision farming worldwide shows North America as the major player with market value estimated at to 1.2 billion Euros in 2014. The main drivers of smart agriculture include: the realities of a changing climate, a need to conserve water, and its emphasis on the enhancement of efficiency through resource optimization and cost minimization.
Keywords: Smart agriculture, Internet of Things (IoT), Precision farming, Variable Rate Technology (VRT), Agriculture drones
INTRODUCTION
Smart agriculture is a farming management concept using modern technology to increase the quantity and quality of agricultural products. Farmers in the 21st century have access to Global Positioning System (GPS), soil scanning, data management, and IoT technologies. By precisely measuring variations within a field and adapting the strategy accordingly, farmers can greatly increase the effectiveness of pesticides and fertilizers, and use them more selectively. Similarly, using smart agriculture techniques, farmers can monitor better the needs of individual animals and adjust their nutrition correspondingly, thereby preventing disease and enhancing herd health.
Knowledge and capital are essential for any innovation. New farming technologies require more and more professional skills. A farmer today is not only a person with a passion for agriculture, he or she is also a legal expert (to find their way through a growing maze of regulations) and a part-time data analyst, economist and accountant (making a living from selling agricultural produce requires bookkeeping skills and an in-depth knowledge of market chains and price volatility).
Furthermore, smart agriculture requires capital. Thankfully, there are a wide range of options available. From using low capital investment smart phone applications that track your livestock to a capital-intensive automated combine. In principle, implementing smart agriculture technologies can be easily upscaled.
Modern farms and agricultural operations work far differently than those a few decades ago, primarily because of advancements in technology, including sensors, devices, machines, and information technology. Today’s agriculture routinely uses sophisticated technologies such as robots, temperature and moisture sensors, aerial images, and GPS technology. These advanced devices and precision agriculture and robotic systems allow businesses to be more profitable, efficient, safer, and more environmentally friendly.
Farmers no longer have to apply water, fertilizers, and pesticides uniformly across entire fields. Instead, they can use the minimum quantities required and target very specific areas, or even treat individual plants differently. Benefits include higher crop productivity, decreased use of water, fertilizer, and pesticides, which in turn keeps food prices down, reduced impact on natural ecosystems, less runoff of chemicals into rivers and groundwater and increased worker safety
In addition, robotic technologies enable more reliable monitoring and management of natural resources, such as air and water quality. It also gives producers greater control over plant and animal production, processing, distribution, and storage, which results in greater efficiencies and lower prices, safer growing conditions and safer foods and reduced environmental and ecological impact.
IoT IN SMART AGRICULTURE
ITU (International Telecommunication Union) defined the Internet of Things as: “A technology that mainly resolves the interconnection between human to a thing, thing to thing, and human to human.” IoT is a world-shattering technology that signifies the future of computing and information interchange (Patil et al. 2012). It is based on the communication between intelligent sensors, RFID (radio-frequency identification), GPS, infrared sensors, remote sensing (RS), mobile communication, and other communication networks (Dlodlo and Kalezhi 2015). It refers to a network of objects and is often a self-configurable wireless network (Patil et al. 2012). The basic purpose of IoT is to make a huge network by the combination of diverse sensor devices such as GPS, RS, RFID, laser scanner, and networks to comprehend the information sharing of global things. IoT can encompass millions of networked embedded smarts devices also called smart things; these smart things are capable of accumulating information about themselves, their environment, and associated smart devices and interconnect this information to other devices and systems via the all connecting Internet (Fig. 1) (Yan 2011).
Precision agriculture
Precision agriculture refers to a suite of technologies that may reduce input costs by providing the farm operator with detailed spatial information that can be used to optimize field management practices (National Research Council 1997; Schimmelpfennig 2016). The adoptions of precision agriculture technologies have, so far, mostly been limited to parts of developed countries. In Australia, by 2013, 90
%of farmers had been using some type of precision agriculture, and 20 % of farmers had been using yield mapping and variable rate fertilizer application (Bramley and Trengove 2013). In Germany, about 10-30 % of farmers were using some form of precision agriculture in 2016 (Paustian and Theuvsen 2017 - cited in Taylor 2018). Keskin & Sekerli (2016) provides related statistics in some other developed countries. In the United States, the use of precision agriculture started growing in the 2000s, and spread considerably, although at varying rates across crops and the types of precision agriculture (Schimmelpfennig 2016). In 2010 in the United States, yield monitoring has spread to almost 70 % of maize and soybean areas, while the adoptions of variable-rate technology have also reached about 30 % of the areas.
In the United States, the progress on precision agriculture has varied across crops. Yield monitoring for cotton lagged behind grain yield monitoring (Watcharaanantapong et al. 2014). This is partly because of equipment problems associated with accuracy, calibration and maintenance of optical sensors; for example, compared to those for maize, development of reliable and accurate cotton yield monitors did not occur until 2000 when yield monitors had already been used on about one-third of corn and soybean area in the Corn Belt, respectively (Schimmelpfennig and Ebel 2011; Watcharaanantapong et al. 2014). Furthermore, even in the US, adoption rates are positively correlated with farm size. For example, the largest corn farms in the country, those over 2,900 acres, are twice as likely to adopt precision agriculture techniques as the average farm (Schimmelpfennig 2016; Taylor 2018). Precision-agriculture technologies for corns, which have spread substantially in the United States recently, are still not adopted by 88% of farmers cultivating less than 600 acres (Schimmelpfennig 2016).
In developing countries, the use of precision agriculture has been limited. In Malaysia, site-specific fertilization is being applied to rubber plantations, and other precision technologies have started being applied for oil palm, although not to rice fields (Mondal and Basu 2009). In Turkey, recently, international companies like Topcon sold about 60 auto-steering system in the Southern part of the countries along Mediterranean regions (Keskin and Sekerli 2016). The service providers of auto-steering service set up own reference station networks for positioning correction, and some farmers owning automatic steering use them to serve other farmers mainly in ridge tillage in cotton and corn cultivation (Keskin and Sekerli 2016). Similar service dealership related to precision agriculture is observed in Brazil as well (Borghi et al. 2016). Similarly in Turkey, an international company (New Holland) is planning to install yield monitors on about 500 combine harvesters countrywide in the last few years (Keskin and Sekerli 2016). In India, technologies related to precision agriculture that have been adopted include drip and sprinkler irrigation, which have been growing steadily, although still accounting for only a fraction of irrigated areas in India (Kalubhai 2015). Similarly, while similarly accounting for a tiny fraction of areas within India, adoptions of laser-land-leveling technologies have been growing in parts of India, including Western Uttar Pradesh and Haryana (Tiwari and Jaga 2012).
Precision livestock farming
“Monitoring animals within groups is challenging, even for the best herdsmen” NPB—Animal Science Committee” (Hostetler et al. 2018). Precision livestock farming implies automated remote detection and monitoring of identified individuals for animal health and welfare using real-time analysis of images, sounds, tracking data, weight and body condition, and biological metrics in livestock (Berckmans 2014, Neethirajan 2017, De Montis 2017). With that, there is the capacity for early detection of illness or physiological status at the farm level. While not a new science (DeShazer et al. 1988), the information, applications, and availability of precision livestock farming has greatly increased due to computer science expertise and inexpensive sensors off-labelled from the video gaming industry (Xbox, PlayStation R, etc.) combined with the growing computer capacity for capturing and processing data (Nasirahmadi et al. 2017, Berckmans 2014, Banhazi et al. 2012).
In order to better understand how current technology may impact the world of precision livestock farming, a basic understanding of remote monitoring sensors, the process of algorithm development and machine learning is required. Remote sensors such as cameras, microphones, thermometers and accelerometers monitor or capture information such as images, sound, heat or motion from groups or individual animals. The data from the sensors, stored in external drives or sent directly to a processing node (analogous to transferring photos from a digital camera to a computer) are then processed by algorithms. An algorithm is a formula, or step-by-step set of operations, used to solve a specific problem or class of problems. A programming algorithm is a computer procedure that tells a computer precisely what steps to take to solve a problem utilizing inputs to determine the outputs. Programmers initiate the process by writing the algorithm that instructs the computer how to perform the specific operations necessary to solve a problem. An algorithm’s value to farmers is dependent on its ability to transform the sensor data or “feature variable” to a biological outcome. Examples of feature variables include percentage of time livestocks are lying down to determine the biological outcome “lameness” or the number of coughs to detect the biological outcome “respiratory disease”. Machine learning is a family of computational methods that allows an algorithm to program itself using large sets of examples. Because the computer “learns” from these example sets of existing data, a system can become highly adept at processing and analyzing large data sets to track variables and produce estimates at a rate that would not be possible for humans or traditional statistical methods (Puri et al. 2015). Taken together, data from remote monitoring sensors is combined with individual animal identification, referenced observations and production data, and then integrated in algorithms to provide credible information and alerts regarding livestock welfare, health and productivity.
Individual identification methods, either currently used in the swine industry or research, include radio frequency identification, optical character recognition, and facial recognition. A well-established technology for livestock identification, health purposes and management on farms is the radio-frequency identification (RFID) chip (Ariff et al. 2014). The device is primarily implanted in ear tags; it stores information such as animal and farm records. The radio wave (low, high or ultra-high frequency) is the medium of communication between the transponder circuit within the tag and an RFID reader to wirelessly read and write data. The working principle of such a device is that when an RFID tag comes within range of an RFID reader, it receives a signal. Furthermore, a second radio frequency signal is induced, carrying data that travels to the reader (Ariff et al. 2014). These data can be stored and analyzed later, or the RFID chips can be used immediately to identify individual animals. Low frequency (LF)—RFID is a valuable component of groups housing electronic sow feeders; these small feeder enclosures fit one sow at a time and dispense a specific limited diet to the sow while also collecting identification data and feeding frequency via RFID (Maselyne et al. 2015). Infrequent visits of certain sows could raise an alert to producers so that health issues or undesirable social behaviors may be addressed early in their progression (Cornou et al. 2008). Nevertheless, LF-RFID has two major disadvantages: a low read range (<1 m), and the inability to identify more than one animal (Adrion et al. 2017) within range. In order to track multiple animals at a greater range (3 to 10 m), researchers have investigated the commercial feasibility of Ultra-High Frequency (UHF) readers (Sales et al. 2015). While promising, the UHF-RFID transponder ear tags are sensitive to interference from ear tissue, leading to false registrations (Brown-Brandl 2018), thus requiring further research to adapt the technology for consistent use in animal identification. Other drawbacks to RFID include loss or attrition of tags, pain and stress of the animal during tagging and required removal from the animal prior to slaughter processing.
A low-cost identification system, optical character recognition, is the recognition of printed, stamped, or written text characters (e.g., license plates, barcodes, and QR codes) by a computer. In livestock production, optical recognition includes characters on ear tags or painted symbols and numbers. Optical character recognition is performed with a digital camera and data is developed with machine learning to provide remote identification (Mittek et al. 2017). Depending on the clarity and color of the markings, there is the capacity to identify large permutations of animals and, with the exception of optical character recognition on tags, the identification may not need to be removed from livestocks prior to slaughter (Mittek et al. 2016). When the characters are painted, visual identification patterns can fade within a day and when livestocks lie close to or on one another, pattern recognition is occluded (Lancaster et al. 2012).
The development of a new technology for precision livestock farming requires a deep understanding of the specific task to identify what type of sensor best suits those needs. There are various ways in which to design and implement such new devices. Single sensor designs are easier to integrate and develop, but are restricted within sensor capabilities. Implementations which include multiple sensor types come with an added benefit of higher accuracy and robustness, but at the cost of the design complexity of sensor fusion to create a joint signal for processing. Validation of data and how to identify the accuracy or precision of such measurement or prediction requires background knowledge in statistics and probability (Kay 1993). In a practical setting, human observations can be taken from well-trained (experts) individuals and can be compared to device results to quickly assess performance. For fine accuracy, statistical methodologies such as least squares and maximum likelihood estimation can be performed on the data to evaluate accuracy. Standard engineering design processes such as preliminary hazard analysis, design failure mode and affect analysis, and design reviews should be utilized especially within farm settings where possible hazards are not accounted for, resulting in performance loss. Having close communication with farms and being able to test and prototype designs will help identify what design constraints are necessary for a successful device.
Variable rate technology
One of the components of precision agriculture concept is Variable Rate Control (VRC) that implements management decisions at right time and place. VRC is a process of applying varying rates of inputs (water, fertilizer, pesticides etc.) at proper zones throughout the field in an optimized way. This optimum utilization of resources helps to maintain economic profit ensuring sustainability and environmental safety. The technology used for variable rate control is known as Variable Rate Technology (VRT) (Council 1997). VRT are briefed in Table 1. From this table it is clear that most of the literatures concentrate on Variable Rate Fertigation (VRF). Very little work is done on the combination of four types of VRT – Variable Rate Irrigation (VRI), VRF, Variable Rate Pesticide (VRP), and Variable Rate Herbicide (VRH). It inspires the researchers to develop a single control unit that considers the correlation among different field and weather parameters and develop a combined VRT. This combined VRT can apply water, fertilizer, pesticide, and herbicide at a prescribed rate with a centralized controller.
Table 1. Technology used in reviewed literature for variable rate control.
References
Technologies
VRF
VRP
VRH
VRI
(Zheng et al. 2013, Chattha et al. 2014, Reyes et al. 2015, Ying-zi et al. 2015)
√
X
X
X
(Jones et al. 2016)
X
√
√
X
(Kargar and Shirzadifar 2013)
X
X
X
X
(Zhang and Song 2014)
√
X
X
√
(Partel et al. 2019)
X
X
√
X
(Weber et al. 2018)
X
√
X
X
Soil monitoring systems
Soil moisture and climatic conditions are the two most important factors in agriculture productivity. During irrigation, water wastage should be avoided. The plants or crop should be irrigated only when they need to be. When plants transpire more amount of water, the relative humidity of atmosphere increases. This presence of large amount of relative humidity increases the chances of disease attack. So, soil moisture levels in the field require periodic inspection, from where one can determine, when the next irrigation should be done and how much amount of water should be applied. Current developments in the miniaturization of electronic devices and wireless communication technology have resulted in the emergence of an energy efficient WSN. This makes it possible to acquire the field information more timely, accurately and conveniently. Monitoring parameters of temperature and humidity is an important means for obtaining high-quality environment. Remote monitoring is an effective method in order to avoid interference environment and improve efficiency. Today, Ethernet network and ZigBee wireless networks are used to transmit data in remote monitoring System. The IoT is a system which integrates the computing devices, possessing the ability of transferring the data over the network via the better route. (Dhumane et al. 2017). A review of remote control and monitoring systems based on existing technologies and a ZigBee based IoT network to allow efficient routing in the network was given.
The opportunity to design a device that monitors soil moisture, temperature and humidity of field atmosphere, and transmit the information to the remote receiver at the farmhouse or outside the field was taken here. The remote receiver is a laptop connected to the Zigbee transceiver. (Zhang et al. 2009) The proposed system consists of Arduino as processing unit and WSN base station. A soil moisture and temperature humidity sensor is connected to the WSN data collection node. The sensor node also consists of a LCD module, where the sensor output is displayed in real time. The sensor node is building block of the WSN. The task of sensor node is to achieve the perception, collection, processing and wireless transmission. The sensor node converts the physical quantity to the voltage signal and Arduino UNO board controls the processing, and manages the communication protocol. The sensor node communicates with the base station using transceiver. The base station collects all the data send by sensor node. The whole system is made with the collaboration of hardware and software components. The hardware components include Arduino UNO board, soil moisture sensor, DHT11 temperature humidity sensor, LCD module and Zigbee transceivers. The software includes Arduino IDE and X-CTU.
Smart irrigation
With the changes in technologies and rapid consumption of natural resources by humans, it is vital to implement smart irrigation techniques to overcome the scarcity of water resources in near future. Smart irrigation using wireless sensors for precision agriculture, drip irrigation automation system for water level monitoring, are discussed by considering various factors like soil moisture, humidity, and temperature in (Gajendran et al. 2017). In (Al-Ghobari et al. 2017), couple of irrigation tools, SmartLine and Hunter Pro-C2, are used to achieve water savings and better crop yield. Here, Hunter Pro-C2 has realized better economic benefits and large amount of irrigation water is saved. Unplanned usage of water and irrigation with automatic valve mechanism based on the soil moisture is illustrated in (Tyagi et al. 2017, Akubattin et al. 2016) which minimizes manual intervention of farmers for crop irrigation. In (Ranade and Takale 2016), a framework for efficient water distribution management is defined using wireless sensor network (WSN) and field-programmable gate array (FPGA), where data is transmitted using ZigBee and based on which control of motor on/off conditions is determined.
The above mentioned research efforts majorly focused on illustrating smart irrigation techniques by using various irrigation tools, automating irrigation systems using actuators, and sensors for minimizing the water consumption in crop irrigation and to achieve better crop yield. But it is vital that these smart irrigation techniques must be extended for preserving water generated from rainfall which will help to increase the groundwater levels in agricultural farms and to enable farmers to save crops from unforeseen rainfall by integrating farms with real-time weather conditions. A framework based on cloud and Internet of things to implement a smart irrigation system that saves crops during unforeseen rainfall, increases groundwater levels
with a competent mechanism, and to reuse excessive water generated during rainfall for crop irrigation was proposed. Based on the defined framework, a use case for smart irrigation system is developed which extends the features depicted in (Tyagi et al. 2017, Akubattin et al. 2016, Ranade and Takale 2016) where agricultural farms are closely monitored based on real-time weather conditions and soil moisture. Also, a competent mechanism is defined to avoid rainwater depletion, utilize excessive water generated from rainfall for crop irrigation, and save farms during unforeseen rainfall.
Early warning system
There is a great deal of the Android applications alert system and agriculture in the literature. The system in (Sharma 2013) uses a mobile phone to detect the activities around the farm. The system will collect the data through the mobile phone (GPS, accelerometer and microphone sensor) carried by the farmers. By obtaining the GPS coordinates, the system can obtain the statistics of the working pattern of any farm worker and frequency of visit to a certain area (different plots) of the farm. The work in (Rachana and Suhas 2014) uses of Android mobile to increase productivity of crops and indirectly increase the knowledge of the farmer society.
In (Dominic 2010), the Android apps make use of the alerts published by the Global Disaster Alert and Coordination System (GDACS). The app shows icons on the map with color green, orange and red, each mapping the severity of the calamities. StormTracker (WSI 2008) makes use of the NOWrad (National Operational Weather radar) which gives one of the best resolution cloud imagery with color codes. It also gives 10-day forecasts with both daily and hourly details. Users can also lock their favorite locations to keep track of the local area. WebGIS (Geographic Information System) Expert System for Rice Brown Hopper Disaster Early-Warning (Chen et al. 2008) can perform disaster evaluation and disaster early-warning. The WebGIS is known for its features to locate and mark area in large scale. It is used to relate how the insects are spreading on the fields.
Most importantly, the farmers need to know about the current situation so that they can react before the insects spread out. Early Detection and Distribution Mapping System for the Southeast Exotic Pest Plant Council (Bargeron and Moorhead, 2007) was developed to fill up the species distribution of invasive plant throughout South America. The location of the data are various, in different formats, and unfitting to produce an overall map. The use of Google Map, instead of the flash map Area Selector and Adobe Flash technology has solved the problem of combining the available data from different locations.
Agriculture drones
Remote sensing techniques utilizing Unmanned Aerial Vehicles (UAVs) are in constant development and the use of tiny MEMS sensors (accelerometers, gyros, magnetometers, and often pressure sensors), small GPS modules, powerful processors, and a range of digital radios makes drones small, low cost and quite easy to use. In just under five years, UAVs have gone from a toy for gadget junkies to an essential tool in many application fields; today, agriculture is one of the fastest growing markets for the commercial UAVs industry. In 2014, the Massachusetts Institute of Technology classified agricultural drones at the primary position among the ten breakthrough technologies (Anderson 2014), helping the farmer in a better utilization of the land. As an example of drone utilization in farming in the wine production sector, multispectral cameras mounted on drones have highlighted the close correlation between the quality of the grapes and the health of the plants. Another example of drone utilization is the reduction in waste of water around a farm: drones can be fitted with remote sensing equipment (multispectral, hyperspectral or thermal sensing systems) in order to quickly and easily identify the driest sections of a field, thus allowing farmers to allocate their water resources more economically. Even the amount of chemicals ground penetration for fighting pest and fungal infestations can be reduced if drones effectively scan the ground of a farm and spray the correct amount of chemical substance modulating the distance from the ground. Moreover, a drone can survey a crop at any requirement of the farmer, thus showing any dangerous changes or other events of interest.
Drones, or UAVs, were first introduced during WWI for enemy surveillance. They are small, radio-controlled airplanes that can be used to take pictures. Nowadays, their use has expanded to small-time hobbyists and is quickly expanding to use in agriculture. Military drones are much larger than ones used by civilians. Crop scouting drones are small enough to be brought to the field in the back of a pickup, often under 15 pounds. The economic impact in the few years after regulations are implemented is predicted to be around $13 billion and 7500 commercial drones by The Association for Unmanned Vehicle Systems International, with 80 to 90% of the growth in the market coming from agricultural use (Karst 2012). The two main designs for UAVs are fixed-wing airplanes and rotary motor helicopters. Each of these has its own benefits. The fixed-wing drones are able to fly at a higher speed, 25 to 35 mph, while still taking pictures. It can cover 600 acres per hour (Patrico 2013), which is how long the charge on the battery lasts. Fixed-wing airplanes have to be thrown or launched into the air and need a large flat area to land upon. A parachute can be used in confined conditions, but that will severely reduce the life of the airplane. Luckily, replacement parts are easily available if the fuselage gets cracked. Rotary motors are able to hover and focus in on specific problem spots in a field while sacrificing maximum speed. They have a little less battery life because they often have to run four engines or more. The takeoffs and landings can be done in more confined areas, so they are better for beginner flyers.
Another aspect of the system is the ground control station. This is where the operator stands and monitors the flight and data coming from the drone. Some systems are simple enough to be operated from a smart phone. A number of different software programs are available to analyze the data. More important ones stitch together the separate images into one smooth picture and then can incorporate the image into GIS systems. Afterward, variable-rate applicators can use those pictures to find portions of the field that are low on nutrients or pressured by weeds. The drones can be manually flown, or pre-determined flight paths can be programed with GPS data. Learning to pilot the planes takes only a few hours, or they can be programed with one touch takeoff and will fly without any steering from the ground. Self-leveling programming can be installed to adjust for wind and to stabilize the images.
Drones are fairly cost effective and can pay for themselves in just a few uses. The cost of these UAVs can range from as little as $1000 for a starter system and can go up to $10,000 or $20,000, depending on the size of the machine and any extra cameras or features added. These systems are designed to have easily replaceable parts in case of crashes. Compare that to the several hundred dollars per hour it costs to have a piloted airplane fly over a field and it does not take long to cover the cost (Anderson 2014). The money saved by targeting areas for fertilizer and pesticide applications can be figured into the price as well. Plus, it benefits the environment to not over apply chemicals to areas that do not need it.
CLIMATE-SMART AGRICULTURE
Climate-Smart Agriculture (CSA) is an approach to help the people who manage agricultural systems respond effectively to climate change. The CSA approach pursues the triple objectives of sustainably increasing productivity and incomes, adapting to climate change and reducing greenhouse gas emissions where possible. This does not imply that every practice applied in every location should produce “triple wins”. Rather the CSA approach seeks to reduce trade-offs and promote synergies by taking these objectives into consideration to inform decisions from the local to the global scales and over short and long time horizons, to derive locally-acceptable solutions.
The majority of the world’s poor live in rural areas and agriculture is their most important income source. Developing the potential to increase the productivity and incomes from smallholder crop, livestock, fish and forest production systems will be the key to achieving global food security over the next twenty years. Climate change is expected to hit developing countries the hardest. Its effects include higher temperatures, changes in precipitation patterns, rising sea levels and more frequent extreme weather events. All of these pose risks for agriculture, food and water supplies. Resilience is therefore a predominant concern. Agriculture is a major source of greenhouse gas emissions. Mitigation can often be a significant co-benefit of actions to strengthen adaptation and enhance food security, and thus mitigation action compatible with national development priorities for agriculture is an important aspect of CSA.
Smart greenhouses
Greenhouses are widely used to produce food in USA, UK, and the Netherlands, but in India, greenhouse technology is still at a nascent stage. By employing greenhouses, food production can be increased by utilizing even the arid and non-fertile lands, thus increasing the yield. Having better control over the processes and maintaining a favorable atmosphere for the crops leads to high quality yield by reducing the wastages. Many systems were proposed for automating certain processes of the greenhouse and to provide remote monitoring of the parameters. Global System for Mobile Communications (GSM)-based systems that provide notifications through short message service (SMS) (Huang et al. 2011) and Zigbee-based wireless sensor network (WSN) (Li et al. 2014) were explored. Certain other systems used android application to provide remote monitoring (Xiaofeng et al. 2015). Some previous studies were also based on Bluetooth technology (Gang 2006).
A relatively new technology, LoRa, is studied and implemented in a farming scenario. Though the concept of greenhouse farming is not adopted on a large scale in India, several countries have benefitted immensely from its usage. Such modern farms can sprawl for hundreds of acres. But the system can also be implemented for small-scale farms. Between efforts to eat more food grown locally, a younger generation of farmers and cheaper component-farming is getting an infusion of data and technology. As the concept of the ‘Internet of Things’ becomes increasingly prevalent, many systems are being devised to allow all manner of data to be gathered and analyzed, and devices controlled via wireless data networks. Connected devices such as smart thermostats and lighting systems are making their way into homes, but another big opportunity for the IoT could be outdoors, in agriculture.
Greenhouse though concept up to speed internationally, the Indian farming economy is only actively enrolling itself in the recent times. Initial automation efforts in the farming sector include the automatic drip irrigation systems, which were developed to water the crops automatically based on the soil moisture level. But such systems automated only one process, and other processes were still monitored and controlled manually. Important parameters to be monitored and controlled in a greenhouse include soil moisture, temperature and humidity and lighting, and it is vital that all the factors are considered. Some of the previous systems developed include an android application to monitor the greenhouse but did not include mechanisms to control it (Luo et al. 2012, Xu et al. 2016). One of them was based on GSM in which notifications are sent via SMS. The drawback to this system was that human presence is required to control the parameters. Other systems using AT8051 microcontroller core were proposed which led to some automation and automatic control, but it was complicated to interface the microcontroller to a wireless network for cloud integration. Bluetooth-based systems had a short range and could not be used for long-distance communication.
Another option explored in this context was the Zigbee-based WSN. This has been widely adopted for farm monitoring and irrigation systems. WSN employing Zigbee protocol stack was efficient and led to complete automation of the process but was often too expensive. Though the WSN-based model boasts diverse nature and scalability, it poses limitations in terms of large area deployment and cost, for farming solutions. A system designed using WSN has a shorter range of coverage. A Zigbee coordinator can support only a few hundred nodes. A possible solution to overcome the above-discussed downfalls of the existing systems in agriculture was proposed. This system comprises of sensors and actuators which would be programmed by using an Mbed board which can aid the overall process of agriculture by automating it and helps keep the farmer updated by sending live feeds via the Internet. The technology of LoRaWAN is used here, as it provides features such as low power and wide range which are desired for this application. The LoRa technology is to provide ranges between 5 and 10 km, and its average battery life is about 5–11 years. This is highly desirable in farming considering the vast area of implementation of the system. LoRaWAN allows around thousand nodes to be attributed to one gateway (Georgiou and Raza 2017). The battery life and its low power consumption also make the system implementation more economical.
Smart fertilizers
Slow-/controlled-release fertilizers
According to Trenkel (1997), slow- or controlled-release fertilizers are those containing a plant nutrient in a form, which either (a) delays its availability for plant uptake and use after application, or (b) is available to the plant significantly longer than a reference “rapidly available nutrient fertilizer” such as ammonium NO - or urea, ammonium phosphate or potassium chloride (AAPFCO, 1995). There is no official differentiation between slow release and controlled-release fertilizers. However, the microbially decomposed N products, such as urea-formaldehydes, are commonly referred to as slow-release fertilizers, and coated or encapsulated products as controlled-release fertilizers (Trenkel, 1997). Delayed availability of nutrients or consistent supply for extended time periods can be achieved through a number of mechanisms. These include semipermeable coatings for controlled solubility of the fertilizer in water, protein materials, occlusion, chemicals, slow hydrolysis of water-soluble compounds of lower molecular weights, and some other unknown means (Naz and Sulaiman 2016).
Other options include utilization of semipermeable materials and sensors of chemical or biological origin within the fertilizer. These are advanced materials, whose physical or chemical properties can change in response to an external stimulus such as temperature, pH, and electric or magnetic fields (Foster, 2013; Mastronardi et al., 2015; Roy and Gupta, 2003). Many coating materials can be used to slow nutrient release, including natural materials such as clays and nanoclays (e.g., allophane), and nondegradable (polysulfone) and biodegradable polymers (e.g., alginate beads). Several studies have shown that urea-based coatings can have variable efficiencies depending on the material used (Du et al., 2006; Naz and Sulaiman, 2016; Shaviv, 2005).
In addition, they can be highly expensive in some cases, pollutants, or toxic (i.e., polymer-coated urea) and mostly difficult to degrade with potential environmental impacts (accumulation) as the major concern. As a consequence, formulation with environmentally safe and biodegradable coating materials seems to be necessary (Ni et al., 2009). In this sense, natural polymeric carbohydrates appear as an alternative to nonbiodegradable materials acting as permeable or impermeable membranes with tiny pores in slow-release fertilizers (e.g., urea) (Butzen 2013). These highly degradable materials have also received attention because of their low cost and low environmental damage due to biodegradability and low accumulation in the environment (Naz and Sulaiman, 2016). However, these polymers may need some modifications to be included into the coating design because of their hydrophilic properties and their weak coating barrier, for example, in the case of starch.
Biofertilizers
One group of microorganisms beneficial for plant growth is PGPR, a heterogeneous group of bacteria that can be found in the rhizosphere, at root surfaces, and in association with roots (Ahmad et al., 2008). These bacteria have several functions, including production and regulation of phytohormones, release of nutrients to plants (e.g., P-, N-fixation, siderophores, among others), and control of phytopathogens (production of antibiotics and siderophores) (Egamberdieva and Adesemoye 2016; Martı´nez-Viveros et al. 2010; Zahid et al. 2015). Phosphobacteria, phytate-mineralizing bacteria, and phosphatesolubilizing bacteria have been commonly isolated from soil and proposed as inoculants for agricultural improvement (Jorquera et al. 2008). For example, a large diversity of microbes in Andisols under pastures and cereal crops are capable of mineralizing phytate (Jorquera et al. 2008; Martı´nez-Viveros et al. 2010; Menezes-Blackburn et al. 2014). They may be used to develop bacterial or enzyme systems as biofertilizers to overcome the limitations of conventional fertilizers in acidic soils, as well as for developing added value products from agricultural wastes. To this end, Calabi-Floody et al. (2012) studied the effect of enzyme–nanoclay complexes on P availability of composted cattle dung and showed that it increased the inorganic P content. Moreover, Menezes-Blackburn et al. (2014) suggest that inoculation of cattle manure with enzyme–nanoclay complexes enhances the organic P cycling and P nutrition of plants grown in P-deficient soils. Low N acquisition by plants is a limiting factor in agricultural ecosystems, and there is interest in using N2-fixing bacteria as an alternative to conventional fertilization.
Diazotrophic bacteria are capable of converting atmospheric dinitrogen (N2) into NH3, which can be used by plants (Islam et al. 2009). Among them, a number of free-living soil bacteria are considered to be PGPR because of their competitive advantage in C-rich and N-poor environments (Kennedy et al. 2004). Free-living N2-fixing bacteria have been considered as an alternative to conventional N fertilizer for promoting plant growth, and several research studies reported significant increases in grain and shoot biomass yield from plants inoculated with freeliving diazotrophic bacteria (Andrade et al. 2013; Barua et al. 2012; Kennedy et al. 2004; Park et al. 2005). Moreover, Vadakattu and Paterson (2006) reported that under intensive wheat rotation at Avon, South Australia, free-living N2-fixing bacteria contributed 20 kg N per ha per year, which represented 30%–50% of total crop requirements. This response was attributed to a combination of factors including enhancement of root development, production of growth regulators, and N2-fixation (Naiman et al. 2009). However, it is well known that bacteria directly inoculated in the soil system could be adversely affected by competition with native microorganisms, unfavorable physicochemical conditions, and fluctuating pH and temperature (Bréant et al. 2002). Encapsulating microorganisms in carrier materials (bioformulation) is designed to protect them during storage and from adverse environmental condition (pH, temperature, etc.), thus ensuring a gradual and prolonged release (Bashan 1986; Kim et al. 2012).
A wide range of microorganisms have been investigated, and a framework for selecting suitable organisms for specific purposes has been developed. Materials suitable for immobilization and preservation of bacteria include alginate gels, synthetic gels (Sol–Gel), polyacrylamide, agar and agarose, polyurethane, vermiculite, and polysaccharides (Bashan 1998; Liu et al. 2008). In addition, composite materials based on biodegradable polymer clay or nanoclays are being studied, including nanocomposites (Calabi-Floody et al. 2009). For example, encapsulation of free-living diazotrophic bacteria has been considered as one of the possible alternatives for inorganic N fertilizer for promoting plant growth and crop yield (Ivanova et al. 2005).
Plant factory
Plant Factories (also known as indoor farms or vertical farms) are gaining widespread attention over the last few years. Pioneered in Japan, the last few years show an acceleration in the adoption of plant factories in other regions such as the United States, Canada, Europe, and Southeast Asia. The differences between regions are becoming apparent: whereas the United States and Canada focus mainly on the local perspective, in Europe the focus is on optimizing yield and quality, while in Japan it is on food safety and maturing the production technology. Recent advancements in technology, knowledge of plant growth, and market development have resulted in plant factories becoming economically viable as a facility for the year-round production of high-quality leafy vegetables and herbs. Especially LED lighting has proven to be an enabler in this respect.
In plant factories, the plants are grown in multiple layers, in a fully controlled and closed environment, without daylight, and close to the consumer. Mostly, LED lighting is used for growing light as well as steering light. With LED lighting, the light level, spectrum, and photoperiod can be tuned to result in optimum growth in terms of yield and quality (Goto 2012 and Kozai et al. 2015). LEDs have shown to be suitable for multilayer growth because of their form-factor, cool temperature, and high energy efficiency. As a result, they can be located close to the plants (Stutte 2015). The economic viability of plant factories is influenced by a number of factors. Compared to greenhouses, plant factories require large investments. Also, the cost of operation of a plant factory is relatively high (Banerjee and Adenaeuer 2014). However, plant factories are very efficient production facilities: the yearly production per unit of growth area easily exceeds the production in the most advanced greenhouses.
To further improve the viability of plant factories and to be able to monitor the effectiveness of advances in systems and knowledge relevant for growth, it helps to define key performance indicators (KPIs). An important KPI for the efficiency of growth is the one relating biomass production to the integral amount of light used for the production (this KPI is hereafter called light-useefficiency or LUE, expressed in gmol–1). This KPI which can be maximized by an optimal lighting design in combination with a transplanting strategy that maximizes the interception of light by the plants is shown. A model to estimate the theoretical maximum LUE achievable was presented. The approach with specific examples of what had been achieved for lettuce growth and the growth of several types of herbs was illustrated. Since the use of energy in a plant factory represents a considerable fraction of the cost of operation, some detail of a KPI related to the energy efficiency of LED lighting performance indicators for the efficiency of other resources are out of scope (Kozai 2013). Next to a high growth efficiency, resulting in a high production, also crop quality is important. It is not obvious that a high quality can be achieved without compromising a high production. As an example, it was shown that the coloration of lettuce and the reduction of nitrate in lettuce can be achieved by a preharvest lighting strategy such as not to compromise the overall growth efficiency.
Vertical farming
Increase in the worldwide population combined with the rise in per capita consumption of modern man has led to increased demand for food. To meet the growing demands and to feed the masses, more agricultural land is required. This has led to increased pressure on existing farmlands (which are being harvested multiple times in a year) as well as necessitated the acquisition of more land for agriculture. But an increased understanding of the adverse effects of deforestation has led man to think of alternative ways to optimize land use in agriculture. One such solution is vertical farming. This technique involves growing crops in a vertically stacked manner in an indoor controlled environment.
Vertical farming is environmentally friendly and pesticide free. But this technique is highly critical as it requires constant monitoring of the ambient parameters. Vertical farming is usually practiced in large warehouses which can be several storey’s tall. The sunlight is replaced by LED lights. The controlling parameters for vertical farming are mainly ambient light, ambient temperature, soil moisture, and humidity. Several automation techniques have been previously suggested for optimizing resource use, such as accommodation of actuation by automated irrigation systems (Kotamaki et al. 2009, Gutiérrez et al. 2014), but wireless monitoring using IoT in the field of vertical agriculture has not been previously suggested. The ambient parameters must be monitored for each stack along the vertical columns. This requires a set of sensors for each vertical stack. The large amounts of data generated by these sensors need an efficient data management system. Using the IoT, we can efficiently manage the sensor data, identify redundant sensor readings, and visualize it with the help of web-based applications (Gondchawar and Kawitkar 2016). Thus, a convenient IoT-based system may be developed for vertical farming.
CONCLUSION
For precision farming, recent research has focused on the spatial variability of production environments, development of efficient and suitable data management systems, efficiency of various types of image analyses and optical sensing, efficiency of sensors and related technologies, designs of precision agriculture equipment, optimal inputs and service uses and their spatial allocations, potentials of UAV and nano-technologies. For precision livestock farming, by collecting and analyzing vast quantities of data that no person would be able to complete on their own, precision livestock farming can provide producers with information about the welfare of the whole herd as well as individual animals. The continued development of precision livestock farming and the possibilities for interconnection in the livestock value chain, ultimately links consumers and farmers. Consumers will make decisions based on farm practices, and farms can make decisions based on consumer practices. For vertical farming, research has often highlighted the variations in technologies based on out-door / indoor systems, ways to improve plants’ access to light (natural or artificial), growing medium and nutrient / water supply, advanced features like electricity generation and integration of production space into office / residential space, and water treatment methods. As for soil monitoring system, Zigbee-based agriculture monitoring system serves as a reliable and efficient system for monitoring agricultural parameters. Wireless monitoring of field will not only allow user to reduce the manual labour, but also to see accurate changes in it. It is cheaper in cost and consumes less power. Such a system can be easily installed and maintained. For low-income South-Asian countries like India, these may be some of the key areas that the country can draw on from other more advanced countries in Asia, or focus in investing further research to improve the relevance of the technologies for the country.
REFERENCES
AAPFCO, 1995. Official Publication No. 48. Published by Association of American Plant Food Control Officials. Association of American Plant Food Control Officials (AAPFCO). Inc., West Lafayette, Indiana, USA.
Ahmad, F., Ahmad, I. and Khan, M.S., 2008. Screening of free-living rhizospheric bacteria for their multiple plant growth promoting activities. Microbiological Research 163: 173–181.
Akubattin, V.L., Bansode, A.P., Ambre, T., Kachroo, A. and SaiPrasad, P., 2016. Smart Irrigation System.
International Journal of Scientific Research in Science and Technology 2: 343-345.
Al-Ghobari, H.M., Mohammad, F.S. and El Marazky, M.S.A., 2017. Evaluating two irrigation controllers under subsurface drip irrigated tomato crop. Spanish Journal of Agricultural Research 14(4): 1206 Anderson, C., 2014. “Agricultural Drones, MIT Technology Review,” [Online]. Available:
https://www.technologyreview.com/featuredstory/526491/agricultural-drones
Andrade, M.M.M., Stamford, N.P., Santos, C.E.R.S., Freitas, A.D.S., Sousa, C.A. and Lira, J.M.A., 2013. Effects of biofertilizer with diazotrophic bacteria and mycorrhizal fungi in soil attribute, cowpea nodulation yield and nutrient uptake in field conditions. Scientia Horticulturae 162: 374–379.
Ariff, M.H., Ismarani, I. and Shamsuddin, N. 2014. RFID based systematic livestock health management system. In Proceedings of the 2014 IEEE Conference on Systems Process Control (ICSPC), Kuala Lumpur, Malaysia,12–14 December 2014, pp. 111–116.
Banerjee, C. and Adenaeuer, L., 2014. Up, up and away! The economics of vertical farming. Journal of Agricultural Studies 2:40–60.
Banhazi, T.M., Lehr, H., Black, J.L., Crabtree, H., Schofield, P., Tscharke, M. and Berckmans, D., 2012. Precision Livestock Farming: An international review of scientific and commercial aspects. International Journal of Agricultural and Biological Engineering 5: 1–9.
Bargeron, C.T., and Moorhead, D.J., 2007. EDDMapS- Early Detection and Distribution Mapping System for the Southeast Exotic Pest Plant Council. Wildland Weeds 10(4):4-8.
Barua, S., Tripathi, S., Chakraborty, A., Ghosh, S. and Chakrabarti, K., 2012. Characterization and crop production efficiency of diazotrophic bacterial isolates from coastal saline soils. Microbiological Research 167: 95–102.
Bashan, Y., 1986. Alginate beads as synthetic inoculant carriers for slow release of bacteria that affect plant growth. Applied and Environmental Microbiology 51(5): 1089–1098.
Bashan, Y., 1998. Inoculants for plant growth promoting bacteria for use in agriculture. Biotechnology Advances 16: 729–770.
Borghi, E., Avanzi, J.C., Bortolon, L., Junior, A.L. and Bortolon, E.S. 2016. Adoption and use of precision agriculture in Brazil: Perception of growers and service dealership. Journal of Agricultural Science 8 (11): 89.
Boselin, P.S.R., Pradeep, M. and Gajendran, E., 2017. An analysis of smart irrigation system using wireless sensor network. Star International Journal 5 3(3): 12-17
Bramley, R., and Trengove, S.A.M., 2013. Precision agriculture in Australia: Present status and recent developments. Engenharia Agrícola 33 (3): 575-588.
Bréant, D., Jézéquel, K. and Lebeau, T., 2002. Optimization of the cell release from immobilized cells of Bacillus simplex cultivated in culture media enriched with Cd2+: influence of Cd2+, inoculum size, culture medium and alginate beads characteristics. Biotechnology Letters 24: 1237–1241.
Butzen, S., 2013. Common nitrogen fertilizers and stabilizers for corn production. In: Crop Insights. vol. 23.
DuPont Pioneer, Johnston, Iowa. No. 2.
Calabi-Floody, M., Theng, B.K.G., Reyes, P. and Mora, M.L., 2009. Natural nanoclays: applications and future trends—a Chilean perspective. Clay Minerals 44 (16): 1–176.
Calabi-Floody, M., Vela´squez, G., Gianfreda, L., Sa ggar, S., Bolan, N., Rumpel, C. and Mora, M.L., 2012. Improving bioavailability of phosphorous from cattle dung by using phosphatase immobilized on natural clay and nanoclay. Chemosphere 89: 644–655.
Chen, X., Luo, Q., Jiang, Y., Lv, Z., and Wu, S. 2008. A WebGIS Expert System for Rice Brown Planthopper Disaster Early-Warning in China's Shanghai. Bioinformatics and Biomedical Engineering, 2485-2488.
De Montis, A., Modica, G. and Arcidiacono, C., 2017. Aginformatics. In Encyclopedia of Big Data, AG2017 ed.; Schintler, L.A.; McNeely, C.L., Eds.; Springer: New York, New York, USA, pp. 1–4. Doi: 10.1007/978-3-319-32001-4.
DeShazer, J.A., Moran, P., Onyango, C.M., Randall, J.M. and Schofield, C.P. 1988. Imaging Systems to improve Stockmanship in Pig Production; AFRC Institute of Engineering Research: Bedfordshire, UK.
Dhumane, A. V., Prasad, R. S. and Prasad, J. R., 2017. An Optimal Routing Algorithm for Internet of Things Enabling Technologies. International Journal of Rough Sets and Data Analysis 4(3):1-16. doi:10.4018/IJRSDA.2017070101
Dlodlo, N. and Kalezhi, J. 2015. The internet of things in agriculture for sustainable rural development. In: 2015 International Conference on Emerging Trends in Networks and Computer Commununication (ETNCC), pp 13–18.
Dominic, 2010. Natural Disaster Monitor. Retrieved from Android 15 November 2010.
Du, C.W., Zhou, J.M. and Shaviv, A., 2006. Release characteristics of nutrients from polymer coated compound controlled release fertilizers. Journal of Polymers and the Environment 14: 223–230.
Egamberdieva, D. and Adesemoye, A.O., 2016. Improvement of crop protection and yield in hostile agroecological conditions with PGPR-based biofertilizer formulations. In: -Arora, N.K., Mehnaz, S., Balestrini, R. (Eds.), Bioformulations: For Sustainable Agriculture. Springer, India, pp. 199–211.
Foster, A., 2013. Development of Biocompatible Aptamer Films as Smart Materials for Novel Fertilizer Systems. Master Thesis, Carleton University, Ottawa, Canada.
Gang, L.L.L. 2006. Design of greenhouse environment monitoring and controlling system based on bluetooth technology. Transactions of the Chinese Society for Agricultural Machinery 10: 97–100
Georgiou, O. and Raza, U., 2017. Low Power Wide Area Network Analysis: Can LoRa Scale? IEEE Wireless Communication Letters PP. 10.1109/LWC.2016.2647247.
Gondchawar, N. and Kawitkar, R.S., 2016. IoT based smart agriculture. International Journal of Advanced Research in Computer and Communication Engineering 5(6): 838-842
Goto, E., 2012. Plant production in a closed plant factory with artificial lighting, VII International Symposium on Light in Horticultural Systems, Vol. 956, pp. 37–49.
Gutiérrez, J., Villa-Medina, J.F., Nieto-Garibay, G. and Porta-Gándara, M.A., 2014. Automated irrigation system using a wireless sensor network and GPRS module. IEEE Transactions on Instrumentation and Measurement 63:166–176
Hostetler, C., Stokes, A. and Becton, L., 2018. National Pork Board Request for Proposals General Call 2018 Writing Research Proposals for the NPB The National Pork Board Is Soliciting Research Proposals Dealing with These Categories; National Pork Board: Ames, Iowa, USA; pp. 1–19.
Huang, H., Bian, H., Zhu, S. and Jin, J., 2011 A greenhouse remote monitoring system based on GSM. In: 2011 International Conference on Information Management, Innovation Management and Industrial Engineering (ICIII), Vol. 2, pp. 357–360.
Islam, M.R., Madhaiyan, M., Boruah, H.P.D., Yim, W., Lee, G., Saravanan, V.S., Fu, Q., Hu, H. and Sa, T., 2009. Characterization of plant growth-promoting traits of free-living diazotrophic bacteria and their inoculation effects on growth and nitrogen uptake of crop plants. Journal of Microbiology and Biotechnology 19 (10): 1213–1222.
Ivanova, E., Teunou, E. and Poncelet, D., 2005. In: Gruev, B., Nikolova, M., Donev, A. (Eds.), Alginate based macrocapsules as inoculants carriers for production of nitrogen biofertilizers. Proceedings of the Balkan Scientific Conference of Biology in Plovdiv (Bulgaria), pp. 90–108.
Jorquera, M., Herna´ndez, M., Rengel, Z., Marschner, P. and Mora, M.L., 2008. Isolation of culturable phosphobacteria with both phytatemineralization and phosphatesolubilization activity from the rhizosphere of plants grown in a volcanic soil. Biology and Fertility of Soils 44: 1025–1034.
Kalubhai, P., 2015. Present Status and Prospects of Micro Irrigation System in Mahisagar District of Gujarat. Doctoral dissertation, Anand Agricultural University, Anand, India.
Kennedy, I.R., Choudhury, A.T.M.A. and Kecske´s, M.L., 2004. Non-symbiotic bacterial diazotrophs in crop-farming systems: can their potential for plant growth promotion be better exploited? Soil Biology and Biochemistry 36: 1229–1244.
Keskin, M., and Sekerli, Y.E., 2016. Awareness and adoption of precision agriculture in the Cukurova region of Turkey. Agronomy Research 14 (4): 1307-1320.
Kim, S.Y., Kim, M., Lee, S., Lee, J. and Ko, G., 2012. Survival of microorganisms on antimicrobial filters and the removal efficiency of bioaerosols in an environmental chamber. Journal of Microbiology and Biotechnology 22 (9): 1288–1295.
Kotamaki, N., Thessler, S., Koskiaho, J., Hannukkala, A.O., Huitu, H., Huttula, T., Havento, J., Jarvenpaa,
M. 2009. Wireless in-situ sensor network for agriculture and water monitoring on a river basin scale
in Southern Finland: evaluation from a data user’s perspective. Sensors 4:2862–2883
Kozai, T., Niu, G. and Takagaki, M., (Eds.), 2015. Plant Factory: An Indoor Vertical Farming System for Efficient Quality Food Production, Elsevier, Amsterdam.
Kozai, T. 2013. Resource use efficiency of closed plant production system with artificial light: concept, estimation and application to plant factory, Proceedings of the Japan Academy Series B 89: 447–461.
Li, R.A., Sha, X. and Lin, K., 2014. Smart greenhouse: a real-time mobile intelligent monitoring system based on WSN. In: Wireless Communications and Mobile Computing Conference (IWCMC) 2014 International, pp. 1152–1156.
Liu, C.H., Wu, J.Y. and Chang, J.S., 2008. Diffusion characteristic and controlled release of bacterial fertilizer from modified calcium alginate capsules. Bioresource Technology 99: 1904–1910.
Luo, H., Yang, P., Li, Y., Xu, F., 2012. An intelligent controlling system for greenhouse environment based on the architecture of the internet of things. Sensor Letters 10(1–2): 514–522
Martı´nez-Viveros, O., Jorquera, M.A., Crowley, D.E., Gajardo, G. and Mora, M.L., 2010. Mechanisms and practical considerations involved in plant growth promotion by rhizobacteria. Journal of Soil Science and Plant Nutrition 10(3): 310–339.
Mastronardi, E., Tsae, P., Zhang, X., Monreal, C.M. and DeRosa, M.C., 2015. Strategic role of nanotechnology in fertilizers: potential and limitations. In: Rai, M., Ribeiro, C., Mattoso, L., Duran, N. (Eds.), Nanotechnologies in Food and Agriculture. Springer, Cham, Switzerland, pp. 25–67.
Menezes-Blackburn, D., Jorquera, M.A., Gianfreda, L., Greiner, R.L. and Mora, M.L., 2014. A novel phosphorus biofertilization strategy using cattle manure treated with phytase–nanoclay complexes. Biology and Fertility of Soils 50: 583–592.
Mondal, P., and Basu, M., 2009. Adoption of precision agriculture technologies in India and in some developing countries: Scope, present status and strategies. Progress in Natural Science 19(6): 659-666. Moorhead, C. T., 2007. Early Detection and Distribution Mapping System for the Southeast Exotic Pest
Plant Council
Naiman, A.D., Latro´nico, A. and Garcı´a de Salamone, I.E., 2009. Inoculation of wheat with Azospirillum brasilense and Pseudomonas fluorescens: impact on the production and culturable rhizosphere microflora. European Journal of Soil Biology 45: 44–51.
National Research Council. 1997. Precision Agriculture in the 21st Century: Geospatial and Information Technologies in Crop Management. Committee on Assessing Crop Yield: Site-Specific Farming, Information Systems, and Research Opportunities. Washington DC: National Academies Press. http://www.nap.edu/catalog/5491/precision-agriculturein-the-21st-century-geospatial-and-informatio n-technologies
Naz, M.Y., Sulaiman, S.A., 2016. Slow release coating remedy for nitrogen loss from conventional urea: a review. Journal of Controlled Release 225(10): 109–120.
Neethirajan, S., 2017. Recent advances in wearable sensors for animal health management. Sensing and Bio-Sensing Research 12: 15–29. doi:10.1016/j.sbsr.2016.11.004.
Ni, B., Liu, M. and Lu, S., 2009. Multifunctional slow-release urea fertilizer from ethylcellulose and superabsorbent coated formulations. Chemical Engineering Journal 155: 892–898.
Park, M., Kim, C., Yang, J., Lee, H., Shin, W., Kim, S. and Sa, T., 2005. Isolation and characterization of diazotrophic growth promoting bacteria from rhizosphere of agricultural crops of Korea. Microbiological Research 160: 127–133.
Paustian, M., and Theuvsen, L., 2017. Adoption of precision agriculture technologies by German crop farmers. Precision Agriculture 18(5): 701–716.
Puri, M., Solanki, A., Padawer, T., Tipparaju, S.M., Moreno, W.A. and Pathak, Y., 2015. Introduction to Artificial Neural Network (ANN) as a Predictive Tool for Drug Design, Discovery, Delivery, and Disposition: Basic Concepts and Modeling; Elsevier Inc.: Amsterdam, the Netherlands, pp. 3–13. doi:10.1016/B978-0-12-801559-9.00001-6.
Rachana, P.K. and Suhas, D.R., 2014. Android Application Agriculture Decision Support System. Int.
Journal of Engineering Research Applications, 63-66.
Ranade, P. and Takale, S.B., 2016. Smart irrigation system using FPGA based wireless sensor network.
IEEE Proceeding 15: 223–224
Roy, I., Gupta, M.N., 2003. Smart polymeric materials: emerging biochemical applications. Chemistry & Biology 10(12): 1161–1171.
Schimmelpfennig, D., 2016. Farm Profits and Adoption of Precision Agriculture (No. 249773). Washington, DC: United States Department of Agriculture, Economic Research Service.
Sharma, S., Raval, J., and Jagyasi, B., 2013. Mobile sensing for agriculture activities detection. 2013 IEEE Global Humanitarian Technology Conference (GHTC).
Shaviv, A., 2005. Controlled Release Fertilizers, IFA International Workshop on Enhanced-Efficiency Fertilizers, Frankfurt. International Fertilizer Industry Association, Paris, France.
Stutte, G.W., 2015 Commercial transition to LEDs: a pathway to high-value products. Hortscience 50: 1297–1300
Taylor, J., 2018. Capital Growth: Precision Agriculture and Vertical Farming in the Corporate Food Regime. New York: CUNY Academic Works.
Trenkel, M.E., 1997. Improving Fertilizer Use Efficiency: Controlled-Release and Stabilized Fertilizers in Agriculture, the International Fertilizer Industry Association, Paris. International Fertilizer Industry Association (IFA), Paris, France, p. 151.
Tyagi, A., Gupta, N., Navani, J.P., Tiwari, M.R. and Gupta, M.A., 2017. Smart Irrigation System.
International Journal for Innovative Research in Science & Technology 3: 9-12.
Vadakattu, G. and Paterson, J., 2006. Free-living bacteria lift soil nitrogen supply. Farming Ahead 169: 40 Watcharaanantapong, P., Roberts, R.K., Lambert, D.M., Larson, J.A., Velandia, M., English, B.C., Rejesus,
R.M. and Wang, C., 2014. Timing of precision agriculture technology adoption in US cotton production. Precision Agriculture 15(4): 427-446
WSI, 2008. Stormtracker 2.8.3. Android 2.3.3
Xiaofeng, L., Linlin, Q., Linjian, L. and Gang, W. 2015. Design and implementation of modern greenhouse remote monitoring system based on the Android system. In: 34th Chinese Control Conference (CCC), pp. 5742–5746.
Xu, Z., Chen, J., Wang, Y. and Fan, Z., 2016. A remote monitoring system for greenhouse based on the internet of things. In: MATEC Web of Conferences, EDP Sciences 77:1-4
Yan-E, D., 2011. Design of intelligent agriculture management information system based on IoT. In: Proceedings of the 4th International Conference on Intelligent Computation Technology Automation ICICTA 2011, Vol. 1, pp 1045–1049
Zahid, M., Abbasi, M.K., Hameed, S. and Rahim, N., 2015. Isolation and identification of indigenous plant growth promoting rhizobacteria from Himalayan region of Kashmir and their effect on improving growth and nutrient contents of maize (Zea mays L.). Frontiers in Microbiology 6(207): 1–10.
Zhang, X., Zhang, C., Fang, J., Yu, X. and Liang, J., 2009. Smart sensor nodes for wireless soil temperature monitoring systems in precision agriculture. Transactions of the Chinese Society for Agricultural Machinery 40: 237-240.