ABSTRACT
Drone application in Taiwan is becoming popular practices. Several kinds of drone’s application in agriculture, forestry, fishery and mountainous rural security management, such as: land management, land cover monitoring, crop and fishery damage assessment of natural hazards, rural 3D landscape and pesticides spray and assessment have conducted by Council of Agriculture.The major task of this paper is reported that some development status of low-altitude and low-volume drones’ technology for plant protection in Taiwan. At moment, the strategies of UAV application in agriculture in Taiwan are focus on establishing the characteristics of spray systems that is suitable for different types of Drones and crop, and evaluating the safety of pesticide dosages. Applying drone with kinds of spectral sensors and location-based function to collect the information of pests and diseases invasive and development on crop, that is, has been identified spectral information of crop samples before symptoms were observed, and identifying the disease status in crops through characteristic bands, which will be become an effective early warning information for precision agriculture of crop pests and diseases prevention actions. The bacterial leaf blight of rice in the second crop of 2017 was collected by using a drone equipped with a 400 to 1000 nm hyperspectral camera, and applied the Spectral Information Divergence (SID) and Normalized Difference Vegetation Index (NDVI) with K-Means algorithm for data clustering and quantification. In the future, using UAS with insects and spore traps to capture pathogen spores in the air, combined with different algorithms for pest and disease early warning model construction, will make the prediction accuracy of pests and diseases more accurate and will make a progress on the pest and disease warning, combined with a variety of technologies such as: IoT and prediction models to improve the coverage and accuracy of agricultural disease prediction.
Keywords: Drone, pesticides spray assessment, crop damage assessment of natural hazards
INTRODUCTION
One of the latest developments is the increase in the use of small, unmanned aerial vehicles (UAVs), commonly known as drones, for agriculture (FAO, 2018). On the farm, yield measurements, forage quality evaluation, weed control effectiveness, and plant nutrient status are some of the popular data collection methods used to measure these risks. These methods rely on such gadgets as portable meters, test strips, manual stand counts, chlorophyll meters, seed sampling, and color charts for plant tissues. Farmers map these farms according to such historical records as soil analysis, crop rotation and pest infestation. Drones have inbuilt sensors, navigation systems and recording devices that single out trouble spots in large farms at a bird’s eye view. They can pick out anomalies that are difficult to see while on the ground. The data collected is of high resolution at an affordable cost. The UAV’s are capable of translating the raw data using complex formulas and present practical statistics to the farmer. The drone’s capabilities are not limited to just pinpointing problem areas. They are useful in monitoring ambitious growth plans undertaken by farmers. Such plans include data collection in a horticultural farm.
As a consequence of population aging and shrinkage in Asian countries, the trend of labor shortage for agricultural production has grown and will continue in future (He et al., 2017). Meantime, the new circumstances of modern agricultural construction are those making agriculture realize the goal of high yield, high quality, high efficiency, ecology and safety, realizing green consumption and sustainable development, and building resource-saving and environmental-friendly agriculture. Therefore, in order to adapt these new circumstances, agricultural UAV is developed quickly in Asia, providing powerful support for promoting agricultural production security, quality and safety of agricultural products, agricultural ecological security and agricultural trade security (He et al., 2010, 2012).
The following are some of the ways in with UAVs are used in crop data collection: The extent of pest and fungal infestation; maps relating to plant anomalies, leaf coverage, flowering, the impact of insecticide application; plant growth surveillance – monitor the weather conditions; Irrigation – Drones are single out fields where water levels need to be increased or reduced. Upon the crop growth, the drones collect data relating to plant density, health and heat emission. Data collection is a crucial aspect of farm management and growth. In the past, farmers have employed weak data collection tools that led to poor decisions and huge losses. Even for large scale growers who engaged experts in the field in collecting accurate data, such data collection would take months to collect. By the time the data was ready for use, it had become irrelevant. Since the early 80’s when the first drones were put into commercial use, there has been and increasing number of farmers using the drones in monitoring their crop production. Now, the UAV has become a data collection tool of choice in the agribusiness sector. In the ensuing discussion, we shall analyze the effectiveness of the use of the unmanned aerial vehicle to collect crop data. The unmanned aerial vehicles use in crop data collection is therefore expected to increase. This increase will be at a much slower pace because of the tough economic conditions prevailing in the agribusiness industry. Mechanization of farms makes the drones an economical option. They now have advance data gathering features and software packages that are updated regularly.
A number of successful studies have been conducted using small, lightweight cameras on board drone and the research area is given a lot of attention worldwide (Nebiker et al. 2008; Bergo et al. 2010; Laliberte al. 2011; Zarco Tejada et al. 2012). For remote sensing applications in precision agriculture (PA), drones are very useful autonomous vehicles. Drone’s applications for localizing weeds and diseases, determining soil properties, detecting vegetation differences and the production of an accurate elevation models are currently possible. In current PA applications, there are some image processing methods that are used to determine the soil and plant characteristics. For this, the drone technology is a powerful method to meet the required resolution levels. Drones have recently become the subject of a number of studies that evaluate their applicability for PA applications.
Application of crop production and protection materials is a crucial component in the high productivity of agriculture. Drones will enable farmers to know more about their fields. UAV spray pesticides has been developed and applied in the fields more than 30 years. In recently years, multi-rotor drone crop protection operations have experienced tremendous growth. Compared with manual operations, UAV has advantages such as high operational efficiency, small pesticides dosages, and low pesticides hazards to human (He et al., 2017). Therefore, farmers will be assisted with producing more food while using fewer chemicals. Agricultural chemical application tried to achieve the aims of spraying pesticides at specific times and locations for accurate site‐specific management of crop pests. The developed spray system has the potential to provide accurate, site‐specific crop management when coupled with UAV systems recently. It also has great potential for vector control in the areas that are not easily accessible by personnel or equipment (Huang, et al. 2009).
UAS are getting increasingly popular in agricultural and forestry insofar as they represent a cost-effective and readily available tool for surveying land and crops, with the purpose of acquiring data for further analysis and to support decision-making and management processes (Telmo Adão et al., 2017). UAVs imagery systems are capable to fill the gap between aerial and terrestrial imagery systems and enhance the use of imagery systems and remote sensing for PA applications. UAVs provide real time and high-quality aerial imagery compared to satellite imagery over agricultural areas. These have a huge potential in agriculture in supporting evidence-based planning and in spatial data collection. Despite some inherent limitations, these tools and technologies can provide valuable data that can then be used to influence policies and decisions. The advantages that “an eye in the sky” provides when combined with analytic tools that can interpret the data and images to actionable information have ushered in a new revolution. However, priority in addressing issues related to privacy, safety and security is the key to the sustainable implementation of these technologies (FAO, 2018).
Hyperspectral Imaging (HSI) is an emerging imaging that contains hundreds of contiguous narrow spectral bands covering a wide range of the electromagnetic spectrum from the visible to the infrared domain (Chang, 2007). Hyperspectral sensors usually generate huge amounts of data since they retrieve spectra sets composed of hundreds of bands across considerable spatial resolution images (Telmo Adão et al., 2017). Such dimensionality demands proper analysis and processing methods. Hyperspectral data processing must be performed towards the retrieval of relevant information, which provides the true benefits for assertive interventions in agricultural crops areas. Hyperspectral-based remote sensing supported by UAV platforms, a survey including hyperspectral sensors, inherent data processing and applications focusing both on agriculture—wherein the combination of UAV and hyperspectral sensors plays a center role (Telmo Adão et al., 2017).
The UAV can automatically flight at low altitudes and with large overlaps, which permit the acquisition of ultra-high spatial resolution images and the generation of the Digital Surface Model (DSM) using automatic photo-reconstruction methods that are based on the “Structure from Motion” approach for 3-D reconstruction. This work presents an innovative procedure for computing the 3-dimensional geometric features of individual trees and tree-rows by applying image analysis techniques. The geometric features of fruit trees such as canopy area, fruit tree height and crown volume provide useful information about plantation status and fruit crop production. The maps generated could be used to understand the linkages between fruit tree grown and field-related factors or to optimize crop management operations in the precision agriculture (Bendig et al., 2013; Díaz-Varela et al., 2015; Torres-Sánchez et al., 2015; Zarco-Tejada et al., 2014).
STATUS OF DRONE APPLICATION IN THE AGRICULTURAL SECTOR OF TAIWAN
Drone’s application in agriculture, forestry, fishery and mountainous rural security management, such as: land management, land cover monitoring, crop and fishery damage assessment of natural hazards, rural 3D landscape and pesticides spray and assessment have conducted by Council of Agriculture. Soil and Water Conservation Bureau of COA utilizes drones with geospatial imagery technology, such as: DSM (Digital Surface Model) and ortho-photograph of AOI (area of interesting) to enhance disaster preparedness and the response activities to the ministry. The activities also generate useful information related to upland agricultural risks such as landslides and erosion that could be used to inform agricultural communities and help them understand the risks and reduce the impacts of any disaster. The Forestry Bureau of COA uses drones to capture images for forest management of cases identification (to prevent illegal logging of trees), driftwood, and monitoring forest fires of barrier lakes after typhoons or earth quakes. The Fisheries Agency of COA developed the technologies for fishing vessels to apply drones to survey fishing groups, assist in salvage operations and conduct aquaculture surveys and management after natural disasters.
Drone also has been applied in Taiwan agriculture that is extending in crop monitoring, pest early warning systems, natural disaster damage assessments, are presented as the followings.
PESTICIDES SPRAY
In recent years, due to the aging of the rural population in Taiwan, the agricultural manpower has been in short supply, and the agricultural productivity has been seriously affected. Application of agricultural spraying and foliar fertilizing drones to save a lot of labor, time saving, water saving, medicine saving, safe use of pesticides, production of safe agricultural products, and promotion of precision farming management of small farmers is the focus of application research of drones aims. Drones, as emerging plant protection machinery, have the advantages of high operational efficiency, high speed, and low drift.
Public media reported that Taiwan have about 1000 young farmers own spray drones and served for other farmers in the field. And another 1000 young farmers will join in this service in the near future. Some professional UAV operation regulation has been announced, the agricultural drone spray pesticides regulation also is under construction. However, the national standard operating procedures for drone spray pesticides are lacking in the field. It is urgently necessary to build and standardize the operational technical specifications for UAV safely spray pesticides and keep the food products safety.
The spraying of agricultural chemicals by using drone speeds up the process and prevents compacting of the soil. However, adverse weather conditions (e.g. the speed and direction of the wind) can impair the effectiveness of the spraying of pesticides in a target crop field. There is a risk that the pesticide can drift to neighboring crop fields. It is believed that a large amount of all the pesticide used in the world drifts outside of the target crop field and only a small amount is effective in controlling pests. The current study aimed to elucidate the characteristics of droplet distribution and drift, control efficiency on crop insects, and attachment and absorption of crop leaves during drone spraying. Field tests have been conducted to evaluate spread factors of pesticides spray formulations on Kromekote cards. The drone spraying test aimed to study the droplet deposition and drifting regularity of the crops by drone spraying. Before spraying, a handheld GPS locator was used to determine the coordinates of each spraying zone.
There are four factors of drone spraying pesticides that affect the adhesion of pesticide on targets (Figure 1). Since the nozzle direction and nozzle pressure are not adjustable, the variation of drone spray pesticides effectiveness is based on drone flight height and flight speed. In order to obtain the effective spraying rate, the maximum flying speed when spraying (requirement of the adhesion specification) and the spray swath of the flight line must be obtained, wherein the swath of the next flight line needs to be evaluated the effectiveness of adhesive droplets on targets according to the spray droplets distribution curve (Figure 2). Kromekote card and filter paper are used as samplers to collect droplets, and the droplet density, coverage rate, deposition, and drift percentage are analyzed. The pooled results showed that the droplet uniformity, the droplet coverage rate, the deposition, and the drifting ability were higher when the drone flight height was 2 m (Lou et al., 2018).
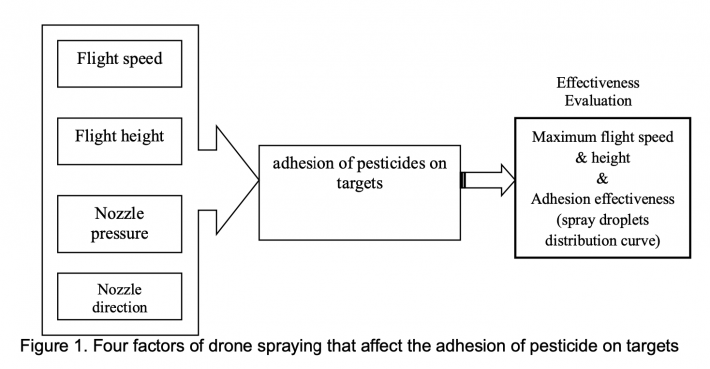
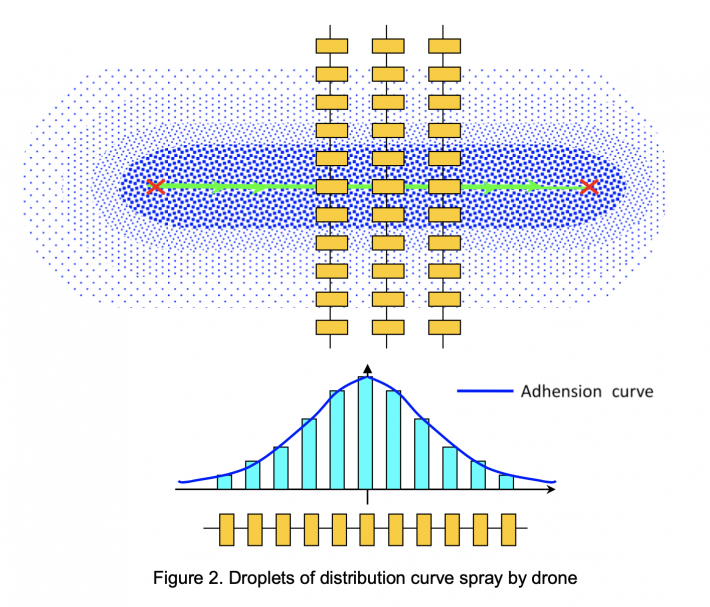
More than 12 kinds of crops that compared with drone spray and manual operation pesticides to control pest experiments were conducted. The drone spray pesticide dose level ranges from 1X to 1/8X. According to the whole season production period of winter season head lettuce, the number of monitored pests of different pests was statistically analyzed as follows: Aphids, there was no difference of control effect between manual and drone spray, and drone spray used 1/8 dose level of pesticide had a similar effectiveness of manual spray pesticide. Whitefly, there is no difference of control effect between manual and drone spray, but the number of whiteflies was no difference between different dose levels. Hummer, there is a difference of control effect between manual and drone spray, and there is no difference between different dose level. On maize experiments (Figure.3), drone spray used 1/3 dose of pesticide has the similar control effect of manual spray. Drone spray used 1/16 dose of pesticide has the similar control effect of manual spray on dragon fruit experiment. But drone spray control effect was failed when it applied on loofah due to the crop leaves structure and cultivate type. Not every crop is suitable for UAV spraying, crop shapes should be carefully selected. The erect type plant and cultivation mode do not hinder the penetration of the drug solution. In addition to the routine control of UAV application, the key hazard sites should grasp the prevention time and adopt manual spraying. The wet rot pathogen of the dragon fruit flower will remain in the fruit. If it is not properly controlled after the rain, the humidity will increase in the carton after harvesting, and it will cause corruption and cause the base rot of the inferior red dragon fruit base. Applying with UAV It is difficult to spray, and manual spray can ensure the control effect. The team evaluated the drone spraying operations on several major crops application. The best benefit is the drone spraying on the dragon fruit orchard as an example, under the current pesticide use regulations, it saves 80% of pesticides and water consumption compared with traditional spraying, and saves 70% of the time.
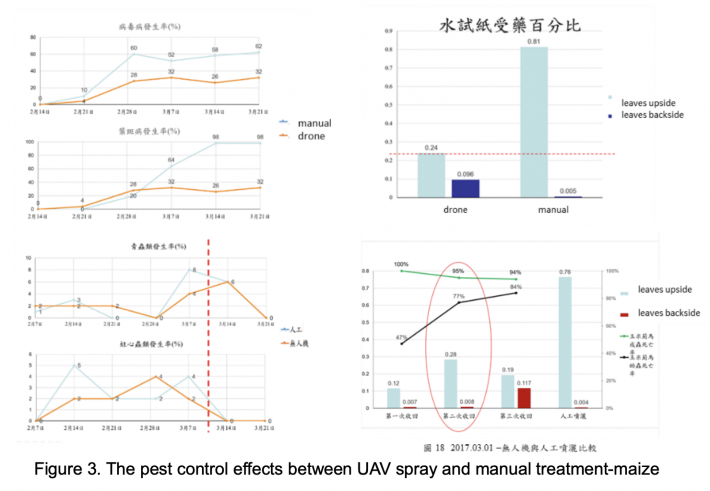
The team of Taiwan Agricultural Research Institute carried out various drone spraying tests. The drone spraying pesticides was evaluated and it could be used to replace manual spray pesticides. The benefits of drone spray pesticides can save labor and time, less hurt human health, less pesticides consumption, less pesticides residual in crop, and achieving food safety goals. The team is committed to the establishment of the Standard Operating Procedure (SOP) of drone spray pesticides for various crops without changing the current pesticide management regulations and the concentration of pesticides use, and provides farmers with the specific operational references. The growth characteristics of crops affect the effect of drone spraying. The current equipment and technology still have space for improvement. The team is currently assisting the drone spray testing and verification of agricultural enterprises such as sweet corn and export lettuce, and they are sure to be recognized by the industry and actively. A large area of rice pest control by drone spray pesticides demonstration project was carried out and cooperated with Tainan City government with GIS and logistics for drone batteries and pesticides supply.
Further research will be aimed on the tiny droplets produced during drone spraying for crop protection also are affected by the rotors air flow and will drift in all directions in an uncontrollable manner, severely affecting the pesticide deposition pattern and resulting in pesticide waste. To improve pesticide use efficiency during multi-rotor drone spraying, an electrostatic spray system should be designed based on electrostatic spray technology and a six-rotor drone.
CROP PRODUCTION MONITORING
The next agricultural revolution will be driven by data, which will help to increase agricultural productivity with minimum damage to the environment and increased livelihoods for agricultural communities. Drone can be used to determine the impact of pest infestation on the crops of in a farm. It was flown over a wide acreage farm to take aerial pictures of the field. These pictures are either streamlined to store in a microchip for further analysis. The analysis results in a crop stress map with graphics showing the impact of the pest invasion. These captured images and transferred data into information, drones allow for permanent monitoring of a crop from planting to harvest. They can also help farmers react faster to threats, such as weeds, pests and stresses; save time crop scouting to take appropriate actions; and improve the application of variable input rates in real time.
The use of drone for agriculture is a valuable source of information on the agriculture practices. Drones have the power that comes from the strength of data processing and analytics that take place after the data is collected. The use of small drones for agriculture as well as access to platforms that can aggregate data from various sources to provide valuable insights would be greatly beneficial to farming managements. Drones are used for aerial survey, high-resolution aerial photos, mapping and land surveying, and handles data that are used to track crop growth stages, weeds, compaction, storm damage and more. Commercial software packages offer an integrated platform to use data from drones, sensors and other devices to automate and optimize farm management. The software converts multispectral images into accurate reflectance and index maps, such as NDVI, and uses red, green and blue (RGB) images to generate high-resolution ortho-mosaics.
Multispectral and hyper-spectral aerial and satellite imagery helps in creating Normalized Difference Vegetation Index (NDVI) maps, which can differentiate soil from grass or forest, detect plants under stress, and differentiate between crops and crop stages. There are strong correlations between crop yield and NDVI data measured at certain crop stages (Huang, Wang, Li, Tian and Pan, 2013). Drones fitted with infrared, multispectral and hyperspectral sensors can analyze crop health and soil conditions precisely and accurately. NDVI data, in combination with other indexes such as the Crop-Water Stress Index (CWSI) and the Canopy-Chlorophyll Content Index (CCCI) in agricultural mapping tools can provide valuable insights into crop health. Precision farming combines sensor data and imaging with real-time data analytics to improve farm productivity through mapping spatial variability in the field. Data collected through drone provide lots of raw data to activate analytical models for agriculture. In supporting precision farming, drones can do soil scans, monitor crop health, assist in planning irrigation schedules, apply fertilizers, estimate yield data and provide valuable data for weather analysis. Data collected through drones combined with other data sources and analytic solutions provide actionable information. Most of the concepts were become true and applied in the field. Precision agriculture becomes available in Asian small holder farming.
In Taiwan, there are 75 diseases that occur during rice cultivation. At present, the main diseases of rice in first crop is rice blast and in second crop is bacterial leaf blight. It is serious economic losses for cutting yield as much as 10-30%. In order to effectively monitor the occurrence of rice diseases, the current monitoring mechanism for rice diseases in Taiwan relies on field investigations conducted by professionals to collect the damage status of rice. TARI team conducted several projects of crops monitoring by drone. UAV equipped with high resolution optical camera, hyperspectral camera, and thermal camera for capturing imagery. The total aerial photography of the drone has been captured more than 40000 hectares (including vegetable production monitoring), with 150 flights each year. These imageries were served as ground truth of sampling sites of land cover databases for satellite image analysis. The process is time-consuming and ineffective to monitor rice production. Due to the development of remote sensing technology in recent years, resource satellite images, aerial images and the unmanned aerial vehicle (UAV) images are a fast and comprehensive way to obtain information. However, there can be applied in different fields by the different sensor.
Hyperspectral camera can perform imaging through the spectrum of hundreds of bands and find the corresponding bands of various targets through hyperspectral analysis. For example, in the second crop of 2017, the bacterial leaf blight was taken as an example. The UAV was equipped with a 400-1000 nm hyperspectral camera to collect information on the damage of bacterial leaf blight, and the Spectral Information Divergence (SID) was applied (Figure 4). Normalized Difference Vegetation Index (NDVI) and K-Means algorithm are used for data grouping and quantification to achieve automated rice bacterial blight damage area. In the future, Technology to conduct a wide range of disease area monitoring, with the relevant units for agricultural damage subsidies, but also to establish the spectral information of different rice phenology to carry out the preliminary prediction of bacterial leaf blight for early detection. It was applied on separating paddy rice varieties in the breeding plots, more than 20 kinds of rice variety were identified. The hyperspectral images acquired by UAV have high potential for applications in the culture of paddy rice. Mapping areas of infected paddy rice provides the exact location of plant health problems in the crop. The precise location of this problem enables the use of pesticides to reach outbreaks of disease or pest, and makes for the most appropriate crop management, either with the application of pesticides or even the disposition of diseased plants.
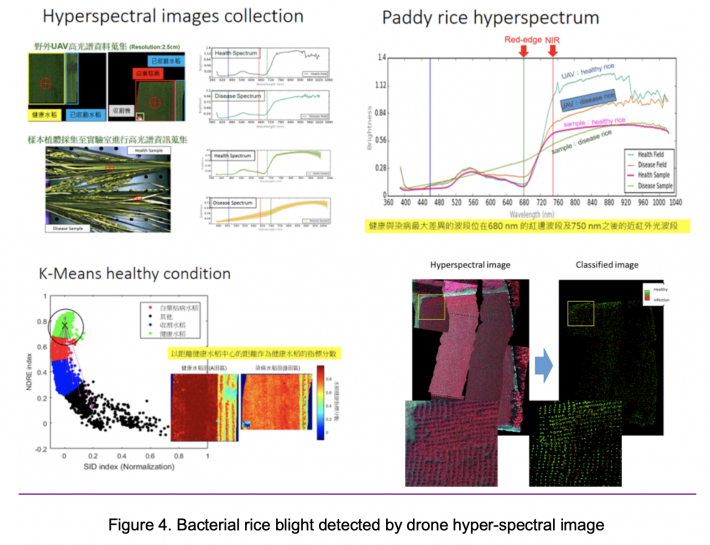
DISASTER DAMAGE ASSESSMENT
FAO has partnered with national counterparts in developing systems to use drones for data collection that assist in disaster risk reduction (DRR) efforts. The drones are equipped with photogrammetric and navigation equipment with a ground resolution of up to two centimeters. This can be programmed to detect details such as NDVI, water stress or lack of specific nutrients in crops. The drone-supporting mapping efforts are now being mainstreamed under the FAO’s disaster risk reduction and management (DRRM) and climate change adaptation (CCA) strategies (Syvester,2018). Crop damage assessments and adjustments have traditionally been difficult to make and are therefore often inaccurate. Typically, after an insurable event such as a flood, infestation or storm, a measurement of damage for a particular crop is carried out manually. First an insurance loss adjuster must identify all areas in a field that are damaged. Finally, an assessment of the damage in that area is assigned to calculate economic impact.
Heavy rainfall in monsoon season and typhoons that invade Taiwan every year often cause serious crop damage. Identification of crop damage compensation processes was a hardship work. To Identify the crop loss is a time-consuming and also delay the rehabilitation time of farmer. Therefore, how to accelerate the process of identification of crop damage by local government officers was an important issue of serving for farmer. Rice lodging is a kind of agricultural disaster that effect rice yield and rice quality. Rice lodging is directly related to rice varieties, cultivation management, pest and strong wind. The TARI team provided the UAV aerial photos and interpretation of rice lodging disaster for the COA disaster policy and trial work, and revised the UAV survey flow chart for the work process for the Agricultural Food Agency (FAA) and the Local County and City Government. An SOP of bidding and detailed procedures was designed for the aerial photos survey and interpretations companies will analyze in the percentage of the paddy rice lodging per plot. 8 counties will be conducted this project on the second crop of this year. The UAV has obvious advantages in the monitoring of rice lodging, it has the characteristics of direct, prompt, accurate and efficient. Staff of the local governments can take active and effective measures to deal with rice lodging, so as to reduce the loss of production.
The influence of climate change on agricultural production and financial risks faced by agricultural producers or agricultural-related industries has been the center of the public discussion for years. Many countries including developed and developing ones have attempted to replace disaster assistance with traditional crop insurance programs in order to reduce costs and improve individual risk protection.
In Taiwan, agricultural producers have historically relied on government disaster assistance to manage the financial risk associated with catastrophic weather events such as typhoons, floods, and droughts. With the help of the Council of Agriculture, Executive Yuan, Fubon Insurance Company has launched the first pilot crop insurance contract in 2015. This contract provides pear producers with protection against production costs due to typhoon, excess rainfall as well as low temperature. Drone imagery is very useful in giving an accurate estimate of loss. Drones are also increasingly used in the agricultural insurance and assessment sector, including in insurance claims forensics (Wadke, 2017).
AGRICULTURAL MAPPING WITH DRONES
Precision Analytics—Agriculture is a web-based portal designed specifically for agriculture users. It automatically processes aerial imagery into 2D maps and 3D elevation models, features a continuously expanding library of on-demand analysis tools, and makes sharing data easy.
Some farmers, especially small-scale farmers have been skeptical about adopting the use of drones in crop data collection. Drone for precision farming can be equipped with a multi-spectrum camera and is used for precision farming by using special software. With this drone we can capture various data about plants, trees, farms, fences fields, traces of agricultural vehicles and other data for precision farming (Figure.5). The images that we capture can also be used to create e.g. NDVI (vegetation volume and condition) reporting. NDVI can show for example, how well the different parts of the land absorb water from irrigation, and where the different kinds of weeds is growing in the crops.
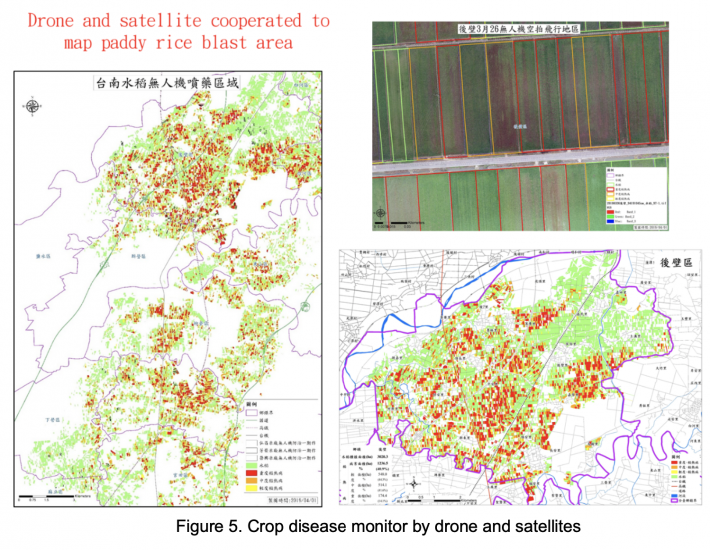
The key success factor of photographic-mapping is that these images are grouped geographically with special software in a recognizable overall picture of the field by imaging processing so that the various colors and reflections of light are converted into readable information such as NDVI or ENDVI reporting. The colorful images provide good view where the crop grows well, is too dry, et cetera.
UAV can be used to obtain crop canopy elevation data, and the Crop Surface Model (CSM) can be used to estimate the height, quality, leaf area index, planting index, chlorophyll index or nitrogen content of crop growth stages, which can save a lot of manpower. Field fertility surveys and more quantitative crop growth data were obtained with higher regional representation. This technology relies on the development or acquisition of sensors and image processing techniques.
At the same time height images, 3D images and an ortho-mosaic image are processed and reported. The 3D model can then manually move into other projections. During a few minutes flight about 20 images (half of ha.) are made up to 250 images (90 ha) during a flight of 30-45 minutes. Then we process the images with special software which will be included an operational instruction for the farmers.
CONCLUSION
Precision Agriculture (PA) management systems are considered among the top ten revolutions in the agriculture industry during the last couple decades. Generally, the PA is a management system that aims to integrate different technologies as navigation and imagery systems to control the use of the agriculture industry inputs aiming to enhance the quality and quantity of its output. One of the important PA applications that uses drone imagery systems, and which drew lots of interest is the crop monitoring, especially that such application is important for other applications such as pest detection and crop yield predication. The of scientific data collection using drones facilitates precision farming in boosting crop production. With improvements in the features, the drones are expected to be affordable even to the small-scale farmer.
TARI team started to apply drone for rice damage monitoring since 2013. It developed crop monitoring and pesticides spraying tech. in 2014. Based on the drone application and development, it will be applied and developed continuously in several aspects, including: (1)to increase accuracy of farmland monitoring and management to implement small-scale agricultural precision farming; (2) to increase the accuracy of pesticides spray and fertilization application for rural labor shortage and environmental protection; (3) to improve regional management efficiency and reduce production costs by data collection; (4) to assist in field trials to investigate crop growth status collection systems; (5) crops damage survey and insurance compensation estimates; (6) cultivated acreage survey and agricultural stresses early warning; (7) application of production tools for facility agricultural automation, etc. in the near future.
REFERENCES
Bendig J, Bolten A, Bennertz S, Broscheit J, Eichfuss S, Bareth G. Estimating Biomass of Barley Using Crop Surface Models (CSMs) Derived from UAV-Based RGB Imaging. Remote Sens. 2014; 6: 10395– 10412.
Chang, C.I. Hyperspectral Data Exploitation: Theory and Applications; John Wiley & Sons: New York, NY, USA, 2007.
Díaz-Varela RA, de la Rosa R, León L, Zarco-Tejada PJ. High-Resolution Airborne UAV Imagery to Assess Olive Tree Crown Parameters Using 3D Photo Reconstruction: Application in Breeding Trials. Remote Sens. 2015; 7: 4213–4232.
He X K, Bonds J, Herbst A, Langenakens J. Recent development of unmanned aerial vehicle for plant protection in East Asia. Int J Agric & Biol Eng., 2017; 10(3): 18–30
He X K, Chen J H, Sun Y M, Cheng X G, Guo H M. Chemical application techniques and equipment for rice in Japan and Korea. Technology Innovation and Modern Plant Protection, 2012; 11 (ISSN 978-7-109-17356-9, Beijing): 95–99. (in Chinese)
He X K. The opportunity and challenge of pesticide application for agriculture and horticulture in China. International Advances in Pesticide Application, 2010, 99: 157–164.
Huang Y B, Hoffmann C, Fritz B, Lan Y B. Development of an unmanned aerial vehicle-based spray system for highly accurate site-specific application. ASABE Meeting Presentation. 2008. Paper number: 083909.
Sylvester G. E-AGRICULTURE IN ACTION: DRONES FOR AGRICULTURE. Published by Food and Agriculture Organization of the United Nations and International Telecommunication Union, Bangkok, 2018.
Torres-Sánchez J, López-Granados F, Serrano N, Arquero O, Peña JM High Throughput 3-D Monitoring of Agricultural-Tree Plantations with Unmanned Aerial Vehicle (UAV) Technology. PLoS ONE 2015, 10(6): e0130479.
Zarco-Tejada PJ, Diaz-Varela R, Angileri V, Loudjani P. Tree height quantification using very high resolution imagery acquired from an unmanned aerial vehicle (UAV) and automatic 3D photo-reconstruction methods. Eur J Agron. 2014; 55: 89–99.
Zhaoxia Lou, Fang Xin, Xiaoqiang Han, Yubin Lan, Tianzhu Duan and Wei Fu. Effect of Unmanned Aerial Vehicle Flight Height on Droplet Distribution, Drift and Control of Cotton Aphids and Spider Mites. Agronomy 2018, 8, 187; 13 Sep 2018,MDPI.
Date submitted: October 29, 2019
Reviewed, edited and uploaded: December 2, 2019
|
Drones Application in Farming Management in Taiwan
ABSTRACT
Drone application in Taiwan is becoming popular practices. Several kinds of drone’s application in agriculture, forestry, fishery and mountainous rural security management, such as: land management, land cover monitoring, crop and fishery damage assessment of natural hazards, rural 3D landscape and pesticides spray and assessment have conducted by Council of Agriculture.The major task of this paper is reported that some development status of low-altitude and low-volume drones’ technology for plant protection in Taiwan. At moment, the strategies of UAV application in agriculture in Taiwan are focus on establishing the characteristics of spray systems that is suitable for different types of Drones and crop, and evaluating the safety of pesticide dosages. Applying drone with kinds of spectral sensors and location-based function to collect the information of pests and diseases invasive and development on crop, that is, has been identified spectral information of crop samples before symptoms were observed, and identifying the disease status in crops through characteristic bands, which will be become an effective early warning information for precision agriculture of crop pests and diseases prevention actions. The bacterial leaf blight of rice in the second crop of 2017 was collected by using a drone equipped with a 400 to 1000 nm hyperspectral camera, and applied the Spectral Information Divergence (SID) and Normalized Difference Vegetation Index (NDVI) with K-Means algorithm for data clustering and quantification. In the future, using UAS with insects and spore traps to capture pathogen spores in the air, combined with different algorithms for pest and disease early warning model construction, will make the prediction accuracy of pests and diseases more accurate and will make a progress on the pest and disease warning, combined with a variety of technologies such as: IoT and prediction models to improve the coverage and accuracy of agricultural disease prediction.
Keywords: Drone, pesticides spray assessment, crop damage assessment of natural hazards
INTRODUCTION
One of the latest developments is the increase in the use of small, unmanned aerial vehicles (UAVs), commonly known as drones, for agriculture (FAO, 2018). On the farm, yield measurements, forage quality evaluation, weed control effectiveness, and plant nutrient status are some of the popular data collection methods used to measure these risks. These methods rely on such gadgets as portable meters, test strips, manual stand counts, chlorophyll meters, seed sampling, and color charts for plant tissues. Farmers map these farms according to such historical records as soil analysis, crop rotation and pest infestation. Drones have inbuilt sensors, navigation systems and recording devices that single out trouble spots in large farms at a bird’s eye view. They can pick out anomalies that are difficult to see while on the ground. The data collected is of high resolution at an affordable cost. The UAV’s are capable of translating the raw data using complex formulas and present practical statistics to the farmer. The drone’s capabilities are not limited to just pinpointing problem areas. They are useful in monitoring ambitious growth plans undertaken by farmers. Such plans include data collection in a horticultural farm.
As a consequence of population aging and shrinkage in Asian countries, the trend of labor shortage for agricultural production has grown and will continue in future (He et al., 2017). Meantime, the new circumstances of modern agricultural construction are those making agriculture realize the goal of high yield, high quality, high efficiency, ecology and safety, realizing green consumption and sustainable development, and building resource-saving and environmental-friendly agriculture. Therefore, in order to adapt these new circumstances, agricultural UAV is developed quickly in Asia, providing powerful support for promoting agricultural production security, quality and safety of agricultural products, agricultural ecological security and agricultural trade security (He et al., 2010, 2012).
The following are some of the ways in with UAVs are used in crop data collection: The extent of pest and fungal infestation; maps relating to plant anomalies, leaf coverage, flowering, the impact of insecticide application; plant growth surveillance – monitor the weather conditions; Irrigation – Drones are single out fields where water levels need to be increased or reduced. Upon the crop growth, the drones collect data relating to plant density, health and heat emission. Data collection is a crucial aspect of farm management and growth. In the past, farmers have employed weak data collection tools that led to poor decisions and huge losses. Even for large scale growers who engaged experts in the field in collecting accurate data, such data collection would take months to collect. By the time the data was ready for use, it had become irrelevant. Since the early 80’s when the first drones were put into commercial use, there has been and increasing number of farmers using the drones in monitoring their crop production. Now, the UAV has become a data collection tool of choice in the agribusiness sector. In the ensuing discussion, we shall analyze the effectiveness of the use of the unmanned aerial vehicle to collect crop data. The unmanned aerial vehicles use in crop data collection is therefore expected to increase. This increase will be at a much slower pace because of the tough economic conditions prevailing in the agribusiness industry. Mechanization of farms makes the drones an economical option. They now have advance data gathering features and software packages that are updated regularly.
A number of successful studies have been conducted using small, lightweight cameras on board drone and the research area is given a lot of attention worldwide (Nebiker et al. 2008; Bergo et al. 2010; Laliberte al. 2011; Zarco Tejada et al. 2012). For remote sensing applications in precision agriculture (PA), drones are very useful autonomous vehicles. Drone’s applications for localizing weeds and diseases, determining soil properties, detecting vegetation differences and the production of an accurate elevation models are currently possible. In current PA applications, there are some image processing methods that are used to determine the soil and plant characteristics. For this, the drone technology is a powerful method to meet the required resolution levels. Drones have recently become the subject of a number of studies that evaluate their applicability for PA applications.
Application of crop production and protection materials is a crucial component in the high productivity of agriculture. Drones will enable farmers to know more about their fields. UAV spray pesticides has been developed and applied in the fields more than 30 years. In recently years, multi-rotor drone crop protection operations have experienced tremendous growth. Compared with manual operations, UAV has advantages such as high operational efficiency, small pesticides dosages, and low pesticides hazards to human (He et al., 2017). Therefore, farmers will be assisted with producing more food while using fewer chemicals. Agricultural chemical application tried to achieve the aims of spraying pesticides at specific times and locations for accurate site‐specific management of crop pests. The developed spray system has the potential to provide accurate, site‐specific crop management when coupled with UAV systems recently. It also has great potential for vector control in the areas that are not easily accessible by personnel or equipment (Huang, et al. 2009).
UAS are getting increasingly popular in agricultural and forestry insofar as they represent a cost-effective and readily available tool for surveying land and crops, with the purpose of acquiring data for further analysis and to support decision-making and management processes (Telmo Adão et al., 2017). UAVs imagery systems are capable to fill the gap between aerial and terrestrial imagery systems and enhance the use of imagery systems and remote sensing for PA applications. UAVs provide real time and high-quality aerial imagery compared to satellite imagery over agricultural areas. These have a huge potential in agriculture in supporting evidence-based planning and in spatial data collection. Despite some inherent limitations, these tools and technologies can provide valuable data that can then be used to influence policies and decisions. The advantages that “an eye in the sky” provides when combined with analytic tools that can interpret the data and images to actionable information have ushered in a new revolution. However, priority in addressing issues related to privacy, safety and security is the key to the sustainable implementation of these technologies (FAO, 2018).
Hyperspectral Imaging (HSI) is an emerging imaging that contains hundreds of contiguous narrow spectral bands covering a wide range of the electromagnetic spectrum from the visible to the infrared domain (Chang, 2007). Hyperspectral sensors usually generate huge amounts of data since they retrieve spectra sets composed of hundreds of bands across considerable spatial resolution images (Telmo Adão et al., 2017). Such dimensionality demands proper analysis and processing methods. Hyperspectral data processing must be performed towards the retrieval of relevant information, which provides the true benefits for assertive interventions in agricultural crops areas. Hyperspectral-based remote sensing supported by UAV platforms, a survey including hyperspectral sensors, inherent data processing and applications focusing both on agriculture—wherein the combination of UAV and hyperspectral sensors plays a center role (Telmo Adão et al., 2017).
The UAV can automatically flight at low altitudes and with large overlaps, which permit the acquisition of ultra-high spatial resolution images and the generation of the Digital Surface Model (DSM) using automatic photo-reconstruction methods that are based on the “Structure from Motion” approach for 3-D reconstruction. This work presents an innovative procedure for computing the 3-dimensional geometric features of individual trees and tree-rows by applying image analysis techniques. The geometric features of fruit trees such as canopy area, fruit tree height and crown volume provide useful information about plantation status and fruit crop production. The maps generated could be used to understand the linkages between fruit tree grown and field-related factors or to optimize crop management operations in the precision agriculture (Bendig et al., 2013; Díaz-Varela et al., 2015; Torres-Sánchez et al., 2015; Zarco-Tejada et al., 2014).
STATUS OF DRONE APPLICATION IN THE AGRICULTURAL SECTOR OF TAIWAN
Drone’s application in agriculture, forestry, fishery and mountainous rural security management, such as: land management, land cover monitoring, crop and fishery damage assessment of natural hazards, rural 3D landscape and pesticides spray and assessment have conducted by Council of Agriculture. Soil and Water Conservation Bureau of COA utilizes drones with geospatial imagery technology, such as: DSM (Digital Surface Model) and ortho-photograph of AOI (area of interesting) to enhance disaster preparedness and the response activities to the ministry. The activities also generate useful information related to upland agricultural risks such as landslides and erosion that could be used to inform agricultural communities and help them understand the risks and reduce the impacts of any disaster. The Forestry Bureau of COA uses drones to capture images for forest management of cases identification (to prevent illegal logging of trees), driftwood, and monitoring forest fires of barrier lakes after typhoons or earth quakes. The Fisheries Agency of COA developed the technologies for fishing vessels to apply drones to survey fishing groups, assist in salvage operations and conduct aquaculture surveys and management after natural disasters.
Drone also has been applied in Taiwan agriculture that is extending in crop monitoring, pest early warning systems, natural disaster damage assessments, are presented as the followings.
PESTICIDES SPRAY
In recent years, due to the aging of the rural population in Taiwan, the agricultural manpower has been in short supply, and the agricultural productivity has been seriously affected. Application of agricultural spraying and foliar fertilizing drones to save a lot of labor, time saving, water saving, medicine saving, safe use of pesticides, production of safe agricultural products, and promotion of precision farming management of small farmers is the focus of application research of drones aims. Drones, as emerging plant protection machinery, have the advantages of high operational efficiency, high speed, and low drift.
Public media reported that Taiwan have about 1000 young farmers own spray drones and served for other farmers in the field. And another 1000 young farmers will join in this service in the near future. Some professional UAV operation regulation has been announced, the agricultural drone spray pesticides regulation also is under construction. However, the national standard operating procedures for drone spray pesticides are lacking in the field. It is urgently necessary to build and standardize the operational technical specifications for UAV safely spray pesticides and keep the food products safety.
The spraying of agricultural chemicals by using drone speeds up the process and prevents compacting of the soil. However, adverse weather conditions (e.g. the speed and direction of the wind) can impair the effectiveness of the spraying of pesticides in a target crop field. There is a risk that the pesticide can drift to neighboring crop fields. It is believed that a large amount of all the pesticide used in the world drifts outside of the target crop field and only a small amount is effective in controlling pests. The current study aimed to elucidate the characteristics of droplet distribution and drift, control efficiency on crop insects, and attachment and absorption of crop leaves during drone spraying. Field tests have been conducted to evaluate spread factors of pesticides spray formulations on Kromekote cards. The drone spraying test aimed to study the droplet deposition and drifting regularity of the crops by drone spraying. Before spraying, a handheld GPS locator was used to determine the coordinates of each spraying zone.
There are four factors of drone spraying pesticides that affect the adhesion of pesticide on targets (Figure 1). Since the nozzle direction and nozzle pressure are not adjustable, the variation of drone spray pesticides effectiveness is based on drone flight height and flight speed. In order to obtain the effective spraying rate, the maximum flying speed when spraying (requirement of the adhesion specification) and the spray swath of the flight line must be obtained, wherein the swath of the next flight line needs to be evaluated the effectiveness of adhesive droplets on targets according to the spray droplets distribution curve (Figure 2). Kromekote card and filter paper are used as samplers to collect droplets, and the droplet density, coverage rate, deposition, and drift percentage are analyzed. The pooled results showed that the droplet uniformity, the droplet coverage rate, the deposition, and the drifting ability were higher when the drone flight height was 2 m (Lou et al., 2018).
More than 12 kinds of crops that compared with drone spray and manual operation pesticides to control pest experiments were conducted. The drone spray pesticide dose level ranges from 1X to 1/8X. According to the whole season production period of winter season head lettuce, the number of monitored pests of different pests was statistically analyzed as follows: Aphids, there was no difference of control effect between manual and drone spray, and drone spray used 1/8 dose level of pesticide had a similar effectiveness of manual spray pesticide. Whitefly, there is no difference of control effect between manual and drone spray, but the number of whiteflies was no difference between different dose levels. Hummer, there is a difference of control effect between manual and drone spray, and there is no difference between different dose level. On maize experiments (Figure.3), drone spray used 1/3 dose of pesticide has the similar control effect of manual spray. Drone spray used 1/16 dose of pesticide has the similar control effect of manual spray on dragon fruit experiment. But drone spray control effect was failed when it applied on loofah due to the crop leaves structure and cultivate type. Not every crop is suitable for UAV spraying, crop shapes should be carefully selected. The erect type plant and cultivation mode do not hinder the penetration of the drug solution. In addition to the routine control of UAV application, the key hazard sites should grasp the prevention time and adopt manual spraying. The wet rot pathogen of the dragon fruit flower will remain in the fruit. If it is not properly controlled after the rain, the humidity will increase in the carton after harvesting, and it will cause corruption and cause the base rot of the inferior red dragon fruit base. Applying with UAV It is difficult to spray, and manual spray can ensure the control effect. The team evaluated the drone spraying operations on several major crops application. The best benefit is the drone spraying on the dragon fruit orchard as an example, under the current pesticide use regulations, it saves 80% of pesticides and water consumption compared with traditional spraying, and saves 70% of the time.
The team of Taiwan Agricultural Research Institute carried out various drone spraying tests. The drone spraying pesticides was evaluated and it could be used to replace manual spray pesticides. The benefits of drone spray pesticides can save labor and time, less hurt human health, less pesticides consumption, less pesticides residual in crop, and achieving food safety goals. The team is committed to the establishment of the Standard Operating Procedure (SOP) of drone spray pesticides for various crops without changing the current pesticide management regulations and the concentration of pesticides use, and provides farmers with the specific operational references. The growth characteristics of crops affect the effect of drone spraying. The current equipment and technology still have space for improvement. The team is currently assisting the drone spray testing and verification of agricultural enterprises such as sweet corn and export lettuce, and they are sure to be recognized by the industry and actively. A large area of rice pest control by drone spray pesticides demonstration project was carried out and cooperated with Tainan City government with GIS and logistics for drone batteries and pesticides supply.
Further research will be aimed on the tiny droplets produced during drone spraying for crop protection also are affected by the rotors air flow and will drift in all directions in an uncontrollable manner, severely affecting the pesticide deposition pattern and resulting in pesticide waste. To improve pesticide use efficiency during multi-rotor drone spraying, an electrostatic spray system should be designed based on electrostatic spray technology and a six-rotor drone.
CROP PRODUCTION MONITORING
The next agricultural revolution will be driven by data, which will help to increase agricultural productivity with minimum damage to the environment and increased livelihoods for agricultural communities. Drone can be used to determine the impact of pest infestation on the crops of in a farm. It was flown over a wide acreage farm to take aerial pictures of the field. These pictures are either streamlined to store in a microchip for further analysis. The analysis results in a crop stress map with graphics showing the impact of the pest invasion. These captured images and transferred data into information, drones allow for permanent monitoring of a crop from planting to harvest. They can also help farmers react faster to threats, such as weeds, pests and stresses; save time crop scouting to take appropriate actions; and improve the application of variable input rates in real time.
The use of drone for agriculture is a valuable source of information on the agriculture practices. Drones have the power that comes from the strength of data processing and analytics that take place after the data is collected. The use of small drones for agriculture as well as access to platforms that can aggregate data from various sources to provide valuable insights would be greatly beneficial to farming managements. Drones are used for aerial survey, high-resolution aerial photos, mapping and land surveying, and handles data that are used to track crop growth stages, weeds, compaction, storm damage and more. Commercial software packages offer an integrated platform to use data from drones, sensors and other devices to automate and optimize farm management. The software converts multispectral images into accurate reflectance and index maps, such as NDVI, and uses red, green and blue (RGB) images to generate high-resolution ortho-mosaics.
Multispectral and hyper-spectral aerial and satellite imagery helps in creating Normalized Difference Vegetation Index (NDVI) maps, which can differentiate soil from grass or forest, detect plants under stress, and differentiate between crops and crop stages. There are strong correlations between crop yield and NDVI data measured at certain crop stages (Huang, Wang, Li, Tian and Pan, 2013). Drones fitted with infrared, multispectral and hyperspectral sensors can analyze crop health and soil conditions precisely and accurately. NDVI data, in combination with other indexes such as the Crop-Water Stress Index (CWSI) and the Canopy-Chlorophyll Content Index (CCCI) in agricultural mapping tools can provide valuable insights into crop health. Precision farming combines sensor data and imaging with real-time data analytics to improve farm productivity through mapping spatial variability in the field. Data collected through drone provide lots of raw data to activate analytical models for agriculture. In supporting precision farming, drones can do soil scans, monitor crop health, assist in planning irrigation schedules, apply fertilizers, estimate yield data and provide valuable data for weather analysis. Data collected through drones combined with other data sources and analytic solutions provide actionable information. Most of the concepts were become true and applied in the field. Precision agriculture becomes available in Asian small holder farming.
In Taiwan, there are 75 diseases that occur during rice cultivation. At present, the main diseases of rice in first crop is rice blast and in second crop is bacterial leaf blight. It is serious economic losses for cutting yield as much as 10-30%. In order to effectively monitor the occurrence of rice diseases, the current monitoring mechanism for rice diseases in Taiwan relies on field investigations conducted by professionals to collect the damage status of rice. TARI team conducted several projects of crops monitoring by drone. UAV equipped with high resolution optical camera, hyperspectral camera, and thermal camera for capturing imagery. The total aerial photography of the drone has been captured more than 40000 hectares (including vegetable production monitoring), with 150 flights each year. These imageries were served as ground truth of sampling sites of land cover databases for satellite image analysis. The process is time-consuming and ineffective to monitor rice production. Due to the development of remote sensing technology in recent years, resource satellite images, aerial images and the unmanned aerial vehicle (UAV) images are a fast and comprehensive way to obtain information. However, there can be applied in different fields by the different sensor.
Hyperspectral camera can perform imaging through the spectrum of hundreds of bands and find the corresponding bands of various targets through hyperspectral analysis. For example, in the second crop of 2017, the bacterial leaf blight was taken as an example. The UAV was equipped with a 400-1000 nm hyperspectral camera to collect information on the damage of bacterial leaf blight, and the Spectral Information Divergence (SID) was applied (Figure 4). Normalized Difference Vegetation Index (NDVI) and K-Means algorithm are used for data grouping and quantification to achieve automated rice bacterial blight damage area. In the future, Technology to conduct a wide range of disease area monitoring, with the relevant units for agricultural damage subsidies, but also to establish the spectral information of different rice phenology to carry out the preliminary prediction of bacterial leaf blight for early detection. It was applied on separating paddy rice varieties in the breeding plots, more than 20 kinds of rice variety were identified. The hyperspectral images acquired by UAV have high potential for applications in the culture of paddy rice. Mapping areas of infected paddy rice provides the exact location of plant health problems in the crop. The precise location of this problem enables the use of pesticides to reach outbreaks of disease or pest, and makes for the most appropriate crop management, either with the application of pesticides or even the disposition of diseased plants.
DISASTER DAMAGE ASSESSMENT
FAO has partnered with national counterparts in developing systems to use drones for data collection that assist in disaster risk reduction (DRR) efforts. The drones are equipped with photogrammetric and navigation equipment with a ground resolution of up to two centimeters. This can be programmed to detect details such as NDVI, water stress or lack of specific nutrients in crops. The drone-supporting mapping efforts are now being mainstreamed under the FAO’s disaster risk reduction and management (DRRM) and climate change adaptation (CCA) strategies (Syvester,2018). Crop damage assessments and adjustments have traditionally been difficult to make and are therefore often inaccurate. Typically, after an insurable event such as a flood, infestation or storm, a measurement of damage for a particular crop is carried out manually. First an insurance loss adjuster must identify all areas in a field that are damaged. Finally, an assessment of the damage in that area is assigned to calculate economic impact.
Heavy rainfall in monsoon season and typhoons that invade Taiwan every year often cause serious crop damage. Identification of crop damage compensation processes was a hardship work. To Identify the crop loss is a time-consuming and also delay the rehabilitation time of farmer. Therefore, how to accelerate the process of identification of crop damage by local government officers was an important issue of serving for farmer. Rice lodging is a kind of agricultural disaster that effect rice yield and rice quality. Rice lodging is directly related to rice varieties, cultivation management, pest and strong wind. The TARI team provided the UAV aerial photos and interpretation of rice lodging disaster for the COA disaster policy and trial work, and revised the UAV survey flow chart for the work process for the Agricultural Food Agency (FAA) and the Local County and City Government. An SOP of bidding and detailed procedures was designed for the aerial photos survey and interpretations companies will analyze in the percentage of the paddy rice lodging per plot. 8 counties will be conducted this project on the second crop of this year. The UAV has obvious advantages in the monitoring of rice lodging, it has the characteristics of direct, prompt, accurate and efficient. Staff of the local governments can take active and effective measures to deal with rice lodging, so as to reduce the loss of production.
The influence of climate change on agricultural production and financial risks faced by agricultural producers or agricultural-related industries has been the center of the public discussion for years. Many countries including developed and developing ones have attempted to replace disaster assistance with traditional crop insurance programs in order to reduce costs and improve individual risk protection.
In Taiwan, agricultural producers have historically relied on government disaster assistance to manage the financial risk associated with catastrophic weather events such as typhoons, floods, and droughts. With the help of the Council of Agriculture, Executive Yuan, Fubon Insurance Company has launched the first pilot crop insurance contract in 2015. This contract provides pear producers with protection against production costs due to typhoon, excess rainfall as well as low temperature. Drone imagery is very useful in giving an accurate estimate of loss. Drones are also increasingly used in the agricultural insurance and assessment sector, including in insurance claims forensics (Wadke, 2017).
AGRICULTURAL MAPPING WITH DRONES
Precision Analytics—Agriculture is a web-based portal designed specifically for agriculture users. It automatically processes aerial imagery into 2D maps and 3D elevation models, features a continuously expanding library of on-demand analysis tools, and makes sharing data easy.
Some farmers, especially small-scale farmers have been skeptical about adopting the use of drones in crop data collection. Drone for precision farming can be equipped with a multi-spectrum camera and is used for precision farming by using special software. With this drone we can capture various data about plants, trees, farms, fences fields, traces of agricultural vehicles and other data for precision farming (Figure.5). The images that we capture can also be used to create e.g. NDVI (vegetation volume and condition) reporting. NDVI can show for example, how well the different parts of the land absorb water from irrigation, and where the different kinds of weeds is growing in the crops.
The key success factor of photographic-mapping is that these images are grouped geographically with special software in a recognizable overall picture of the field by imaging processing so that the various colors and reflections of light are converted into readable information such as NDVI or ENDVI reporting. The colorful images provide good view where the crop grows well, is too dry, et cetera.
UAV can be used to obtain crop canopy elevation data, and the Crop Surface Model (CSM) can be used to estimate the height, quality, leaf area index, planting index, chlorophyll index or nitrogen content of crop growth stages, which can save a lot of manpower. Field fertility surveys and more quantitative crop growth data were obtained with higher regional representation. This technology relies on the development or acquisition of sensors and image processing techniques.
At the same time height images, 3D images and an ortho-mosaic image are processed and reported. The 3D model can then manually move into other projections. During a few minutes flight about 20 images (half of ha.) are made up to 250 images (90 ha) during a flight of 30-45 minutes. Then we process the images with special software which will be included an operational instruction for the farmers.
CONCLUSION
Precision Agriculture (PA) management systems are considered among the top ten revolutions in the agriculture industry during the last couple decades. Generally, the PA is a management system that aims to integrate different technologies as navigation and imagery systems to control the use of the agriculture industry inputs aiming to enhance the quality and quantity of its output. One of the important PA applications that uses drone imagery systems, and which drew lots of interest is the crop monitoring, especially that such application is important for other applications such as pest detection and crop yield predication. The of scientific data collection using drones facilitates precision farming in boosting crop production. With improvements in the features, the drones are expected to be affordable even to the small-scale farmer.
TARI team started to apply drone for rice damage monitoring since 2013. It developed crop monitoring and pesticides spraying tech. in 2014. Based on the drone application and development, it will be applied and developed continuously in several aspects, including: (1)to increase accuracy of farmland monitoring and management to implement small-scale agricultural precision farming; (2) to increase the accuracy of pesticides spray and fertilization application for rural labor shortage and environmental protection; (3) to improve regional management efficiency and reduce production costs by data collection; (4) to assist in field trials to investigate crop growth status collection systems; (5) crops damage survey and insurance compensation estimates; (6) cultivated acreage survey and agricultural stresses early warning; (7) application of production tools for facility agricultural automation, etc. in the near future.
REFERENCES
Bendig J, Bolten A, Bennertz S, Broscheit J, Eichfuss S, Bareth G. Estimating Biomass of Barley Using Crop Surface Models (CSMs) Derived from UAV-Based RGB Imaging. Remote Sens. 2014; 6: 10395– 10412.
Chang, C.I. Hyperspectral Data Exploitation: Theory and Applications; John Wiley & Sons: New York, NY, USA, 2007.
Díaz-Varela RA, de la Rosa R, León L, Zarco-Tejada PJ. High-Resolution Airborne UAV Imagery to Assess Olive Tree Crown Parameters Using 3D Photo Reconstruction: Application in Breeding Trials. Remote Sens. 2015; 7: 4213–4232.
He X K, Bonds J, Herbst A, Langenakens J. Recent development of unmanned aerial vehicle for plant protection in East Asia. Int J Agric & Biol Eng., 2017; 10(3): 18–30
He X K, Chen J H, Sun Y M, Cheng X G, Guo H M. Chemical application techniques and equipment for rice in Japan and Korea. Technology Innovation and Modern Plant Protection, 2012; 11 (ISSN 978-7-109-17356-9, Beijing): 95–99. (in Chinese)
He X K. The opportunity and challenge of pesticide application for agriculture and horticulture in China. International Advances in Pesticide Application, 2010, 99: 157–164.
Huang Y B, Hoffmann C, Fritz B, Lan Y B. Development of an unmanned aerial vehicle-based spray system for highly accurate site-specific application. ASABE Meeting Presentation. 2008. Paper number: 083909.
Sylvester G. E-AGRICULTURE IN ACTION: DRONES FOR AGRICULTURE. Published by Food and Agriculture Organization of the United Nations and International Telecommunication Union, Bangkok, 2018.
Torres-Sánchez J, López-Granados F, Serrano N, Arquero O, Peña JM High Throughput 3-D Monitoring of Agricultural-Tree Plantations with Unmanned Aerial Vehicle (UAV) Technology. PLoS ONE 2015, 10(6): e0130479.
Zarco-Tejada PJ, Diaz-Varela R, Angileri V, Loudjani P. Tree height quantification using very high resolution imagery acquired from an unmanned aerial vehicle (UAV) and automatic 3D photo-reconstruction methods. Eur J Agron. 2014; 55: 89–99.
Zhaoxia Lou, Fang Xin, Xiaoqiang Han, Yubin Lan, Tianzhu Duan and Wei Fu. Effect of Unmanned Aerial Vehicle Flight Height on Droplet Distribution, Drift and Control of Cotton Aphids and Spider Mites. Agronomy 2018, 8, 187; 13 Sep 2018,MDPI.
Date submitted: October 29, 2019
Reviewed, edited and uploaded: December 2, 2019