ABSTRACT
The bigdata is produced by various industry fields. Especially, the agriculture bigdata is collected from IoT (Internet of Things) technology. In this study, we researched the Smart Farm Big Data management and utilization. The smartfarm bigdata in Korea refers to real-time environment data (temperature, relative humidity and solar radiation, etc.) from control system which applies ICT technology to facilities such as a greenhouse or livestock farm, and growth data, yield, and cultivation information of the farm. In smart farm research, the simple smart farm technology was developed from 2014. It seeks farmer's agricultural working convenience in managing greenhouses through remote control and monitoring. Currently, to enhance productivity of farm households, the research and development of utilization big data technology is actively carried out. In Rural Development Administration, we collect and manage the horticultural smart farm bigdata, and analyze and develop the productivity improvement model since 2017. The major crop in Korea horticulture are tomato, strawberry, paprika and oriental melon, etc. The number of collecting farm bigdata is 280 including the 9 crop items. The bigdata collected from smart farm is environmental, irrigation, and yield. First, the collecting and managing system (Agriculture Bigdata Management System: ABMS) is constructed for utilization the data. Second, we studied the productivity improvement model which had the characteristics of the crop (tomato, strawberry) and farmer's cultivation and management difficulties. The model means the development of environment setting for proper growth and production in cultivation period. For example, it is necessary to harvest stable production tomato in a harvest period for tomato productivity improvement. In order to develop the model, environmental variables are selected by high correlation production and growth from association analysis in growth stage. And the conditions of the environment variables (temperature, solar radiation) were compared with the production. Not only smartfarm data management and modelling, but also it can be applied other precise agriculture fields, such as livestock, fruit, and crop.
Keywords: Bigdata, management, platform, horticulture
INTRODUCTION
The term “bigdata” designates the definition caused by internet of things (IoT) and information communication Technology (ICT). The bigdata is very interesting topic for researcher to analysis their phenomenon. The google took over the bigdata analysis industry recently. The bigdata analysis industry has technology that planning the company management, performance management, and predicting the market using bigdata. That means bigdata has big meaning for determining based on data and can be big background for new market, data cloud computing and platform service. For example, in agriculture and consumption of agrifood, in Rural Development Administration (RDA), it has collected the bigdata for consumption of agrifood from Korean consumer panel. They have collected the data for consuming the agrifood receipt from market from 2010. So, the data become bigdata for Korean agrifood consumption. The researcher for agrifood field can analyze the consumer characteristic for agrifood, and can make the decision the harvesting for farmer and establishing the strategy. The bigdata is the huge data set for out-of-hand data management and analysis system. First, Cox and Ellsworth talked the bigdata concept, and Gartner definited the bigdata characteristic from 2001. The bigdata has 3 characteristic, velocity, volume, and variety. That means the information technology, IT has high technology, so it can collect the data, sending the data in short time.
To understanding the concept of Korean “smartfarm”, we need to understand the gardening on greenhouse. In Korea, we build the various greenhouse and grow the kinds of crops in it. The Korean smartfarm is one of the areas of precision agriculture, not a new one. This agriculture system is using automated facilities, and information communication technology, monitoring and managing the environment in real time. And the smartfarm has complex environment control system. The reason why we call the samrtfarm is a smartphone or a tablet to monitor and control the facilities. The smartfarm in agriculuture is one of the national innovation projects in Korea from 2017. There is big smartfarm innovation complex by 2022 in main horticulture vegetable site, and education program is proceeded that young people has knowledge about the agriculturesmartfarm. This project purpose means having chance of young man’s job and development of the farm, rural areas from smartfarm. The Korean smart farm is widely divided in controlled horticulture, open field, fruit trees, and livestock, etc. The horticulture facility area is 4,000 ha in 2017, but the planning of extension to 7,000 ha in horticulture, and livestock 5750 farms.
In this smartfarm technology, Netherlands has the top research and technology of the precision farming. But it is very difficult to apply Netherlands technology to Korea because of climate condition, a king of crop, horticulture facility type, and area. Also, many farmers of horticulture in Korea, talked to us that the smartfarm is very convenient for farming (satisfaction score: 7.3 / 10), but it is much expensive to build smartfarm only for convenience. That means it is low satisfaction score for increasing productivity (6.0/10). and Farmers know the generated bigdata from smartfarm and control the environment using environment and growth data. Above of all, farmers insist that it is very important to increase crop productivity from information such as data, not convenience, and they have their income increasing. In Rural Development Administration, from the farmer’s thinking and Netherlands technology, we have researched the development of productivity improvement model from 2016.
Data collecting and management
First to develop the model, we need to collect the data. The data is generated by horticulture farms. The first data is environment data in a facility. The environmental data is collected by environment control system. The environment factors depend on equipment. The second is growth data. Because the crop growth condition is managed by environment condition. And third is yield, harvesting the fruit. These are very high relation for yield. We planned to collecting environment, growth, yield from smartfarm. And then we plan the collecting data of various crops in Korea. There are many crops from smartfarm but it is different to cultivate a kind of crop by site. We collected the tomato, strawberry, paprika, cucumber, flower, etc. in smartfarm. The number of farms collecting data is 280 farms from RDA. The number of smartfarm crop is table 1.
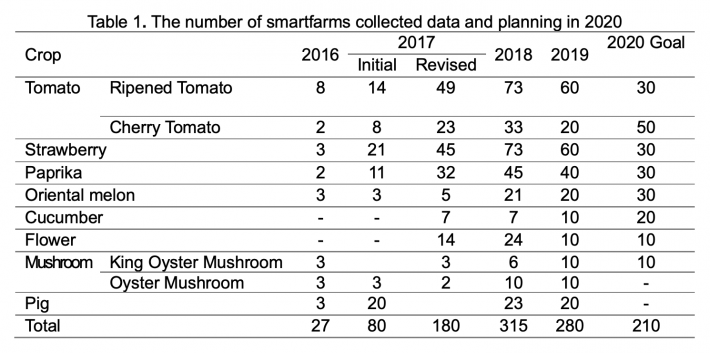
Agriculture Bigdata Management System
The collected bigdata is environmental, irrigation, and yield. First, the collecting and managing system (Agriculture Bigdata Management System: ABMS) is constructed for utilization the data in RDA. So, researchers can be connected the system (ABMS) and upload and download data easily. Also, the data can be transformed by analyzing the data. The ABMS structure is Figure 1. This system flow is summarized as follows. First the data from smartfarm is collected to ABMS. Second, the collected data are stacked the converged for analysis. Also, it can be analyzed the smartfarm data using statistical method and machine learning, etc. Finally, the information from analyzing the data is serviced to farmer that provide the data. The system can be used for researcher in RDA and province agriculture researcher.
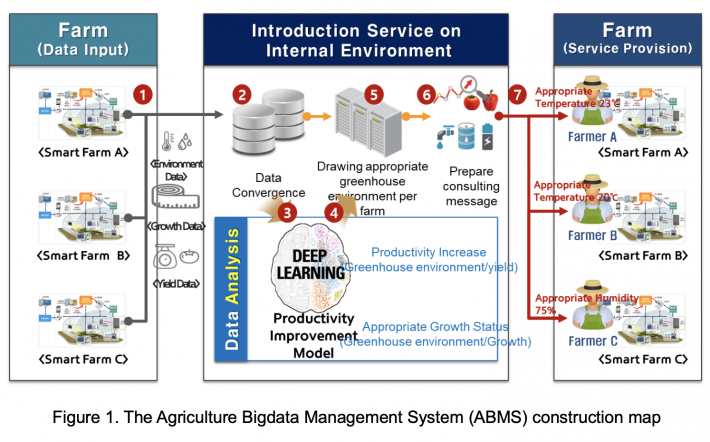
The collecting the smartfarm bigdata
There are 3 data type of smarttarm, environment, growth, yield data. First is environment data, depending on the installed sensor, the environment data, for example inside temperature, relative humidity, solar radiation, existed CO2, watering (supply EC, pH, the number of watering, amount of water per a plant). The data is measured, and also displayed in the database for real-time. The second and third are growth data and yield. This data is not collected automatically, we planned the researching program that training researcher about smartfarm. They can investigate the growth condition, for example growth length, number of leaves, flower condition, fruit condition, and yield. Also, they can consult the farms that they visited later.
The development of productivity improvement model
From the collected data, we designed the development of productivity improvement model, first of all, the first crop is tomato. The reason why is that tomato is most famous vegetable in a whole world. Also, it can be cultivated all sites in Korea. So, we selected the 41 farms data from Jeonbuk, Jeonnam, Kyoungnam site in multi-span greenhouse. Their cultivation has the planting in Summer (middle of August) to ending in next summer for 1 year. To use the data, we need to understand what data is collected and growth characteristics of crop, physiology of tomato. The tomato has 7~8 weeks’ growth period from flowering to harvest fruit. It means the tomato yield is affected by 7~8 weeks’ environment condition, not right now (Figure 2). Also, for productivity improvement for tomato fruit, it is different to control inside temperature from collected data such as Figure 3. This result means that it is very important to control to long period (7-8 weeks) and short period (1 day). So, we used the characteristic the tomato physiology and environment condition.
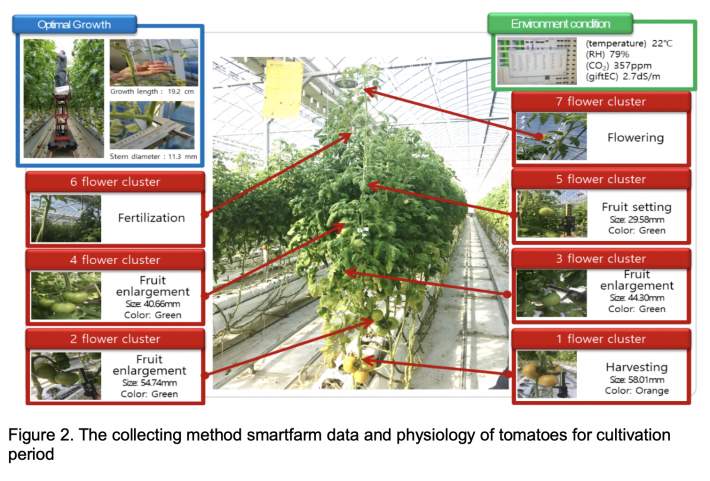
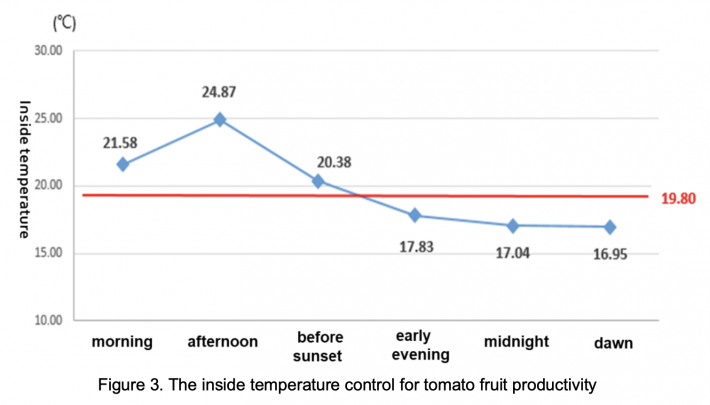
Monitoring method for smartfarm farmer
First, we developed the monitoring method the smartfarm data for farmer. The method is the farmers want that overall their farm consulting from their data. The guideline for smartfarm is based on crop growth characteristics. These methods are the decision tool for environment factor in growth stage and able to smartfarm management using various statistic tables and graph. Then we consulted the tomato farms using collected bigdata. The Figure 4 is 24 hours, daytime and night inside temperatures in smartfarm cultivation period. This farm was well managed by environment factor. The tomato growth temperature is 15~ 25 Celsius. So, for productivity improvement of tomato, this farm is considered another factor, watering, and growth. So, the method is published by book. And the farmer can be analyzed the own data easily.
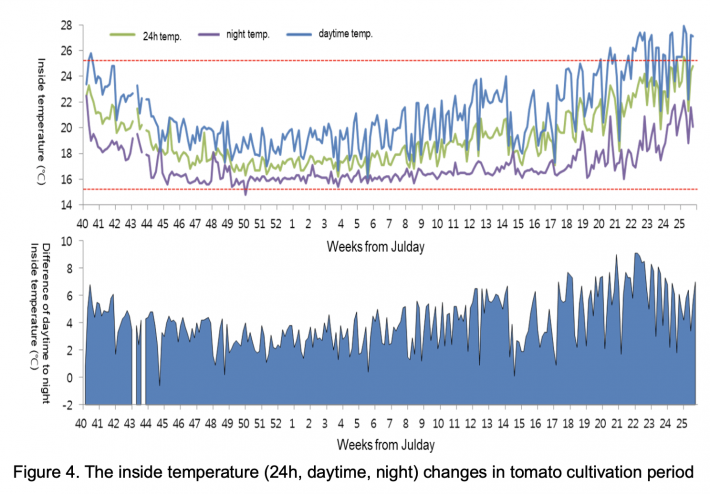
For these results, it is necessary to understand the principle of bigdata analysis. There is one point for analyzing the data is to reduce the time dimension of data. The environment data is generated by minute time unit form facility sensor. So, there are many data generated. But the growth data is collected by 1 week with replication from researcher. For analyzing the data, it is necessary to summarize the data by time.
Development the productivity improvement model
For developing the model, we analyzed the environment factor and growth condition and yield relation. We used the analysis method, multiple regression in statistics to find environment variable that affect the tomato yield. From this analysis the environment variables were transformed by 7 weeks of tomato crop growth. Therefore, inside temperature, solar radiation, amount of watering per plant, the number of watering per day, supply EC, supply pH and relative humidity were selected in model. And from the selected environment variable farm’s yield level. The daytime temperature has 18-29 Celsius, nighttime temperature 15~23 Celsius. It is similar to controlling temperature all smart farms (Figure 5). But the supply water is depending on season. Therefore, it is different go watering control to farm’s productivity level such as Figure 6.
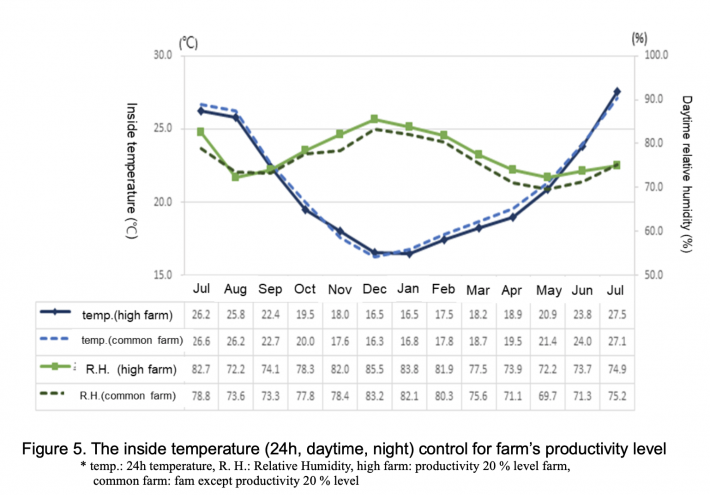
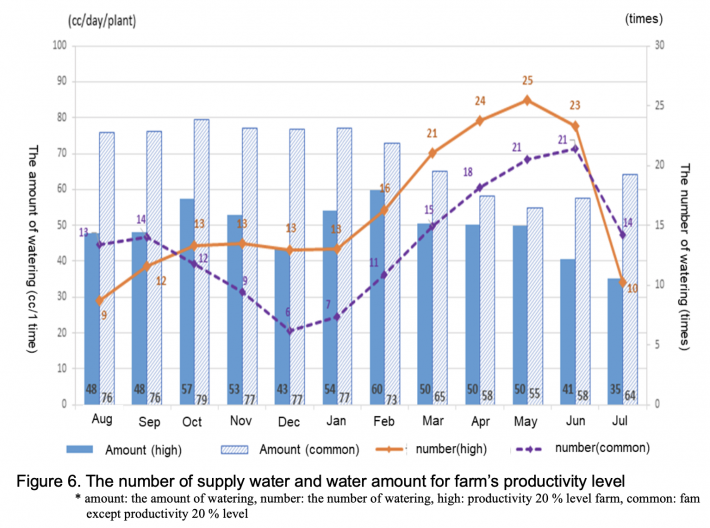
Furthermore, from growth and yield data, it is different to growth level for growth condition and season. It means the environment factor control in smartfarm is very important to improve tomato’s growth and yield. So, we developed the model that provides a way to control the environment setting value for farmer from collected environment, growth, and yield data. we developed the productivity improvement model. The model has the short environment condition setting for maintaining optimal growth stage and season. It is enable to increasing tomato yield, control growth condition by data. If anyone control the growth level using environment condition, it can be harvest y 150 kg/3.3 m2 for 1 year (Table 2). For example, in middle growth stage (winter in Korea: solar radiation 993~1146J/cm2), for growth length in tomato up, height of flower be downer, the inside temperature become control up, nighttime temperature become up such as table 3. So, the farmer can do control the environment condition comparison with crop growth.
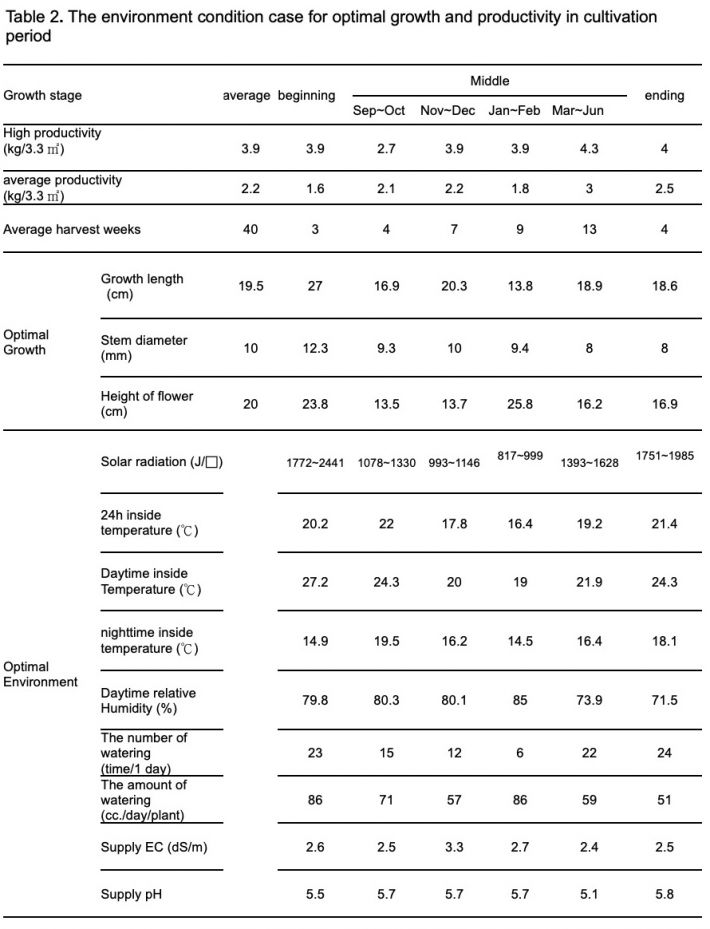
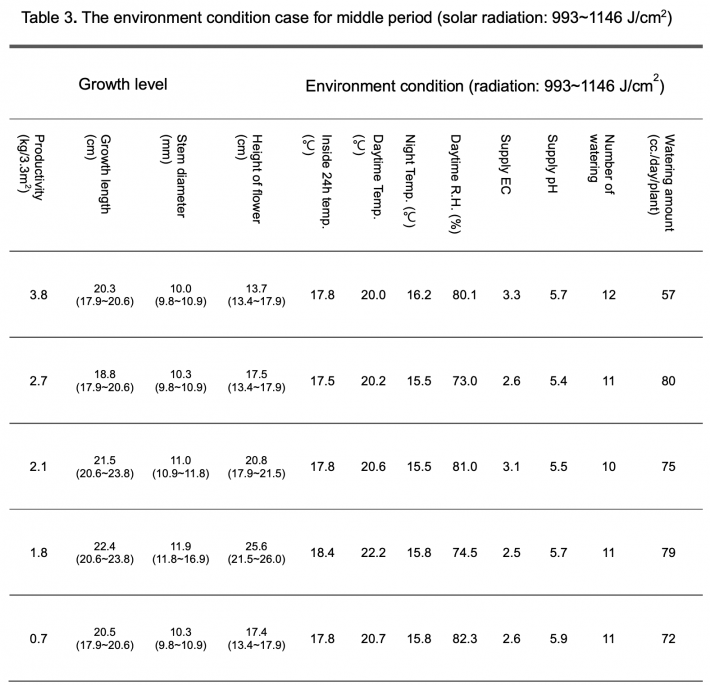
Future plan for smartfarm productivity improvement model
It is the future plan in smart farm bigdata research figure (Figure 7). For productivity improvement model and consulting from with data, we expand collection of farm data. And data will be also collected continuously by cropping season and data quality management will be maintained. Also, we develop the basic model for crop productivity improvement. And then we test the model for high accuracy model fitting from tomato smartfarm demonstration research. So, we will modify the productivity improvement model. Also, we will service the model for farmers from cloud service platform in RDA. Therefore, the model and data are used by smart farm industry, a farmer, and consumers.
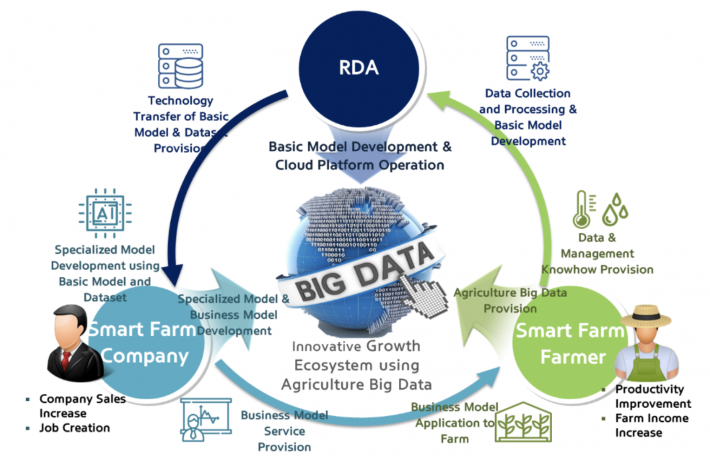
CONCLUSION
In this study, we introduced the bigdata definition and bigdata in agriculture. Especially there are various data, environment, growth and yield data from smartfarm data in Korea. We collected the environment data from sensor automatically, and growth and yield data by researcher. These data are stacked with the Agriculture Bigdata Management System in RDA, and is managed to analyze. We have developed the tomato productivity improvement model and consulting farmers from with data. For precision of model, we expand collection of farm data in various field, like horticulture, main vegetable. There are a small number of data because of smartfarm type, size of facility and species of crop. Data will be also collected continuously by cropping season and data quality management will be maintained. Also, we will develop and the basic model for crop productivity improvement. And then we will service the model for farmers from cloud service platform in RDA.
REFERENCES
Cho, I. H., J. K. Kwon, D. M. Oh, H. D. Lee and T. W. Jung, 2013. The guideline for agriculture technology: horticulture, Rural Development Administration, Jeonju, Korea.
Han J. and M. K., 2015. Data Mining: concepts and Techniques, Elsevier, Inc, NY, USA.
Lee, H. R. and J.E. Song. 2018. The study of sharing and utilization horticulture bigdata, E-business research winter symposium.
Lee, G. H., Y. G. Ham, Y. D. Kim, J. H. Lee, and J. H. Won, 2016. The understanding of bigdata, knou press, Seoul, Korea.
Lee, H. R., S. H. Park, S. J. Park and D. H. Kim, 2018. The research of farmer feedback for improvement of productivity using horticulture smartfarm bigdata, horticultural science and technology 36(2): 211.
Lee, H. R., M. O. Park and S. J. Park 2018. The study of environment and growth variation in the regional tomato greenhouse facility by abnormal weather, 19thconference on agricultural and forest methodology: 132.
Lee, H. R., M. O. Kim, Y. B. Cho, S. J. Park and J. H. Hwang, 2018. The economic model for enhancement upgrade of tomato growth data in horticulture smart farm, horticultural science and technology 36(1): 80.
Lee, H. R., J. H. Hwang, M. O. Kim, and Y. B. Cho, 2017. Development of economic model for enhancement of tomato farmer’s productivity using Smartfarm bigdata, horticultural science and technology 36(2): 100.
Lee, H. R., Y. B. Cho, J. H. Hwang, D. H. Kim, Y. S. Yu, D. W. Choi, S. R. Kim, Y. J. Ahn, I. K. Ham, M. H. Jeon, G. W. Park, T. W. Kang, M. H. Yoon and S. Y. Lee, 2017. The research of collecting the controlled horticulture smart farm bigdata, horticultural science and technology 35(2): 1021.
Lee, H. R., S. J. Park, S. H. Park, and D. H. Kim, 2018. The bigdata analysis method for smartfarm environment factors management (tomato, 2nd edition), Rural Development Administration, Jeonju, Korea.
Lee, M. S., J. K. Jang and D. H. Kim, 2017. The guideline for agriculture technology: tomato, Rural Development Administration, Jeonju, Korea.
Park, S. H., H. R. Lee, S. J. Park and Y. B. Cho, 2018. Facility horticulture smart farm environment integrated solar radiation quality management of bigdata, horticultural science and technology 36(2): 213.
Park, Y. H., W. H. Cho, M. H. Na, D. H. Kim, Y. B. Cho, and H. Y. Lee, 2018. Extraction of Environmental Factors Influencing Strawberry Yield in the facility farms using pattern recognition techniques, horticultural science and technology 36(1): 48-49.
Yeom, J. K., D. K. Kim and I. H. Jang, 2017. The linear regression analysis using SAS and R, Jayu Academy, Seoul, Korea.
Date submitted: October 29, 2019
Reviewed, edited and uploaded: November 28, 2019 |
The Bigdata Management and Use Case Study for Agriculture Based on Data
ABSTRACT
The bigdata is produced by various industry fields. Especially, the agriculture bigdata is collected from IoT (Internet of Things) technology. In this study, we researched the Smart Farm Big Data management and utilization. The smartfarm bigdata in Korea refers to real-time environment data (temperature, relative humidity and solar radiation, etc.) from control system which applies ICT technology to facilities such as a greenhouse or livestock farm, and growth data, yield, and cultivation information of the farm. In smart farm research, the simple smart farm technology was developed from 2014. It seeks farmer's agricultural working convenience in managing greenhouses through remote control and monitoring. Currently, to enhance productivity of farm households, the research and development of utilization big data technology is actively carried out. In Rural Development Administration, we collect and manage the horticultural smart farm bigdata, and analyze and develop the productivity improvement model since 2017. The major crop in Korea horticulture are tomato, strawberry, paprika and oriental melon, etc. The number of collecting farm bigdata is 280 including the 9 crop items. The bigdata collected from smart farm is environmental, irrigation, and yield. First, the collecting and managing system (Agriculture Bigdata Management System: ABMS) is constructed for utilization the data. Second, we studied the productivity improvement model which had the characteristics of the crop (tomato, strawberry) and farmer's cultivation and management difficulties. The model means the development of environment setting for proper growth and production in cultivation period. For example, it is necessary to harvest stable production tomato in a harvest period for tomato productivity improvement. In order to develop the model, environmental variables are selected by high correlation production and growth from association analysis in growth stage. And the conditions of the environment variables (temperature, solar radiation) were compared with the production. Not only smartfarm data management and modelling, but also it can be applied other precise agriculture fields, such as livestock, fruit, and crop.
Keywords: Bigdata, management, platform, horticulture
INTRODUCTION
The term “bigdata” designates the definition caused by internet of things (IoT) and information communication Technology (ICT). The bigdata is very interesting topic for researcher to analysis their phenomenon. The google took over the bigdata analysis industry recently. The bigdata analysis industry has technology that planning the company management, performance management, and predicting the market using bigdata. That means bigdata has big meaning for determining based on data and can be big background for new market, data cloud computing and platform service. For example, in agriculture and consumption of agrifood, in Rural Development Administration (RDA), it has collected the bigdata for consumption of agrifood from Korean consumer panel. They have collected the data for consuming the agrifood receipt from market from 2010. So, the data become bigdata for Korean agrifood consumption. The researcher for agrifood field can analyze the consumer characteristic for agrifood, and can make the decision the harvesting for farmer and establishing the strategy. The bigdata is the huge data set for out-of-hand data management and analysis system. First, Cox and Ellsworth talked the bigdata concept, and Gartner definited the bigdata characteristic from 2001. The bigdata has 3 characteristic, velocity, volume, and variety. That means the information technology, IT has high technology, so it can collect the data, sending the data in short time.
To understanding the concept of Korean “smartfarm”, we need to understand the gardening on greenhouse. In Korea, we build the various greenhouse and grow the kinds of crops in it. The Korean smartfarm is one of the areas of precision agriculture, not a new one. This agriculture system is using automated facilities, and information communication technology, monitoring and managing the environment in real time. And the smartfarm has complex environment control system. The reason why we call the samrtfarm is a smartphone or a tablet to monitor and control the facilities. The smartfarm in agriculuture is one of the national innovation projects in Korea from 2017. There is big smartfarm innovation complex by 2022 in main horticulture vegetable site, and education program is proceeded that young people has knowledge about the agriculturesmartfarm. This project purpose means having chance of young man’s job and development of the farm, rural areas from smartfarm. The Korean smart farm is widely divided in controlled horticulture, open field, fruit trees, and livestock, etc. The horticulture facility area is 4,000 ha in 2017, but the planning of extension to 7,000 ha in horticulture, and livestock 5750 farms.
In this smartfarm technology, Netherlands has the top research and technology of the precision farming. But it is very difficult to apply Netherlands technology to Korea because of climate condition, a king of crop, horticulture facility type, and area. Also, many farmers of horticulture in Korea, talked to us that the smartfarm is very convenient for farming (satisfaction score: 7.3 / 10), but it is much expensive to build smartfarm only for convenience. That means it is low satisfaction score for increasing productivity (6.0/10). and Farmers know the generated bigdata from smartfarm and control the environment using environment and growth data. Above of all, farmers insist that it is very important to increase crop productivity from information such as data, not convenience, and they have their income increasing. In Rural Development Administration, from the farmer’s thinking and Netherlands technology, we have researched the development of productivity improvement model from 2016.
Data collecting and management
First to develop the model, we need to collect the data. The data is generated by horticulture farms. The first data is environment data in a facility. The environmental data is collected by environment control system. The environment factors depend on equipment. The second is growth data. Because the crop growth condition is managed by environment condition. And third is yield, harvesting the fruit. These are very high relation for yield. We planned to collecting environment, growth, yield from smartfarm. And then we plan the collecting data of various crops in Korea. There are many crops from smartfarm but it is different to cultivate a kind of crop by site. We collected the tomato, strawberry, paprika, cucumber, flower, etc. in smartfarm. The number of farms collecting data is 280 farms from RDA. The number of smartfarm crop is table 1.
Agriculture Bigdata Management System
The collected bigdata is environmental, irrigation, and yield. First, the collecting and managing system (Agriculture Bigdata Management System: ABMS) is constructed for utilization the data in RDA. So, researchers can be connected the system (ABMS) and upload and download data easily. Also, the data can be transformed by analyzing the data. The ABMS structure is Figure 1. This system flow is summarized as follows. First the data from smartfarm is collected to ABMS. Second, the collected data are stacked the converged for analysis. Also, it can be analyzed the smartfarm data using statistical method and machine learning, etc. Finally, the information from analyzing the data is serviced to farmer that provide the data. The system can be used for researcher in RDA and province agriculture researcher.
The collecting the smartfarm bigdata
There are 3 data type of smarttarm, environment, growth, yield data. First is environment data, depending on the installed sensor, the environment data, for example inside temperature, relative humidity, solar radiation, existed CO2, watering (supply EC, pH, the number of watering, amount of water per a plant). The data is measured, and also displayed in the database for real-time. The second and third are growth data and yield. This data is not collected automatically, we planned the researching program that training researcher about smartfarm. They can investigate the growth condition, for example growth length, number of leaves, flower condition, fruit condition, and yield. Also, they can consult the farms that they visited later.
The development of productivity improvement model
From the collected data, we designed the development of productivity improvement model, first of all, the first crop is tomato. The reason why is that tomato is most famous vegetable in a whole world. Also, it can be cultivated all sites in Korea. So, we selected the 41 farms data from Jeonbuk, Jeonnam, Kyoungnam site in multi-span greenhouse. Their cultivation has the planting in Summer (middle of August) to ending in next summer for 1 year. To use the data, we need to understand what data is collected and growth characteristics of crop, physiology of tomato. The tomato has 7~8 weeks’ growth period from flowering to harvest fruit. It means the tomato yield is affected by 7~8 weeks’ environment condition, not right now (Figure 2). Also, for productivity improvement for tomato fruit, it is different to control inside temperature from collected data such as Figure 3. This result means that it is very important to control to long period (7-8 weeks) and short period (1 day). So, we used the characteristic the tomato physiology and environment condition.
Monitoring method for smartfarm farmer
First, we developed the monitoring method the smartfarm data for farmer. The method is the farmers want that overall their farm consulting from their data. The guideline for smartfarm is based on crop growth characteristics. These methods are the decision tool for environment factor in growth stage and able to smartfarm management using various statistic tables and graph. Then we consulted the tomato farms using collected bigdata. The Figure 4 is 24 hours, daytime and night inside temperatures in smartfarm cultivation period. This farm was well managed by environment factor. The tomato growth temperature is 15~ 25 Celsius. So, for productivity improvement of tomato, this farm is considered another factor, watering, and growth. So, the method is published by book. And the farmer can be analyzed the own data easily.
For these results, it is necessary to understand the principle of bigdata analysis. There is one point for analyzing the data is to reduce the time dimension of data. The environment data is generated by minute time unit form facility sensor. So, there are many data generated. But the growth data is collected by 1 week with replication from researcher. For analyzing the data, it is necessary to summarize the data by time.
Development the productivity improvement model
For developing the model, we analyzed the environment factor and growth condition and yield relation. We used the analysis method, multiple regression in statistics to find environment variable that affect the tomato yield. From this analysis the environment variables were transformed by 7 weeks of tomato crop growth. Therefore, inside temperature, solar radiation, amount of watering per plant, the number of watering per day, supply EC, supply pH and relative humidity were selected in model. And from the selected environment variable farm’s yield level. The daytime temperature has 18-29 Celsius, nighttime temperature 15~23 Celsius. It is similar to controlling temperature all smart farms (Figure 5). But the supply water is depending on season. Therefore, it is different go watering control to farm’s productivity level such as Figure 6.
Furthermore, from growth and yield data, it is different to growth level for growth condition and season. It means the environment factor control in smartfarm is very important to improve tomato’s growth and yield. So, we developed the model that provides a way to control the environment setting value for farmer from collected environment, growth, and yield data. we developed the productivity improvement model. The model has the short environment condition setting for maintaining optimal growth stage and season. It is enable to increasing tomato yield, control growth condition by data. If anyone control the growth level using environment condition, it can be harvest y 150 kg/3.3 m2 for 1 year (Table 2). For example, in middle growth stage (winter in Korea: solar radiation 993~1146J/cm2), for growth length in tomato up, height of flower be downer, the inside temperature become control up, nighttime temperature become up such as table 3. So, the farmer can do control the environment condition comparison with crop growth.
Future plan for smartfarm productivity improvement model
It is the future plan in smart farm bigdata research figure (Figure 7). For productivity improvement model and consulting from with data, we expand collection of farm data. And data will be also collected continuously by cropping season and data quality management will be maintained. Also, we develop the basic model for crop productivity improvement. And then we test the model for high accuracy model fitting from tomato smartfarm demonstration research. So, we will modify the productivity improvement model. Also, we will service the model for farmers from cloud service platform in RDA. Therefore, the model and data are used by smart farm industry, a farmer, and consumers.
CONCLUSION
In this study, we introduced the bigdata definition and bigdata in agriculture. Especially there are various data, environment, growth and yield data from smartfarm data in Korea. We collected the environment data from sensor automatically, and growth and yield data by researcher. These data are stacked with the Agriculture Bigdata Management System in RDA, and is managed to analyze. We have developed the tomato productivity improvement model and consulting farmers from with data. For precision of model, we expand collection of farm data in various field, like horticulture, main vegetable. There are a small number of data because of smartfarm type, size of facility and species of crop. Data will be also collected continuously by cropping season and data quality management will be maintained. Also, we will develop and the basic model for crop productivity improvement. And then we will service the model for farmers from cloud service platform in RDA.
REFERENCES
Cho, I. H., J. K. Kwon, D. M. Oh, H. D. Lee and T. W. Jung, 2013. The guideline for agriculture technology: horticulture, Rural Development Administration, Jeonju, Korea.
Han J. and M. K., 2015. Data Mining: concepts and Techniques, Elsevier, Inc, NY, USA.
Lee, H. R. and J.E. Song. 2018. The study of sharing and utilization horticulture bigdata, E-business research winter symposium.
Lee, G. H., Y. G. Ham, Y. D. Kim, J. H. Lee, and J. H. Won, 2016. The understanding of bigdata, knou press, Seoul, Korea.
Lee, H. R., S. H. Park, S. J. Park and D. H. Kim, 2018. The research of farmer feedback for improvement of productivity using horticulture smartfarm bigdata, horticultural science and technology 36(2): 211.
Lee, H. R., M. O. Park and S. J. Park 2018. The study of environment and growth variation in the regional tomato greenhouse facility by abnormal weather, 19thconference on agricultural and forest methodology: 132.
Lee, H. R., M. O. Kim, Y. B. Cho, S. J. Park and J. H. Hwang, 2018. The economic model for enhancement upgrade of tomato growth data in horticulture smart farm, horticultural science and technology 36(1): 80.
Lee, H. R., J. H. Hwang, M. O. Kim, and Y. B. Cho, 2017. Development of economic model for enhancement of tomato farmer’s productivity using Smartfarm bigdata, horticultural science and technology 36(2): 100.
Lee, H. R., Y. B. Cho, J. H. Hwang, D. H. Kim, Y. S. Yu, D. W. Choi, S. R. Kim, Y. J. Ahn, I. K. Ham, M. H. Jeon, G. W. Park, T. W. Kang, M. H. Yoon and S. Y. Lee, 2017. The research of collecting the controlled horticulture smart farm bigdata, horticultural science and technology 35(2): 1021.
Lee, H. R., S. J. Park, S. H. Park, and D. H. Kim, 2018. The bigdata analysis method for smartfarm environment factors management (tomato, 2nd edition), Rural Development Administration, Jeonju, Korea.
Lee, M. S., J. K. Jang and D. H. Kim, 2017. The guideline for agriculture technology: tomato, Rural Development Administration, Jeonju, Korea.
Park, S. H., H. R. Lee, S. J. Park and Y. B. Cho, 2018. Facility horticulture smart farm environment integrated solar radiation quality management of bigdata, horticultural science and technology 36(2): 213.
Park, Y. H., W. H. Cho, M. H. Na, D. H. Kim, Y. B. Cho, and H. Y. Lee, 2018. Extraction of Environmental Factors Influencing Strawberry Yield in the facility farms using pattern recognition techniques, horticultural science and technology 36(1): 48-49.
Yeom, J. K., D. K. Kim and I. H. Jang, 2017. The linear regression analysis using SAS and R, Jayu Academy, Seoul, Korea.
Reviewed, edited and uploaded: November 28, 2019