ABSTRACT
The number of animals per a farm worker tends to be larger as animal husbandry is shifting from small-holder family farms to corporate-owned large-scale farms in Japan, Asia-Pacific and Western countries. Emerging technologies are necessary to manage a large number of animas by a small number of people. The aim is to save on labor by means of precise farming, namely, keeping better animal welfare while improving animal production, increasing animal feed efficiency, and earlier detection of animal illness and zoonosis diseases. Precision livestock farming (PLF) might be defined as a real-time monitoring technologiy aimed to manage the smallest manageable production units, otherwise known as the ‘sensor-based’ individual animal approach or animal-focused decision-making approach, involves real-time activity, production and physiology sensing. PLF sensors generate huge amounts of data, and many actors benefit from PLF data along the livestock value chain. No well-accepted standards currently exist for sharing sensor-generated data, limiting the use of commercial sensors. This NARO-FFTC presentation will explore few selected PLF AI case studies currently running at the ARO PLF lab from academic research to on farm applications:
(1) Identifying the individual cow feed efficiency by 3D camera and artificial intelligence (AI).
(2) Mitigation of heat stress; sensing and controlling thermoregulation of individual broilers.
(3) Attenuating heat stress of dairy cows; applying rumen bolus temperature and real-time animal response.
(4) Monitoring water intake for treating individual animal in small ruminants (sheep and goats).
(5) Artificial Intelligence (AI) based bird deterrence from fish ponds.
(6) 3D camera, machine vision and machine learning in cattle body condition scoring
(7) Automatic lameness detection; tree-based model vs. 3D camera.
(8) Applying 3D camera and machine learning for heifer height and weight estimation.
PLF (using IoT and AI) have been developed in Japan and Western countries to facilitate smart livestock management. Further research may focus on how to adapt and validate PLF mentioned above in Japan and other Asia-Pacific regions and vice-versa adapting Japanese PLF technologies in other regions.
Presentation objectives. Sharing information on the state of the art research in PLF animal husbandry. Exchanging of ideas, technologies and knowledge on smart livestock industry with potential applications in Asian and Pacific region and establishing collaboration in research.
Expected audience. Participants from the academia, public sectors, companies and private sectors including policy makers, researchers, students and a general public.
Keywords: Precision Livestock Farming (PLF), cattle, beef, dairy, poultry, sheep, goats, farm management, animal welfare, IoT, machine vison, machine learning, lasso regression
INTRODUCTION
Precision livestock farming (PLF) might be defined as real-time monitoring technologies aimed at managing the smallest manageable production unit, otherwise known as the ‘sensor-based’ individual animal approach” or animal-centric decision-making approach involves real-time activity, production and physiology sensing (Halachmi et al., 2019). PLF sensors generate huge amounts of data, and many actors along the livestock value-chain benefit from PLF data. No well-accepted standards currently exist for sharing sensor-generated data, limiting the use of commercial sensors and sharing PLF data (Halachmi et al., 2019).
The text below is divided into few use cases that will be presented in the symposium:
WHO OWNS THE DATA? WHO BENEFITS FROM THE DATA?
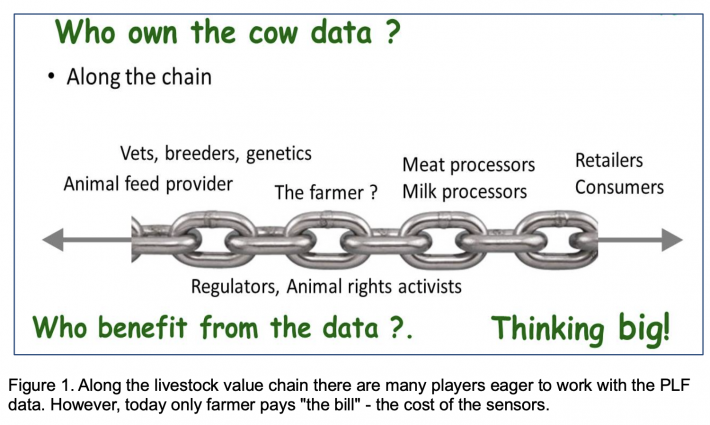
Figure 1. Along the livestock value chain there are many players eager to work with the PLF data. However, today only farmer pays "the bill" - the cost of the sensors.
It can be seen (Figure 1) that a farmer collects the animal data, which, though, can be beneficial also to animal feed suppliers, consultants such as vets, breeders, meat and milk processors, retailers, regulators, and animal rights movements and many others. However, today only the farmer pays the cost of the sensors. In future, other business models may allow other partners along the value chain to share the costs.
COW INDIVIDUAL FEED EFFICIENCY
The first widely adopted PLF application, years before the term PLF was coined, was the individual electronic milk meter for dairy cows in the 1970s (Peles, 1978), followed by commercialized behavior-based estrus detection, rumination tags (Bar & Solomon, 2010) and online real-time milk analyzers (Schmilovitch et al., 2001). Soon afterward, PLF was applied to other species (Halachmi et al., 2019)’s Table 1) maps the main sensors column wise, with the main applications written in rows (Halachmi et al., 2019 Table 1).
Individual feed intake of dairy cow is an important variable in dairy management (Halachmi et al., 2016; Holtenius et al., 2018; Bloch et al., 2019; Connor et al., 2019). Modern dairy farming focuses attention on individual cow productivity (González et al., 2008). ‘Individual feed efficiency’ can be estimated with information about the feed consumption of each individual cow, its milk production and composition (Volden, 2011). Furthermore, cow feeding is a significant component of farm expenses (Buza et al., 2014; Halachmi et al., 2016). To meet the demand for today’s expected milk production, dairy cows must consume a matched amount of feed, which constitutes approximately 50 % of the total production costs (Chiba, 2009). Feed wasted by a cow is a direct loss of profit for the farm, since the money spent on that feed cannot be recovered (Shelley, 2013). Proper monitoring of feed intake can determine the productivity of dairy cows and improve farm management decision-making (Shalloo et al., 2004). This may lead to increased overall farm productivity (Vandehaar, 1998; Herd et al., 2003; Buza et al., 2014; Halachmi et al., 2016).
Cow individual feed efficiency is an important parameter in the future dairy operation. Individual cow feed intake is intensively measured in research farms (Halachmi et al., 2019). In commercial farms, sensing the individual animal feed efficiency can be performed via three methods:
1. Mathematical models, including feeding behavior (Halachmi et al., 2016; Halachmi et al., 2004);
2. Camera and sensors. Observing eating behavior via camera and photogrammetry (Bloch et al., 2019) may provide an accurate measurement of feed intake, providing a measurement of the individual cows feed efficiency on commercial farms.; and direct measurements (bloch et al., 2020)
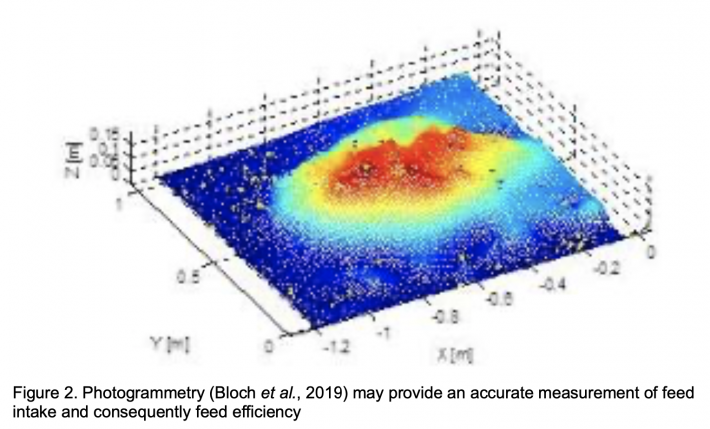
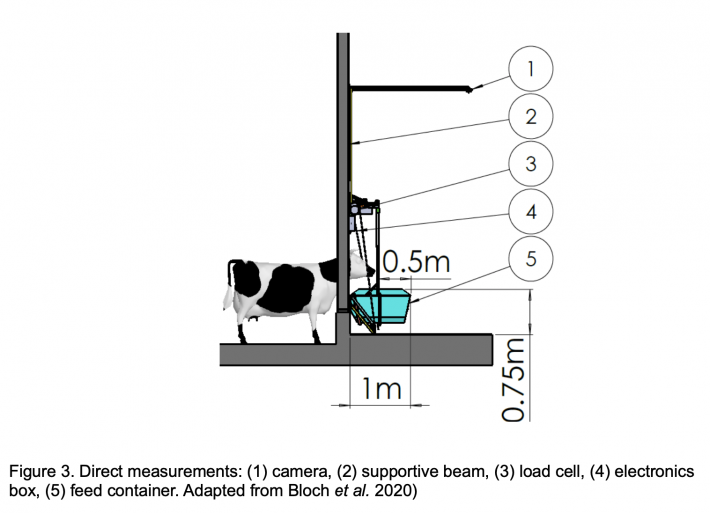
A new system (Figure 4. right side) was easy to install and maintain, and has self-cleaning feature. It yields a huge amount of data on a single meal level and the farm level.
Cow individual feed efficiency results
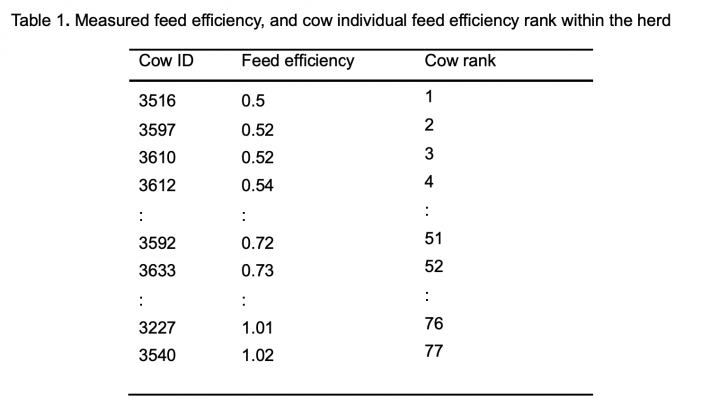
It can be seen (Table 1) that the individual cow feed efficiency has vast variation, from 500 g feed/kg milk to 1 kg feed/kg of milk.
The amount of data on a level of a single meal of individual cow raises up data owning issue mentioned above.
DETECTION OF LAMENESS IN DAIRY COWS: MACHINE VISION
Lameness in dairy cows is one of the most painful illnesses and therefore impairs animal welfare (Schlageter-Tello et al., 2014b; Schlageter-Tello et al., 2014a). Lameness follows only the mastitis in terms of its detrimental effects on herd productivity (Booth et al., 2004). Its annual incidence ranges between 4 and 55 cases per 100 cows per year (Booth et al., 2004), depending on farm, location, and year of study. The overall cost in the literature varies, approximately US$446 per case in the UK (Esslemont & Kossaibati, 1997) to an average cost per case of sole ulcer, digital dermatitis, and foot rot of 216.07, 132.96 and 120.70, respectively, in the USD (Cha et al., 2010). Detection of severe lameness is relatively easy; however, by the time the animal becomes severely lame, it is often difficult to treat the animal successfully. Dairy producers often miss subtle signs of lameness, hence, a monitoring system which is able to detect milder, subclinical cases of lameness could be beneficial. In 2002, at the University of Maryland Baltimore, it was hypothesized that measurements of vertical ground reaction forces as animals walk over a force–plate system could provide the basis for detecting lameness (G. Rajkondawar et al., 2002). This concept went through all the development processes to the market (Rajkondawar et al., 2006; G. Rajkondawar et al., 2002). Its commercial name was the StepMetrix™ lameness detection system by BouMatic. A pressure-sensor mat on which cows walk once or twice a day was developed (Van Nuffel et al., 2013; Chapinal et al., 2010; Van Der Tol et al., 2003) besides weight distribution systems (Pastell et al., 2010), but these systems are rather expensive. In 2006, a machine-vision based system was proposed (Song et al., 2008; Poursaberi et al., 2010; Pluk et al., 2010). This concept was explored in Israel together with other animal-related data that already existed in the farm management software (parameters that are correlated with lameness such as milk production and neck activity (Van Hertem et al., 2013b)). A side-view concept was validated (Van Hertem et al., 2013a) in 2013 and was replaced by an upper-view 3D camera (Schlageter-Tello et al., 2015; Viazzi et al., 2014; Van Hertem et al., 2014). Today, the combined system – a 3D camera together with animal production and related behavior parameters (Van Hertem et al., 2016) appears to be the “winning setup” (Van Hertem et al., 2017).
MITIGATING HEAT STRESS IN DAIRY COWS
Tailoring the cooling management to the cow’s thermal response can mitigate heat stress months (Ferreira et al., 2016; Polsky & von Keyserlingk, 2017; St-Pierre et al., 2003; Stull et al., 2008; West, 2003). Nowadays, cooling systems often function at a constant schedule, based on measuring the ambient environment temperature and not on monitoring the animal itself. We propose a dynamic cooling system, based on sensors (bolus) that measure the cows' body temperatures. With the help of this system, the cooling can be activated when needed approaching better efficiency. A total of 30 lactating cows were randomly assigned to one of two groups which received two different evaporative cooling regimes: a control group, which treated by the common methods used in farms, time-based cooling; and an experimental group, which received the sensor-based cooling regime, that was changed weekly (3 months in total) according to the cow's response to last week's change in terms of body temperature, as measured by boluses inserted to the reticulorumen. The two treatment groups of cows had similar milk yields (44.7 kg/d), but those in the experimental group had higher milk fat (3.65 vs 3.43%), higher milk protein (3.23 vs 3.13%), higher energy corrected milk (ECM, 42.84 vs 41.48 kg/d), higher fat corrected milk 4%; (FCM, 42.76 vs 41.34 kg/d) and shorter heat stress time (5.03 vs 9.46 hours/day) comparing to the control. Dry matter intake was higher in the experimental group. Daily visits to the feed trough were less frequent, and with each visit lasting longer. therefore, the sensor-based cooling regime may be an effective tool to detect and ease heat stress in high-producing dairy cows under summer heat load in arid and semi-arid zones.
CONCLUSION
Where are we now?
Technologies providing accurate data can only enhance a well-managed system by increasing the information available. The way how the data provided by these technologies is turned into actionable solutions is critical. At this point, wearable technologies dominate the market; most of the PLF applications are based on monitoring tags attached to the animal (neck, leg, or ear tags) or inside the animal (boluses). Therefore, current PLF applications are mainly used for larger animals such as dairy cows, beef cattle, and horses. The economic value of every single large animal justifies the investment of a monitoring tag per animal, and large animals provide many places to hang sensors on them. A single sensor, like a camera (Halachmi et al., 2008; Bercovich et al., 2013; Halachmi et al., 2013; Van Hertem et al., 2016; Van Hertem et al., 2017) or robot (Halachmi, 2004; John et al., 2016) should be sufficient for many smaller animals that naturally yields lower profits . Most existing commercial applications for pigs, fish and poultry monitor groups using cameras, sound or data from feeding systems. New sensor systems , from primarily wearable technologies to more image and milk-based systems, are expected to be introduced into the market. In “less value-per-animal” systems such as sheep, goats, pigs, poultry, and fish, raised intensively in large herds, flocks or schools, one sensor per herd (not a sensor per animal) such as camera or robot will be even more common (Halachmi et al., 2019).
Investment decisions should include a thorough, formal evaluation of profitability. The human factors related to successful technology adoption cannot be overlooked. Farmers are frequently skeptical about new business models, especially with new technology involved. Often, it is difficult to convince farmers to collaborate with digital innovation. That is one of the reasons why collaborative business models in the livestock sector are at a relatively early stage. If scientists are able to transfer their knowledge to farmers in a reliable and transparent way, perhaps, with the help of knowledge-sharing platforms and e-learning tools, there is potential to overcome such barriers to implement and generate significant value for all parts along the value chain. Excitement about technical capabilities must be balanced with consideration of implementation challenges and economic realities. In some cases, although the technologies may be scientifically and technically promising, the economic returns relative to the cost of the system limit adoption of the new technology. It is important to remember that livestock systems are, by nature, quite complex, and precision livestock farming technologies must be considered within the context of the whole system. For the most part, many of these technologies are still in the early phase within the adoption cycle. As they progress and become more mainstream, end-user demands for technology performance will increase (Halachmi et al., 2019).
What can be learned?
The PLF sensors generate huge amounts of data. Many actors benefit from PLF data along the chain: the animal feed providers can design their inventory based on animal growth, the meat or milk processing factories can predict coming quantity and quality and plan production accordingly, some consumers hope to apply objective animal welfare standards based on animal sensors, and the farmer who, based on the sensors’ alarms, can treat those individual animals that need special care. No standards currently exist for sharing sensor generated data, which limits the use of commercial sensors for research, animal breeding and benchmarking purposes, among others. Data sharing needs to be enabled in a way that benefits all parties, in order to fully utilize sensor-generated data and the opportunities arising from combining multiple data sources for new applications (Halachmi et al., 2019).
However, at the end of the day, the farmer pays the bill – the price of installing the PLF applications on his farm. The PLF applications that are economically justified, reduce labor, easy to use, and fit into known farm practices have a better chance to succeed commercially. Perhaps, one of the most important lessons learned with these technologies thus far is that they are not a magic fix for poor management. The livestock producers, who benefit the most from the use of these technologies, are the better managers.
Existing applications have mainly focused on dealing with single issues (such as diseases, estrus detection, heat stress mitigation, etc.), and in many cases use a single data source. Clearly, detect multiple conditions with the same sensor would be more useful, and this should be reflected in the study designs. Higher precision in detection of anomalies could also be achieved by combining data from multiple sources, e.g. production, physiology and behavior and by incorporating existing information about risk factors into the models.
Current studies have focused more on sensor development and modeling data collected from small to medium scale experiments (Halachmi et al., 2019). In order to turn such studies into actionable solutions and obtain accurate and robust detection of anomalies in the future, more focus is needed for large scale data collection with high-quality golden standards. This can be made possible by increasing the number of sensors deployed to commercial farms. More efficient use of expensive research data can also be facilitated via joint international modeling, data sharing initiatives, and adopting a collaboration model between industry, researchers, farmers, and stakeholders. The value of data increases when (un)structured data is processed, enriched and analyzed to create actionable insights. Operations can be further improved when farmers also share the information collected across the supply chain with relevant stakeholders, such as veterinarians, slaughterhouses, meat processors, and animal feed producers.
Where are we going?
Sustainable food production is one of today’s key global challenges. According to an FAO report (Robinson et al., 2011; Robinson et al., 2014); meat consumption in the first world has leveled off, but the share of animal products in the diet is increasing in developing countries (Figure 6). Between 1997/99 and 2030, annual meat consumption in developing countries is projected to increase from 25.5 to 37 kg per person, compared with an increase from 88 to 100 kg in industrialized countries. Consumption of milk and dairy products will rise from 45 kg/ person/p.a. to 66 kg in developing countries, and from 212 to 221 kg in industrialized countries. For eggs, consumption will grow from 6.5 to 8.9 kg in developing countries but only from 13.5 to 13.8 kg in industrialized countries. Adding up, by 2050 an expanded world population will be consuming two thirds more animal protein than it does today. Meat consumption is projected to rise nearly 73 percent by 2050; dairy consumption will grow 58 percent over current levels. The higher demand for animal products, together with public pressure to raise animals in compassionate ways, the concomitant decrease in available arable land, along with the desire to create smaller environmental footprints, will all encourage the industry to adopt more PLF applications. Furthermore, the world continues to become more affluent, and finding the labor for livestock farms is increasingly challenging. These challenges open the door for increased automation, sustainable intensification of livestock farms, consequently increasing development and use of PLF applications (Halachmi et al., 2019).
Digitalization offers the potential to make farming more sustainable. The implementation of Information and Communication Technology (ICT) in the livestock industry, and the recent use of Smart Networked Objects (SNO) and the Internet of Things (IoT), has opened a new era of communication in which things, humans and animals are part of a data network exchange, leading to a new concept of farming (Halachmi et al., 2019). Remote or wearable sensors can be combined with smart algorithms to continuously monitor a wide range of animal responses linked to stress, health status, and welfare. The idea of real-time monitoring assumes a simple way to measure variables that can provide clear and suitable early warnings to farmers, mitigating the severity and length of medical problems and improving outcomes. The prompt reaction to any change in health, welfare and production status is the key for the reduction in drug use and for the improvement of animal wellbeing. Precision Livestock Farming could be considered the right environment to realize these goals.
This NARO-FFTC presentation will explore selected PLF AI case studies currently running at the ARO PLF lab from academic research to on farm applications:
ACKNOWLEDGMENTS
This study was support by grants from the Israeli Chief Scientist of Agriculture, projects 459-451415 ("Kendel") and the Israel Dairy Board, fund number 459-4490, and we gratefully thank all the members of the Precision Livestock Farming (PLF) Laboratory. Special thanks to the lab's business development manager – Mr. Eitan Metuki (eitan@spark.co.il), Dr. Joshua Miron, Dr. Gaby Adin, and Mr. Ehud Ram.
REFERENCES
Bar D & Solomon R Year Rumination collars: what can they tell us. In Proceedings of the Proceedings of the First North American Conference on Precision Dairy Management, pp. 214-215.
Bercovich A, Edan Y, Alchanatis V, Moallem U, Parmet Y, Honig H, Maltz E, Antler A & Halachmi I 2013 Development of an automatic cow body condition scoring using body shape signature and Fourier descriptors. Journal of Dairy Science 96(12) 8047-8059
Bloch V, Levit H & Halachmi I 2019 Assessing the potential of photogrammetry to monitor feed intake of dairy cows. Journal of Dairy Research 1-6
bloch v, Levit H & I H 2020 Technical note: Design of a system for measuring individual cow feed intake in commercial dairies. Under review:
Booth CJ, Warnick LD, Gröhn YT, Maizon DO, Guard CL & Janssen D 2004 Effect of Lameness on Culling in Dairy Cows. Journal of Dairy Science 87(12) 4115-4122
Cha E, Hertl JA, Bar D & Gröhn YT 2010 The cost of different types of lameness in dairy cows calculated by dynamic programming. Prev Vet Med 97(1) 1-8
Chapinal N, de Passillé AM, Rushen J & Wagner S 2010 Automated methods for detecting lameness and measuring analgesia in dairy cattle. Journal of Dairy Science 93(5) 2007-2013
Esslemont RJ & Kossaibati MA 1997 Culling in 50 dairy herds in England. The Veterinary record 140(2) 36-39
Ferreira F, Gennari R, Dahl G & De Vries A 2016 Economic feasibility of cooling dry cows across the United States. Journal of dairy science 99(12) 9931-9941
G. Rajkondawar P, Tasch U, M. Lefcourt A, Erez B, M. Dyer R & A. Varner M 2002 A SYSTEM FOR IDENTIFYING LAMENESS IN DAIRY CATTLE. Applied Engineering in Agriculture 18(1) 87
Halachmi I 2004 Designing the automatic milking farm in a hot climate. Journal of Dairy Science 87(3) 764-775
Halachmi I, Edan Y, Moallem U & Maltz E 2004 Predicting feed intake of the individual dairy cow. Journal of Dairy Science 87(7) 2254-2267
Halachmi I, Guarino M, Bewley J & Pastell M 2019 Smart Animal Agriculture: Application of Real-Time Sensors to Improve Animal Well-Being and Production. Annual review of animal biosciences 7 403-425
Halachmi I, Klopčič M, Polak P, Roberts DJ & Bewley JM 2013 Automatic assessment of dairy cattle body condition score using thermal imaging. Computers and Electronics in Agriculture 99 35-40
Halachmi I, Meir YB, Miron J & Maltz E 2016 Feeding behavior improves prediction of dairy cow voluntary feed intake but cannot serve as the sole indicator. Animal 10(09) 1501-1506
Halachmi I, Polak P, Roberts DJ & Klopcic M 2008 Cow body shape and automation of condition scoring. Journal of Dairy Science 91(11) 4444-4451
John A, Clark C, Freeman M, Kerrisk K, Garcia S & Halachmi I 2016 Review: Milking robot utilization, a successful precision livestock farming evolution. Animal 10(09) 1484-1492
Pastell M, Hänninen L, de Passillé AM & Rushen J 2010 Measures of weight distribution of dairy cows to detect lameness and the presence of hoof lesions. Journal of Dairy Science 93(3) 954-960
Peles E 1978 Liquid Meter. In Book Liquid Meter, Vol. Au1978041401, pp. (Ed.^Eds. Editor).Series Liquid Meter. Afikim
Pluk A, Bahr C, Leroy T, Poursaberi A, Song X, Vranken E, Maertens W, Van Nuffel A & Berckmans D 2010 Evaluation of Step Overlap as an Automatic Measure in Dairy Cow Locomotion. Transactions of the Asabe 53(4) 1305
Polsky L & von Keyserlingk MA 2017 Invited review: Effects of heat stress on dairy cattle welfare. Journal of dairy science 100(11) 8645-8657
Poursaberi A, Bahr C, Pluk A, Van Nuffel A & Berckmans D 2010 Real-time automatic lameness detection based on back posture extraction in dairy cattle: Shape analysis of cow with image processing techniques. Computers and Electronics in Agriculture 74(1) 110-119
Rajkondawar PG, Liu M, Dyer RM, Neerchal NK, Tasch U, Lefcourt AM, Erez B & Varner MA 2006 Comparison of models to identify lame cows based on gait and lesion scores, and limb movement variables. Journal of Dairy Science 89(11) 4267-4275
Robinson TP, Thornton PK, Franceschini G, Kruska R, Chiozza F, Notenbaert AMO, Cecchi G, Herrero M, Epprecht M & Fritz S 2011 Global livestock production systems. FAO and ILRI
Robinson TP, Wint GW, Conchedda G, Van Boeckel TP, Ercoli V, Palamara E, Cinardi G, D'Aietti L, Hay SI & Gilbert M 2014 Mapping the global distribution of livestock. PLoS One 9(5) e96084
Schlageter-Tello A, Bokkers EA, Groot Koerkamp PW, Van Hertem T, Viazzi S, Romanini CE, Halachmi I, Bahr C, Berckmans D & Lokhorst K 2014a Effect of merging levels of locomotion scores for dairy cows on intra- and interrater reliability and agreement. Journal of Dairy Science 97(9) 5533-5542
Schlageter-Tello A, Bokkers EA, Koerkamp PW, Van Hertem T, Viazzi S, Romanini CE, Halachmi I, Bahr C, Berckmans D & Lokhorst K 2014b Manual and automatic locomotion scoring systems in dairy cows: a review. Prev Vet Med 116(1-2) 12-25
Schlageter-Tello A, Bokkers EAM, Groot Koerkamp PWG, Van Hertem T, Viazzi S, Romanini CEB, Halachmi I, Bahr C, Berckmans D & Lokhorst K 2015 Comparison of locomotion scoring for dairy cows by experienced and inexperienced raters using live or video observation methods. Animal Welfare 24(1) 69-79
Schmilovitch Z, Katz G, Halachmi I, Hoffman A, Kutscher M, Sarig M, Unar E, Egozi H & Maltz E 2001 Spectroscopic fluid analyzer. In Book Spectroscopic fluid analyzer, Vol., pp. (Ed.^Eds. Editor).Series Spectroscopic fluid analyzer.
Song X, Leroy T, Vranken E, Maertens W, Sonck B & Berckmans D 2008 Automatic detection of lameness in dairy cattle—Vision-based trackway analysis in cow's locomotion. Computers and Electronics in Agriculture 64(1) 39-44
St-Pierre N, Cobanov B & Schnitkey G 2003 Economic losses from heat stress by US livestock industries1. Journal of dairy science 86 E52-E77
Stull C, Messam LM, Collar C, Peterson N, Castillo A, Reed B, Andersen K & VerBoort W 2008 Precipitation and temperature effects on mortality and lactation parameters of dairy cattle in California. Journal of dairy science 91(12) 4579-4591
Van Der Tol PPJ, Metz JHM, Noordhuizen-Stassen EN, Back W, Braam CR & Weijs WA 2003 The vertical ground reaction force and the pressure distribution on the claws of dairy cows while walking on a flat substrate. Journal of Dairy Science 86(9) 2875-2883
Van Hertem T, Alchanatis V, Antler A, Maltz E, Halachmi I, Schlageter-Tello A, Lokhorst C, Viazzi S, Romanini CEB, Pluk A, Bahr C & Berckmans D 2013a Comparison of segmentation algorithms for cow contour extraction from natural barn background in side view images. Computers and Electronics in Agriculture 91 65-74
Van Hertem T, Bahr C, Tello AS, Viazzi S, Steensels M, Romanini C, Lokhorst C, Maltz E, Halachmi I & Berckmans D 2016 Lameness detection in dairy cattle: single predictor v. multivariate analysis of image-based posture processing and behaviour and performance sensing. Animal 10(09) 1525-1532
Van Hertem T, Maltz E, Antler A, Romanini CEB, Viazzi S, Bahr C, Schlageter-Tello A, Lokhorst C, Berckmans D & Halachmi I 2013b Lameness detection based on multivariate continuous sensing of milk yield, rumination, and neck activity. Journal of Dairy Science 96(7) 4286-4298
Van Hertem T, Schlageter Tello A, Viazzi S, Steensels M, Bahr C, Romanini CEB, Lokhorst K, Maltz E, Halachmi I & Berckmans D 2017 Implementation of an automatic 3D vision monitor for dairy cow locomotion in a commercial farm. Biosystems Engineering
Van Hertem T, Viazzi S, Steensels M, Maltz E, Antler A, Alchanatis V, Schlageter-Tello AA, Lokhorst K, Romanini ECB, Bahr C, Berckmans D & Halachmi I 2014 Automatic lameness detection based on consecutive 3D-video recordings. Biosystems Engineering 119 108-116
Van Nuffel A, Vangeyte J, Mertens KC, Pluym L, De Campeneere S, Saeys W, Opsomer G & Van Weyenberg S 2013 Exploration of measurement variation of gait variables for early lameness detection in cattle using the GAITWISE. Livestock Science 156(1-3) 88-95
Viazzi S, Bahr C, Van Hertem T, Schlageter-Tello A, Romanini CEB, Halachmi I, Lokhorst C & Berckmans D 2014 Comparison of a three-dimensional and two-dimensional camera system for automated measurement of back posture in dairy cows. Computers and Electronics in Agriculture 100 139-147
West J 2003 Effects of heat-stress on production in dairy cattle. Journal of dairy science 86(6) 2131-2144
Date submitted: October 24, 2019
Reviewed, edited and uploaded: November 18, 2019
|
Current Trends and Perspective of Precision Livestock Farming (PLF) with Relation to IoT and Data Science Tools
ABSTRACT
The number of animals per a farm worker tends to be larger as animal husbandry is shifting from small-holder family farms to corporate-owned large-scale farms in Japan, Asia-Pacific and Western countries. Emerging technologies are necessary to manage a large number of animas by a small number of people. The aim is to save on labor by means of precise farming, namely, keeping better animal welfare while improving animal production, increasing animal feed efficiency, and earlier detection of animal illness and zoonosis diseases. Precision livestock farming (PLF) might be defined as a real-time monitoring technologiy aimed to manage the smallest manageable production units, otherwise known as the ‘sensor-based’ individual animal approach or animal-focused decision-making approach, involves real-time activity, production and physiology sensing. PLF sensors generate huge amounts of data, and many actors benefit from PLF data along the livestock value chain. No well-accepted standards currently exist for sharing sensor-generated data, limiting the use of commercial sensors. This NARO-FFTC presentation will explore few selected PLF AI case studies currently running at the ARO PLF lab from academic research to on farm applications:
(1) Identifying the individual cow feed efficiency by 3D camera and artificial intelligence (AI).
(2) Mitigation of heat stress; sensing and controlling thermoregulation of individual broilers.
(3) Attenuating heat stress of dairy cows; applying rumen bolus temperature and real-time animal response.
(4) Monitoring water intake for treating individual animal in small ruminants (sheep and goats).
(5) Artificial Intelligence (AI) based bird deterrence from fish ponds.
(6) 3D camera, machine vision and machine learning in cattle body condition scoring
(7) Automatic lameness detection; tree-based model vs. 3D camera.
(8) Applying 3D camera and machine learning for heifer height and weight estimation.
PLF (using IoT and AI) have been developed in Japan and Western countries to facilitate smart livestock management. Further research may focus on how to adapt and validate PLF mentioned above in Japan and other Asia-Pacific regions and vice-versa adapting Japanese PLF technologies in other regions.
Presentation objectives. Sharing information on the state of the art research in PLF animal husbandry. Exchanging of ideas, technologies and knowledge on smart livestock industry with potential applications in Asian and Pacific region and establishing collaboration in research.
Expected audience. Participants from the academia, public sectors, companies and private sectors including policy makers, researchers, students and a general public.
Keywords: Precision Livestock Farming (PLF), cattle, beef, dairy, poultry, sheep, goats, farm management, animal welfare, IoT, machine vison, machine learning, lasso regression
INTRODUCTION
Precision livestock farming (PLF) might be defined as real-time monitoring technologies aimed at managing the smallest manageable production unit, otherwise known as the ‘sensor-based’ individual animal approach” or animal-centric decision-making approach involves real-time activity, production and physiology sensing (Halachmi et al., 2019). PLF sensors generate huge amounts of data, and many actors along the livestock value-chain benefit from PLF data. No well-accepted standards currently exist for sharing sensor-generated data, limiting the use of commercial sensors and sharing PLF data (Halachmi et al., 2019).
The text below is divided into few use cases that will be presented in the symposium:
WHO OWNS THE DATA? WHO BENEFITS FROM THE DATA?
Figure 1. Along the livestock value chain there are many players eager to work with the PLF data. However, today only farmer pays "the bill" - the cost of the sensors.
It can be seen (Figure 1) that a farmer collects the animal data, which, though, can be beneficial also to animal feed suppliers, consultants such as vets, breeders, meat and milk processors, retailers, regulators, and animal rights movements and many others. However, today only the farmer pays the cost of the sensors. In future, other business models may allow other partners along the value chain to share the costs.
COW INDIVIDUAL FEED EFFICIENCY
The first widely adopted PLF application, years before the term PLF was coined, was the individual electronic milk meter for dairy cows in the 1970s (Peles, 1978), followed by commercialized behavior-based estrus detection, rumination tags (Bar & Solomon, 2010) and online real-time milk analyzers (Schmilovitch et al., 2001). Soon afterward, PLF was applied to other species (Halachmi et al., 2019)’s Table 1) maps the main sensors column wise, with the main applications written in rows (Halachmi et al., 2019 Table 1).
Individual feed intake of dairy cow is an important variable in dairy management (Halachmi et al., 2016; Holtenius et al., 2018; Bloch et al., 2019; Connor et al., 2019). Modern dairy farming focuses attention on individual cow productivity (González et al., 2008). ‘Individual feed efficiency’ can be estimated with information about the feed consumption of each individual cow, its milk production and composition (Volden, 2011). Furthermore, cow feeding is a significant component of farm expenses (Buza et al., 2014; Halachmi et al., 2016). To meet the demand for today’s expected milk production, dairy cows must consume a matched amount of feed, which constitutes approximately 50 % of the total production costs (Chiba, 2009). Feed wasted by a cow is a direct loss of profit for the farm, since the money spent on that feed cannot be recovered (Shelley, 2013). Proper monitoring of feed intake can determine the productivity of dairy cows and improve farm management decision-making (Shalloo et al., 2004). This may lead to increased overall farm productivity (Vandehaar, 1998; Herd et al., 2003; Buza et al., 2014; Halachmi et al., 2016).
Cow individual feed efficiency is an important parameter in the future dairy operation. Individual cow feed intake is intensively measured in research farms (Halachmi et al., 2019). In commercial farms, sensing the individual animal feed efficiency can be performed via three methods:
1. Mathematical models, including feeding behavior (Halachmi et al., 2016; Halachmi et al., 2004);
2. Camera and sensors. Observing eating behavior via camera and photogrammetry (Bloch et al., 2019) may provide an accurate measurement of feed intake, providing a measurement of the individual cows feed efficiency on commercial farms.; and direct measurements (bloch et al., 2020)
A new system (Figure 4. right side) was easy to install and maintain, and has self-cleaning feature. It yields a huge amount of data on a single meal level and the farm level.
Cow individual feed efficiency results
It can be seen (Table 1) that the individual cow feed efficiency has vast variation, from 500 g feed/kg milk to 1 kg feed/kg of milk.
The amount of data on a level of a single meal of individual cow raises up data owning issue mentioned above.
DETECTION OF LAMENESS IN DAIRY COWS: MACHINE VISION
Lameness in dairy cows is one of the most painful illnesses and therefore impairs animal welfare (Schlageter-Tello et al., 2014b; Schlageter-Tello et al., 2014a). Lameness follows only the mastitis in terms of its detrimental effects on herd productivity (Booth et al., 2004). Its annual incidence ranges between 4 and 55 cases per 100 cows per year (Booth et al., 2004), depending on farm, location, and year of study. The overall cost in the literature varies, approximately US$446 per case in the UK (Esslemont & Kossaibati, 1997) to an average cost per case of sole ulcer, digital dermatitis, and foot rot of 216.07, 132.96 and 120.70, respectively, in the USD (Cha et al., 2010). Detection of severe lameness is relatively easy; however, by the time the animal becomes severely lame, it is often difficult to treat the animal successfully. Dairy producers often miss subtle signs of lameness, hence, a monitoring system which is able to detect milder, subclinical cases of lameness could be beneficial. In 2002, at the University of Maryland Baltimore, it was hypothesized that measurements of vertical ground reaction forces as animals walk over a force–plate system could provide the basis for detecting lameness (G. Rajkondawar et al., 2002). This concept went through all the development processes to the market (Rajkondawar et al., 2006; G. Rajkondawar et al., 2002). Its commercial name was the StepMetrix™ lameness detection system by BouMatic. A pressure-sensor mat on which cows walk once or twice a day was developed (Van Nuffel et al., 2013; Chapinal et al., 2010; Van Der Tol et al., 2003) besides weight distribution systems (Pastell et al., 2010), but these systems are rather expensive. In 2006, a machine-vision based system was proposed (Song et al., 2008; Poursaberi et al., 2010; Pluk et al., 2010). This concept was explored in Israel together with other animal-related data that already existed in the farm management software (parameters that are correlated with lameness such as milk production and neck activity (Van Hertem et al., 2013b)). A side-view concept was validated (Van Hertem et al., 2013a) in 2013 and was replaced by an upper-view 3D camera (Schlageter-Tello et al., 2015; Viazzi et al., 2014; Van Hertem et al., 2014). Today, the combined system – a 3D camera together with animal production and related behavior parameters (Van Hertem et al., 2016) appears to be the “winning setup” (Van Hertem et al., 2017).
MITIGATING HEAT STRESS IN DAIRY COWS
Tailoring the cooling management to the cow’s thermal response can mitigate heat stress months (Ferreira et al., 2016; Polsky & von Keyserlingk, 2017; St-Pierre et al., 2003; Stull et al., 2008; West, 2003). Nowadays, cooling systems often function at a constant schedule, based on measuring the ambient environment temperature and not on monitoring the animal itself. We propose a dynamic cooling system, based on sensors (bolus) that measure the cows' body temperatures. With the help of this system, the cooling can be activated when needed approaching better efficiency. A total of 30 lactating cows were randomly assigned to one of two groups which received two different evaporative cooling regimes: a control group, which treated by the common methods used in farms, time-based cooling; and an experimental group, which received the sensor-based cooling regime, that was changed weekly (3 months in total) according to the cow's response to last week's change in terms of body temperature, as measured by boluses inserted to the reticulorumen. The two treatment groups of cows had similar milk yields (44.7 kg/d), but those in the experimental group had higher milk fat (3.65 vs 3.43%), higher milk protein (3.23 vs 3.13%), higher energy corrected milk (ECM, 42.84 vs 41.48 kg/d), higher fat corrected milk 4%; (FCM, 42.76 vs 41.34 kg/d) and shorter heat stress time (5.03 vs 9.46 hours/day) comparing to the control. Dry matter intake was higher in the experimental group. Daily visits to the feed trough were less frequent, and with each visit lasting longer. therefore, the sensor-based cooling regime may be an effective tool to detect and ease heat stress in high-producing dairy cows under summer heat load in arid and semi-arid zones.
CONCLUSION
Where are we now?
Technologies providing accurate data can only enhance a well-managed system by increasing the information available. The way how the data provided by these technologies is turned into actionable solutions is critical. At this point, wearable technologies dominate the market; most of the PLF applications are based on monitoring tags attached to the animal (neck, leg, or ear tags) or inside the animal (boluses). Therefore, current PLF applications are mainly used for larger animals such as dairy cows, beef cattle, and horses. The economic value of every single large animal justifies the investment of a monitoring tag per animal, and large animals provide many places to hang sensors on them. A single sensor, like a camera (Halachmi et al., 2008; Bercovich et al., 2013; Halachmi et al., 2013; Van Hertem et al., 2016; Van Hertem et al., 2017) or robot (Halachmi, 2004; John et al., 2016) should be sufficient for many smaller animals that naturally yields lower profits . Most existing commercial applications for pigs, fish and poultry monitor groups using cameras, sound or data from feeding systems. New sensor systems , from primarily wearable technologies to more image and milk-based systems, are expected to be introduced into the market. In “less value-per-animal” systems such as sheep, goats, pigs, poultry, and fish, raised intensively in large herds, flocks or schools, one sensor per herd (not a sensor per animal) such as camera or robot will be even more common (Halachmi et al., 2019).
Investment decisions should include a thorough, formal evaluation of profitability. The human factors related to successful technology adoption cannot be overlooked. Farmers are frequently skeptical about new business models, especially with new technology involved. Often, it is difficult to convince farmers to collaborate with digital innovation. That is one of the reasons why collaborative business models in the livestock sector are at a relatively early stage. If scientists are able to transfer their knowledge to farmers in a reliable and transparent way, perhaps, with the help of knowledge-sharing platforms and e-learning tools, there is potential to overcome such barriers to implement and generate significant value for all parts along the value chain. Excitement about technical capabilities must be balanced with consideration of implementation challenges and economic realities. In some cases, although the technologies may be scientifically and technically promising, the economic returns relative to the cost of the system limit adoption of the new technology. It is important to remember that livestock systems are, by nature, quite complex, and precision livestock farming technologies must be considered within the context of the whole system. For the most part, many of these technologies are still in the early phase within the adoption cycle. As they progress and become more mainstream, end-user demands for technology performance will increase (Halachmi et al., 2019).
What can be learned?
The PLF sensors generate huge amounts of data. Many actors benefit from PLF data along the chain: the animal feed providers can design their inventory based on animal growth, the meat or milk processing factories can predict coming quantity and quality and plan production accordingly, some consumers hope to apply objective animal welfare standards based on animal sensors, and the farmer who, based on the sensors’ alarms, can treat those individual animals that need special care. No standards currently exist for sharing sensor generated data, which limits the use of commercial sensors for research, animal breeding and benchmarking purposes, among others. Data sharing needs to be enabled in a way that benefits all parties, in order to fully utilize sensor-generated data and the opportunities arising from combining multiple data sources for new applications (Halachmi et al., 2019).
However, at the end of the day, the farmer pays the bill – the price of installing the PLF applications on his farm. The PLF applications that are economically justified, reduce labor, easy to use, and fit into known farm practices have a better chance to succeed commercially. Perhaps, one of the most important lessons learned with these technologies thus far is that they are not a magic fix for poor management. The livestock producers, who benefit the most from the use of these technologies, are the better managers.
Existing applications have mainly focused on dealing with single issues (such as diseases, estrus detection, heat stress mitigation, etc.), and in many cases use a single data source. Clearly, detect multiple conditions with the same sensor would be more useful, and this should be reflected in the study designs. Higher precision in detection of anomalies could also be achieved by combining data from multiple sources, e.g. production, physiology and behavior and by incorporating existing information about risk factors into the models.
Current studies have focused more on sensor development and modeling data collected from small to medium scale experiments (Halachmi et al., 2019). In order to turn such studies into actionable solutions and obtain accurate and robust detection of anomalies in the future, more focus is needed for large scale data collection with high-quality golden standards. This can be made possible by increasing the number of sensors deployed to commercial farms. More efficient use of expensive research data can also be facilitated via joint international modeling, data sharing initiatives, and adopting a collaboration model between industry, researchers, farmers, and stakeholders. The value of data increases when (un)structured data is processed, enriched and analyzed to create actionable insights. Operations can be further improved when farmers also share the information collected across the supply chain with relevant stakeholders, such as veterinarians, slaughterhouses, meat processors, and animal feed producers.
Where are we going?
Sustainable food production is one of today’s key global challenges. According to an FAO report (Robinson et al., 2011; Robinson et al., 2014); meat consumption in the first world has leveled off, but the share of animal products in the diet is increasing in developing countries (Figure 6). Between 1997/99 and 2030, annual meat consumption in developing countries is projected to increase from 25.5 to 37 kg per person, compared with an increase from 88 to 100 kg in industrialized countries. Consumption of milk and dairy products will rise from 45 kg/ person/p.a. to 66 kg in developing countries, and from 212 to 221 kg in industrialized countries. For eggs, consumption will grow from 6.5 to 8.9 kg in developing countries but only from 13.5 to 13.8 kg in industrialized countries. Adding up, by 2050 an expanded world population will be consuming two thirds more animal protein than it does today. Meat consumption is projected to rise nearly 73 percent by 2050; dairy consumption will grow 58 percent over current levels. The higher demand for animal products, together with public pressure to raise animals in compassionate ways, the concomitant decrease in available arable land, along with the desire to create smaller environmental footprints, will all encourage the industry to adopt more PLF applications. Furthermore, the world continues to become more affluent, and finding the labor for livestock farms is increasingly challenging. These challenges open the door for increased automation, sustainable intensification of livestock farms, consequently increasing development and use of PLF applications (Halachmi et al., 2019).
Digitalization offers the potential to make farming more sustainable. The implementation of Information and Communication Technology (ICT) in the livestock industry, and the recent use of Smart Networked Objects (SNO) and the Internet of Things (IoT), has opened a new era of communication in which things, humans and animals are part of a data network exchange, leading to a new concept of farming (Halachmi et al., 2019). Remote or wearable sensors can be combined with smart algorithms to continuously monitor a wide range of animal responses linked to stress, health status, and welfare. The idea of real-time monitoring assumes a simple way to measure variables that can provide clear and suitable early warnings to farmers, mitigating the severity and length of medical problems and improving outcomes. The prompt reaction to any change in health, welfare and production status is the key for the reduction in drug use and for the improvement of animal wellbeing. Precision Livestock Farming could be considered the right environment to realize these goals.
This NARO-FFTC presentation will explore selected PLF AI case studies currently running at the ARO PLF lab from academic research to on farm applications:
ACKNOWLEDGMENTS
This study was support by grants from the Israeli Chief Scientist of Agriculture, projects 459-451415 ("Kendel") and the Israel Dairy Board, fund number 459-4490, and we gratefully thank all the members of the Precision Livestock Farming (PLF) Laboratory. Special thanks to the lab's business development manager – Mr. Eitan Metuki (eitan@spark.co.il), Dr. Joshua Miron, Dr. Gaby Adin, and Mr. Ehud Ram.
REFERENCES
Bar D & Solomon R Year Rumination collars: what can they tell us. In Proceedings of the Proceedings of the First North American Conference on Precision Dairy Management, pp. 214-215.
Bercovich A, Edan Y, Alchanatis V, Moallem U, Parmet Y, Honig H, Maltz E, Antler A & Halachmi I 2013 Development of an automatic cow body condition scoring using body shape signature and Fourier descriptors. Journal of Dairy Science 96(12) 8047-8059
Bloch V, Levit H & Halachmi I 2019 Assessing the potential of photogrammetry to monitor feed intake of dairy cows. Journal of Dairy Research 1-6
bloch v, Levit H & I H 2020 Technical note: Design of a system for measuring individual cow feed intake in commercial dairies. Under review:
Booth CJ, Warnick LD, Gröhn YT, Maizon DO, Guard CL & Janssen D 2004 Effect of Lameness on Culling in Dairy Cows. Journal of Dairy Science 87(12) 4115-4122
Cha E, Hertl JA, Bar D & Gröhn YT 2010 The cost of different types of lameness in dairy cows calculated by dynamic programming. Prev Vet Med 97(1) 1-8
Chapinal N, de Passillé AM, Rushen J & Wagner S 2010 Automated methods for detecting lameness and measuring analgesia in dairy cattle. Journal of Dairy Science 93(5) 2007-2013
Esslemont RJ & Kossaibati MA 1997 Culling in 50 dairy herds in England. The Veterinary record 140(2) 36-39
Ferreira F, Gennari R, Dahl G & De Vries A 2016 Economic feasibility of cooling dry cows across the United States. Journal of dairy science 99(12) 9931-9941
G. Rajkondawar P, Tasch U, M. Lefcourt A, Erez B, M. Dyer R & A. Varner M 2002 A SYSTEM FOR IDENTIFYING LAMENESS IN DAIRY CATTLE. Applied Engineering in Agriculture 18(1) 87
Halachmi I 2004 Designing the automatic milking farm in a hot climate. Journal of Dairy Science 87(3) 764-775
Halachmi I, Edan Y, Moallem U & Maltz E 2004 Predicting feed intake of the individual dairy cow. Journal of Dairy Science 87(7) 2254-2267
Halachmi I, Guarino M, Bewley J & Pastell M 2019 Smart Animal Agriculture: Application of Real-Time Sensors to Improve Animal Well-Being and Production. Annual review of animal biosciences 7 403-425
Halachmi I, Klopčič M, Polak P, Roberts DJ & Bewley JM 2013 Automatic assessment of dairy cattle body condition score using thermal imaging. Computers and Electronics in Agriculture 99 35-40
Halachmi I, Meir YB, Miron J & Maltz E 2016 Feeding behavior improves prediction of dairy cow voluntary feed intake but cannot serve as the sole indicator. Animal 10(09) 1501-1506
Halachmi I, Polak P, Roberts DJ & Klopcic M 2008 Cow body shape and automation of condition scoring. Journal of Dairy Science 91(11) 4444-4451
John A, Clark C, Freeman M, Kerrisk K, Garcia S & Halachmi I 2016 Review: Milking robot utilization, a successful precision livestock farming evolution. Animal 10(09) 1484-1492
Pastell M, Hänninen L, de Passillé AM & Rushen J 2010 Measures of weight distribution of dairy cows to detect lameness and the presence of hoof lesions. Journal of Dairy Science 93(3) 954-960
Peles E 1978 Liquid Meter. In Book Liquid Meter, Vol. Au1978041401, pp. (Ed.^Eds. Editor).Series Liquid Meter. Afikim
Pluk A, Bahr C, Leroy T, Poursaberi A, Song X, Vranken E, Maertens W, Van Nuffel A & Berckmans D 2010 Evaluation of Step Overlap as an Automatic Measure in Dairy Cow Locomotion. Transactions of the Asabe 53(4) 1305
Polsky L & von Keyserlingk MA 2017 Invited review: Effects of heat stress on dairy cattle welfare. Journal of dairy science 100(11) 8645-8657
Poursaberi A, Bahr C, Pluk A, Van Nuffel A & Berckmans D 2010 Real-time automatic lameness detection based on back posture extraction in dairy cattle: Shape analysis of cow with image processing techniques. Computers and Electronics in Agriculture 74(1) 110-119
Rajkondawar PG, Liu M, Dyer RM, Neerchal NK, Tasch U, Lefcourt AM, Erez B & Varner MA 2006 Comparison of models to identify lame cows based on gait and lesion scores, and limb movement variables. Journal of Dairy Science 89(11) 4267-4275
Robinson TP, Thornton PK, Franceschini G, Kruska R, Chiozza F, Notenbaert AMO, Cecchi G, Herrero M, Epprecht M & Fritz S 2011 Global livestock production systems. FAO and ILRI
Robinson TP, Wint GW, Conchedda G, Van Boeckel TP, Ercoli V, Palamara E, Cinardi G, D'Aietti L, Hay SI & Gilbert M 2014 Mapping the global distribution of livestock. PLoS One 9(5) e96084
Schlageter-Tello A, Bokkers EA, Groot Koerkamp PW, Van Hertem T, Viazzi S, Romanini CE, Halachmi I, Bahr C, Berckmans D & Lokhorst K 2014a Effect of merging levels of locomotion scores for dairy cows on intra- and interrater reliability and agreement. Journal of Dairy Science 97(9) 5533-5542
Schlageter-Tello A, Bokkers EA, Koerkamp PW, Van Hertem T, Viazzi S, Romanini CE, Halachmi I, Bahr C, Berckmans D & Lokhorst K 2014b Manual and automatic locomotion scoring systems in dairy cows: a review. Prev Vet Med 116(1-2) 12-25
Schlageter-Tello A, Bokkers EAM, Groot Koerkamp PWG, Van Hertem T, Viazzi S, Romanini CEB, Halachmi I, Bahr C, Berckmans D & Lokhorst K 2015 Comparison of locomotion scoring for dairy cows by experienced and inexperienced raters using live or video observation methods. Animal Welfare 24(1) 69-79
Schmilovitch Z, Katz G, Halachmi I, Hoffman A, Kutscher M, Sarig M, Unar E, Egozi H & Maltz E 2001 Spectroscopic fluid analyzer. In Book Spectroscopic fluid analyzer, Vol., pp. (Ed.^Eds. Editor).Series Spectroscopic fluid analyzer.
Song X, Leroy T, Vranken E, Maertens W, Sonck B & Berckmans D 2008 Automatic detection of lameness in dairy cattle—Vision-based trackway analysis in cow's locomotion. Computers and Electronics in Agriculture 64(1) 39-44
St-Pierre N, Cobanov B & Schnitkey G 2003 Economic losses from heat stress by US livestock industries1. Journal of dairy science 86 E52-E77
Stull C, Messam LM, Collar C, Peterson N, Castillo A, Reed B, Andersen K & VerBoort W 2008 Precipitation and temperature effects on mortality and lactation parameters of dairy cattle in California. Journal of dairy science 91(12) 4579-4591
Van Der Tol PPJ, Metz JHM, Noordhuizen-Stassen EN, Back W, Braam CR & Weijs WA 2003 The vertical ground reaction force and the pressure distribution on the claws of dairy cows while walking on a flat substrate. Journal of Dairy Science 86(9) 2875-2883
Van Hertem T, Alchanatis V, Antler A, Maltz E, Halachmi I, Schlageter-Tello A, Lokhorst C, Viazzi S, Romanini CEB, Pluk A, Bahr C & Berckmans D 2013a Comparison of segmentation algorithms for cow contour extraction from natural barn background in side view images. Computers and Electronics in Agriculture 91 65-74
Van Hertem T, Bahr C, Tello AS, Viazzi S, Steensels M, Romanini C, Lokhorst C, Maltz E, Halachmi I & Berckmans D 2016 Lameness detection in dairy cattle: single predictor v. multivariate analysis of image-based posture processing and behaviour and performance sensing. Animal 10(09) 1525-1532
Van Hertem T, Maltz E, Antler A, Romanini CEB, Viazzi S, Bahr C, Schlageter-Tello A, Lokhorst C, Berckmans D & Halachmi I 2013b Lameness detection based on multivariate continuous sensing of milk yield, rumination, and neck activity. Journal of Dairy Science 96(7) 4286-4298
Van Hertem T, Schlageter Tello A, Viazzi S, Steensels M, Bahr C, Romanini CEB, Lokhorst K, Maltz E, Halachmi I & Berckmans D 2017 Implementation of an automatic 3D vision monitor for dairy cow locomotion in a commercial farm. Biosystems Engineering
Van Hertem T, Viazzi S, Steensels M, Maltz E, Antler A, Alchanatis V, Schlageter-Tello AA, Lokhorst K, Romanini ECB, Bahr C, Berckmans D & Halachmi I 2014 Automatic lameness detection based on consecutive 3D-video recordings. Biosystems Engineering 119 108-116
Van Nuffel A, Vangeyte J, Mertens KC, Pluym L, De Campeneere S, Saeys W, Opsomer G & Van Weyenberg S 2013 Exploration of measurement variation of gait variables for early lameness detection in cattle using the GAITWISE. Livestock Science 156(1-3) 88-95
Viazzi S, Bahr C, Van Hertem T, Schlageter-Tello A, Romanini CEB, Halachmi I, Lokhorst C & Berckmans D 2014 Comparison of a three-dimensional and two-dimensional camera system for automated measurement of back posture in dairy cows. Computers and Electronics in Agriculture 100 139-147
West J 2003 Effects of heat-stress on production in dairy cattle. Journal of dairy science 86(6) 2131-2144
Date submitted: October 24, 2019
Reviewed, edited and uploaded: November 18, 2019