ABSTRACT
In Thailand, agriculture is a key social activity and operate by 5.911 million small farms using 18.7 million ha and covers approximately 30% of land resources with diverse biotic and abiotic ecosystems. However, with demands for food security with environmentally friendly solutions as well as efficient resource utilization situations, it is imperative for researchers, small farms operators, consumers and policy makers to form partnership to co-develop operational and practical smart agriculture solutions for environmentally and consumer friendly food production. TRF-PA network, established in 2015 under agriculture division, aimed to transform Thai Agriculture, with Sustainable Development Goals (SDG) under Sufficiency Economy Philosophy (SEP) as our background operational framework. The network created teams of farmers and researchers to handle such situations. The teams co-designed research outputs that strengthen the capacity of the growers to solve the situation based on local and global databases as a component of various decision support system tools. This is an example of ‘SDGs Thailand model’ for enhancing livelihood of small farms with stability, prosperity and sustainability under SEP.
Keywords: Minimum Data Set, Resource Use Efficiency, immediate evidence under long-term view, Education systems.
INTRODUCTION
Sustainable and smart agriculture for environmentally and consumer friendly food production is a widely discussed topics and concerned by various stakeholder and concerned partners in global goal (URL1) and technical meetings and Conference (Hayati, 2017). The term sustainable agriculture for environmentally and consumer friendly food production begun its first appearance in 1980s with a variety of implementations. Sustainable agriculture defined as the ability of a given agricultural production system within an agroecosystem, mostly by small farms, that can return to same level of productivity after a period under stress and/or perturbations (Conway, 1985; Gold, 1990; Pretty, 2008). It is also a long-term view that research institutions and society must invest in generating and integrating wide array of knowledge and technologies to make better decisions at all societal levels to allocate limited resources to produce raw materials for food, fuel, feed, and medicine for happy family and society and health ecosystems (Brundtland, 1987; Buhler et al., 2002). However, the definition implies integrated efforts of concerned parties to maintain the ability of the system to produce a certain level of quantity, not so much on quality of the products and the environment.
The term smart agriculture for environmentally and consumer friendly food production begun its first appearance in 2010s with a variety of implementations. A more common term in recent years is ‘Climate-Smart Agriculture’, which defined as agricultural practices that sustainably improve production, resilience of production systems, and reduce greenhouse gas emissions is required to overcome climate extremes and variability (FAO, 2013; Nhamo and Chikoye, 2017). This definition implies integrated efforts of concerned parties to maintain the ability of the system to produce a certain level of quantity and maintain quality of the products and the environment, however, more resources must be allocated to improve livelihood quality of small farm communities, consumers and the environment.
Graeub et al. (2016) documented, at the global level, family farms constitute over 98% of all farms (475,067,424 holdings) and work on 53% of global agricultural land (1,009,507,053 ha out of 1,906,921,938 ha). In Thailand, agricultural census in 2013 revealed that a total of 5.911 million farm families, of which 47.5% agriculture was the only source of income. These farms operate on 18.7 million ha, some 70.0% are located in the Northeast (46.8%) and North (23.6%) regions of the country. The national average farm size was 3.2 ha per household and remained relative stable since 1993 agricultural census (NSO, 2014).
Recognizing the need to improve small farm’s livelihood with efficiency and effectiveness of agricultural and natural resource uses without causing negative impact on the environment, in October 2015 the Agriculture Division established the TRF-PA network as a coordinating office to support the Division’s efforts in identifying and prioritizing research agenda and approach to achieve the need, based on precision agriculture technology (PAT) and a series of precision agriculture prototype (PAP). We are interacting with PAP to develop and implement PATs in order to improve their situations through the full range of system approaches and improved understanding of the benefits of ecological and agronomic management, manipulation and redesign the current practices towards sustainable and smart precision agricultural production systems (Table 1). Our network focuses our resources to achieve the Sustainable Development Goals (SDG) under Sufficiency Economy Philosophy (SEP), as our background operational framework. This is a partnership of ‘SDGs Thailand model’ for enhancing livelihood of small farms with stability, properity and sustainability under SEP.
TRF-PA is a coordinating office of the Agriculture Division, a continuation of TRF-DSS network (Jintrawet et al., 2012), that uses a peer-support process and interdisciplinary in nature and based on system approaches which has been practiced in the Division. The process is flexible and links production systems to logistics and consumers, using both soft and hard system methods to gain better understandings and identify key and common situations. TRF-PA vision is ‘Thailand is a precision production agriculture country’.
Table 1: Comparison of Natural ecosystems, Sustainable-Smart TRF-PA and Other PA.
Issue
|
Natural ecosystems
|
Sustainable-Smart TRF-PA project
|
Other Agricultural systems
|
Nature of task/team work
|
|
- Variations & resource use efficiency situation faced by producers and concerned by Producer -Environment-Consumer-driven (PEC-driven or Team-driven).
- Peer-support (User, researcher, TRF-Ag Division).
- Multi-discipline/networking with peers.
|
- Precision Agriculture Technology-driven (PAT-driven).
- Single discipline.
|
Research questions and inputs
|
- None, high dependence on local resources.
|
- Medium dependence on local resources.
- Dialogue sessions based on situation faced by Producers.
- Peer-support process to co-design research activities to develop solutions to improve situation with Precision Agriculture Technology (PAT).
|
- High dependence on external resources.
- Based on researcher’s interests.
|
Research, Monitor & Evaluation process
|
|
- Natural processes plus Minimum Data Sets from PAT to make decisions.
- On-site with producer’s real situation and Site visit.
- Six month progress reports & Annual meeting with experts.
|
- In Laboratory, on-station of each discipline.
|
Outputs
|
- Medium productivity, high species diversity, closed nutrient cycling, medium output stability.
|
- Medium-high productivity, medium species diversity, semi-closed nutrient cycling, medium output stability.
- New integrated knowledge and publications.
- Minimum Data Sets of Agricultural resource database and decision support systems to handle variations.
- Learning media and tools to handle variations & resource use efficiency situation.
- Manual for implementation of solution with PAT to enhance producer’s capacity to handle situation.
- Patents (Weather datalogger by Asst. Prof. Dr. Krirk’s team, KKU).
- Startup companies (Chemical Sensor Systems for soil nutrient status by Prof. Dr. Kate’s team, CMU).
- Integrated weather, irrigation and fertiligation systems from small farms.
|
- High productivity, low species diversity, open nutrient cycling, medium-low output stability.
- New knowledge & Publications in journals with impact factor, within disciplinary line.
- Patents.
|
Outcome
|
|
- Enhance capacity to handle situations based on teamwork and PAT skills & manuals.
- TRF-PA Network in and outside Thailand.
|
- Citations and deepening disciplinary knowledge-base.
|
Impact
|
|
- PEC transformation from analog to digital agriculture using PAT.
- PEC ability to handle complexity into SDG(SEP).
|
- Citations and deepening disciplinary knowledge-base.
|
Source: Conway (1985); Pretty (2008); Jintrawet (2019b).
OBJECTIVES OF TRF-PA
To achieve the vision, TRF-PA was tasked with two main objectives as follows;
- To establish PA teams to co-design and co-implement knowledge and agricultural production technologies to put the right amount of resources to the right time and right place based on geo-referenced data sets for efficient resource utilization, and
- To collaborate and establish PA cases and prototypes to deal with the situation based on minimum data sets.
TRF-PA Approach to identify and priority research agenda
The key contribution of TRF-PA is our approach into handling a given situation faced by small farms through a peer-support process (Jintrawet et al., 2012). The process is our background operational framework also based on Sustainable Development Goals (SDG) and implemented under Sufficiency Economy Philosophy (SEP). Therefore, the process allow team to focus our resources on research agenda that relevant to and directly operating by small holder producers and local networks of producers (the primary production component or the upstream component), manufacturing and logistic companies (the middle component, linking production to consumption component or the mid-stream component) and the consumers (the main user of agricultural and natural products or the downstream component).
The peer-support process is based on the principles that peers provide comments and technical knowledge to encourage research members and teams from various institutions to join in efforts to co-develop solutions with users in the real crop production situation and settings. Team members interact to achieve the common and defined goals. The group collectively defines research activities, approaches to the questions, expected PA outputs and outcomes, and proposed research budget to TRF for funding. The project workload is also defined and shared among team members. TRF-PA peer-support process consists of six interrelated steps (Figure 1).
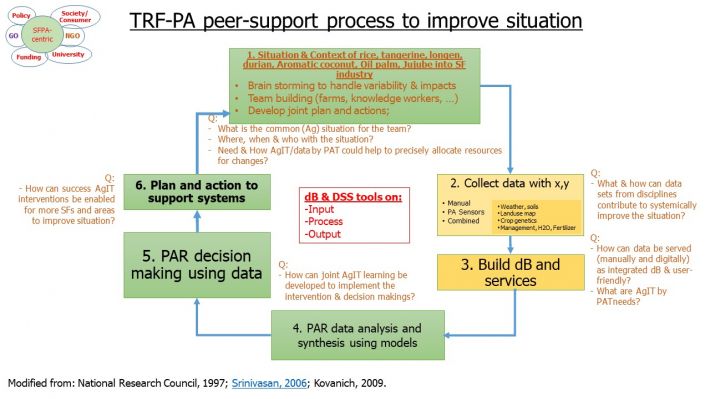
Figure 1: TRF-PA Peer-support process for identifying and prioritizing research agenda/theme
Source: Jintrawet (2019a)
Step 1: TRF-PA and the researcher team organized a series of brainstorm[1] sessions, widely used in medical (Krone, 2017) and higher education (Al-Samarraie and Hurmuzan, 2018) situations, to gain better understandings of grower’s situation under TRF’s granting policy context. Once a common research topic has been reached, TRF-PA provides guidelines for the team to formulate a research proposal and related documents for comments from anonymous experts and approval from TRF. This step normally takes 3-6 months, then another 2-3 weeks for TRF to transfer their first six-month budget for the team to operate.
Step 2: Once TRF transferred the research fund, the research team and growers/farmers jointly implement the project and start to collect geo-referenced data sets with precision agriculture technologies (PAT). Under TRF, PATs such as weather datalogger, soil chemical sensor (CSS), and integrated weather datalogger and irrigation and fertigation systems were funded to develop affordable and reliable PATs. Data sets collected by these PATs allow communication and interaction between the researcher team and growers/farmers to improve the situations as well as support the transition into digital agriculture (REF). These unbiased data sets generate better understandings of the situation, predict consequences of a given production systems if the farmer allocate resources differently, and allow farmer to make better decisions to plan and manage limited farm resources. In addition, these data sets provide evidence to gain better understanding of the relationships of various components in the given agroecosystem, provide valuable platform to develop, calibrate and evaluate process-oriented and empirical models to capture the behaviors of the agroecosystem under various scenarios, and the partners can use the models to collaborate to allocate limited resources to improve resource use efficiency at the plot-farm level.
Step 3: The research team develops the databases and decision support system tools to provide services for users to gain access to the data sets in improve their situations (Table 2). These databases, models and DSS tools are important component of the quest for high quality and evidence-based decision making process in agriculture to improve situations, i.e., farm productivity and environmental outputs (Rose et al., 2016). Currently, observed (URL2, 2019) and simulated (URL3, 2019) weather databases are being developed and implemented for user’s access and applications. A DSS tool was designed to assist in seasonal fragrant rice yield forecasts in Thailand using simulated weather data sets, soil data sets, and CSM-CERES-Rice simulation model (Kaeomuangmoon, 2018).
Step 4: The research team and the growers/farmers participate in using data sets to analyze and synthesize appropriate choices to improve a given situation overtime and space. For example, the aromatic coconut (Sisanong et al., 2019) and the oil palm (Anothai et al., 2018) teams installed integrated irrigation and crop nutrient application systems based on data sets collected by the weather data sensors and logger and crop water requirement (Allen et al., 1998). Irrigation amount and timing for both crops was triggered by evapotranspiration calculation using data sensed by on-site weather condition sensor and logger.
Step 5: TRF Agriculture Division, TRF-PA, and the research team participate in making decision to strategically allocate resources to implement the evaluated and accepted solution for a given situation. Specifically, the integrated weather datalogger and irrigation and nutrient application system is being considered by durian, tangerine and jujube growers in major production provinces in Thailand. In addition, CCS is currently under consideration by the Thailand Rice Department to speed up a task of producing reasonable soil fertility map of 22 consolidated field, a development-oriented project initiated by the Ministry of Agriculture and Cooperatives (MOAC), in the Lower part of the Northeast of Thailand.
Step 6: The successful PATs and PAPs are systemically scaling up based on supportive policy, plan, action and sufficient resources to reach the expected state with supports from various Government agencies and individual grower and their cooperatives. This is in line with our vision that TRF-PA network had demonstrated that small farm agricultural production systems in the future is sustainable and smart based on the successful integration of a range of global knowledge and adaptive technologies for the local settings that are climate responsive and environmentally enhancing.
The peer support process has the potential to provide considerable amounts of valuable data and information for decision making in every step in ‘new’ and ‘local’ precision agriculture. Opportunities are also arising for us to collectively enhance our capacity to convert these data sets into useful suggestions to aid in the decision-making process of various key personnel of the whole spectrum of ARD.
Table 2: Aims and outputs of research projects under TRF-PA by granting group.
Project
|
Aim
|
Output
|
Outcome
|
Reference
|
PA Protype of crop production situation at the field level (PAP)
|
Tangerine deteriorating growth and yield.
|
Co-develop mixed cultural practices to improve the plant.
|
Bio-technique to improve tangerine growth and yield.
|
Other growers are adopting the bio-technique
|
To-anan et al. (2019)
|
Aromatic coconut
|
Co-develop integrated system for irrigation and nutrient management system.
|
Integrated system of weather station for irrigation and nutrient management system.
|
The grower expanding the new systems
|
On-going project.
|
Phytophthora Disease of Durian
|
Co-develop bio-technique to control the disease.
|
Bio-technique to manage the disease
|
|
On-going project.
|
Oil palm seedlings establishment
|
Co-develop integrated system for irrigation and nutrient management system.
|
Integrated system of weather station for irrigation and nutrient management system.
|
|
On-going project.
|
Jujube water and nutrients management
|
Co-develop integrated system for irrigation and nutrient management system.
|
Integrated system of weather station for irrigation and nutrient management system.
|
|
On-going project.
|
PA Protype of crop production situation at the provincial level (PAP) and watershed level
|
Organic rice suitability
|
Co-develop agro-informatic system for self-evaluate suitability of a plot
|
ORSuit an App. for farmers’ self-assessment the suitability class of his plot to join the project.
|
|
On-going project.
|
Antibiotics in tangerine products, Fang Watershed, Chiang Mai
|
|
|
|
On-going project.
|
PAT for irrigation and crop nutrient management
|
Weather datalogger
|
Design and develop weather datalogger unit.
|
Weather datalogger system and data service system
|
Patent and commercial production
|
Panengpetch (2016)
|
Soil Chemical Sensor System (CSS)
|
Co-develop CSS.
|
CSSa, CSSb1, CSSb2
|
Start-up company
|
Grudpan et al. (2017 and 2019)
|
Agricultural resource database and DSS tool deployment
|
WRF seasonal weather forecast
|
Develop weather forecast and services
|
Seasonal weather data service system
|
Organized seasonal weather data service system
|
Chotamonsak et al. (2018)
|
Rice4cast
|
Co-deploy seasonal rice yield and production forecasts
|
Seasonal Rice yield and production forecast
|
Warming system
|
Kaeomuangmoon (2018)
|
Source: Jintrawet (2019b).
KEY PERSONNEL INVOLVED IN TRF-PA PROCESS
TRF-PA network is an innovation in ARD, consist of various key personal or users of the whole spectrum of research to improve farm situations, the next users in Government line agencies, and finally the end users which are small farms and national policy makers (Figure 2).
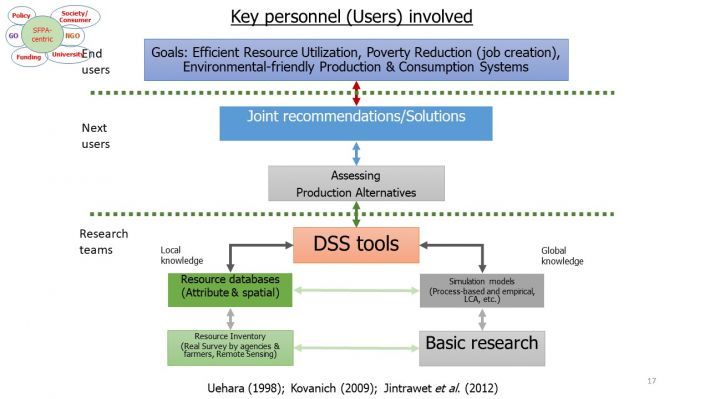
Figure 2: Three layers of key personnel (users) involved in TRF-PA process (Jintrawet, 2019a).
At the research team level, it ensures that the research team is able to integrate local and global knowledge bases as well as to take advantages of the availability of Information and Communication Technologies (ICTs) to deliver solutions. Global knowledge is based on rigorous scientific evaluation and apply to all situations, whereas local knowledge reflects the specific conditions. At this level, the Director of Agriculture Division, the TRF-PA Coordinator, growers, related line agencies staff, and researchers are actively interacting and engaging in the common goal of a given situation of a given crop production system.
At the next user level, key personnel are related line agencies staff, the TRF-PA Coordinator, growers, and the Director of Agriculture Division are involving in assessing the promising solutions or alternatives of the situations in order to scale up to a larger small farm settings with joint recommendations and/or policies.
The top level is the end user, where policy makers, the Director of TRF, and the Director of Agriculture Division-TRF are the key personnel in implementing the promising solutions or alternatives of the situations in order to scale up to a larger small farm setting.
With TRF-PA, users in all level must change to ‘new’ worldview and philosophy, from average field/production situations to the variation and interaction of those conditions, i.e., no more ‘one-size-fit-all’ policy and decision making. At the farm and field level, the farmer must their inputs to accommodate known variability of the important biotic and abiotic factors. In the agricultural research institution level, it allows users to understand the current ARD methods, teaching and training in higher education institutions may not be the most appropriate for the future or even the next season.
POLICIES PROMOTING TRF-PA INNOVATION
We have good policy to support teams to gain better understanding the impact of multivariate and multi-dimensional interactions of a given crop production system on growers/farmers, funding agencies, and agricultural researchers. These understandings enhance our collectively effort and ability to drawn sensible and useful information, leading to appropriate plan and actions.
The Agriculture Division under TRF is implementing a policy to invest in regional agricultural research hubs based on ecological principles and boundary NOT administrative boundary. These hubs are responsible to improve situations of the local and regional livelihoods using TRF-PA innovations, where growers, consumer, researchers and funding agencies could meet, brainstorm and plan to take collectively actions.
Currently, TRF-PA and the Pomology Coordinating Office is working on seven crop production systems, i.e., rice, tangerine, sweet corn, aromatic coconut, oil palm, jujube, and durian. The policy allows teams to develop PAT such as weather data logger and data delivery system and Chemical Soil Sensor Systems (CSS).
The weather data logger system is patented and under production and commercially available in a cost must lower than the imported systems, with similar functions and configured to communication in Thai language. The CSS is being registered as a ‘startup’ company at Chiang Mai University to provide services for larger demands in various crop production systems.
Currently, at least 25 higher education institutions in Thailand are offering Bachelor of Science (Agriculture) degree, with a total output of approximately 5,000 graduates per year. Agricultural education and curriculum in higher education institutions must change and proactive to produce new batch of graduates with skills to understand and handle the changes as a team with the producers, middleman, consumers and industries. Professors and would-be professors must be judged according to the standards of research ‘in’ sustainable development NOT ‘for’ sustainable development.
CONCLUSION
TRF-PA is an example of a new way of practical funding to support agricultural research and development teams to co-innovate practical precision agriculture under small farm contexts in Thailand. It has evolved to unprecedented levels of integration of various disciplines to achieve crop production efficient with PATs. These advances have resulted from three decades of system approach to agricultural research and development in Thailand, especially in Chiang Mai University and other collaborators inside and outside Thailand. It generated four major opportunities in ARD in Thailand, namely; established platforms to work with small farms as a team, focused on resource use efficiency based on data generated from PAT, co-implemented PATs to transform analog agriculture into digital agriculture, and small farms able to reach expected RUE. The network is able to promote the phrase, ‘putting the right amount of agricultural resources at the right time and place for sustainable yield & livelihood and environment based on good data sets.
ACKNOWLEDGEMENTS
TRF-PA network appreciates growers and farmers joining the teams to co-develop PAT and crop cultural practices and alternatives to improve situations. We grateful to the former (Associate Prof. Dr. Prapaporn Khopaibool) and the current (Associate Prof. Dr. Thongchai Suwonsichon) Directors of Agriculture Division of the Thailand Research Fund (TRF). Network of researchers and growers/farmers.
REFERENCES
Allen, R. G., L. S. Pereira, D. Raes and M. Smith. 1998. Crop evapotranspiration: Guidelines for computing crop water requirements. Food and Agriculture Organization (FAO) Irrigation and drainage paper 56. Rome, Italy, 300 pp.
Al-Samarraie, H. and S. Hurmuzan. 2018. A review of brainstorming techniques in higher education. Thinking Skills and Creativity, 27: 78-91.
Brundtland, G. H. 1987. Our Common Future, From One Earth to One World.
Buhler, W., S. Morse, E. Arthur, S. Bolton and J. Mann. 2002. Science Agriculture and Research: A Compromised Participation. London, U.K., Earthscan Publications Ltd. pp 176.
Chotamonsak, C, O. Wiranwetchayan, D. Lapyai, and P. Thanadolmethaphom. 2018. Seasonal Climate Forecasting Model for Rice Yield Modeling over Thailand. A final report submitted to the Thailand Research Fund, Project ID SRI5920206. 398 pp. (in Thai).
Conway, G. R. 1985. Agroecosystem analysis. Agricultural Administration, 20: 31-55.
FAO. 2013. Climate-Smart Agriculture Sourcebook. Rome, Italy, FAO. pp 570.
Gold, M.V. 1990. Sustainable Agriculture: Definitions and Terms. Accessed from https://www.nal.usda.gov/afsic/sustainable-agriculture-definitions-and-terms#toc2 on March 19, 2019.
Graeub, B. E., M. J. Chappell, H. Wittman, S. Ledermann, R. B. Kerr and B. Gemmill-Herren. 2016. The State of Family Farms in the World. World Development, 87: 1-15.
Grudpan, Kate, Wasin Wongwilai, Kitti Phojuang, Kanokwan Kiwfo, and Pheeraya Jaikang. 2017. The development of chemical sensor for analysis of plant nutrients in soil system for precision agriculture. A final report submitted to the Thailand Research Fund, Project ID SRI5920209. Xx pp. (in Thai).
Grudpan, Kate, Chalermpong Saenjum, Wasin Wongwilai Sittichai lordkaew, Tupthai Norsuwan, Kitti Phojuang, Kanokwan Kiwfo, and Pheeraya Jaikang. 2019. Development and Application of Chemical Sensor Systems for Precision Agriculture (CSS-PASS): From Spot to Spatial Data. A final report submitted to the Thailand Research Fund, Project ID SRI6120019. Xx pp. (in Thai)
Hayati, D. 2017. A Literature Review on Frameworks and Methods for Measuring and Monitoring Sustainable Agriculture. Technical Report Series GO-22-2017 Rome, Global Strategy Technical Report. 98.
Jintrawet, Attachai. 2019a. A platform for digitizing and scaling up options with small farms into SDG: A review. Forthcoming NARO-FFTC-MACRO Monograph in April 2019.
Jintrawet, Attachai. 2019b. Thailand Research Fund-Precision Agriculture Research, Development and Implementaion Network. A final Report submitted to the Thailand Research Fund, Project ID SRC6020201. Forthcoming in April 2019. (in Thai)
Jintrawet, A., C. Saengchyosawat, T. Onpraphai, M. Ekasingh, P. Gypmantasiri, B. Ekasingh, C. Narongrit, D. Kamthonkiat, K. HONDA, V. Sarawat, S. Ratanasriwong, P. Tantassanawong, S. Lertlum, A. Choulean, I. Sokrithy, P. Sampaongen, S. Laohasiriwong, K. Pannangpetch, R. Katawatin, W. Amaritsut, N. Boonbrah and T. Sathonwisit. 2012. Decision Support System Research and Development Network for Agricultural and Natural Resource Management in Thailand: A TRF-DSS Experience. Southeast Asian Studies 1:141-162.
Kaeomuangmoon, Thewin. 2018. Development of seasonal rice yield forecast system for KDML105 rice variety in Thailand. Doctoral dissertation in Agricultural Systems Management, Graduate School, Chiang Mai University, Chiang Mai, Thailand. 141 pp.
Korvanich, Amnat. 2009. A framework for Decision Support Systems research and development funding under Thailand Research Fund. page 1–8, In The Thailand Research Fund-Decision Support Systems Research and Development Network (TRF-DSS): A summary of six years achievements (2003–08), Chiang Mai: Thailand Research Fund. (in Thai).
Krone, R. 2017. Not Just Guess Work: Tips for Observation, Brainstorming, and Prototyping. Techniques in Vascular and Interventional Radiology, 20:94-100.
National Research Council. 1997. Precision Agriculture in the 21st Century: Geospatial and Information Technologies in Crop Management. Washington, DC: The National Academies Press. https://doi.org/10.17226/5491.
National Statistical Office (NSO). 2014. 2013 Agricultural Census Whole Kingdom. Agricultural Statistics Group, Social Statistics Bureau, National Statistical Office, The Government Complex, Laksi, Bangkok, 10210, Thailand. 187 pp.
Nhamo, N. and D. Chikoye. 2017. Chapter 1 - Smart Agriculture: Scope, Relevance, and Important Milestones to Date. pp 1-20, In N. Nhamo, D. Chikoye and T. Gondwe. Smart Technologies for Sustainable Smallholder Agriculture. Academic Press.
Panengpetch, K. 2016. Development of wireless weather station for precision agriculture. A final report submitted to the Thailand Research Fund, Project ID SRI5920205, 52 pp. (in Thai).
Pretty, J. 2008. Agricultural sustainability: concepts, principles and evidence. Philosophical Transactions of the Royal Society Biological Science, 363:447-465.
Rose, D. C., W. J. Sutherland, C. Parker, M. Lobley, M. Winter, C. Morris, S. Twining, C. Ffoulkes, T. Amano and L. V. Dicks. 2016. Decision support tools for agriculture: Towards effective design and delivery. Agricultural Systems 149:165-174.
Srinivasan, A. 2006. Handbook of precision agriculture: Principles and applications. New York, CRC Press. 683 pp.
To-anan, C., R.J. McGovern, R. Cheewangkoon, K. Soytong, and T. Wongwan 2019. Precision and sustainable management of citrus decline disease in Chiang Mai province. A final report submitted to the Thailand Research Fund, Project ID RDG5920052. 115 pp. (in Thai).
Uehara, G. 1998. Synthesis. pp 389-392, In G. Y. Tsuji, G. Hoogenboom and P. K. Thornton. Understanding Options for Agricultural Production. Great Britain, Kluwer Academic Publishers.
URL1, 2019. http://www.fao.org/sustainable-development-goals/indicators/241/en/
URL2, 2019. http://www.mcc.cmu.ac.th/projects/iWeatherStation/
URL3, 2019. http://rcces.soc.cmu.ac.th/climate/dssat
A paper presented at the International Symposium on Smart Agriculture for Environment and Consumer Friendly Food Production. Co-organized by National Pingtung University of Science and Technology, Taiwan Agricultural Research Institute, Taiwan, World Vegetable Center and Food and Fertilizer Technology Center for the Asian and Pacific Region, Taipei, Taiwan; at the National Pingtung University of Science and Technology, No. 1, Xuefu Road, Neipu Township, Pingtung; May 27-31, 2019.
[1] Brainstorming is a group method that provides information and understandings for a given situation from conceptual, operational and strategic dimensions. Together with prototyping, brainstorming is crucial ingredients to innovation (Krone, 2017).
Date submitted: May 28-29, 2019
Reviewed, edited and uploaded: Jun. 18, 2019
|
Thailand Research Fund-Precision Agriculture Research Network: Approach and Practices
ABSTRACT
In Thailand, agriculture is a key social activity and operate by 5.911 million small farms using 18.7 million ha and covers approximately 30% of land resources with diverse biotic and abiotic ecosystems. However, with demands for food security with environmentally friendly solutions as well as efficient resource utilization situations, it is imperative for researchers, small farms operators, consumers and policy makers to form partnership to co-develop operational and practical smart agriculture solutions for environmentally and consumer friendly food production. TRF-PA network, established in 2015 under agriculture division, aimed to transform Thai Agriculture, with Sustainable Development Goals (SDG) under Sufficiency Economy Philosophy (SEP) as our background operational framework. The network created teams of farmers and researchers to handle such situations. The teams co-designed research outputs that strengthen the capacity of the growers to solve the situation based on local and global databases as a component of various decision support system tools. This is an example of ‘SDGs Thailand model’ for enhancing livelihood of small farms with stability, prosperity and sustainability under SEP.
Keywords: Minimum Data Set, Resource Use Efficiency, immediate evidence under long-term view, Education systems.
INTRODUCTION
Sustainable and smart agriculture for environmentally and consumer friendly food production is a widely discussed topics and concerned by various stakeholder and concerned partners in global goal (URL1) and technical meetings and Conference (Hayati, 2017). The term sustainable agriculture for environmentally and consumer friendly food production begun its first appearance in 1980s with a variety of implementations. Sustainable agriculture defined as the ability of a given agricultural production system within an agroecosystem, mostly by small farms, that can return to same level of productivity after a period under stress and/or perturbations (Conway, 1985; Gold, 1990; Pretty, 2008). It is also a long-term view that research institutions and society must invest in generating and integrating wide array of knowledge and technologies to make better decisions at all societal levels to allocate limited resources to produce raw materials for food, fuel, feed, and medicine for happy family and society and health ecosystems (Brundtland, 1987; Buhler et al., 2002). However, the definition implies integrated efforts of concerned parties to maintain the ability of the system to produce a certain level of quantity, not so much on quality of the products and the environment.
The term smart agriculture for environmentally and consumer friendly food production begun its first appearance in 2010s with a variety of implementations. A more common term in recent years is ‘Climate-Smart Agriculture’, which defined as agricultural practices that sustainably improve production, resilience of production systems, and reduce greenhouse gas emissions is required to overcome climate extremes and variability (FAO, 2013; Nhamo and Chikoye, 2017). This definition implies integrated efforts of concerned parties to maintain the ability of the system to produce a certain level of quantity and maintain quality of the products and the environment, however, more resources must be allocated to improve livelihood quality of small farm communities, consumers and the environment.
Graeub et al. (2016) documented, at the global level, family farms constitute over 98% of all farms (475,067,424 holdings) and work on 53% of global agricultural land (1,009,507,053 ha out of 1,906,921,938 ha). In Thailand, agricultural census in 2013 revealed that a total of 5.911 million farm families, of which 47.5% agriculture was the only source of income. These farms operate on 18.7 million ha, some 70.0% are located in the Northeast (46.8%) and North (23.6%) regions of the country. The national average farm size was 3.2 ha per household and remained relative stable since 1993 agricultural census (NSO, 2014).
Recognizing the need to improve small farm’s livelihood with efficiency and effectiveness of agricultural and natural resource uses without causing negative impact on the environment, in October 2015 the Agriculture Division established the TRF-PA network as a coordinating office to support the Division’s efforts in identifying and prioritizing research agenda and approach to achieve the need, based on precision agriculture technology (PAT) and a series of precision agriculture prototype (PAP). We are interacting with PAP to develop and implement PATs in order to improve their situations through the full range of system approaches and improved understanding of the benefits of ecological and agronomic management, manipulation and redesign the current practices towards sustainable and smart precision agricultural production systems (Table 1). Our network focuses our resources to achieve the Sustainable Development Goals (SDG) under Sufficiency Economy Philosophy (SEP), as our background operational framework. This is a partnership of ‘SDGs Thailand model’ for enhancing livelihood of small farms with stability, properity and sustainability under SEP.
TRF-PA is a coordinating office of the Agriculture Division, a continuation of TRF-DSS network (Jintrawet et al., 2012), that uses a peer-support process and interdisciplinary in nature and based on system approaches which has been practiced in the Division. The process is flexible and links production systems to logistics and consumers, using both soft and hard system methods to gain better understandings and identify key and common situations. TRF-PA vision is ‘Thailand is a precision production agriculture country’.
Table 1: Comparison of Natural ecosystems, Sustainable-Smart TRF-PA and Other PA.
Issue
Natural ecosystems
Sustainable-Smart TRF-PA project
Other Agricultural systems
Nature of task/team work
Research questions and inputs
Research, Monitor & Evaluation process
Outputs
Outcome
Impact
Source: Conway (1985); Pretty (2008); Jintrawet (2019b).
OBJECTIVES OF TRF-PA
To achieve the vision, TRF-PA was tasked with two main objectives as follows;
TRF-PA Approach to identify and priority research agenda
The key contribution of TRF-PA is our approach into handling a given situation faced by small farms through a peer-support process (Jintrawet et al., 2012). The process is our background operational framework also based on Sustainable Development Goals (SDG) and implemented under Sufficiency Economy Philosophy (SEP). Therefore, the process allow team to focus our resources on research agenda that relevant to and directly operating by small holder producers and local networks of producers (the primary production component or the upstream component), manufacturing and logistic companies (the middle component, linking production to consumption component or the mid-stream component) and the consumers (the main user of agricultural and natural products or the downstream component).
The peer-support process is based on the principles that peers provide comments and technical knowledge to encourage research members and teams from various institutions to join in efforts to co-develop solutions with users in the real crop production situation and settings. Team members interact to achieve the common and defined goals. The group collectively defines research activities, approaches to the questions, expected PA outputs and outcomes, and proposed research budget to TRF for funding. The project workload is also defined and shared among team members. TRF-PA peer-support process consists of six interrelated steps (Figure 1).
Figure 1: TRF-PA Peer-support process for identifying and prioritizing research agenda/theme
Source: Jintrawet (2019a)
Step 1: TRF-PA and the researcher team organized a series of brainstorm[1] sessions, widely used in medical (Krone, 2017) and higher education (Al-Samarraie and Hurmuzan, 2018) situations, to gain better understandings of grower’s situation under TRF’s granting policy context. Once a common research topic has been reached, TRF-PA provides guidelines for the team to formulate a research proposal and related documents for comments from anonymous experts and approval from TRF. This step normally takes 3-6 months, then another 2-3 weeks for TRF to transfer their first six-month budget for the team to operate.
Step 2: Once TRF transferred the research fund, the research team and growers/farmers jointly implement the project and start to collect geo-referenced data sets with precision agriculture technologies (PAT). Under TRF, PATs such as weather datalogger, soil chemical sensor (CSS), and integrated weather datalogger and irrigation and fertigation systems were funded to develop affordable and reliable PATs. Data sets collected by these PATs allow communication and interaction between the researcher team and growers/farmers to improve the situations as well as support the transition into digital agriculture (REF). These unbiased data sets generate better understandings of the situation, predict consequences of a given production systems if the farmer allocate resources differently, and allow farmer to make better decisions to plan and manage limited farm resources. In addition, these data sets provide evidence to gain better understanding of the relationships of various components in the given agroecosystem, provide valuable platform to develop, calibrate and evaluate process-oriented and empirical models to capture the behaviors of the agroecosystem under various scenarios, and the partners can use the models to collaborate to allocate limited resources to improve resource use efficiency at the plot-farm level.
Step 3: The research team develops the databases and decision support system tools to provide services for users to gain access to the data sets in improve their situations (Table 2). These databases, models and DSS tools are important component of the quest for high quality and evidence-based decision making process in agriculture to improve situations, i.e., farm productivity and environmental outputs (Rose et al., 2016). Currently, observed (URL2, 2019) and simulated (URL3, 2019) weather databases are being developed and implemented for user’s access and applications. A DSS tool was designed to assist in seasonal fragrant rice yield forecasts in Thailand using simulated weather data sets, soil data sets, and CSM-CERES-Rice simulation model (Kaeomuangmoon, 2018).
Step 4: The research team and the growers/farmers participate in using data sets to analyze and synthesize appropriate choices to improve a given situation overtime and space. For example, the aromatic coconut (Sisanong et al., 2019) and the oil palm (Anothai et al., 2018) teams installed integrated irrigation and crop nutrient application systems based on data sets collected by the weather data sensors and logger and crop water requirement (Allen et al., 1998). Irrigation amount and timing for both crops was triggered by evapotranspiration calculation using data sensed by on-site weather condition sensor and logger.
Step 5: TRF Agriculture Division, TRF-PA, and the research team participate in making decision to strategically allocate resources to implement the evaluated and accepted solution for a given situation. Specifically, the integrated weather datalogger and irrigation and nutrient application system is being considered by durian, tangerine and jujube growers in major production provinces in Thailand. In addition, CCS is currently under consideration by the Thailand Rice Department to speed up a task of producing reasonable soil fertility map of 22 consolidated field, a development-oriented project initiated by the Ministry of Agriculture and Cooperatives (MOAC), in the Lower part of the Northeast of Thailand.
Step 6: The successful PATs and PAPs are systemically scaling up based on supportive policy, plan, action and sufficient resources to reach the expected state with supports from various Government agencies and individual grower and their cooperatives. This is in line with our vision that TRF-PA network had demonstrated that small farm agricultural production systems in the future is sustainable and smart based on the successful integration of a range of global knowledge and adaptive technologies for the local settings that are climate responsive and environmentally enhancing.
The peer support process has the potential to provide considerable amounts of valuable data and information for decision making in every step in ‘new’ and ‘local’ precision agriculture. Opportunities are also arising for us to collectively enhance our capacity to convert these data sets into useful suggestions to aid in the decision-making process of various key personnel of the whole spectrum of ARD.
Table 2: Aims and outputs of research projects under TRF-PA by granting group.
Project
Aim
Output
Outcome
Reference
PA Protype of crop production situation at the field level (PAP)
Tangerine deteriorating growth and yield.
Co-develop mixed cultural practices to improve the plant.
Bio-technique to improve tangerine growth and yield.
Other growers are adopting the bio-technique
To-anan et al. (2019)
Aromatic coconut
Co-develop integrated system for irrigation and nutrient management system.
Integrated system of weather station for irrigation and nutrient management system.
The grower expanding the new systems
On-going project.
Phytophthora Disease of Durian
Co-develop bio-technique to control the disease.
Bio-technique to manage the disease
On-going project.
Oil palm seedlings establishment
Co-develop integrated system for irrigation and nutrient management system.
Integrated system of weather station for irrigation and nutrient management system.
On-going project.
Jujube water and nutrients management
Co-develop integrated system for irrigation and nutrient management system.
Integrated system of weather station for irrigation and nutrient management system.
On-going project.
PA Protype of crop production situation at the provincial level (PAP) and watershed level
Organic rice suitability
Co-develop agro-informatic system for self-evaluate suitability of a plot
ORSuit an App. for farmers’ self-assessment the suitability class of his plot to join the project.
On-going project.
Antibiotics in tangerine products, Fang Watershed, Chiang Mai
On-going project.
PAT for irrigation and crop nutrient management
Weather datalogger
Design and develop weather datalogger unit.
Weather datalogger system and data service system
Patent and commercial production
Panengpetch (2016)
Soil Chemical Sensor System (CSS)
Co-develop CSS.
CSSa, CSSb1, CSSb2
Start-up company
Grudpan et al. (2017 and 2019)
Agricultural resource database and DSS tool deployment
WRF seasonal weather forecast
Develop weather forecast and services
Seasonal weather data service system
Organized seasonal weather data service system
Chotamonsak et al. (2018)
Rice4cast
Co-deploy seasonal rice yield and production forecasts
Seasonal Rice yield and production forecast
Warming system
Kaeomuangmoon (2018)
Source: Jintrawet (2019b).
KEY PERSONNEL INVOLVED IN TRF-PA PROCESS
TRF-PA network is an innovation in ARD, consist of various key personal or users of the whole spectrum of research to improve farm situations, the next users in Government line agencies, and finally the end users which are small farms and national policy makers (Figure 2).
Figure 2: Three layers of key personnel (users) involved in TRF-PA process (Jintrawet, 2019a).
At the research team level, it ensures that the research team is able to integrate local and global knowledge bases as well as to take advantages of the availability of Information and Communication Technologies (ICTs) to deliver solutions. Global knowledge is based on rigorous scientific evaluation and apply to all situations, whereas local knowledge reflects the specific conditions. At this level, the Director of Agriculture Division, the TRF-PA Coordinator, growers, related line agencies staff, and researchers are actively interacting and engaging in the common goal of a given situation of a given crop production system.
At the next user level, key personnel are related line agencies staff, the TRF-PA Coordinator, growers, and the Director of Agriculture Division are involving in assessing the promising solutions or alternatives of the situations in order to scale up to a larger small farm settings with joint recommendations and/or policies.
The top level is the end user, where policy makers, the Director of TRF, and the Director of Agriculture Division-TRF are the key personnel in implementing the promising solutions or alternatives of the situations in order to scale up to a larger small farm setting.
With TRF-PA, users in all level must change to ‘new’ worldview and philosophy, from average field/production situations to the variation and interaction of those conditions, i.e., no more ‘one-size-fit-all’ policy and decision making. At the farm and field level, the farmer must their inputs to accommodate known variability of the important biotic and abiotic factors. In the agricultural research institution level, it allows users to understand the current ARD methods, teaching and training in higher education institutions may not be the most appropriate for the future or even the next season.
POLICIES PROMOTING TRF-PA INNOVATION
We have good policy to support teams to gain better understanding the impact of multivariate and multi-dimensional interactions of a given crop production system on growers/farmers, funding agencies, and agricultural researchers. These understandings enhance our collectively effort and ability to drawn sensible and useful information, leading to appropriate plan and actions.
The Agriculture Division under TRF is implementing a policy to invest in regional agricultural research hubs based on ecological principles and boundary NOT administrative boundary. These hubs are responsible to improve situations of the local and regional livelihoods using TRF-PA innovations, where growers, consumer, researchers and funding agencies could meet, brainstorm and plan to take collectively actions.
Currently, TRF-PA and the Pomology Coordinating Office is working on seven crop production systems, i.e., rice, tangerine, sweet corn, aromatic coconut, oil palm, jujube, and durian. The policy allows teams to develop PAT such as weather data logger and data delivery system and Chemical Soil Sensor Systems (CSS).
The weather data logger system is patented and under production and commercially available in a cost must lower than the imported systems, with similar functions and configured to communication in Thai language. The CSS is being registered as a ‘startup’ company at Chiang Mai University to provide services for larger demands in various crop production systems.
Currently, at least 25 higher education institutions in Thailand are offering Bachelor of Science (Agriculture) degree, with a total output of approximately 5,000 graduates per year. Agricultural education and curriculum in higher education institutions must change and proactive to produce new batch of graduates with skills to understand and handle the changes as a team with the producers, middleman, consumers and industries. Professors and would-be professors must be judged according to the standards of research ‘in’ sustainable development NOT ‘for’ sustainable development.
CONCLUSION
TRF-PA is an example of a new way of practical funding to support agricultural research and development teams to co-innovate practical precision agriculture under small farm contexts in Thailand. It has evolved to unprecedented levels of integration of various disciplines to achieve crop production efficient with PATs. These advances have resulted from three decades of system approach to agricultural research and development in Thailand, especially in Chiang Mai University and other collaborators inside and outside Thailand. It generated four major opportunities in ARD in Thailand, namely; established platforms to work with small farms as a team, focused on resource use efficiency based on data generated from PAT, co-implemented PATs to transform analog agriculture into digital agriculture, and small farms able to reach expected RUE. The network is able to promote the phrase, ‘putting the right amount of agricultural resources at the right time and place for sustainable yield & livelihood and environment based on good data sets.
ACKNOWLEDGEMENTS
TRF-PA network appreciates growers and farmers joining the teams to co-develop PAT and crop cultural practices and alternatives to improve situations. We grateful to the former (Associate Prof. Dr. Prapaporn Khopaibool) and the current (Associate Prof. Dr. Thongchai Suwonsichon) Directors of Agriculture Division of the Thailand Research Fund (TRF). Network of researchers and growers/farmers.
REFERENCES
Allen, R. G., L. S. Pereira, D. Raes and M. Smith. 1998. Crop evapotranspiration: Guidelines for computing crop water requirements. Food and Agriculture Organization (FAO) Irrigation and drainage paper 56. Rome, Italy, 300 pp.
Al-Samarraie, H. and S. Hurmuzan. 2018. A review of brainstorming techniques in higher education. Thinking Skills and Creativity, 27: 78-91.
Brundtland, G. H. 1987. Our Common Future, From One Earth to One World.
Buhler, W., S. Morse, E. Arthur, S. Bolton and J. Mann. 2002. Science Agriculture and Research: A Compromised Participation. London, U.K., Earthscan Publications Ltd. pp 176.
Chotamonsak, C, O. Wiranwetchayan, D. Lapyai, and P. Thanadolmethaphom. 2018. Seasonal Climate Forecasting Model for Rice Yield Modeling over Thailand. A final report submitted to the Thailand Research Fund, Project ID SRI5920206. 398 pp. (in Thai).
Conway, G. R. 1985. Agroecosystem analysis. Agricultural Administration, 20: 31-55.
FAO. 2013. Climate-Smart Agriculture Sourcebook. Rome, Italy, FAO. pp 570.
Gold, M.V. 1990. Sustainable Agriculture: Definitions and Terms. Accessed from https://www.nal.usda.gov/afsic/sustainable-agriculture-definitions-and-terms#toc2 on March 19, 2019.
Graeub, B. E., M. J. Chappell, H. Wittman, S. Ledermann, R. B. Kerr and B. Gemmill-Herren. 2016. The State of Family Farms in the World. World Development, 87: 1-15.
Grudpan, Kate, Wasin Wongwilai, Kitti Phojuang, Kanokwan Kiwfo, and Pheeraya Jaikang. 2017. The development of chemical sensor for analysis of plant nutrients in soil system for precision agriculture. A final report submitted to the Thailand Research Fund, Project ID SRI5920209. Xx pp. (in Thai).
Grudpan, Kate, Chalermpong Saenjum, Wasin Wongwilai Sittichai lordkaew, Tupthai Norsuwan, Kitti Phojuang, Kanokwan Kiwfo, and Pheeraya Jaikang. 2019. Development and Application of Chemical Sensor Systems for Precision Agriculture (CSS-PASS): From Spot to Spatial Data. A final report submitted to the Thailand Research Fund, Project ID SRI6120019. Xx pp. (in Thai)
Hayati, D. 2017. A Literature Review on Frameworks and Methods for Measuring and Monitoring Sustainable Agriculture. Technical Report Series GO-22-2017 Rome, Global Strategy Technical Report. 98.
Jintrawet, Attachai. 2019a. A platform for digitizing and scaling up options with small farms into SDG: A review. Forthcoming NARO-FFTC-MACRO Monograph in April 2019.
Jintrawet, Attachai. 2019b. Thailand Research Fund-Precision Agriculture Research, Development and Implementaion Network. A final Report submitted to the Thailand Research Fund, Project ID SRC6020201. Forthcoming in April 2019. (in Thai)
Jintrawet, A., C. Saengchyosawat, T. Onpraphai, M. Ekasingh, P. Gypmantasiri, B. Ekasingh, C. Narongrit, D. Kamthonkiat, K. HONDA, V. Sarawat, S. Ratanasriwong, P. Tantassanawong, S. Lertlum, A. Choulean, I. Sokrithy, P. Sampaongen, S. Laohasiriwong, K. Pannangpetch, R. Katawatin, W. Amaritsut, N. Boonbrah and T. Sathonwisit. 2012. Decision Support System Research and Development Network for Agricultural and Natural Resource Management in Thailand: A TRF-DSS Experience. Southeast Asian Studies 1:141-162.
Kaeomuangmoon, Thewin. 2018. Development of seasonal rice yield forecast system for KDML105 rice variety in Thailand. Doctoral dissertation in Agricultural Systems Management, Graduate School, Chiang Mai University, Chiang Mai, Thailand. 141 pp.
Korvanich, Amnat. 2009. A framework for Decision Support Systems research and development funding under Thailand Research Fund. page 1–8, In The Thailand Research Fund-Decision Support Systems Research and Development Network (TRF-DSS): A summary of six years achievements (2003–08), Chiang Mai: Thailand Research Fund. (in Thai).
Krone, R. 2017. Not Just Guess Work: Tips for Observation, Brainstorming, and Prototyping. Techniques in Vascular and Interventional Radiology, 20:94-100.
National Research Council. 1997. Precision Agriculture in the 21st Century: Geospatial and Information Technologies in Crop Management. Washington, DC: The National Academies Press. https://doi.org/10.17226/5491.
National Statistical Office (NSO). 2014. 2013 Agricultural Census Whole Kingdom. Agricultural Statistics Group, Social Statistics Bureau, National Statistical Office, The Government Complex, Laksi, Bangkok, 10210, Thailand. 187 pp.
Nhamo, N. and D. Chikoye. 2017. Chapter 1 - Smart Agriculture: Scope, Relevance, and Important Milestones to Date. pp 1-20, In N. Nhamo, D. Chikoye and T. Gondwe. Smart Technologies for Sustainable Smallholder Agriculture. Academic Press.
Panengpetch, K. 2016. Development of wireless weather station for precision agriculture. A final report submitted to the Thailand Research Fund, Project ID SRI5920205, 52 pp. (in Thai).
Pretty, J. 2008. Agricultural sustainability: concepts, principles and evidence. Philosophical Transactions of the Royal Society Biological Science, 363:447-465.
Rose, D. C., W. J. Sutherland, C. Parker, M. Lobley, M. Winter, C. Morris, S. Twining, C. Ffoulkes, T. Amano and L. V. Dicks. 2016. Decision support tools for agriculture: Towards effective design and delivery. Agricultural Systems 149:165-174.
Srinivasan, A. 2006. Handbook of precision agriculture: Principles and applications. New York, CRC Press. 683 pp.
To-anan, C., R.J. McGovern, R. Cheewangkoon, K. Soytong, and T. Wongwan 2019. Precision and sustainable management of citrus decline disease in Chiang Mai province. A final report submitted to the Thailand Research Fund, Project ID RDG5920052. 115 pp. (in Thai).
Uehara, G. 1998. Synthesis. pp 389-392, In G. Y. Tsuji, G. Hoogenboom and P. K. Thornton. Understanding Options for Agricultural Production. Great Britain, Kluwer Academic Publishers.
URL1, 2019. http://www.fao.org/sustainable-development-goals/indicators/241/en/
URL2, 2019. http://www.mcc.cmu.ac.th/projects/iWeatherStation/
URL3, 2019. http://rcces.soc.cmu.ac.th/climate/dssat
A paper presented at the International Symposium on Smart Agriculture for Environment and Consumer Friendly Food Production. Co-organized by National Pingtung University of Science and Technology, Taiwan Agricultural Research Institute, Taiwan, World Vegetable Center and Food and Fertilizer Technology Center for the Asian and Pacific Region, Taipei, Taiwan; at the National Pingtung University of Science and Technology, No. 1, Xuefu Road, Neipu Township, Pingtung; May 27-31, 2019.
[1] Brainstorming is a group method that provides information and understandings for a given situation from conceptual, operational and strategic dimensions. Together with prototyping, brainstorming is crucial ingredients to innovation (Krone, 2017).
Date submitted: May 28-29, 2019
Reviewed, edited and uploaded: Jun. 18, 2019