ABSTRACT
The scale of animal farming in Japan has changed significantly over recent decades, with a move towards larger, more intensive, primarily due to market pressures. This change has resulted in demand for technologies that can reduce costs and labor inputs while increasing farm productivity. This is mainly achieved through the use of farm automation and advanced technological techniques. Modern sensor systems can be used to monitor the status of a farm and its inhabitants and thereby allow to infer the comfort levels of animals based on sensory output and this information can be used to improve the general conditions of the farm to the benefit of both animals and farmers. One of the more straightforward applications of sensor systems is related to breeding and health management. While most of the non-invasive wearable sensors (such as neck/leg mounted accelerometers) focus only on behavioral changes, some invasive wearable sensors (such as lumen and vaginal boluses) have been available for monitoring physiological changes which are important for identifying animal health status. We have recently developed two types of wearable wireless sensors: 1) for measurement of the body surface temperature at the ventral tail base (Tail Sensor) and 2) for simultaneous measurement of vaginal temperature and electric resistance (Multifunctional Vaginal Sensor) for estrus detection in cattle. The Tail Sensor could be used also for prediction of calving time and early detection of fever, such as bovine respiratory disease complex in calves and peripartum disease in dairy cows. Moreover, we try to develop early detection system for respiratory diseases in pigs using body-conducted sound. In this review paper, we introduce recent data and perspectives on wearable sensor system on our ongoing project.
Keywords: Calving time, estrus detection, fever detection, machine learning
INTRODUCTION
At present, there are 15000 dairy farms, 46300 beef farms and 4320 pig farms in Japan and they have been decreasing 3% to 4% every year. Although the total numbers of animals (1.3 million dairy cattle, 2.5 million beef cattle and 9.2 million pigs) are also slightly decreasing or have barely changed in recent years, the numbers of animals per farm are increasing every year. Therefore, the farms have grown larger and become more profit driven. The main reason for this development is the competition, market pressure and a significant reduction in the cost of products (Awasthi et al. 2016). With this framework, very few people take care to a large number of animals, hence decreasing the direct contact with them. Moreover, animal welfare may be one potential trade-off in favor of sustainable intensification (Benjamin and Yik 2019). Modern sensor systems can be used to monitor the status of a farm and its inhabitants and thereby allow to infer the comfort levels of animals based on sensory output and this information can be used to improve the general conditions of the farm to the benefit of both animals and farmers.
Recently, several dozens of sensors and sensor systems for livestock management are available. A sensor is a device that measures a physiological or behavioral parameter of individual animals and enabling automated, on-farm detection of its changes, are potent tools for health and reproductive management in the field (Fig. 1). A sensor system consists of the device itself plus the software that processes the data to produce information or advice. Sensors are categorized into two types, wearable (attached) and non-wearable (non-attached). Wearable sensors may be 'on-animal' sensors that are fitted on the outside of the body, or 'in-animal' sensors that are inside the body (e.g., rumen bolus or implant). Non-wearable sensors are 'off-animal' sensors that animals pass by, over, or through for measurement. Two distinct forms of nonattached sensors are in-line and on-line sensors. In-line sensors take measurements in a continuous flow of product from the animal. The only available option for in-line measurement is in the milk line in dairy cows. On-line sensors automatically take a sample, such as milk, that is analyzed by the sensor (Rutten et al. 2013).
Although a sensor system can be applied for techniques that measure something about the animal (e.g., activity), interpretations that summarize changes in the sensor data (e.g., increase in activity) to produce information about the animal’s status (e.g., estrus) or integration of information where sensor information is supplemented with other information (e.g., economic information) to produce advice (e.g., whether to inseminate a cow or not) (Rutten et al. 2013). Moreover, the sensor system could support famer decisions regarding the outcome of the data or make the decision automatically. Recent development of machine learning techniques used with the combination of big-data technology could also improve the quality of the predictions and prescriptions of certain events detected by sensors.
The purpose of this review paper is to describe, compare and analyze various wearable technologies that have been developed recently towards providing solutions for farmed animal health management. We also introduce recent data and perspectives on wearable sensor system on our ongoing project.
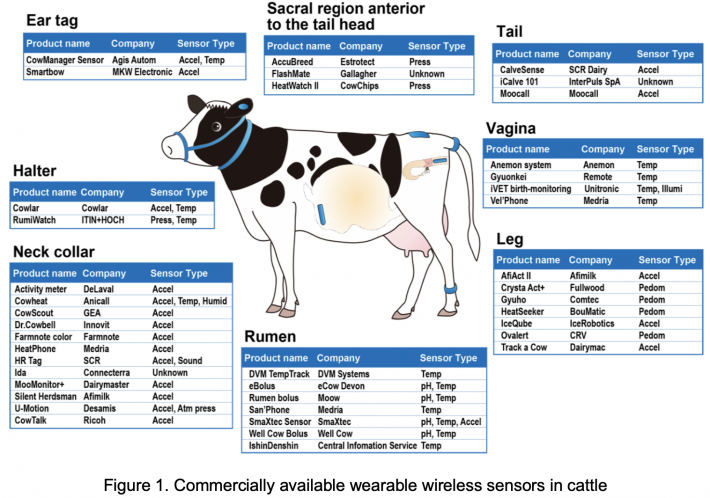
DETECTION OF DISEASES BY WEARABLE SENSORS
Modern agricultural practices and veterinary research are increasingly converging toward complete control of all environmental conditions during an animal’s life span. Physiological parameters must be precisely known and monitored continuously in order to detect the smallest changes, which will reflect physiological health and well-being of animals (Sellier et al. 2014). Sensors and wearable technologies can be implanted on animals to measure body temperature (Nogami et al. 2014, Sellier et al. 2014), observe behavior and movement (Cornou et al. 2010, Van Nuffel et al. 2015, Rahman et al. 2018), analyze sound (Ferrari et al. 2008, Berckmans et al. 2015, Lee et al. 2015) and detect pH (Mottram et al. 2008, Humer et al. 2015). Wearable sensors help farmers catch disease early, and thereby prevent deaths of animals. Farmers can also cull diseased animals in time to prevent the spread of disease in whole herds through prediction (Neethirajan 2017). Mottram (1997) reviewed potential target disease conditions in dairy cows and identified parturition, mastitis and failures in nutrition as the most significant causes of loss. Table 1 shows morbidity in adult dairy cows in the United States in 2002 and 2007 (USDA, 2010). The three most common disease conditions in cows identified by producers in 2007 were clinical mastitis, lameness, and infertility problems. According to “the number of cows having disease accidents classified by disease types” reported in Statistical Tables of Livestock Mutual Relief, 2017 in Japan, 29% of about 1,313,000 dairy cows had udder diseases, of which around 90% had mastitis, 23% had reproductive diseases and 14% had digestive diseases. Also, in the number of beef cattle having disease accidents classified by disease types, 36% of about 1,101,000 had respiratory diseases, 29% had digestive diseases and 19% had reproductive diseases. Reproductive events have to be precisely monitored to improve both the management and the economic performance of herds. Estrus and parturition are the most sensitive steps: they require time for their detection and once detected, crucial decisions, including insemination and human intervention for newborn delivery, have to be made. Thus, it is suggested that sensing technology could provide more economic benefits in those area of high cost to the farmer (fertility, metabolic disorders, mastitis, lameness and calving).
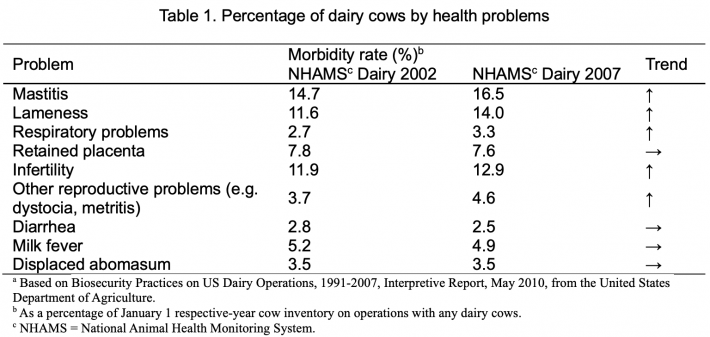
Among physiological measures, body temperature and its variations are very important indicators of the physiological health and well-being of animals. Thermoregulation is the process of maintaining body temperature homeothermy in mammals and birds. Body temperature is the most related to an important number of varied functions of the organism, such as nutrition, reproduction, activity, stress responses, and obviously preservation of health. For many years, body core temperature has been used as a reference by veterinarians and farmers to represent the basic status of the animal at rest or as a sign of disease, if abnormal. Most often, the body core temperature was easily obtained through use of a rectal thermometer. Basal rectal temperatures are quite well known within all species, and references are defined for different status of animals in order to detect many problems, primary health problems (Sellier et al. 2014). But even the relatively simple task of obtaining a temperature through rectal thermometer requires the animal to be restrained, often for more than a minute; this is likely to cause a stress response, resulting in stress-induced hyperthermia, which would decrease the likelihood of accurate assessment of body temperature (Torrao et al. 2011). Although temperature is easier to measure than other complex traits and attempts to measure body temperature of cattle have been made at various anatomical locations including rectum, ear (tympanic), vagina, reticulum-rumen, and udder (milk) (Fig. 1, Prendiville et al. 2002), there are still some complications when measuring animal’s temperatures.
TAIL SENSOR MEASURING BODY SURFACE TEMPERATURE OF VENTRAL TAIL BASE FOR DETECTION OF ESTRUS, CALVING AND FEVER IN CATTLE
The rapid development of wireless telemetry technology laid a foundation for the automatic determination of body temperature. Early in 1974, Bligh proposed the use of wireless telemetry technology to measure animal body temperature (Bligh and Heal 1974). This led to an expansion in the research of automated monitoring technology to detect dairy cow’s body temperature using infrared and implantable measurement devices. Recently, a wearable wireless sensor has been developed for measurement of the body surface temperature (ST) at the ventral tail base in calves (Nogami et al. 2013, 2014). Monitoring the ST in this way might be a gentler and less invasive procedure than measuring body core temperature by 'in-animal' sensors. Now, we have been developing the sensor system using a wearable wireless sensor (named Tail Sensor: Fig.2), which have been improved from the sensor developed by Nogami et al. (2013).
Peripheral temperature, such as ST depends on core temperature, environmental conditions and the peripheral blood system regulation. Environmental conditions such as ambient temperature, humidity, wind, sun, shade, and air movements have a strong impact on body temperature (Sellier et al. 2014). Therefore, the variations in body temperature are dependent on a number of internal and external causes. For given thermoneutral environmental conditions, modulations of body temperature are directly and inherently subjected to circadian rhythms (Lowe et al. 2001). Thus, development of an algorithm that is able to exclude the influence of the circadian rhythm, seasonal effect, and difference from core temperature may be required for a wide and accurate analysis of changes in ST associated with certain events.
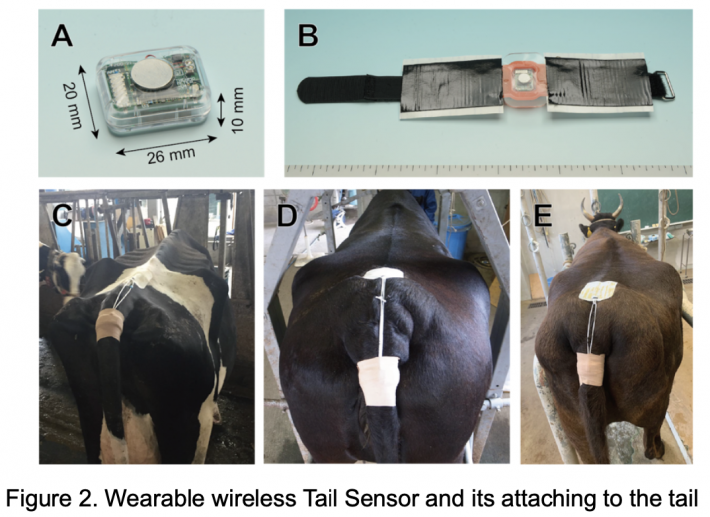
A: Tail Sensor for monitoring the body surface temperature of the ventral tail base. The size and weight of the sensor was 20 mm × 26 mm × 10 mm, weighed 5.5 g with a battery. B: Tail Sensor in an attachment unit. The sensor was inserted into a pocket formed in a silicone rubber belt and sealed with urethane gel sheet. C–E: Cows attached the sensor to their ventral tail base. The sensor was fixed on surface of the ventral tail base with a sensor attachment unit using double-sided adhesive tape and stabilize its position by surrounding hook-and-loop fastener. The attachment unit suspended from the sacral region of the animal by rubber loop attaching hook-and-loop fasteners and then wrapped with elastic medical bandages to cover.
Estrous detection in cattle
One of the more straightforward applications of sensor systems is related to breeding. This is especially important for high-value livestock such as cattle. Both dairy farming and beef production are critically dependent on breeding and each breeding opportunity is of economic significance. Many wearable sensors have been developed to aid estrous detection (Fig. 1, Mottram 2016). We attempted to monitor the ventral tail base ST using the Tail Sensor for estrous detection in cattle (Miura et al. 2017). In brief, on Day 11 of the estrous cycle (Day 0 = the day of ovulation), the sensor was attached to the surface of the ventral tail base and ST was measured every 2 min until Day 11 of the next cycle. Hourly maximum ST values were used for analysis. To exclude circadian rhythm and seasonal effects, ST changes were expressed as residual temperatures (RT = actual ST – mean ST for the same hour on the previous 3 days). Clear circadian rhythm of the ST was observed and daily changes in the ST significantly differed among seasons. However, there was no significant seasonal difference in the RT. The mean RT increased significantly ~24 h compared with ~48 h before ovulation. The mean maximum RT was 1.27 ± 0.30 °C, which was observed 5.6 ± 2.4 h after the onset of estrus, 2.4 ± 1.3 h before LH peak, and 26.9 ± 1.2 h before ovulation. We tested several threshold values derived from the standard deviation (SD) of the RT for detecting estrus. When at least two serial points of ³ +2 SD and £ –1 SD within the previous 72 h was chosen as a threshold for estrous detection, the sensitivity and accuracy values were both ³ 0.7. The ST of the ventral tail base could be monitored throughout the estrous cycle and could detect a substantial change around estrus. While this method seems to have been better in the present study, it might not be sufficient for estrous detection in the dairy or beef cattle industries.
Prediction of calving
Prediction of calving time is a key element in livestock farming; it is used to decide when to move a cow to the maternity pen (at the latest on the day pre-calving) and to decide whether human supervision is required, especially at night. Up to 17% and 51% of calvings in beef and dairy cattle, respectively, require human assistance (Saint-Dizier and Chastant-Maillard 2015). Dystocia, defined as delayed or difficult parturition, adversely affects the health of calves and dams, making human presence at the time of calving important. Calving assistance, when appropriate, is associated with a significant decrease in calf mortality, placental retention and post-partum uterine infections, together with a shorter calving to conception interval (Paolucci et al. 2010, Palombi et al. 2013). However, the growing size of dairy farms often makes this difficult. Therefore, we attempted to determine whether the ventral tail base ST measured by the Tail Sensor can be used to predict calving time in dairy cows (Koyama et al. 2018). In brief, pregnant Holstein cows were enrolled during the warm (daily average air temperature = 10–20°C, n = 13) and cool (daily average air temperature < 10°C, n = 22) seasons, and the Tail Sensor was attached to the surface of the ventral tail base of each cow 9–12 days before the predicted calving date. The ST was then measured every 2 min until 24 h after calving. Hourly maximum ST values were used in the analysis and changes in ST were expressed as RT to exclude any circadian effects. In both seasons, there was a continual decrease in RT from 24 h before calving compared with the control RT from 120 to 97 h before calving. The areas under the receiver-operating characteristic curves (ROC-AUCs) for RT as a predictor of calving were 0.88–0.95, and ROC-AUCs as a predictor of calving within 24 h were higher in the warm season than in the cool season. These findings demonstrate that calving time in dairy cows can be predicted by monitoring ST with the Tail Sensor.
Kovács et al. (2017) indicated that the decrease in ruminal temperature before calving occurs 12 h earlier in dystocia cows than in eutocia cows. Therefore, it is likely that changes in ST before calving will also be different between dystocia and eutocia cows. Unlike vaginal sensors, Tail Sensor does not fall off when the fetus is delivered and so can continue to be worn after delivery. Therefore, this technology may also have an application in postpartum body temperature management, which is important for the management of disease in dairy cows (Smith and Risco 2005). Thus, future studies should verify the usefulness of the Tail Sensor for the detection of dystocia and fever.
Fever detection in calves
All the mechanisms involved in the fight against external pathogenic aggressors, during an infection, or even locally when there is a tissue inflammation will lead to hyperthermia. Consequently, body temperature is a criterion of great interest and needs to be monitored as closely as possible, in an attempt to increase early detection and treatment of a number of economically important illnesses, such as cattle foot and mouth disease, bovine respiratory disease (BRD), horse lameness, classical swine fever virus or poultry bumblefoot (Sellier et al. 2014).
BRD among calves account for a large percentage of productivity losses in dairy and beef industries. Stress of transportation and relocation to a novel herd with other cattle is one of the factors that cause BRD. Because increases of body temperature precede other clinical symptoms of pneumonia, it is one of the most effective diagnostic parameters (Timsit et al. 2011). Thus, fever detection may be an effective tool to find early stage of BRD. We investigated changes in ST of calves after transportation to a commercial farm and their relationship with inflammation marker. Moreover, a novel indicator calculated from ST values has been developed to detect fever. In brief, 10 of 38 Holstein calves (female, 4-6 months old) transported to a commercial farm were selected and attached the Tail Sensor to ventral tail base with adhesive tape for 22 days in winter 2016. Blood samples were collected from jugular vein by venipuncture at Days 0, 7, 10, 15 and 21 post-transportation (Day 0 = the day of transportation) for measuring serum haptoglobin (Hp). Hourly Maximum ST values assessed every 30 min were used for analysis. The ST values were calculated to numerically estimate values of rectal temperatures (corrected ST) using the formulation reported by Miyamoto et al. (2016). When the corrected ST values showed more than 39.7 °C during 24 h of each day, the accumulated value for 24 h was defined as the accumulated fever value (AFV). In addition, the fever index (FI) was calculated from the AFV on 1 day and/or 2 days before the day of serum sampling. The relationship between FI and concentration of serum Hp was analyzed using ROC curve. Eight of 10 calves were considered to have fever based on the AFV on Day 5 to Day 14. When concentration of serum Hp was 500 μg /ml or more, it was determined to have inflammation and the cut-off value of FI calculated from the calculation formula ([AF on 1 day before sampling] + [AF on 2 days before sampling] × 0.5) in ROC analysis was 3.89 (sensitivity 0.79, specificity 0.96, AUC = 0.91). These results indicate that FI is a highly predictable index for fever with inflammation. It was suggested that the monitoring of ST on farm and the detection of fever using FI as an indicator was applicable to diagnose disease related to fever such as BRD and to determine necessity of treatment.
ESTROUS DETECTION BY MULTIFUNCTIONAL VAGINAL SENSOR MEASURING VAGINAL TEMPERATURE AND CONDUCTIVITY WITH SUPERVISED MACHINE LEARNING IN CATTLE
Although estrus in cattle is associated with physiological and behavioral changes (Roelofs et al. 2010, Mottram 2016), most sensors focus on estrus-accompanying/-specific behavioral changes, such as pedometer and neck/leg mounted accelerometer to monitor the increase in physical activity and rump-fixed pressure sensor for detecting the standing-to-be-mounted behavior (Roelofs and van Erp-van der Kooij 2015, Mottram 2016). However, behavioral expression of estrus is decreased by numerous biological and management factors (Roelofs et al. 2010, Saint‐Dizier and Chastant‐Maillard 2012).
Recently, we developed a new wearable wireless vaginal sensor (Multifunctional Vaginal Sensor) equipped with a thermistor and a pair of ring conductivity electrodes, which can sequentially measure vaginal temperature (VT) and conductivity (VC) simultaneously (Andersson et al. 2016). We evaluated the effectiveness of estrous detection technique based on continuous measurements of VT and VC with supervised machine learning in cattle (Higaki et al. 2019). In Brief, VT and VC of 17 cows in tie-stalls were measured using Multifunctional Vaginal Sensor from Day 11 (Day 0 = ovulation day) to Day 11 of the subsequent estrous cycle at 15-min interval. After the maximum VT and VC were extracted hourly, their changes were expressed as residual VT (rVT = actual VT − mean VT for the same time on the previous 3 days) and as VC ratio (VCr = actual VC/mean VC for the same time on Day 11 to 13), respectively, and were used for analysis. Standing estrus was confirmed by testing with herd mates at 3-h interval and ovulation was determined by monitoring hourly from the end of estrus via transrectal ultrasonography. After noise reduction, features, possible to follow-up estrus-associated changes in rVT and VCr, were extracted and used for developing estrous detection models; 9 models were developed with 3 feature sets (features extracted from rVT alone, VCr alone, and combination of rVT and VCr) and 3 machine learning algorithms (decision tree: DT, support vector machine: SVM, and artificial neural network: ANN). The rVT decreased transiently a few days before estrus and a sharp increase during estrus, while the VCr increased as estrus approached, correspond (Fig. 3). VT and VC changes in concert through the estrous cycle, and combining these two physiological parameters for estrous detection should mitigate individual drawbacks (i.e., false positive and false negative detections of estrus) and thus maximize performance. Using the best model of ANN, 16 of 17 estruses were correctly detected with one false-positive (both sensitivity and precision were 0.94). Furthermore, both mean and variance of the interval from the beginning of the estrous detection alert to ovulation (27.3 ± 6.7 h, mean ± SD of 16 estruses) were not significantly different to those from the onset of standing estrus to ovulation (30.8 ± 5.8 h, n = 17), indicating that the estrus can be detected real-time by the present technique. Overall, these results demonstrate successful use of a combination of sequential data of VT and VC through supervised machine learning for estrous detection in cattle.
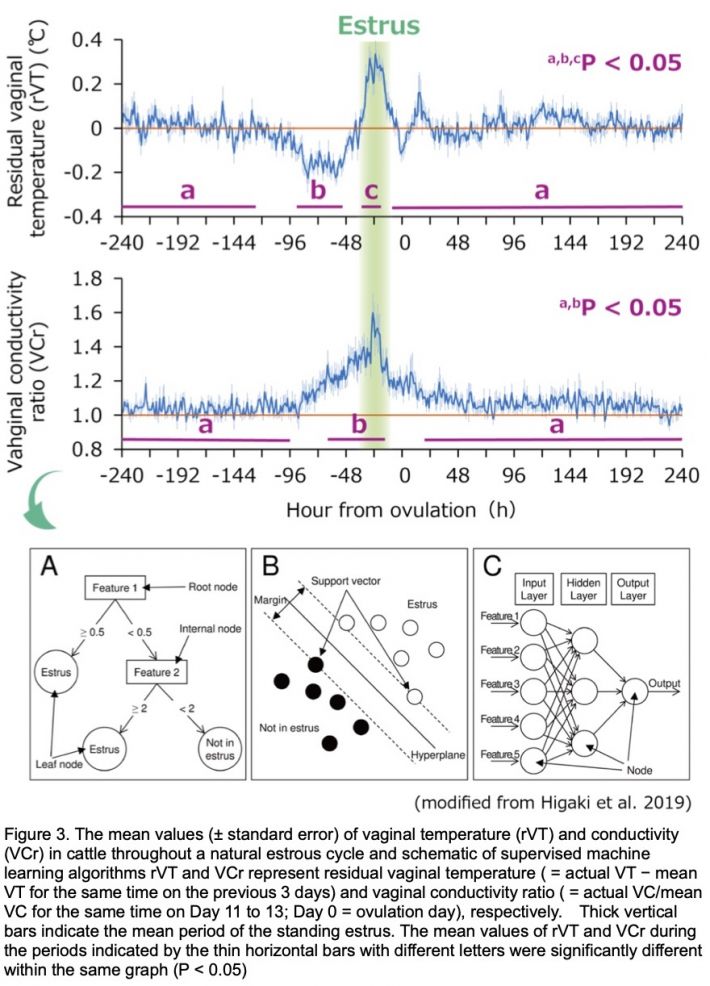
EARLY DETECTION SYSTEM FOR RESPIRATORY DISEASES IN PIGS USING BODY-CONDUCTED SOUND
Wearable sensors have not been well developed in pigs compared to cattle. The sound an animal makes can give a hint to its physical well-being. Respiratory pathologies have a high prevalence in intensive pig farming and cough is their principal symptom. It is well-known that, under intensive breeding conditions, it is very unlikely for a pig to reach the slaughter weight without having shown any kind of respiratory infection. Detection methods for respiratory diseases in group-housed pigs have been reported (Aerts et al. 2005). Moreover, it has been reported that respiratory diseases can be detected by a real-time algorithm for cough detection in controlled conditions (Exadaktylos et al. 2008) and by characterizing pig cough sounds from infected (pneumonia) piglets and non-infectious healthy piglets using real-time cough classification algorithm (Ferrari et al. 2008). In previous studies, the diagnosis of respiratory diseases has been attempted by assessing cough through sound monitoring using a microphone installed in a breeding house. This approach however is difficult to be applied in large pig houses with many animals and a possibly harmful environment. It is necessary to record the biological signal individually by attaching a sensor to each subject in the noisy environment.
We developed a recording system to detect respiratory diseases using a pig’s body-conducted sound (Narusawa et al. 2018) Body-conducted sound is used to identify biological signals, such as the heart sound of each pig, even if they are in a group. We used a piezoelectric sensor to extract the body-conducted sound and record signals from the sensor by transmitting the signals as waves in the FM band. With this system, we were able to separately record the sounds of several pigs from a distance. We verified that the recording system can record the body-conducted sounds of pigs. Moreover, we conducted an experiment to record the body-conducted sound of pigs that were inoculated with one kind of respiratory disease, porcine reproductive and respiratory syndrome virus (PRRSV) virus (Yibing et al. 2019). The body-conducted sounds were recorded before PRRSV inoculation, and 3, 5, 7 and 10 days after inoculation. As a result of acoustic analysis, there were significant differences in zero cross and Mel-frequency cepstral coefficients (MFCC) between before and after inoculation. Therefore, it is suggested that early detection of respiratory disease may be possible by assessing the acoustic features of body-conducted sounds.
ACKNOWLEDGEMENTS
This study was supported by a grant, the Research Project for the Future Agricultural Production Utilizing Artificial Intelligence (grant No. ai01), from the Project of the Bio-oriented Technology Research Advancement Institution, NARO.
REFERENCES
Aerts, J.-M., P. Jans, D. Halloy, P. Gustin, and D. Berckmans. 2005. Labeling of cough data from pigs for on-line disease monitoring by sound analysis. Transactions of the American Society of Agricultural and Biological Engineers 48: 351–354.
Andersson, L.M., H. Okada, R. Miura, Y. Zhang, K. Yoshioka, H. Aso, and T. Itoh. 2016. Wearable wireless estrus detection sensor for cows. Computers and Electronics in Agriculture 127: 101–108.
Awasthi, A., D. Riordan, and J. Walsh. 2016. Non-invasive sensor technology for the development of a dairy cattle health monitoring system. Computers 5: 23.
Benjamin, M. and S. Yik. 2019. Precision livestock farming in swine welfare: a review for swine practitioners. Animals 9: 133.
Berckmans, D., M. Hemeryck, D. Berckmans, E. Vranken, and T. van Waterschoot. 2015. Animal sound... talks! Real-time sound analysis for health monitoring in livestock, Proceedings of International Symposium on Animal Environment and Welfare 2015, pp. 215–222.
Bligh, J. and J.W. Heal. 1974. The use of radio-telemetry in the study of animal physiology. The Proceedings of the Nutrition Society 33: 173–181.
Cornou, C. and S. Lundbye-Christensen. 2010. Classification of sows’ activity types from acceleration patterns using univariate and multivariate models. Computers and Electronics in Agriculture 72: 53–60.
Exadaktylos, V., M. Silva, J.M. Aerts, C.J. Taylor, and D. Berckmans. 2008. Real-time recognition of sick pig cough sounds. Computers and Electronics in Agriculture 63: 207–214.
Ferrari, S., M. Silva, M. Guarino, J.M. Aerts, and D. Berckmans. 2008. Cough sound analysis to identify respiratory infection in pigs. Computers and Electronics in Agriculture 64: 318–325.
Gil, Z., J. Kural, J. Szarek, and E. Wierzchoś. 2001. Increase in milk and body temperature of cows as a sign of embryo entry into the uterus. Theriogenology 56: 685–697.
Higaki, S., R. Miura, T. Suda, L.M. Andersson, H. Okad, Y. Zhang, T. Itoh, F. Miwakeichi, and K. Yoshioka. 2019. Estrous detection by continuous measurements of vaginal temperature and conductivity with supervised machine learning in cattle. Theriogenology 123: 90–99.
Humer, E., K. Ghareeb, H. Harder, E. Mickdam, A. Khol-Parisini, and Q. Zebeli. 2015. Peripartal changes in reticuloruminal pH and temperature in dairy cows differing in the susceptibility to subacute rumen acidosis. Journal of Dairy Science 98: 8788–8799.
Kovács, L., F.L. Kézér, F. Ruff, and O. Szenci. 2017. Rumination time and reticuloruminal temperature as possible predictors of dystocia in dairy cows. Journal of Dairy Science 100: 1568–1579.
Koyama, K., T. Koyama, M. Sugimoto, N. Kusakari, R. Miura, K. Yoshioka, and M. Hirako. 2018. Prediction of calving time in Holstein dairy cows by monitoring the ventral tail base surface temperature. The Veterinary Journal 240: 1–5.
Lee, J., B. Noh, S. Jang, D. Park, Y. Chung, and H.H. Chang. 2015. Stress detection and classification of laying hens by sound analysis. Asian-Australasian Journal of Animal Sciences 28: 592–598.
Lewis, G.S. and S.K. Newman. 1984. Changes throughout oestrous cycles of variables that might indicate oestrus in dairy cows. Journal of Dairy Science 67: 146–152.
Lowe, T.E., C.J. Cook, J.R. Ingram, and P.J. Harris. 2001. Impact of climate on thermal rhythm in pastoral sheep. Physiology and Behaviour 74: 659–664.
Miura, R., K. Yoshioka, T. Miyamoto, H. Nogami, H. Okad, and T. Itoh. 2017. Estrous detection by monitoring ventral tail base surface temperature using a wearable wireless sensor in cattle. Animal Reproduction Science 180: 50–57.
Miyamoto, T., Y. Takahashi, T. Itoh, H. Okada, M. Oya, and Y. Aoyama. 2016. Estimation of rectal temperature from body surface temperature data in calves. Proceedings of the 159th Annual Meeting of Japanese Society of Veterinary Science p.440 (in Japanese).
Mottram, T. 1997. Automatic monitoring of the health and metabolic status of dairy cows. Livestock Production Science 48: 209–217.
Mottram, T. 2016. Animal board invited review: precision livestock farming for dairy cows with a focus on oestrus detection. Animals 10: 1575–1584.
Mottram T.T.F, J. Lowe, M. McGowan, and N. Phillips. 2008. Technical note: a wireless telemetric method of monitoring clinical acidosis in dairy cows. Computers and Electronics in Agriculture 64: 45–48.
Narusawa, K., C. Yibing, S. Ishimitsu, M. Nakayama, S. Iijima, A. Ishida, and O Mikami. 2018. Development of a respiratory disease detection system for livestock using body-conducted sound. Proceedings of the 25th International Congress on Sound and Vibration 2018 p.3590–3594.
Neethirajan, S. 2017. Recent advances in wearable sensors for animal health management. Sensing and Bio-Sensing Research 12: 15–29.
Nogami, H., H. Okada, T. Miyamoto, R. Maeda, and T. Itoh. 2013. Wearable and compact wireless sensor nodes for measuring the temperature of the base of a calf’s tail. Sensors and Materials 25: 577–582.
Nogami, H., H. Okada, T. Miyamoto, R. Maeda, and T. Itoh. 2014. Wearable wireless temperature sensor nodes appressed to base of a calf's tail. Sensors and Materials 26: 539–545.
Palombi, C., M. Paolucci, G. Stradaioli, M. Corubolo, P.B. Pascolo, and M. Monaci. 2013. Evaluation of remote monitoring of parturition in dairy cattle as a new tool for calving management. BMC Veterinary Research 9, 191.
Paolucci, M., L. Sylla, A. Di Giambattista, C. Palombi, A. Elad, G. Stradaioli, P. Pascolo, and M. Monaci. 2010. Improving calving management to further enhance reproductive performance in dairy cattle. Veterinary Research Communications 34: S37–S40.
Prendiville, D.J., J. Lowe, B. Earley, C. Spahr, and P. Kettlewell. 2002. Radiotelemetry systems for measuring body temperature. Grange Research Centre, Tunsany, Ireland (https://t-stor.teagasc.ie/bitstream/handle/11019/1358/eopr-4806.pdf?sequ...)
Rahman A, D.V. Smith, B. Little, A.B. Ingham, P.L. Greenwood, and G.J. Bishop-Hurley. 2018. Cattle behaviour classification from collar, halter, and ear tag sensors. Information and Processing in Agriculture 5: 124–133.
Roelofs, J., F. Lopez-Gatius, R. Hunter, F. Van Eerdenburg, and C. Hanzen. 2010. When is a cow in estrus? Clinical and practical aspects. Theriogenology 74: 327–344.
Roelofs, J.B. and E. van Erp-van der Kooij. 2015. Estrus detection tools and their applicability in cattle: recent and perspectival situation. Animal Reproduction 12: 498–504.
Rutten, C.J., A.G.J. Velthuis, W. Steeneveld, and H. Hogeveen. 2013. Sensors to support health management on dairy farms. Journal of Dairy Science 96: 1928–1952.
Saint‐Dizier, M. and S. Chastant‐Maillard. 2012. Towards an automated detection of oestrus in dairy cattle. Reproduction in Domestic Animal 47: 1056–1061.
Saint-Dizier, M. and S. Chastant-Maillard. 2015. Methods and on-farm devices to predict calving time in cattle. Veterinary Journal 205: 349–356.
Schaefer, A.L., N.J. Cook, C. Bench, J.B. Chabot, J. Colyn, T. Liu, E.K. Okine, M. Stewart, J.R. Webster. 2012. The non-invasive and automated detection of bovine respiratory disease onset in receiver calves using infrared thermography. Research in Veterinary Science 93: 928–935.
Sellier, N., E. Guettier, and C. Staub. 2014. A review of methods to measure animal body temperature in precision farming. American Journal of Agricultural Science and Technology 2: 74–99.
Smith, B.I. and C.A. Risco. 2005. Management of periparturient disorders in dairy cattle. Veterinary Clinics of North America: Food Animal Practice 21: 503–521.
Talukder, S., K.L. Kerrisk, L. Ingenhoff, P.C. Thomson, S.C. Garcia, and P. Celi. 2014. Infrared technology for estrus detection and as a predictor of time of ovulation in dairy cows in a pasture-based system. Theriogenology 81: 925–935.
Timsit, E., S. Assié, R. Quiniou, H. Seegers, and N. Bareille. 2011. Early detection of bovine respiratory disease in young bulls using reticulo-rumen temperature boluses. Veterinary Journal 190: 136–142.
Torrao, N.A., R.S. Hetem, L.C.R. Meyer, and L.G. Fick. 2011. Assessment of the use of temperature-sensitive microchips to determine core body temperature in goats. Veterinary Record 168: 328.
United States Department of Agriculture. 2010. Biosecurity Practices on US Dairy Operations, 1991-2007, Interpretive Report, May 2010. (https://www.aphis.usda.gov/animal_health/nahms/dairy/downloads/dairy07/D...)
Van Nuffel, A., I. Zwertvaegher, S. Van Weyenberg, M. Pastell, V.M. Thorup, C. Bahr, B. Sonck, and W. Saeys. 2015. Lameness detection in dairy cows: part 2. Use of sensors to auomatically register changes in locomotion or behavior. Animals 5: 861–885.
Wrenn, T.R., J. Bitman, and J.F. Sykes. 1958. Body Temperature variations in dairy cattle during the estrous cycle and pregnancy. Journal of Dairy Science 41: 1071–1076.
Yibing, C., K. Narusawa, S. Ishimitsu. S. Iijima, M. Nakayama, H. Inoue, and O. Mikami. 2019. Diagnosis system for respiratory diseases of pigs using body-conducted sound. Proceedings of the 2019 RISP International Workshop on Nonlinear Circuits, Communications and Signal Processing p.
Date submitted: October 24, 2019
Reviewed, edited and uploaded: November 25, 2019 |
Early Detection of Livestock Diseases by Using Wearable Wireless Sensors
ABSTRACT
The scale of animal farming in Japan has changed significantly over recent decades, with a move towards larger, more intensive, primarily due to market pressures. This change has resulted in demand for technologies that can reduce costs and labor inputs while increasing farm productivity. This is mainly achieved through the use of farm automation and advanced technological techniques. Modern sensor systems can be used to monitor the status of a farm and its inhabitants and thereby allow to infer the comfort levels of animals based on sensory output and this information can be used to improve the general conditions of the farm to the benefit of both animals and farmers. One of the more straightforward applications of sensor systems is related to breeding and health management. While most of the non-invasive wearable sensors (such as neck/leg mounted accelerometers) focus only on behavioral changes, some invasive wearable sensors (such as lumen and vaginal boluses) have been available for monitoring physiological changes which are important for identifying animal health status. We have recently developed two types of wearable wireless sensors: 1) for measurement of the body surface temperature at the ventral tail base (Tail Sensor) and 2) for simultaneous measurement of vaginal temperature and electric resistance (Multifunctional Vaginal Sensor) for estrus detection in cattle. The Tail Sensor could be used also for prediction of calving time and early detection of fever, such as bovine respiratory disease complex in calves and peripartum disease in dairy cows. Moreover, we try to develop early detection system for respiratory diseases in pigs using body-conducted sound. In this review paper, we introduce recent data and perspectives on wearable sensor system on our ongoing project.
Keywords: Calving time, estrus detection, fever detection, machine learning
INTRODUCTION
At present, there are 15000 dairy farms, 46300 beef farms and 4320 pig farms in Japan and they have been decreasing 3% to 4% every year. Although the total numbers of animals (1.3 million dairy cattle, 2.5 million beef cattle and 9.2 million pigs) are also slightly decreasing or have barely changed in recent years, the numbers of animals per farm are increasing every year. Therefore, the farms have grown larger and become more profit driven. The main reason for this development is the competition, market pressure and a significant reduction in the cost of products (Awasthi et al. 2016). With this framework, very few people take care to a large number of animals, hence decreasing the direct contact with them. Moreover, animal welfare may be one potential trade-off in favor of sustainable intensification (Benjamin and Yik 2019). Modern sensor systems can be used to monitor the status of a farm and its inhabitants and thereby allow to infer the comfort levels of animals based on sensory output and this information can be used to improve the general conditions of the farm to the benefit of both animals and farmers.
Recently, several dozens of sensors and sensor systems for livestock management are available. A sensor is a device that measures a physiological or behavioral parameter of individual animals and enabling automated, on-farm detection of its changes, are potent tools for health and reproductive management in the field (Fig. 1). A sensor system consists of the device itself plus the software that processes the data to produce information or advice. Sensors are categorized into two types, wearable (attached) and non-wearable (non-attached). Wearable sensors may be 'on-animal' sensors that are fitted on the outside of the body, or 'in-animal' sensors that are inside the body (e.g., rumen bolus or implant). Non-wearable sensors are 'off-animal' sensors that animals pass by, over, or through for measurement. Two distinct forms of nonattached sensors are in-line and on-line sensors. In-line sensors take measurements in a continuous flow of product from the animal. The only available option for in-line measurement is in the milk line in dairy cows. On-line sensors automatically take a sample, such as milk, that is analyzed by the sensor (Rutten et al. 2013).
Although a sensor system can be applied for techniques that measure something about the animal (e.g., activity), interpretations that summarize changes in the sensor data (e.g., increase in activity) to produce information about the animal’s status (e.g., estrus) or integration of information where sensor information is supplemented with other information (e.g., economic information) to produce advice (e.g., whether to inseminate a cow or not) (Rutten et al. 2013). Moreover, the sensor system could support famer decisions regarding the outcome of the data or make the decision automatically. Recent development of machine learning techniques used with the combination of big-data technology could also improve the quality of the predictions and prescriptions of certain events detected by sensors.
The purpose of this review paper is to describe, compare and analyze various wearable technologies that have been developed recently towards providing solutions for farmed animal health management. We also introduce recent data and perspectives on wearable sensor system on our ongoing project.
DETECTION OF DISEASES BY WEARABLE SENSORS
Modern agricultural practices and veterinary research are increasingly converging toward complete control of all environmental conditions during an animal’s life span. Physiological parameters must be precisely known and monitored continuously in order to detect the smallest changes, which will reflect physiological health and well-being of animals (Sellier et al. 2014). Sensors and wearable technologies can be implanted on animals to measure body temperature (Nogami et al. 2014, Sellier et al. 2014), observe behavior and movement (Cornou et al. 2010, Van Nuffel et al. 2015, Rahman et al. 2018), analyze sound (Ferrari et al. 2008, Berckmans et al. 2015, Lee et al. 2015) and detect pH (Mottram et al. 2008, Humer et al. 2015). Wearable sensors help farmers catch disease early, and thereby prevent deaths of animals. Farmers can also cull diseased animals in time to prevent the spread of disease in whole herds through prediction (Neethirajan 2017). Mottram (1997) reviewed potential target disease conditions in dairy cows and identified parturition, mastitis and failures in nutrition as the most significant causes of loss. Table 1 shows morbidity in adult dairy cows in the United States in 2002 and 2007 (USDA, 2010). The three most common disease conditions in cows identified by producers in 2007 were clinical mastitis, lameness, and infertility problems. According to “the number of cows having disease accidents classified by disease types” reported in Statistical Tables of Livestock Mutual Relief, 2017 in Japan, 29% of about 1,313,000 dairy cows had udder diseases, of which around 90% had mastitis, 23% had reproductive diseases and 14% had digestive diseases. Also, in the number of beef cattle having disease accidents classified by disease types, 36% of about 1,101,000 had respiratory diseases, 29% had digestive diseases and 19% had reproductive diseases. Reproductive events have to be precisely monitored to improve both the management and the economic performance of herds. Estrus and parturition are the most sensitive steps: they require time for their detection and once detected, crucial decisions, including insemination and human intervention for newborn delivery, have to be made. Thus, it is suggested that sensing technology could provide more economic benefits in those area of high cost to the farmer (fertility, metabolic disorders, mastitis, lameness and calving).
Among physiological measures, body temperature and its variations are very important indicators of the physiological health and well-being of animals. Thermoregulation is the process of maintaining body temperature homeothermy in mammals and birds. Body temperature is the most related to an important number of varied functions of the organism, such as nutrition, reproduction, activity, stress responses, and obviously preservation of health. For many years, body core temperature has been used as a reference by veterinarians and farmers to represent the basic status of the animal at rest or as a sign of disease, if abnormal. Most often, the body core temperature was easily obtained through use of a rectal thermometer. Basal rectal temperatures are quite well known within all species, and references are defined for different status of animals in order to detect many problems, primary health problems (Sellier et al. 2014). But even the relatively simple task of obtaining a temperature through rectal thermometer requires the animal to be restrained, often for more than a minute; this is likely to cause a stress response, resulting in stress-induced hyperthermia, which would decrease the likelihood of accurate assessment of body temperature (Torrao et al. 2011). Although temperature is easier to measure than other complex traits and attempts to measure body temperature of cattle have been made at various anatomical locations including rectum, ear (tympanic), vagina, reticulum-rumen, and udder (milk) (Fig. 1, Prendiville et al. 2002), there are still some complications when measuring animal’s temperatures.
TAIL SENSOR MEASURING BODY SURFACE TEMPERATURE OF VENTRAL TAIL BASE FOR DETECTION OF ESTRUS, CALVING AND FEVER IN CATTLE
The rapid development of wireless telemetry technology laid a foundation for the automatic determination of body temperature. Early in 1974, Bligh proposed the use of wireless telemetry technology to measure animal body temperature (Bligh and Heal 1974). This led to an expansion in the research of automated monitoring technology to detect dairy cow’s body temperature using infrared and implantable measurement devices. Recently, a wearable wireless sensor has been developed for measurement of the body surface temperature (ST) at the ventral tail base in calves (Nogami et al. 2013, 2014). Monitoring the ST in this way might be a gentler and less invasive procedure than measuring body core temperature by 'in-animal' sensors. Now, we have been developing the sensor system using a wearable wireless sensor (named Tail Sensor: Fig.2), which have been improved from the sensor developed by Nogami et al. (2013).
Peripheral temperature, such as ST depends on core temperature, environmental conditions and the peripheral blood system regulation. Environmental conditions such as ambient temperature, humidity, wind, sun, shade, and air movements have a strong impact on body temperature (Sellier et al. 2014). Therefore, the variations in body temperature are dependent on a number of internal and external causes. For given thermoneutral environmental conditions, modulations of body temperature are directly and inherently subjected to circadian rhythms (Lowe et al. 2001). Thus, development of an algorithm that is able to exclude the influence of the circadian rhythm, seasonal effect, and difference from core temperature may be required for a wide and accurate analysis of changes in ST associated with certain events.
A: Tail Sensor for monitoring the body surface temperature of the ventral tail base. The size and weight of the sensor was 20 mm × 26 mm × 10 mm, weighed 5.5 g with a battery. B: Tail Sensor in an attachment unit. The sensor was inserted into a pocket formed in a silicone rubber belt and sealed with urethane gel sheet. C–E: Cows attached the sensor to their ventral tail base. The sensor was fixed on surface of the ventral tail base with a sensor attachment unit using double-sided adhesive tape and stabilize its position by surrounding hook-and-loop fastener. The attachment unit suspended from the sacral region of the animal by rubber loop attaching hook-and-loop fasteners and then wrapped with elastic medical bandages to cover.
Estrous detection in cattle
One of the more straightforward applications of sensor systems is related to breeding. This is especially important for high-value livestock such as cattle. Both dairy farming and beef production are critically dependent on breeding and each breeding opportunity is of economic significance. Many wearable sensors have been developed to aid estrous detection (Fig. 1, Mottram 2016). We attempted to monitor the ventral tail base ST using the Tail Sensor for estrous detection in cattle (Miura et al. 2017). In brief, on Day 11 of the estrous cycle (Day 0 = the day of ovulation), the sensor was attached to the surface of the ventral tail base and ST was measured every 2 min until Day 11 of the next cycle. Hourly maximum ST values were used for analysis. To exclude circadian rhythm and seasonal effects, ST changes were expressed as residual temperatures (RT = actual ST – mean ST for the same hour on the previous 3 days). Clear circadian rhythm of the ST was observed and daily changes in the ST significantly differed among seasons. However, there was no significant seasonal difference in the RT. The mean RT increased significantly ~24 h compared with ~48 h before ovulation. The mean maximum RT was 1.27 ± 0.30 °C, which was observed 5.6 ± 2.4 h after the onset of estrus, 2.4 ± 1.3 h before LH peak, and 26.9 ± 1.2 h before ovulation. We tested several threshold values derived from the standard deviation (SD) of the RT for detecting estrus. When at least two serial points of ³ +2 SD and £ –1 SD within the previous 72 h was chosen as a threshold for estrous detection, the sensitivity and accuracy values were both ³ 0.7. The ST of the ventral tail base could be monitored throughout the estrous cycle and could detect a substantial change around estrus. While this method seems to have been better in the present study, it might not be sufficient for estrous detection in the dairy or beef cattle industries.
Prediction of calving
Prediction of calving time is a key element in livestock farming; it is used to decide when to move a cow to the maternity pen (at the latest on the day pre-calving) and to decide whether human supervision is required, especially at night. Up to 17% and 51% of calvings in beef and dairy cattle, respectively, require human assistance (Saint-Dizier and Chastant-Maillard 2015). Dystocia, defined as delayed or difficult parturition, adversely affects the health of calves and dams, making human presence at the time of calving important. Calving assistance, when appropriate, is associated with a significant decrease in calf mortality, placental retention and post-partum uterine infections, together with a shorter calving to conception interval (Paolucci et al. 2010, Palombi et al. 2013). However, the growing size of dairy farms often makes this difficult. Therefore, we attempted to determine whether the ventral tail base ST measured by the Tail Sensor can be used to predict calving time in dairy cows (Koyama et al. 2018). In brief, pregnant Holstein cows were enrolled during the warm (daily average air temperature = 10–20°C, n = 13) and cool (daily average air temperature < 10°C, n = 22) seasons, and the Tail Sensor was attached to the surface of the ventral tail base of each cow 9–12 days before the predicted calving date. The ST was then measured every 2 min until 24 h after calving. Hourly maximum ST values were used in the analysis and changes in ST were expressed as RT to exclude any circadian effects. In both seasons, there was a continual decrease in RT from 24 h before calving compared with the control RT from 120 to 97 h before calving. The areas under the receiver-operating characteristic curves (ROC-AUCs) for RT as a predictor of calving were 0.88–0.95, and ROC-AUCs as a predictor of calving within 24 h were higher in the warm season than in the cool season. These findings demonstrate that calving time in dairy cows can be predicted by monitoring ST with the Tail Sensor.
Kovács et al. (2017) indicated that the decrease in ruminal temperature before calving occurs 12 h earlier in dystocia cows than in eutocia cows. Therefore, it is likely that changes in ST before calving will also be different between dystocia and eutocia cows. Unlike vaginal sensors, Tail Sensor does not fall off when the fetus is delivered and so can continue to be worn after delivery. Therefore, this technology may also have an application in postpartum body temperature management, which is important for the management of disease in dairy cows (Smith and Risco 2005). Thus, future studies should verify the usefulness of the Tail Sensor for the detection of dystocia and fever.
Fever detection in calves
All the mechanisms involved in the fight against external pathogenic aggressors, during an infection, or even locally when there is a tissue inflammation will lead to hyperthermia. Consequently, body temperature is a criterion of great interest and needs to be monitored as closely as possible, in an attempt to increase early detection and treatment of a number of economically important illnesses, such as cattle foot and mouth disease, bovine respiratory disease (BRD), horse lameness, classical swine fever virus or poultry bumblefoot (Sellier et al. 2014).
BRD among calves account for a large percentage of productivity losses in dairy and beef industries. Stress of transportation and relocation to a novel herd with other cattle is one of the factors that cause BRD. Because increases of body temperature precede other clinical symptoms of pneumonia, it is one of the most effective diagnostic parameters (Timsit et al. 2011). Thus, fever detection may be an effective tool to find early stage of BRD. We investigated changes in ST of calves after transportation to a commercial farm and their relationship with inflammation marker. Moreover, a novel indicator calculated from ST values has been developed to detect fever. In brief, 10 of 38 Holstein calves (female, 4-6 months old) transported to a commercial farm were selected and attached the Tail Sensor to ventral tail base with adhesive tape for 22 days in winter 2016. Blood samples were collected from jugular vein by venipuncture at Days 0, 7, 10, 15 and 21 post-transportation (Day 0 = the day of transportation) for measuring serum haptoglobin (Hp). Hourly Maximum ST values assessed every 30 min were used for analysis. The ST values were calculated to numerically estimate values of rectal temperatures (corrected ST) using the formulation reported by Miyamoto et al. (2016). When the corrected ST values showed more than 39.7 °C during 24 h of each day, the accumulated value for 24 h was defined as the accumulated fever value (AFV). In addition, the fever index (FI) was calculated from the AFV on 1 day and/or 2 days before the day of serum sampling. The relationship between FI and concentration of serum Hp was analyzed using ROC curve. Eight of 10 calves were considered to have fever based on the AFV on Day 5 to Day 14. When concentration of serum Hp was 500 μg /ml or more, it was determined to have inflammation and the cut-off value of FI calculated from the calculation formula ([AF on 1 day before sampling] + [AF on 2 days before sampling] × 0.5) in ROC analysis was 3.89 (sensitivity 0.79, specificity 0.96, AUC = 0.91). These results indicate that FI is a highly predictable index for fever with inflammation. It was suggested that the monitoring of ST on farm and the detection of fever using FI as an indicator was applicable to diagnose disease related to fever such as BRD and to determine necessity of treatment.
ESTROUS DETECTION BY MULTIFUNCTIONAL VAGINAL SENSOR MEASURING VAGINAL TEMPERATURE AND CONDUCTIVITY WITH SUPERVISED MACHINE LEARNING IN CATTLE
Although estrus in cattle is associated with physiological and behavioral changes (Roelofs et al. 2010, Mottram 2016), most sensors focus on estrus-accompanying/-specific behavioral changes, such as pedometer and neck/leg mounted accelerometer to monitor the increase in physical activity and rump-fixed pressure sensor for detecting the standing-to-be-mounted behavior (Roelofs and van Erp-van der Kooij 2015, Mottram 2016). However, behavioral expression of estrus is decreased by numerous biological and management factors (Roelofs et al. 2010, Saint‐Dizier and Chastant‐Maillard 2012).
Recently, we developed a new wearable wireless vaginal sensor (Multifunctional Vaginal Sensor) equipped with a thermistor and a pair of ring conductivity electrodes, which can sequentially measure vaginal temperature (VT) and conductivity (VC) simultaneously (Andersson et al. 2016). We evaluated the effectiveness of estrous detection technique based on continuous measurements of VT and VC with supervised machine learning in cattle (Higaki et al. 2019). In Brief, VT and VC of 17 cows in tie-stalls were measured using Multifunctional Vaginal Sensor from Day 11 (Day 0 = ovulation day) to Day 11 of the subsequent estrous cycle at 15-min interval. After the maximum VT and VC were extracted hourly, their changes were expressed as residual VT (rVT = actual VT − mean VT for the same time on the previous 3 days) and as VC ratio (VCr = actual VC/mean VC for the same time on Day 11 to 13), respectively, and were used for analysis. Standing estrus was confirmed by testing with herd mates at 3-h interval and ovulation was determined by monitoring hourly from the end of estrus via transrectal ultrasonography. After noise reduction, features, possible to follow-up estrus-associated changes in rVT and VCr, were extracted and used for developing estrous detection models; 9 models were developed with 3 feature sets (features extracted from rVT alone, VCr alone, and combination of rVT and VCr) and 3 machine learning algorithms (decision tree: DT, support vector machine: SVM, and artificial neural network: ANN). The rVT decreased transiently a few days before estrus and a sharp increase during estrus, while the VCr increased as estrus approached, correspond (Fig. 3). VT and VC changes in concert through the estrous cycle, and combining these two physiological parameters for estrous detection should mitigate individual drawbacks (i.e., false positive and false negative detections of estrus) and thus maximize performance. Using the best model of ANN, 16 of 17 estruses were correctly detected with one false-positive (both sensitivity and precision were 0.94). Furthermore, both mean and variance of the interval from the beginning of the estrous detection alert to ovulation (27.3 ± 6.7 h, mean ± SD of 16 estruses) were not significantly different to those from the onset of standing estrus to ovulation (30.8 ± 5.8 h, n = 17), indicating that the estrus can be detected real-time by the present technique. Overall, these results demonstrate successful use of a combination of sequential data of VT and VC through supervised machine learning for estrous detection in cattle.
EARLY DETECTION SYSTEM FOR RESPIRATORY DISEASES IN PIGS USING BODY-CONDUCTED SOUND
Wearable sensors have not been well developed in pigs compared to cattle. The sound an animal makes can give a hint to its physical well-being. Respiratory pathologies have a high prevalence in intensive pig farming and cough is their principal symptom. It is well-known that, under intensive breeding conditions, it is very unlikely for a pig to reach the slaughter weight without having shown any kind of respiratory infection. Detection methods for respiratory diseases in group-housed pigs have been reported (Aerts et al. 2005). Moreover, it has been reported that respiratory diseases can be detected by a real-time algorithm for cough detection in controlled conditions (Exadaktylos et al. 2008) and by characterizing pig cough sounds from infected (pneumonia) piglets and non-infectious healthy piglets using real-time cough classification algorithm (Ferrari et al. 2008). In previous studies, the diagnosis of respiratory diseases has been attempted by assessing cough through sound monitoring using a microphone installed in a breeding house. This approach however is difficult to be applied in large pig houses with many animals and a possibly harmful environment. It is necessary to record the biological signal individually by attaching a sensor to each subject in the noisy environment.
We developed a recording system to detect respiratory diseases using a pig’s body-conducted sound (Narusawa et al. 2018) Body-conducted sound is used to identify biological signals, such as the heart sound of each pig, even if they are in a group. We used a piezoelectric sensor to extract the body-conducted sound and record signals from the sensor by transmitting the signals as waves in the FM band. With this system, we were able to separately record the sounds of several pigs from a distance. We verified that the recording system can record the body-conducted sounds of pigs. Moreover, we conducted an experiment to record the body-conducted sound of pigs that were inoculated with one kind of respiratory disease, porcine reproductive and respiratory syndrome virus (PRRSV) virus (Yibing et al. 2019). The body-conducted sounds were recorded before PRRSV inoculation, and 3, 5, 7 and 10 days after inoculation. As a result of acoustic analysis, there were significant differences in zero cross and Mel-frequency cepstral coefficients (MFCC) between before and after inoculation. Therefore, it is suggested that early detection of respiratory disease may be possible by assessing the acoustic features of body-conducted sounds.
ACKNOWLEDGEMENTS
This study was supported by a grant, the Research Project for the Future Agricultural Production Utilizing Artificial Intelligence (grant No. ai01), from the Project of the Bio-oriented Technology Research Advancement Institution, NARO.
REFERENCES
Aerts, J.-M., P. Jans, D. Halloy, P. Gustin, and D. Berckmans. 2005. Labeling of cough data from pigs for on-line disease monitoring by sound analysis. Transactions of the American Society of Agricultural and Biological Engineers 48: 351–354.
Andersson, L.M., H. Okada, R. Miura, Y. Zhang, K. Yoshioka, H. Aso, and T. Itoh. 2016. Wearable wireless estrus detection sensor for cows. Computers and Electronics in Agriculture 127: 101–108.
Awasthi, A., D. Riordan, and J. Walsh. 2016. Non-invasive sensor technology for the development of a dairy cattle health monitoring system. Computers 5: 23.
Benjamin, M. and S. Yik. 2019. Precision livestock farming in swine welfare: a review for swine practitioners. Animals 9: 133.
Berckmans, D., M. Hemeryck, D. Berckmans, E. Vranken, and T. van Waterschoot. 2015. Animal sound... talks! Real-time sound analysis for health monitoring in livestock, Proceedings of International Symposium on Animal Environment and Welfare 2015, pp. 215–222.
Bligh, J. and J.W. Heal. 1974. The use of radio-telemetry in the study of animal physiology. The Proceedings of the Nutrition Society 33: 173–181.
Cornou, C. and S. Lundbye-Christensen. 2010. Classification of sows’ activity types from acceleration patterns using univariate and multivariate models. Computers and Electronics in Agriculture 72: 53–60.
Exadaktylos, V., M. Silva, J.M. Aerts, C.J. Taylor, and D. Berckmans. 2008. Real-time recognition of sick pig cough sounds. Computers and Electronics in Agriculture 63: 207–214.
Ferrari, S., M. Silva, M. Guarino, J.M. Aerts, and D. Berckmans. 2008. Cough sound analysis to identify respiratory infection in pigs. Computers and Electronics in Agriculture 64: 318–325.
Gil, Z., J. Kural, J. Szarek, and E. Wierzchoś. 2001. Increase in milk and body temperature of cows as a sign of embryo entry into the uterus. Theriogenology 56: 685–697.
Higaki, S., R. Miura, T. Suda, L.M. Andersson, H. Okad, Y. Zhang, T. Itoh, F. Miwakeichi, and K. Yoshioka. 2019. Estrous detection by continuous measurements of vaginal temperature and conductivity with supervised machine learning in cattle. Theriogenology 123: 90–99.
Humer, E., K. Ghareeb, H. Harder, E. Mickdam, A. Khol-Parisini, and Q. Zebeli. 2015. Peripartal changes in reticuloruminal pH and temperature in dairy cows differing in the susceptibility to subacute rumen acidosis. Journal of Dairy Science 98: 8788–8799.
Kovács, L., F.L. Kézér, F. Ruff, and O. Szenci. 2017. Rumination time and reticuloruminal temperature as possible predictors of dystocia in dairy cows. Journal of Dairy Science 100: 1568–1579.
Koyama, K., T. Koyama, M. Sugimoto, N. Kusakari, R. Miura, K. Yoshioka, and M. Hirako. 2018. Prediction of calving time in Holstein dairy cows by monitoring the ventral tail base surface temperature. The Veterinary Journal 240: 1–5.
Lee, J., B. Noh, S. Jang, D. Park, Y. Chung, and H.H. Chang. 2015. Stress detection and classification of laying hens by sound analysis. Asian-Australasian Journal of Animal Sciences 28: 592–598.
Lewis, G.S. and S.K. Newman. 1984. Changes throughout oestrous cycles of variables that might indicate oestrus in dairy cows. Journal of Dairy Science 67: 146–152.
Lowe, T.E., C.J. Cook, J.R. Ingram, and P.J. Harris. 2001. Impact of climate on thermal rhythm in pastoral sheep. Physiology and Behaviour 74: 659–664.
Miura, R., K. Yoshioka, T. Miyamoto, H. Nogami, H. Okad, and T. Itoh. 2017. Estrous detection by monitoring ventral tail base surface temperature using a wearable wireless sensor in cattle. Animal Reproduction Science 180: 50–57.
Miyamoto, T., Y. Takahashi, T. Itoh, H. Okada, M. Oya, and Y. Aoyama. 2016. Estimation of rectal temperature from body surface temperature data in calves. Proceedings of the 159th Annual Meeting of Japanese Society of Veterinary Science p.440 (in Japanese).
Mottram, T. 1997. Automatic monitoring of the health and metabolic status of dairy cows. Livestock Production Science 48: 209–217.
Mottram, T. 2016. Animal board invited review: precision livestock farming for dairy cows with a focus on oestrus detection. Animals 10: 1575–1584.
Mottram T.T.F, J. Lowe, M. McGowan, and N. Phillips. 2008. Technical note: a wireless telemetric method of monitoring clinical acidosis in dairy cows. Computers and Electronics in Agriculture 64: 45–48.
Narusawa, K., C. Yibing, S. Ishimitsu, M. Nakayama, S. Iijima, A. Ishida, and O Mikami. 2018. Development of a respiratory disease detection system for livestock using body-conducted sound. Proceedings of the 25th International Congress on Sound and Vibration 2018 p.3590–3594.
Neethirajan, S. 2017. Recent advances in wearable sensors for animal health management. Sensing and Bio-Sensing Research 12: 15–29.
Nogami, H., H. Okada, T. Miyamoto, R. Maeda, and T. Itoh. 2013. Wearable and compact wireless sensor nodes for measuring the temperature of the base of a calf’s tail. Sensors and Materials 25: 577–582.
Nogami, H., H. Okada, T. Miyamoto, R. Maeda, and T. Itoh. 2014. Wearable wireless temperature sensor nodes appressed to base of a calf's tail. Sensors and Materials 26: 539–545.
Palombi, C., M. Paolucci, G. Stradaioli, M. Corubolo, P.B. Pascolo, and M. Monaci. 2013. Evaluation of remote monitoring of parturition in dairy cattle as a new tool for calving management. BMC Veterinary Research 9, 191.
Paolucci, M., L. Sylla, A. Di Giambattista, C. Palombi, A. Elad, G. Stradaioli, P. Pascolo, and M. Monaci. 2010. Improving calving management to further enhance reproductive performance in dairy cattle. Veterinary Research Communications 34: S37–S40.
Prendiville, D.J., J. Lowe, B. Earley, C. Spahr, and P. Kettlewell. 2002. Radiotelemetry systems for measuring body temperature. Grange Research Centre, Tunsany, Ireland (https://t-stor.teagasc.ie/bitstream/handle/11019/1358/eopr-4806.pdf?sequ...)
Rahman A, D.V. Smith, B. Little, A.B. Ingham, P.L. Greenwood, and G.J. Bishop-Hurley. 2018. Cattle behaviour classification from collar, halter, and ear tag sensors. Information and Processing in Agriculture 5: 124–133.
Roelofs, J., F. Lopez-Gatius, R. Hunter, F. Van Eerdenburg, and C. Hanzen. 2010. When is a cow in estrus? Clinical and practical aspects. Theriogenology 74: 327–344.
Roelofs, J.B. and E. van Erp-van der Kooij. 2015. Estrus detection tools and their applicability in cattle: recent and perspectival situation. Animal Reproduction 12: 498–504.
Rutten, C.J., A.G.J. Velthuis, W. Steeneveld, and H. Hogeveen. 2013. Sensors to support health management on dairy farms. Journal of Dairy Science 96: 1928–1952.
Saint‐Dizier, M. and S. Chastant‐Maillard. 2012. Towards an automated detection of oestrus in dairy cattle. Reproduction in Domestic Animal 47: 1056–1061.
Saint-Dizier, M. and S. Chastant-Maillard. 2015. Methods and on-farm devices to predict calving time in cattle. Veterinary Journal 205: 349–356.
Schaefer, A.L., N.J. Cook, C. Bench, J.B. Chabot, J. Colyn, T. Liu, E.K. Okine, M. Stewart, J.R. Webster. 2012. The non-invasive and automated detection of bovine respiratory disease onset in receiver calves using infrared thermography. Research in Veterinary Science 93: 928–935.
Sellier, N., E. Guettier, and C. Staub. 2014. A review of methods to measure animal body temperature in precision farming. American Journal of Agricultural Science and Technology 2: 74–99.
Smith, B.I. and C.A. Risco. 2005. Management of periparturient disorders in dairy cattle. Veterinary Clinics of North America: Food Animal Practice 21: 503–521.
Talukder, S., K.L. Kerrisk, L. Ingenhoff, P.C. Thomson, S.C. Garcia, and P. Celi. 2014. Infrared technology for estrus detection and as a predictor of time of ovulation in dairy cows in a pasture-based system. Theriogenology 81: 925–935.
Timsit, E., S. Assié, R. Quiniou, H. Seegers, and N. Bareille. 2011. Early detection of bovine respiratory disease in young bulls using reticulo-rumen temperature boluses. Veterinary Journal 190: 136–142.
Torrao, N.A., R.S. Hetem, L.C.R. Meyer, and L.G. Fick. 2011. Assessment of the use of temperature-sensitive microchips to determine core body temperature in goats. Veterinary Record 168: 328.
United States Department of Agriculture. 2010. Biosecurity Practices on US Dairy Operations, 1991-2007, Interpretive Report, May 2010. (https://www.aphis.usda.gov/animal_health/nahms/dairy/downloads/dairy07/D...)
Van Nuffel, A., I. Zwertvaegher, S. Van Weyenberg, M. Pastell, V.M. Thorup, C. Bahr, B. Sonck, and W. Saeys. 2015. Lameness detection in dairy cows: part 2. Use of sensors to auomatically register changes in locomotion or behavior. Animals 5: 861–885.
Wrenn, T.R., J. Bitman, and J.F. Sykes. 1958. Body Temperature variations in dairy cattle during the estrous cycle and pregnancy. Journal of Dairy Science 41: 1071–1076.
Yibing, C., K. Narusawa, S. Ishimitsu. S. Iijima, M. Nakayama, H. Inoue, and O. Mikami. 2019. Diagnosis system for respiratory diseases of pigs using body-conducted sound. Proceedings of the 2019 RISP International Workshop on Nonlinear Circuits, Communications and Signal Processing p.
Reviewed, edited and uploaded: November 25, 2019