ABSTRACT
Drone-related technologies have evolved rapidly in recent years. Since the flight performance of consumer drones has improved and become cheaper, these technologies have been widely applied to agriculture studies. For instance, drone-based remote sensing has enabled the frequent monitoring of crop biomass, pest damage, and weed condition at high spatial resolution. Moreover, computer vision technologies named SfM (Structure from Motion) and MVS (Multi View Stereos) can visualize three-dimensional information on crop volume and on the roughness of the agricultural field surface. In this paper we introduce the results of the research project entitled “Strategic Innovation Program” (SIP), which evaluated crop growth based on a drone-based remote sensing method. Considering a three-dimensional model created from drone images, we reconstructed the unevenness of the ground surface (“Furiku”) in paddy fields affected by the 2016 Kumamoto Earthquake.
Keywords: Drone, Field management, Crop diagnosis SfM/MVS
INTRODUCTION
Currently, the major problem for Japan's agriculture is the aging and decline in the number of farmers, which is paralleled by an increase of the farmland area per farmer. In this context, the application of precision agriculture (smart agriculture) is expected to strongly improve the farming efficiency. Drone-related technologies, which have evolved rapidly in recent years, can be employed in precision agriculture. As a result, remarkable price reduction and improvement of the performance of drone have been advanced, and their application in various aspects of agriculture is expected.
One of the applications of drones is farmland monitoring (e.g., crop growth, pests damage, weed condition), through the acquirement of high time frequency and spatial resolution images. Another application is the evaluation of farmland surface conditions and crop growth through the creation of a three-dimensional model. Such model can be derived from multiple aerial drone images and based on Commuter Vision technologies (i.e., SfM (Structure from Motion) and MVS (Multi View Stereos)).
In this paper, we introduce a method developed in the SIP (Strategic Innovation Program) for the evaluation of crop growth based on drone images. Moreover, we describe a sample application: a three-dimensional model of a paddy field affected by the 2016 Kumamoto Earthquake was created from drone images and its accuracy was determined by assessing the “Furiku” (unevenness of the ground surface).
APPLICATION OF DRONE REMOTE SENSING-BASED PADDY FIELD DIAGNOSTICS DEVELOPED IN THE STRATEGIC INNOVATION PROGRAM
One of the major drone applications for precision agriculture is crop diagnosis by remote sensing. In Japan, this type of studies have been previously performed by satellite remote sensing. For example, rice protein content has been evaluated in the Aomori Prefecture, leading to the production of high quality-rice of special value (Fig. 1). In that project, the authors had used satellite images obtained from optical sensors. The advantages of using this type of images are: 1) wide spatial coverage, 2) high spectral resolution, and 3) free availability of middle and low spatial resolution images; at the same time, however, image availability depends on the weather. In monsoon Asian countries (e.g., Japan) the acquisition of images through optical sensors is complicated by the occurrence of numerous rainy and cloudy days, possibly hindering crop diagnosis.
In contrast to satellite remote sensing, drone remote sensing can provide more frequent aerial images captured at appropriate times, although the flight coverage is narrower. In the context of the Strategic Innovation Program (SIP), a research project led by the Cabinet Office of Japan, our institute developed a field diagnosis method based on drone images. The targets of the SIP included rice, wheat, soybean, and other crops. In this paper, we will introduce the results of our research centered on a paddy rice field, which started with the development of appropriate image acquiring methods by drone and of a processing algorithm. Fig. 2 shows the estimated nitrogen content (related to rice quality) at the rice panicle formation stage (Inoue and Yokoyama, 2017). In order to apply precision agriculture (e.g., for the implementation of additional fertilization) it is necessary to advance the collaboration between drones and agricultural machinery. The crop diagnostics derived from drone sensing data collected over a wheat field were employed to conduct an experiment of additional fertilization (Fig. 3). In the future, we would like to improve this method and apply it to paddy rice fields.
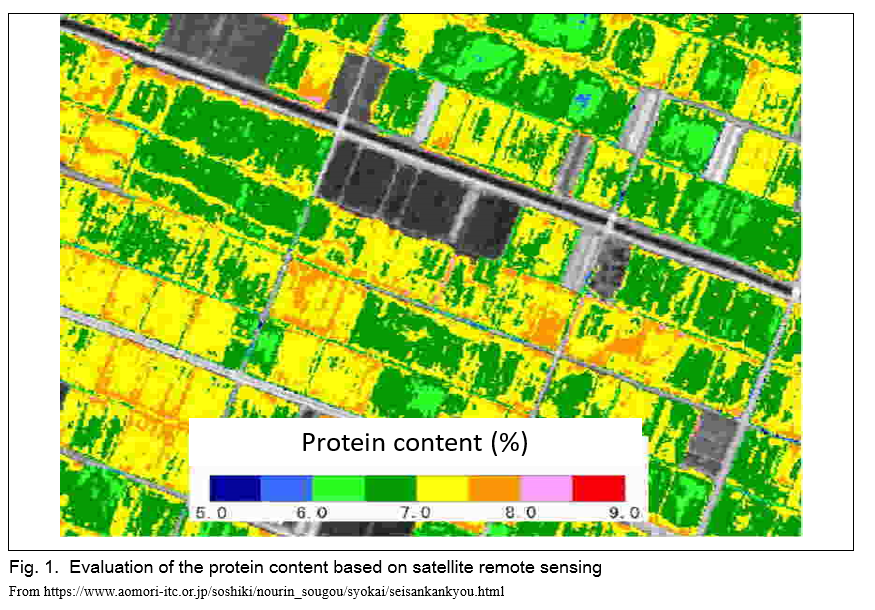
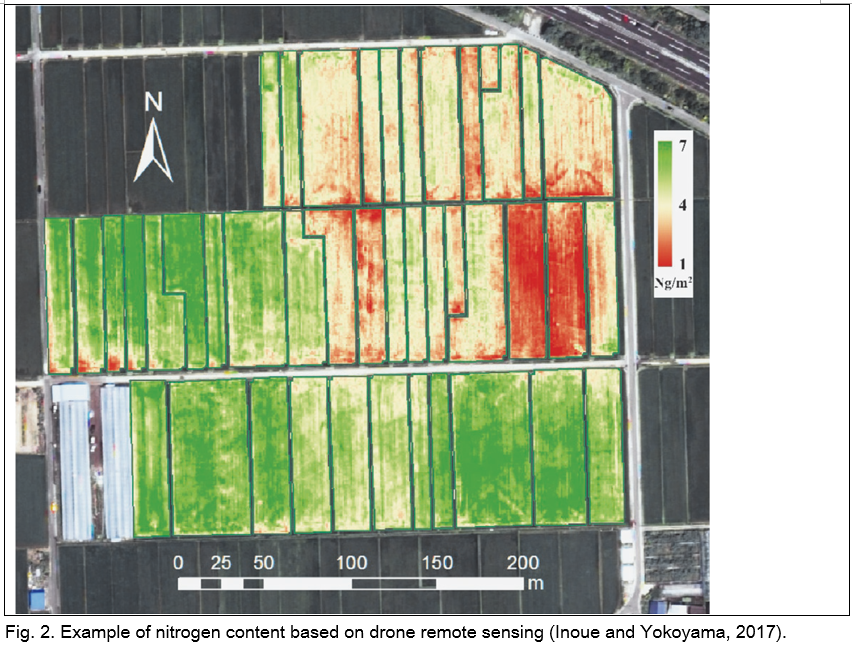
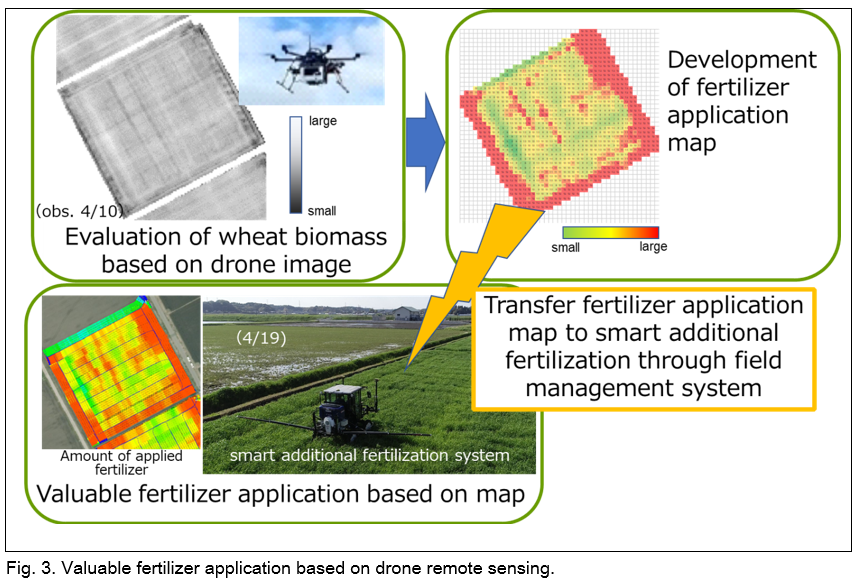
FIELD MANAGEMENT WITH THREE-DIMENSIONAL MODELS DERIVED FROM DRONE IMAGES — THE CASE STUDY OF FARMLANDS AFFECTED BY THE KUMAMOTO EARTHQUAKE
On April 14 and 16, 2016, Kumamoto prefecture was affected by a large earthquake (Mw = 7) that caused great damage (e.g., landslides, house collapses, and damages to agricultural fields). The recovery efforts in farmlands differed depending on the degree and type of damages; generally, they manifested in the form of enhanced ground surface unevenness and required a rapid and inexpensive method for their measurement. To this aim, we investigated the use of drone aerial images processed by Computer Vision (CV) techniques (i.e., Structure from Motion (SfM) and Multi View Stereo (MVS). The SfM (photogrammetric) method was used to estimate three-dimensional structures from image sequences: first, a feature point was detected in each image and matched with those identified in other images; second, the positions at which each photograph was taken were estimated. Once this process was applied to all images, we determined the relationship between all the feature points and the position/posture of the images (Fig. 4). The MVS method was used to generate a dense point cloud, based on the feature points and image data derived from the SfM (Fig. 5). Subsequently, a DSM (Digital Surface Model) was created considering the dense point cloud and the coordinates obtained from aerial photos (provided by the Geospatial Information Authority of Japan) and the simplified results of a ground-based survey. It was also possible to obtain more accurate positional information by using a RTK-GNSS receiver. The average relative height of each field was calculated from the DMS; then, the difference from the average was regarded as the unevenness of the farm fields.
The results of these analyses were used to obtain an orthorectified photo and a DSM with spatial resolutions of 2.5 cm and 5 cm, respectively, which clearly displayed the unevenness damage in the agricultural fields. In the Akitsu area (Kumamoto city) the area ratio with irregularities of ± 5 cm or more from the field average surface was of 54.6%. The degree of unevenness obtained from the drone images and SfM/MVS was accurate and did not show significant differences with that calculated from the (publicly approved) aircraft LiDAR survey. The advantage of the unevenness measurements by drone lays in their cost-effectiveness: about one-third of those from the survey. In addition, drones allow the quick and flexible observation of damages related to natural hazards (e.g., earthquakes). Overall, the results of this investigation indicated an unexpected homogeneous unevenness in the Akitsu area (Fig. 6). When the orthorectified photo and the DSM were compared with the aerial photographs taken in 1962, the main concavity matched a drainage channel: the history of the agricultural field influenced the damages reported during the Kumamoto earthquake.
In conclusion, this research confirmed the possibility of performing quick measurements and evaluating the amount of unevenness in fields using drones. Similar three-dimensional measurements may applicable not only to restoration planning, but, for example, also to crop growth monitoring and field equalization.
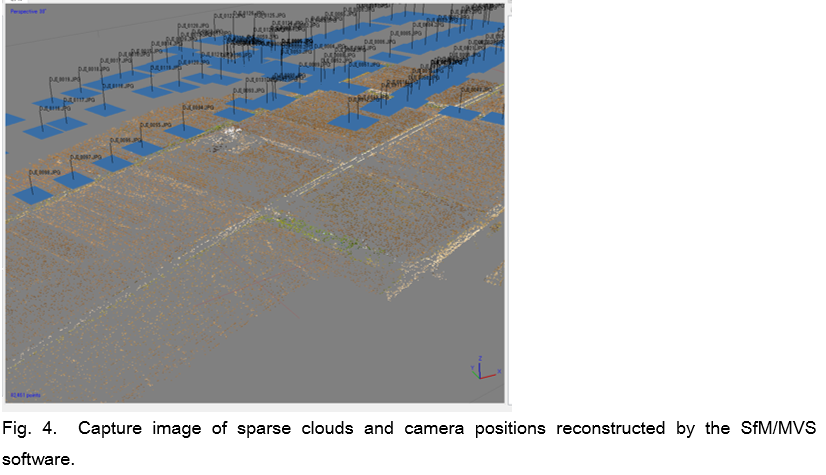
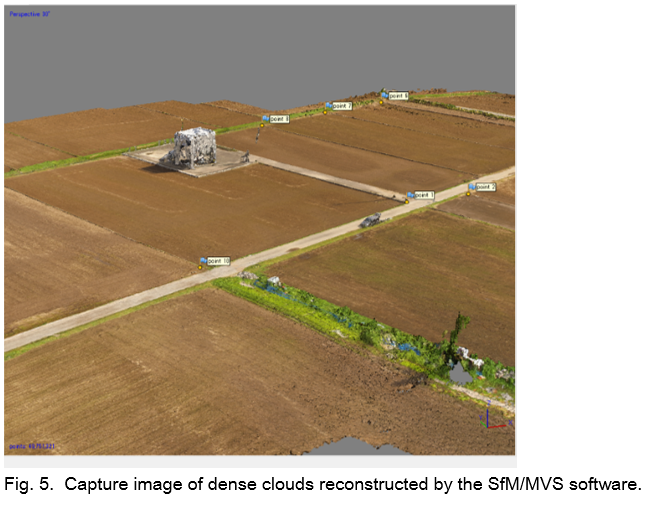
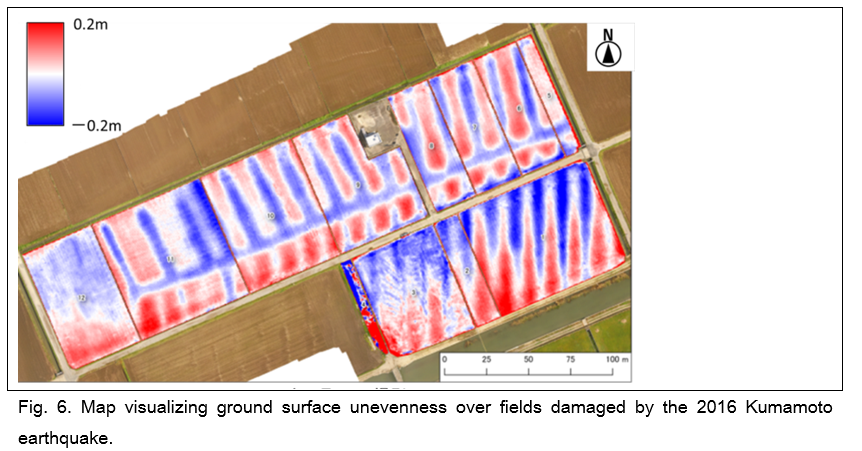
CONCLUSION
In this paper, we presented the use of drones in NARO and for the spraying of pesticides. Since high-precision RTK-GNSS receivers are becoming cheaper, it is becoming possible to perform quick and accurate measurements of unevenness and cracks in the field using drones. Such three-dimensional measurements are expected to have various applications.
The future advancement of precision agriculture will require not only the enhancement of drones, but also the development a whole new system integrating GNSS receivers, promoting IoT(Internet of Things), and connecting them.
REFERENCES
Aomori Prefecture Industrial Technology Center, (https://www.aomori-itc.or.jp/soshiki/nourin_sougou/syokai/seisankankyou.html; Accessed 17 July 2016)
Inoue, Y. and M. Yokoyama, 2017. Drone-based remote sensing of crops and soil and its application smart agriculture. Journal of the Remote Sensing Society of Japan 37(3): 224-235 (In Japanese).
Ishitsuka, N., N. Iwasaki and T. Sakamoto, 2018. Unevenness measurement of damaged paddy rice fields by the Kumamoto Earthquake using multicopper type UAV and factor investigation of the unevenness damage outbreak. Journal of the Japanese Agricultural Systems Society 34(2): 41-47 (In Japanese).
Drone Applications for Precision Agriculture and Paddy Field Management
ABSTRACT
Drone-related technologies have evolved rapidly in recent years. Since the flight performance of consumer drones has improved and become cheaper, these technologies have been widely applied to agriculture studies. For instance, drone-based remote sensing has enabled the frequent monitoring of crop biomass, pest damage, and weed condition at high spatial resolution. Moreover, computer vision technologies named SfM (Structure from Motion) and MVS (Multi View Stereos) can visualize three-dimensional information on crop volume and on the roughness of the agricultural field surface. In this paper we introduce the results of the research project entitled “Strategic Innovation Program” (SIP), which evaluated crop growth based on a drone-based remote sensing method. Considering a three-dimensional model created from drone images, we reconstructed the unevenness of the ground surface (“Furiku”) in paddy fields affected by the 2016 Kumamoto Earthquake.
Keywords: Drone, Field management, Crop diagnosis SfM/MVS
INTRODUCTION
Currently, the major problem for Japan's agriculture is the aging and decline in the number of farmers, which is paralleled by an increase of the farmland area per farmer. In this context, the application of precision agriculture (smart agriculture) is expected to strongly improve the farming efficiency. Drone-related technologies, which have evolved rapidly in recent years, can be employed in precision agriculture. As a result, remarkable price reduction and improvement of the performance of drone have been advanced, and their application in various aspects of agriculture is expected.
One of the applications of drones is farmland monitoring (e.g., crop growth, pests damage, weed condition), through the acquirement of high time frequency and spatial resolution images. Another application is the evaluation of farmland surface conditions and crop growth through the creation of a three-dimensional model. Such model can be derived from multiple aerial drone images and based on Commuter Vision technologies (i.e., SfM (Structure from Motion) and MVS (Multi View Stereos)).
In this paper, we introduce a method developed in the SIP (Strategic Innovation Program) for the evaluation of crop growth based on drone images. Moreover, we describe a sample application: a three-dimensional model of a paddy field affected by the 2016 Kumamoto Earthquake was created from drone images and its accuracy was determined by assessing the “Furiku” (unevenness of the ground surface).
APPLICATION OF DRONE REMOTE SENSING-BASED PADDY FIELD DIAGNOSTICS DEVELOPED IN THE STRATEGIC INNOVATION PROGRAM
One of the major drone applications for precision agriculture is crop diagnosis by remote sensing. In Japan, this type of studies have been previously performed by satellite remote sensing. For example, rice protein content has been evaluated in the Aomori Prefecture, leading to the production of high quality-rice of special value (Fig. 1). In that project, the authors had used satellite images obtained from optical sensors. The advantages of using this type of images are: 1) wide spatial coverage, 2) high spectral resolution, and 3) free availability of middle and low spatial resolution images; at the same time, however, image availability depends on the weather. In monsoon Asian countries (e.g., Japan) the acquisition of images through optical sensors is complicated by the occurrence of numerous rainy and cloudy days, possibly hindering crop diagnosis.
In contrast to satellite remote sensing, drone remote sensing can provide more frequent aerial images captured at appropriate times, although the flight coverage is narrower. In the context of the Strategic Innovation Program (SIP), a research project led by the Cabinet Office of Japan, our institute developed a field diagnosis method based on drone images. The targets of the SIP included rice, wheat, soybean, and other crops. In this paper, we will introduce the results of our research centered on a paddy rice field, which started with the development of appropriate image acquiring methods by drone and of a processing algorithm. Fig. 2 shows the estimated nitrogen content (related to rice quality) at the rice panicle formation stage (Inoue and Yokoyama, 2017). In order to apply precision agriculture (e.g., for the implementation of additional fertilization) it is necessary to advance the collaboration between drones and agricultural machinery. The crop diagnostics derived from drone sensing data collected over a wheat field were employed to conduct an experiment of additional fertilization (Fig. 3). In the future, we would like to improve this method and apply it to paddy rice fields.
FIELD MANAGEMENT WITH THREE-DIMENSIONAL MODELS DERIVED FROM DRONE IMAGES — THE CASE STUDY OF FARMLANDS AFFECTED BY THE KUMAMOTO EARTHQUAKE
On April 14 and 16, 2016, Kumamoto prefecture was affected by a large earthquake (Mw = 7) that caused great damage (e.g., landslides, house collapses, and damages to agricultural fields). The recovery efforts in farmlands differed depending on the degree and type of damages; generally, they manifested in the form of enhanced ground surface unevenness and required a rapid and inexpensive method for their measurement. To this aim, we investigated the use of drone aerial images processed by Computer Vision (CV) techniques (i.e., Structure from Motion (SfM) and Multi View Stereo (MVS). The SfM (photogrammetric) method was used to estimate three-dimensional structures from image sequences: first, a feature point was detected in each image and matched with those identified in other images; second, the positions at which each photograph was taken were estimated. Once this process was applied to all images, we determined the relationship between all the feature points and the position/posture of the images (Fig. 4). The MVS method was used to generate a dense point cloud, based on the feature points and image data derived from the SfM (Fig. 5). Subsequently, a DSM (Digital Surface Model) was created considering the dense point cloud and the coordinates obtained from aerial photos (provided by the Geospatial Information Authority of Japan) and the simplified results of a ground-based survey. It was also possible to obtain more accurate positional information by using a RTK-GNSS receiver. The average relative height of each field was calculated from the DMS; then, the difference from the average was regarded as the unevenness of the farm fields.
The results of these analyses were used to obtain an orthorectified photo and a DSM with spatial resolutions of 2.5 cm and 5 cm, respectively, which clearly displayed the unevenness damage in the agricultural fields. In the Akitsu area (Kumamoto city) the area ratio with irregularities of ± 5 cm or more from the field average surface was of 54.6%. The degree of unevenness obtained from the drone images and SfM/MVS was accurate and did not show significant differences with that calculated from the (publicly approved) aircraft LiDAR survey. The advantage of the unevenness measurements by drone lays in their cost-effectiveness: about one-third of those from the survey. In addition, drones allow the quick and flexible observation of damages related to natural hazards (e.g., earthquakes). Overall, the results of this investigation indicated an unexpected homogeneous unevenness in the Akitsu area (Fig. 6). When the orthorectified photo and the DSM were compared with the aerial photographs taken in 1962, the main concavity matched a drainage channel: the history of the agricultural field influenced the damages reported during the Kumamoto earthquake.
In conclusion, this research confirmed the possibility of performing quick measurements and evaluating the amount of unevenness in fields using drones. Similar three-dimensional measurements may applicable not only to restoration planning, but, for example, also to crop growth monitoring and field equalization.
CONCLUSION
In this paper, we presented the use of drones in NARO and for the spraying of pesticides. Since high-precision RTK-GNSS receivers are becoming cheaper, it is becoming possible to perform quick and accurate measurements of unevenness and cracks in the field using drones. Such three-dimensional measurements are expected to have various applications.
The future advancement of precision agriculture will require not only the enhancement of drones, but also the development a whole new system integrating GNSS receivers, promoting IoT(Internet of Things), and connecting them.
REFERENCES
Aomori Prefecture Industrial Technology Center, (https://www.aomori-itc.or.jp/soshiki/nourin_sougou/syokai/seisankankyou.html; Accessed 17 July 2016)
Inoue, Y. and M. Yokoyama, 2017. Drone-based remote sensing of crops and soil and its application smart agriculture. Journal of the Remote Sensing Society of Japan 37(3): 224-235 (In Japanese).
Ishitsuka, N., N. Iwasaki and T. Sakamoto, 2018. Unevenness measurement of damaged paddy rice fields by the Kumamoto Earthquake using multicopper type UAV and factor investigation of the unevenness damage outbreak. Journal of the Japanese Agricultural Systems Society 34(2): 41-47 (In Japanese).