ABSTRACT
Farmnote challenge started form 6 years ago in Japan. At present, we have achieved significant growth by providing herd management systems and sensors for diary and beef farm in Japan. Introduce case examples of Farmnote that provide IoT solutions for dairy and beef farm. We will show how technology can contribute to improve farmer productivity and how much you can improve farmers' earnings.
Keywords: IoT, sensor, rumination, disease
IOT DEVICES USED IN THE JAPANESE DAIRY AND LIVESTOCK INDUSTRY
The use of IoT devices is also active in the Japanese dairy and livestock industries. Cattle calve detection and heat detection system. "Mobile GYUONKEI" (Remote Co., Ltd.) (GYUONKEI, 2019), using an intravaginal body temperature sensor with a wireless sensing function, Measured changing vagina temperature in units of 0.1 ° C every 5 minutes, and stored and managed by the monitoring server via the mobile data communication network.
It is possible to more efficient management of the breeding and calve than visual management.
“ISHINDENSHIN” (Central Information Service Co., Ltd.) (ISHINDENSHIN, 2019), which performs AI learning from measurement data of lumen temperature. Predict body temperature and alert if abnormal temperature. You can also check the graph.
Moreover, the number of times of water supply can be confirmed in a graph due to the temperature drop in the lumen. You can check the fever and the number of water supply of the cow without trouble, and can take early action.
"USHIRASE" (Farmnoote Inc.) (USHIRASE, 2019) detects the difficulty in standing up in the late fattening period of beef cattle. A sensor is attached to the cow's chin, detects the difficulty in standing up the cow, and sends information to the base station installed in the barn. Notify the smartphone application when standing up is difficult. Cattle that are in a difficult-to-stand position may die from suffocation due to gas accumulation. The purpose is to reduce the number of dead heads by detecting such a situation quickly.
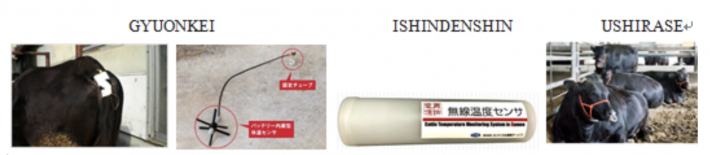
DATA ANALYSIS USING IOT (3-AXIS ACCELERATION) SENSOR
Introduction
Using the feeding management data recorded in Farmnote and the acceleration data recorded in Farmnote Color, rumination time was measured and the perinatal disease risk of Holstein was evaluated (平 勇人, 2018).
Method
Farmnote Color, a 3-axis accelerometer, was attached to about 280 heads of 300 dairy farms. The data accumulated in Farmnote Color was relayed through a gateway and sent to a database in the cloud environment, and the algorithm was analyzed on the database to classify the behavior of cattle per 24 hours into rumination, rest and activity.
The average of rumination time data acquired between August 2017 and June 2018 includes the group of cattle whose perinatal disease diagnosis was recorded after calving and within 60 days after calving(Hypocalcemia, ketosis, fatty liver, placental stagnation, postpartum fever, rumen displacement) (disease groupe). Comparison was made by dividing into groups of cattle with no disease diagnosis records (healthy groupe).
Similarly, for the group of cattle that died within 60 days after calving (dead groupe), the average perinatal rumination time was compared with that of the perinatal disease group and the healthy group.
Results
Compared with the healthy group, the ruminant time of the disease group and the dead group consistently showed a low value from 60 days before calving to the day before calving, compared with the healthy group. 26.8 minutes and 67.0 minutes shorter. The difference in rumination time between the disease group and the healthy group was 37.0 minutes (p < 0.05) shorter than the healthy group 14 days before calving. The difference in rumination time between the dead group and the healthy group was 56.0 minutes (p < 0.05) shorter than the healthy group 14 days before calving.
Discussion
The rumination time that can be obtaine with the three-axis accelerometer, Farmnote Color, showed that the risk of perinatal disease could be evaluated at the same time or earlier than the measurement of rumination time with a microphone. Moreover, it was thought that the prognosis including the risk of death after calving can be predicted by evaluating the value of decrease in rumination time in the dry period.
It was found that there was a difference between the disease group and the healthy group. In the future, we will build an AI model that distinguishes perinatal disease risk using dry season acceleration data. This is an example in which an AI model can be constructed by gathering a large amount of data from the onset group and the healthy group, instead of following the data of each cow.
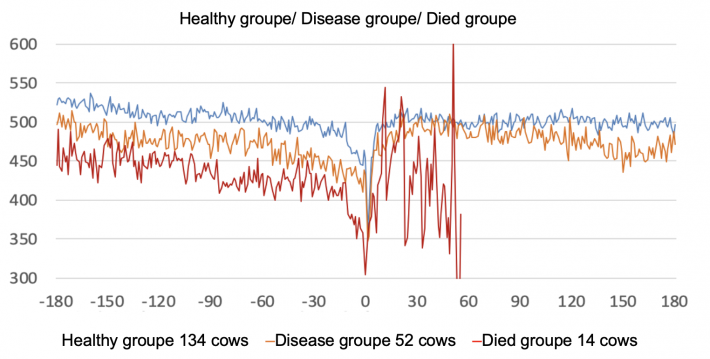
REFERENCES
GYUONKEI – Remote Co., Ltd. - http://www.gyuonkei.jp/
ISHINDENSHIN - Central Information Service Co.,Ltd http://www.cisnet.co.jp/ishindenshin.htm
USHIRASE – Farmnote Inc. - https://www.sonyengineering.co.jp/technique/products/ushirase/
平 勇人, 2018, 3軸加速度センサーFarmnote Colorが取得する反芻時間を利用したホルスタイン種の周産期疾病リスク評価, 北海道獣医師会 平成30年度北海道地区学会
Date submitted: October 24, 2019
Reviewed, edited and uploaded: November 18, 2019 |
To be Brain of Agriculture
ABSTRACT
Farmnote challenge started form 6 years ago in Japan. At present, we have achieved significant growth by providing herd management systems and sensors for diary and beef farm in Japan. Introduce case examples of Farmnote that provide IoT solutions for dairy and beef farm. We will show how technology can contribute to improve farmer productivity and how much you can improve farmers' earnings.
Keywords: IoT, sensor, rumination, disease
IOT DEVICES USED IN THE JAPANESE DAIRY AND LIVESTOCK INDUSTRY
The use of IoT devices is also active in the Japanese dairy and livestock industries. Cattle calve detection and heat detection system. "Mobile GYUONKEI" (Remote Co., Ltd.) (GYUONKEI, 2019), using an intravaginal body temperature sensor with a wireless sensing function, Measured changing vagina temperature in units of 0.1 ° C every 5 minutes, and stored and managed by the monitoring server via the mobile data communication network.
It is possible to more efficient management of the breeding and calve than visual management.
“ISHINDENSHIN” (Central Information Service Co., Ltd.) (ISHINDENSHIN, 2019), which performs AI learning from measurement data of lumen temperature. Predict body temperature and alert if abnormal temperature. You can also check the graph.
Moreover, the number of times of water supply can be confirmed in a graph due to the temperature drop in the lumen. You can check the fever and the number of water supply of the cow without trouble, and can take early action.
"USHIRASE" (Farmnoote Inc.) (USHIRASE, 2019) detects the difficulty in standing up in the late fattening period of beef cattle. A sensor is attached to the cow's chin, detects the difficulty in standing up the cow, and sends information to the base station installed in the barn. Notify the smartphone application when standing up is difficult. Cattle that are in a difficult-to-stand position may die from suffocation due to gas accumulation. The purpose is to reduce the number of dead heads by detecting such a situation quickly.
DATA ANALYSIS USING IOT (3-AXIS ACCELERATION) SENSOR
Introduction
Using the feeding management data recorded in Farmnote and the acceleration data recorded in Farmnote Color, rumination time was measured and the perinatal disease risk of Holstein was evaluated (平 勇人, 2018).
Method
Farmnote Color, a 3-axis accelerometer, was attached to about 280 heads of 300 dairy farms. The data accumulated in Farmnote Color was relayed through a gateway and sent to a database in the cloud environment, and the algorithm was analyzed on the database to classify the behavior of cattle per 24 hours into rumination, rest and activity.
The average of rumination time data acquired between August 2017 and June 2018 includes the group of cattle whose perinatal disease diagnosis was recorded after calving and within 60 days after calving(Hypocalcemia, ketosis, fatty liver, placental stagnation, postpartum fever, rumen displacement) (disease groupe). Comparison was made by dividing into groups of cattle with no disease diagnosis records (healthy groupe).
Similarly, for the group of cattle that died within 60 days after calving (dead groupe), the average perinatal rumination time was compared with that of the perinatal disease group and the healthy group.
Results
Compared with the healthy group, the ruminant time of the disease group and the dead group consistently showed a low value from 60 days before calving to the day before calving, compared with the healthy group. 26.8 minutes and 67.0 minutes shorter. The difference in rumination time between the disease group and the healthy group was 37.0 minutes (p < 0.05) shorter than the healthy group 14 days before calving. The difference in rumination time between the dead group and the healthy group was 56.0 minutes (p < 0.05) shorter than the healthy group 14 days before calving.
Discussion
The rumination time that can be obtaine with the three-axis accelerometer, Farmnote Color, showed that the risk of perinatal disease could be evaluated at the same time or earlier than the measurement of rumination time with a microphone. Moreover, it was thought that the prognosis including the risk of death after calving can be predicted by evaluating the value of decrease in rumination time in the dry period.
It was found that there was a difference between the disease group and the healthy group. In the future, we will build an AI model that distinguishes perinatal disease risk using dry season acceleration data. This is an example in which an AI model can be constructed by gathering a large amount of data from the onset group and the healthy group, instead of following the data of each cow.
REFERENCES
GYUONKEI – Remote Co., Ltd. - http://www.gyuonkei.jp/
ISHINDENSHIN - Central Information Service Co.,Ltd http://www.cisnet.co.jp/ishindenshin.htm
USHIRASE – Farmnote Inc. - https://www.sonyengineering.co.jp/technique/products/ushirase/
平 勇人, 2018, 3軸加速度センサーFarmnote Colorが取得する反芻時間を利用したホルスタイン種の周産期疾病リスク評価, 北海道獣医師会 平成30年度北海道地区学会
Reviewed, edited and uploaded: November 18, 2019